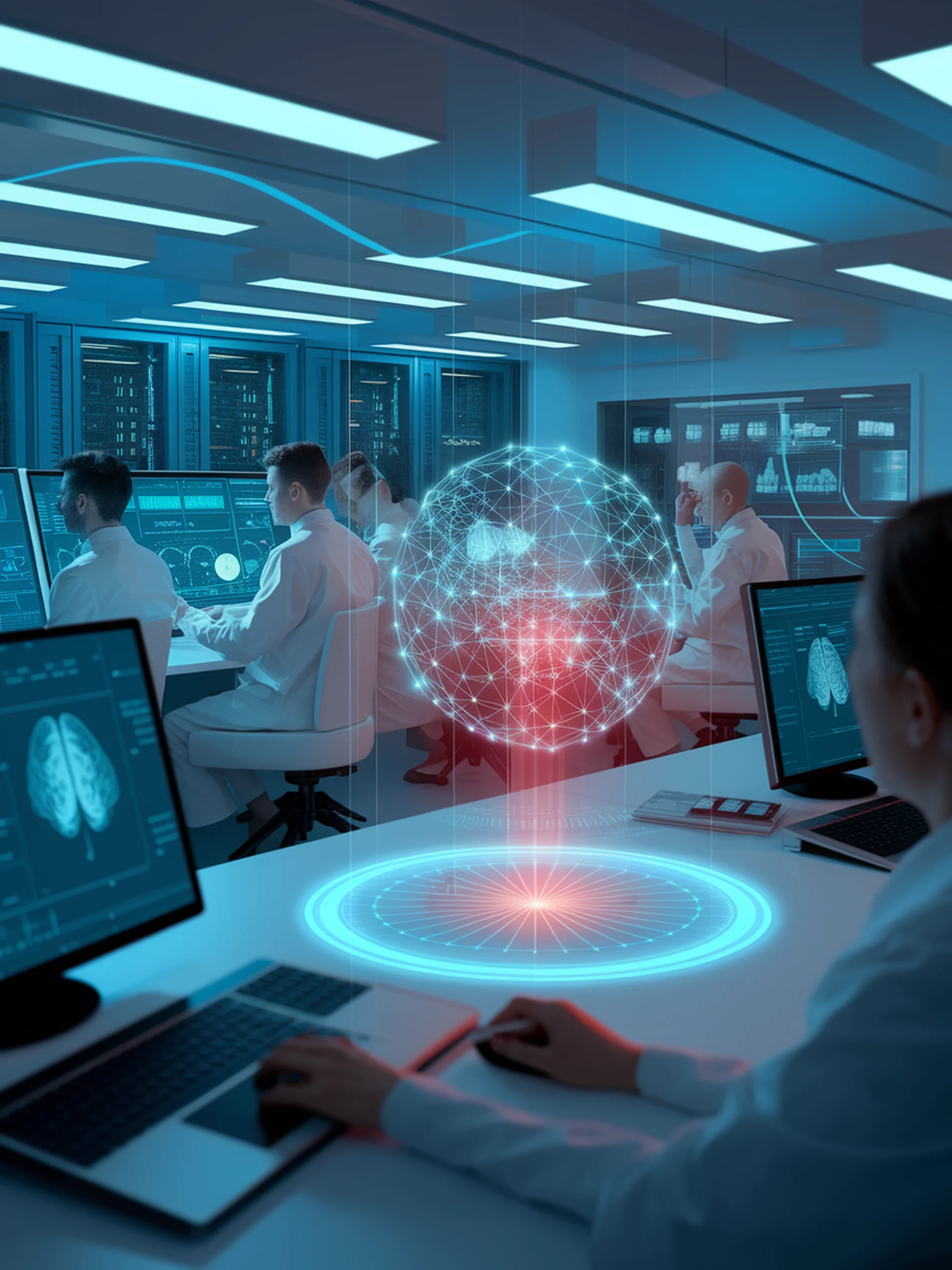
Safer AI: Selective Memory Control for LLMs
Targeted knowledge removal without compromising overall performance
This research introduces an effective technique for selectively removing harmful knowledge from Large Language Models while preserving their general capabilities.
- Uses Conditional Sparse Autoencoder Clamping to target specific knowledge domains
- Successfully reduces model responses about dangerous topics like bioweapons and cyberattacks
- Maintains normal functionality for harmless queries
- Applied approach works across different model sizes and architectures
This advancement addresses critical security concerns by providing AI developers with a precise tool to mitigate potential misuse of language models without diminishing their overall utility or requiring complete retraining.
Original Paper: Don't Forget It! Conditional Sparse Autoencoder Clamping Works for Unlearning