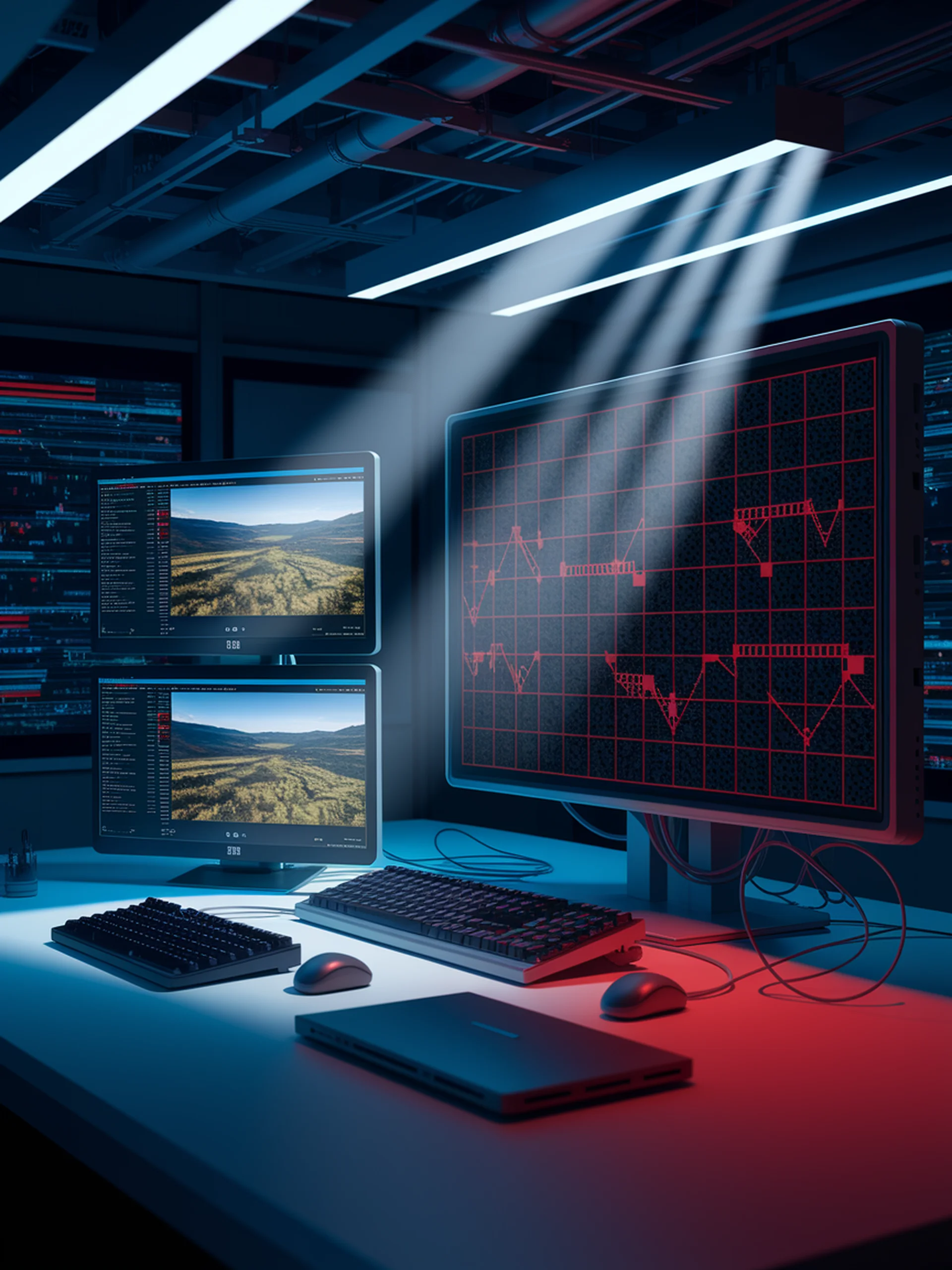
Web Artifact Attacks: A New Security Threat to AI Vision
How seemingly harmless web elements can manipulate vision-language models
This research reveals how vision-language models (VLMs) like CLIP and LLaVA can be manipulated through unintended correlations they learn from web data.
- Models trained on web data learn to associate visual concepts with irrelevant artifacts (watermarks, borders, text overlays)
- Attackers can exploit these correlations to manipulate model predictions without changing the core image content
- The paper demonstrates how inserting specific visual artifacts can cause targeted misclassifications
- Researchers propose defense strategies to make VLMs more robust against these attacks
This work highlights critical security vulnerabilities in widely-used AI vision systems and emphasizes the need for more careful data curation and model training to build trustworthy AI systems.