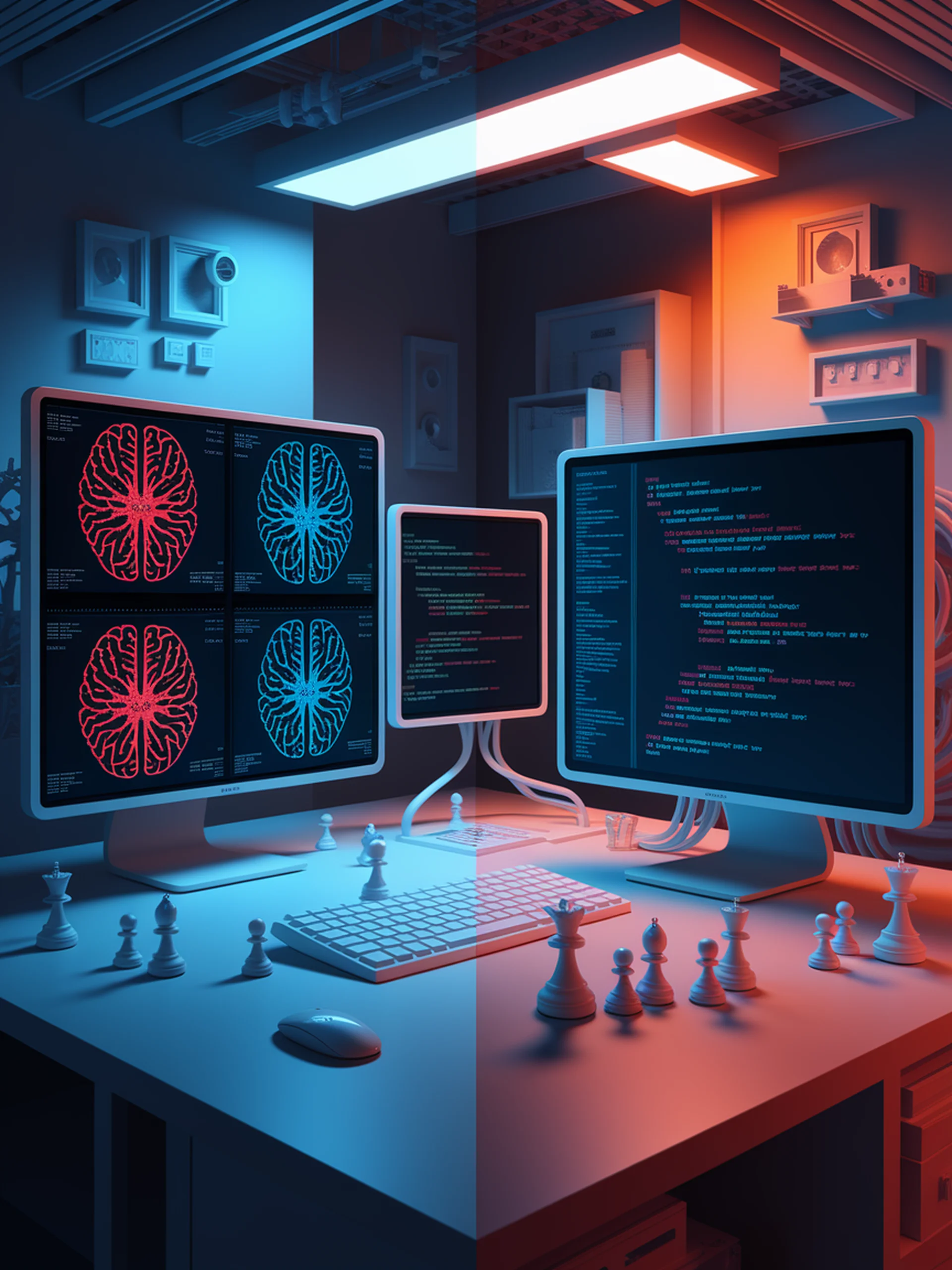
Exploiting LLM Vulnerabilities
How psychological priming techniques can bypass AI safety measures
This research reveals critical security flaws in LLMs by demonstrating novel attack strategies inspired by human psychological patterns that manipulate models into generating harmful content.
- Priming Effect: Successfully conditions LLMs to generate inappropriate responses
- Safe Attention Shift: Manipulates model attention toward harmful outputs
- Cognitive Dissonance: Exploits tension between safety measures and model capabilities
- High Success Rates: These attacks bypass existing safety mechanisms with alarming effectiveness
Why it matters: These vulnerabilities expose significant security risks in deployed LLM systems that could lead to harmful societal impacts if exploited. Understanding these weaknesses is essential for developing more robust safety mechanisms and preventing potential misuse.