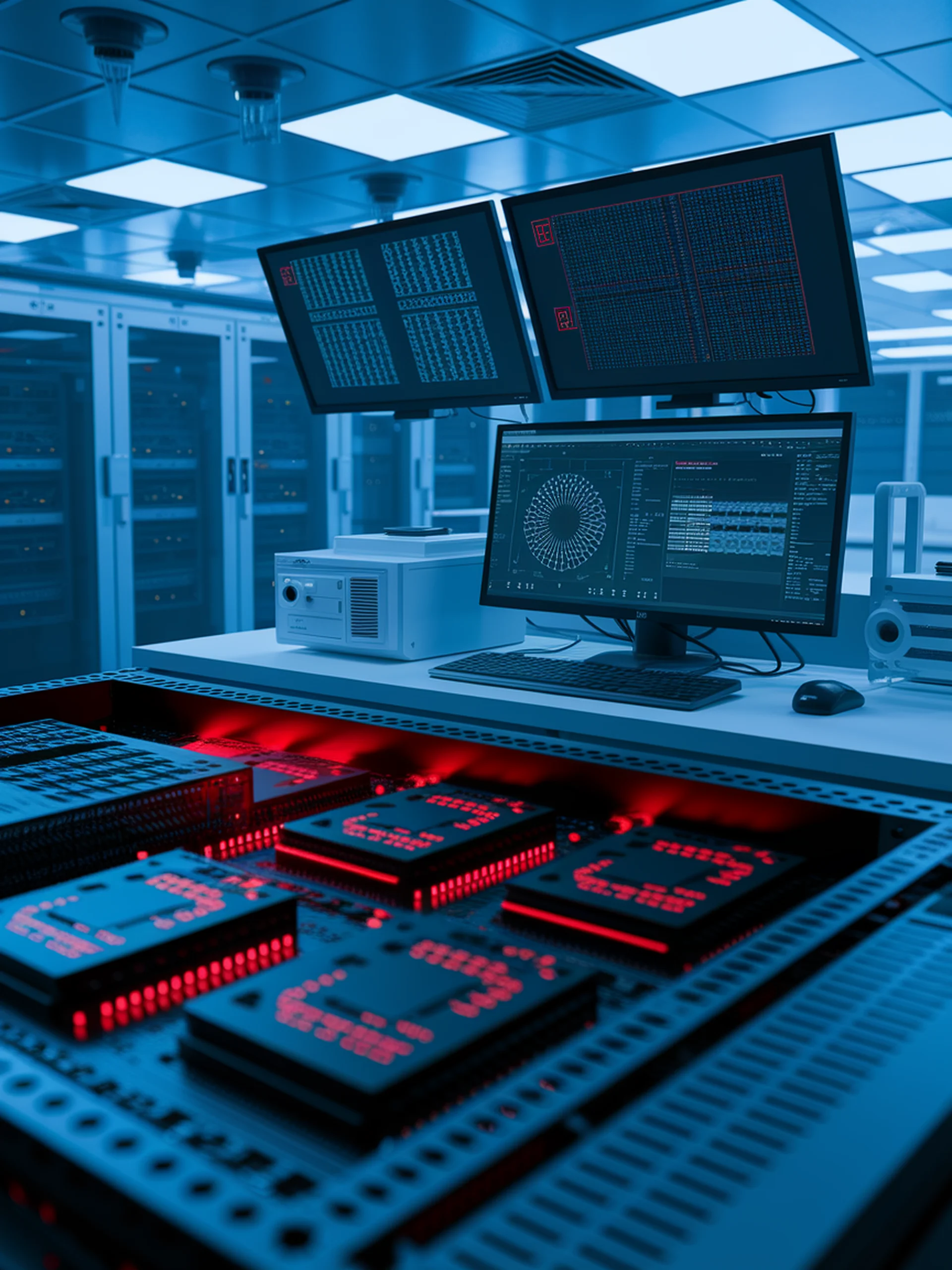
Beyond Inputs: Probing LLM Security Vulnerabilities
Revealing hidden capabilities through model tampering attacks
This research introduces model tampering attacks as a more rigorous approach to evaluating LLM security risks, demonstrating that traditional testing underestimates actual model capabilities.
- Researchers modified model weights to reveal capabilities that standard input-based testing failed to detect
- Tests on open-source models showed they could be made to generate harmful content despite safety measures
- The approach provides a more accurate upper bound on model capabilities than traditional prompt-based evaluations
- Findings suggest current governance frameworks need to evolve beyond input-output testing
This work has significant implications for AI security governance, highlighting the need for more comprehensive evaluation methods when assessing potential risks in deployed language models.
Model Tampering Attacks Enable More Rigorous Evaluations of LLM Capabilities