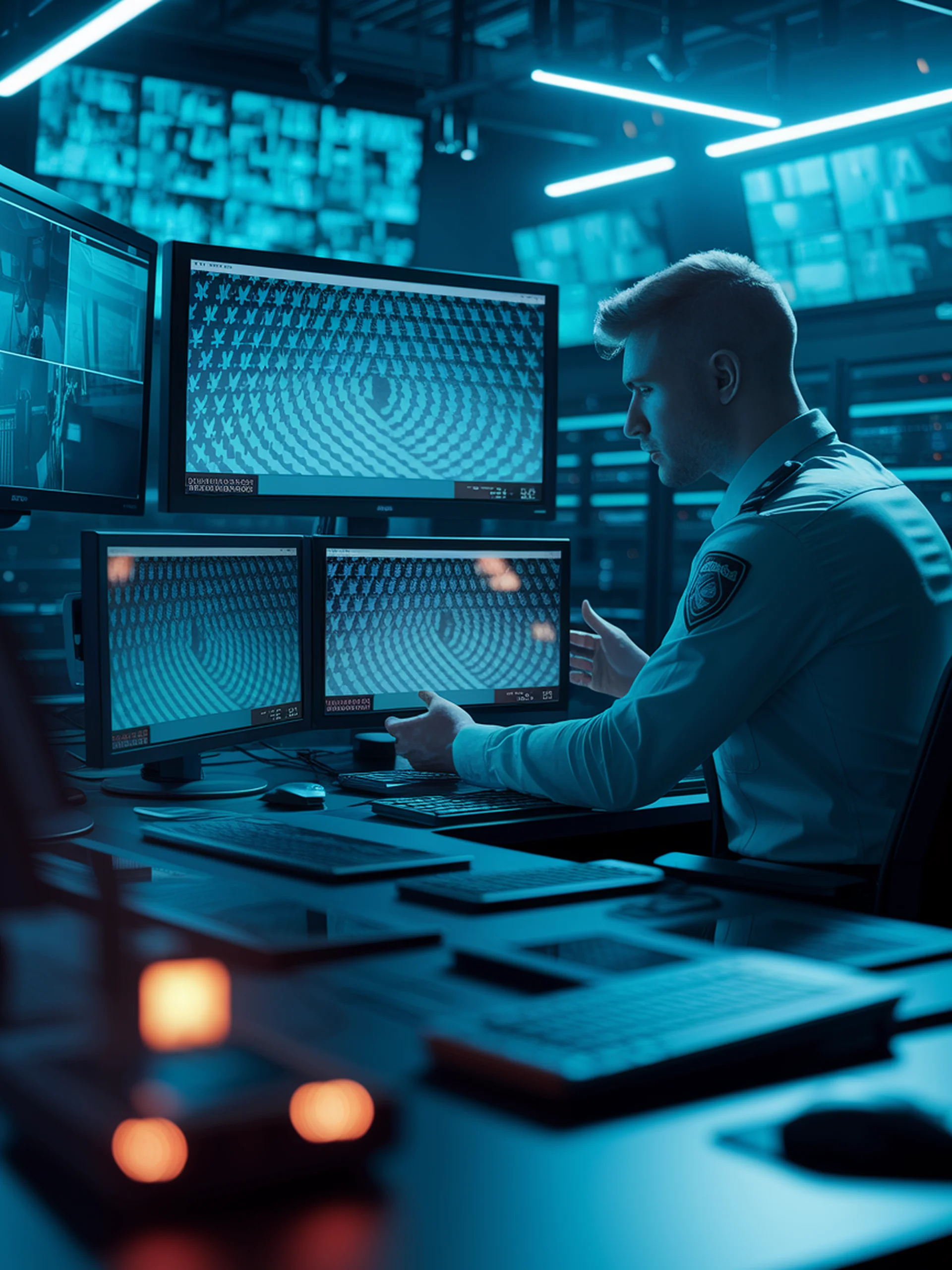
Disrupting AI Video Surveillance
Protective Watermarking to Prevent Unauthorized Video Analysis
This research introduces a visual protection framework to prevent video-based LLMs from unauthorized annotation of personal video content.
- Develops a specialized adversarial watermark that disrupts AI video analysis while preserving human viewing experience
- Achieves over 80% reduction in automated annotation accuracy
- Demonstrates effectiveness across multiple leading video-based LLMs including LLaVA-NeXT and Video-LLaMA
- Provides a practical defense against unauthorized use of video in training data extraction
As AI video analysis becomes ubiquitous, this protection mechanism empowers content creators to shield their privacy while maintaining control over how their visual data is processed by AI systems.
Protecting Your Video Content: Disrupting Automated Video-based LLM Annotations