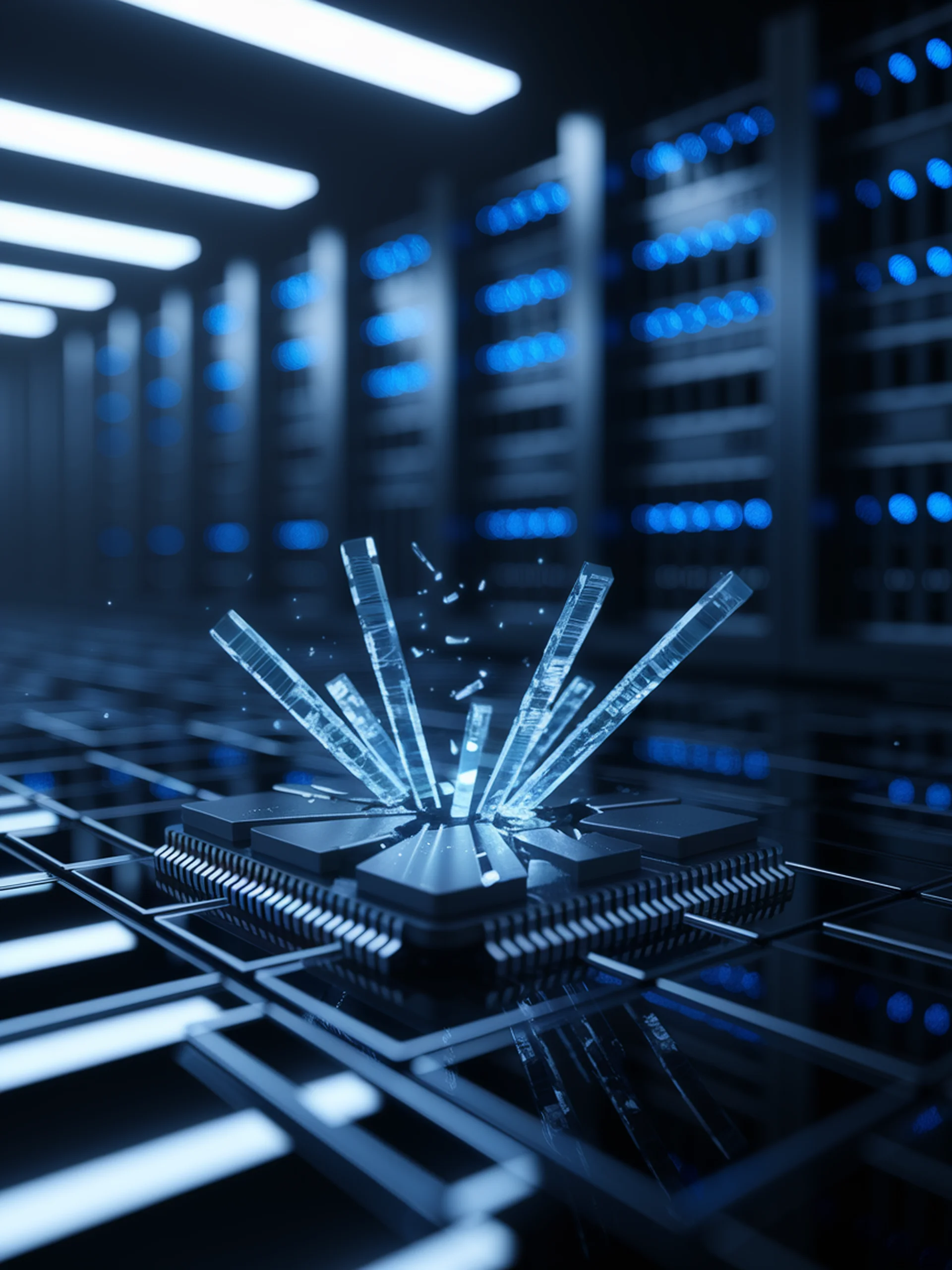
The Illusion of Forgetting in LLMs
How quantization can resurrect 'unlearned' knowledge in language models
This research reveals a critical security vulnerability in machine unlearning techniques for Large Language Models. When LLMs are quantized after unlearning, they can inadvertently recover sensitive information that was supposedly removed.
Key findings:
- Standard unlearning methods fail to truly remove sensitive knowledge when models undergo quantization
- Quantized models can restore up to 95% of supposedly 'forgotten' information
- This vulnerability creates significant security and privacy risks when handling sensitive data
- The findings challenge current approaches to removing problematic content from LLMs
For security professionals, this research highlights critical concerns about data privacy compliance and the effectiveness of current unlearning methods, requiring new approaches to truly eliminate sensitive information from language models.