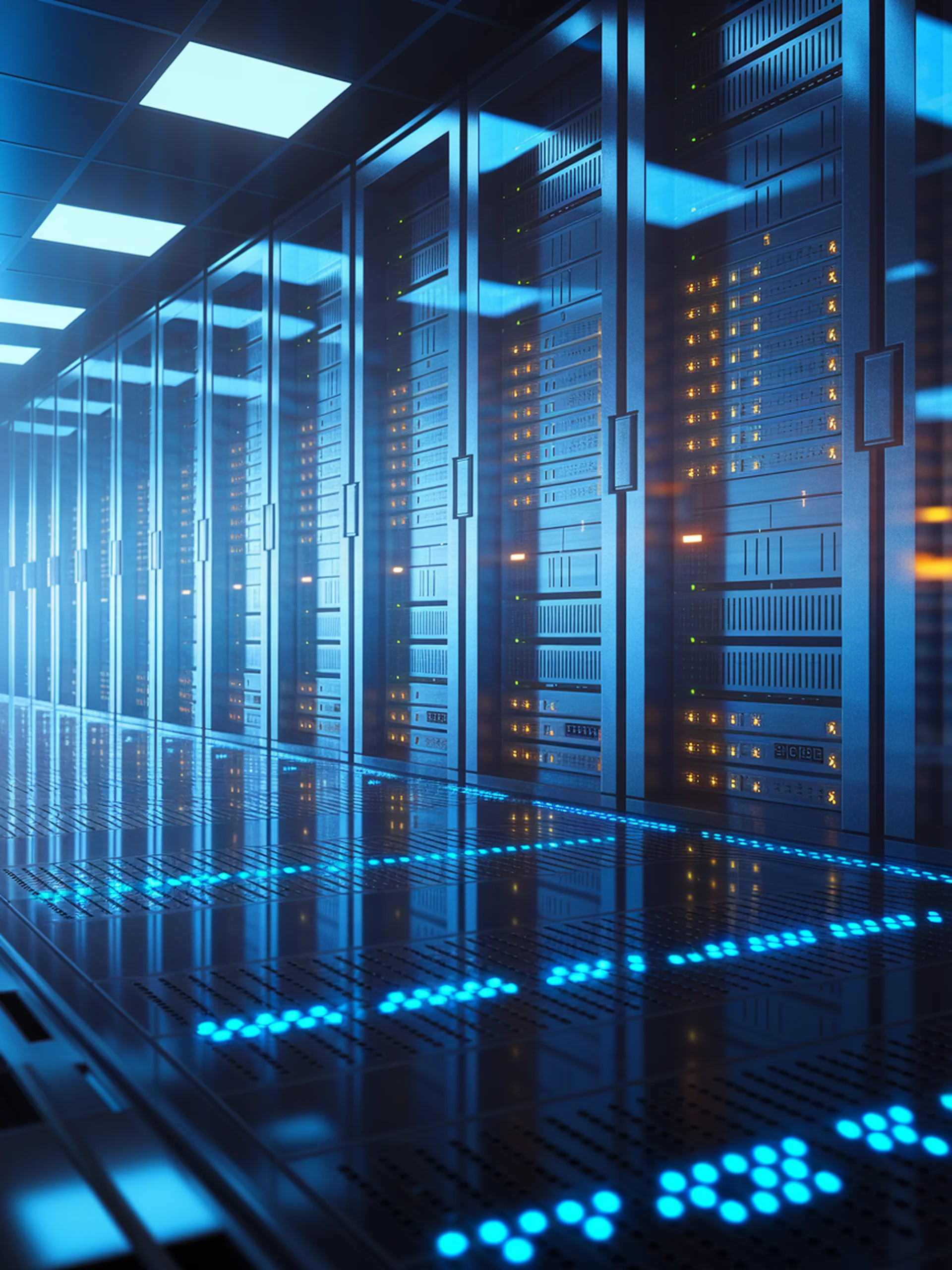
Forgetting by Design: LLM Unlearning
Selectively removing sensitive data without full retraining
Machine unlearning provides a principled approach to removing sensitive or problematic information from Large Language Models while preserving their overall utility.
- Targeted Forgetting: Enables selective removal of specific data without retraining the entire model
- Privacy Protection: Creates more secure LLMs by eliminating personal or sensitive information
- Regulatory Compliance: Helps meet emerging legal requirements for data deletion and privacy
- Efficiency: Avoids costly full retraining processes while maintaining model performance
This approach is critical for security as it allows organizations to deploy LLMs with reduced risk of exposing sensitive information or generating harmful content, while maintaining compliance with privacy regulations.
A Comprehensive Survey of Machine Unlearning Techniques for Large Language Models