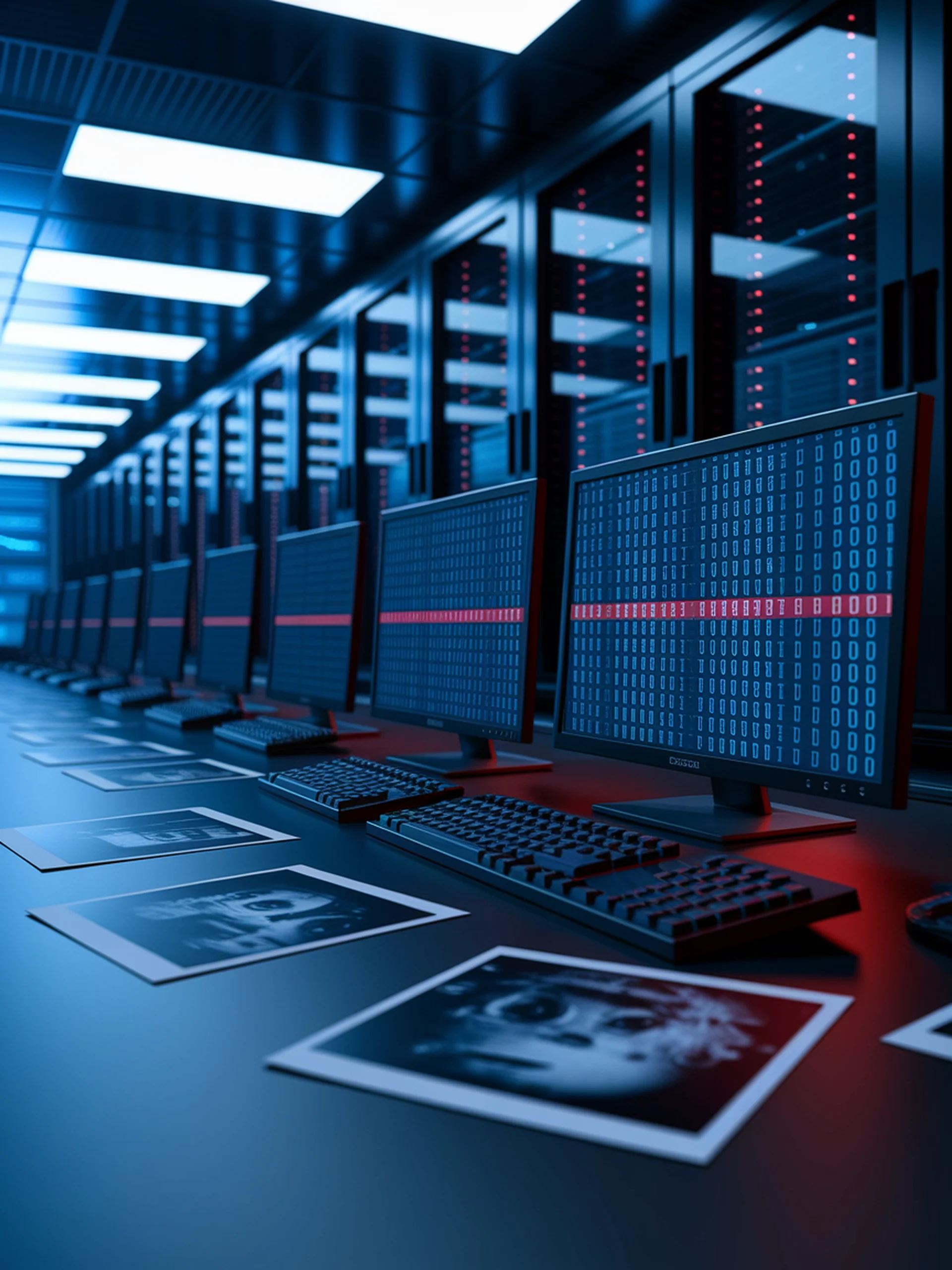
Stealthy Typographic Attacks on Vision-Language Models
New vulnerabilities in multi-image settings reveal enhanced security risks
This research uncovers how Large Vision-Language Models (LVLMs) can be compromised through typographic attacks across multiple images, presenting more sophisticated security challenges than single-image attacks.
- Introduces a multi-image attack setting where attackers use different text across multiple images rather than repeating the same attack
- Demonstrates these non-repeating attacks are more stealthy and better at evading security gatekeepers
- Reveals critical security vulnerabilities in modern AI systems processing multiple images simultaneously
- Suggests the need for robust defense mechanisms against these sophisticated attacks
This work highlights significant security implications for industries deploying vision-language AI in production environments where multiple images are processed, including content moderation, autonomous systems, and visual search applications.