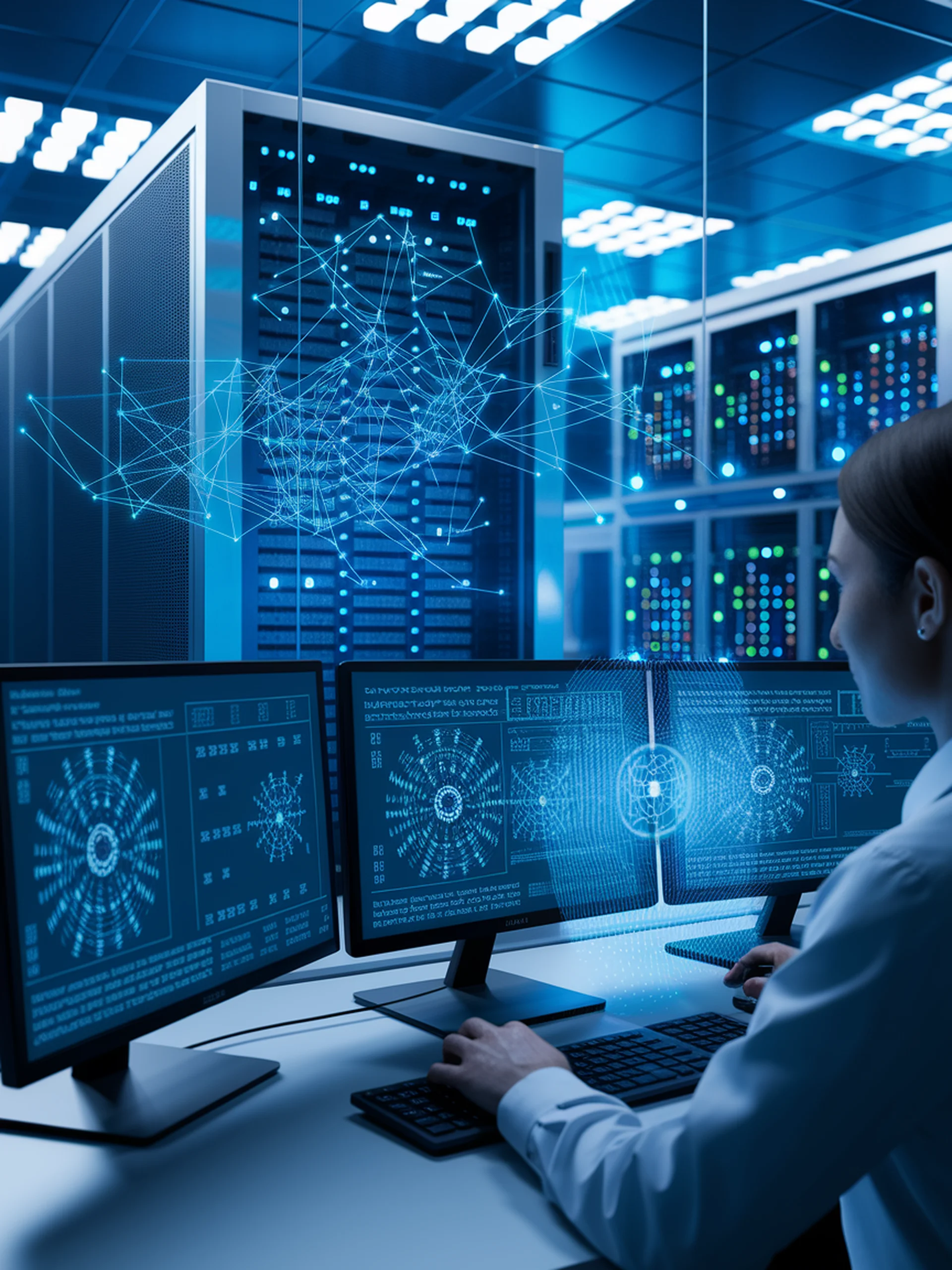
Selective Unlearning in LLMs
Efficiently Removing Sensitive Data Without Full Retraining
This research introduces techniques for selectively removing specific information from large language models without the computational burden of complete retraining.
- Addresses critical privacy and compliance challenges when handling sensitive data
- Explores global weight modification approaches for targeted knowledge removal
- Evaluates effectiveness through the SemEval 2025 Task 4 competition framework
- Balances forgetting targeted information while preserving general capabilities
These advances are crucial for cybersecurity applications as they enable organizations to comply with privacy regulations and remove security vulnerabilities without sacrificing model performance or incurring prohibitive retraining costs.
Forgotten but Not Lost: The Balancing Act of Selective Unlearning in Large Language Models