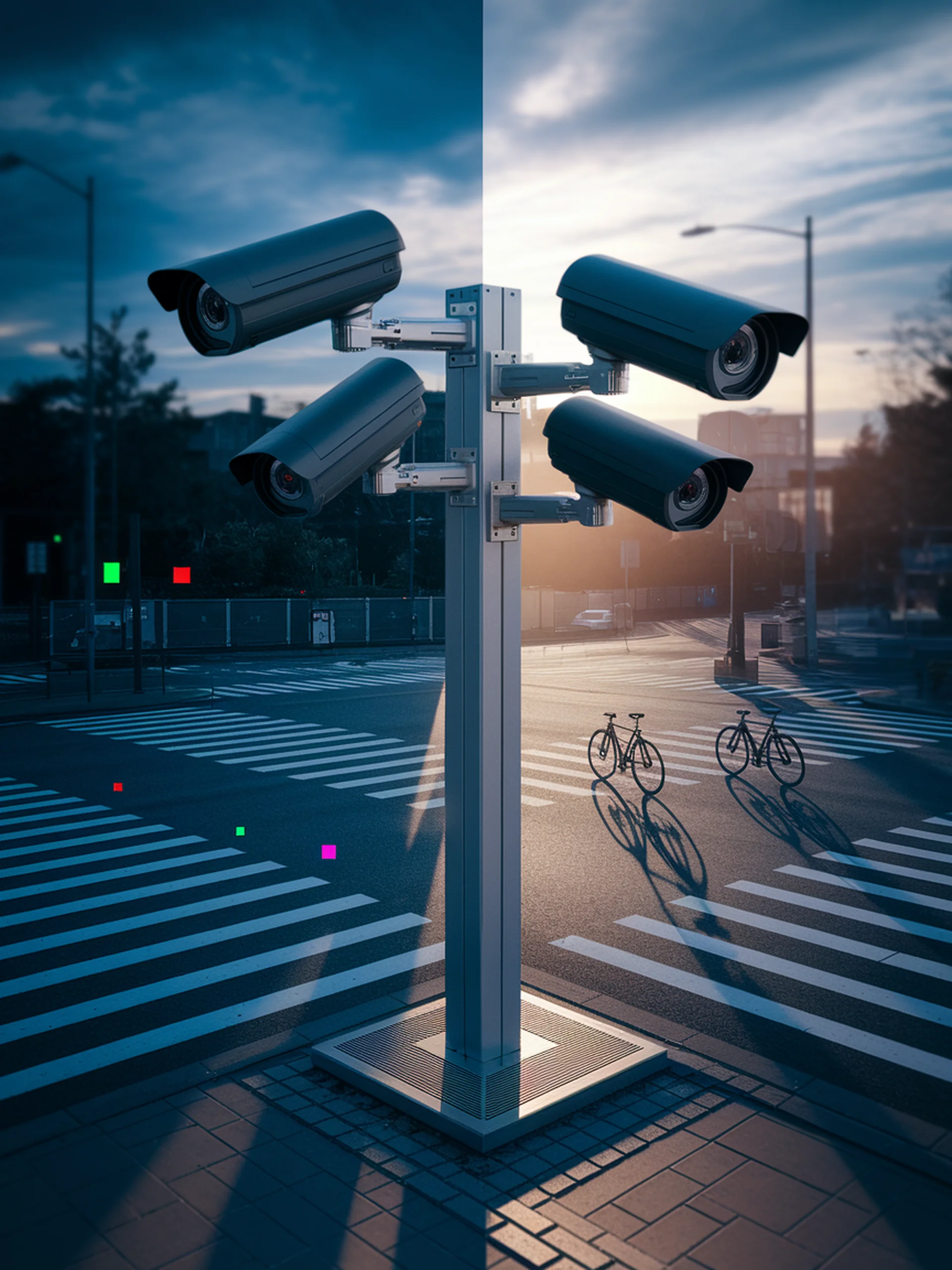
Robust Object Detection Across Changing Environments
A new benchmark for measuring resilience to distribution shifts
This research introduces COUNTS, a comprehensive benchmark for evaluating how well object detectors and multimodal large language models handle distribution shifts.
- Addresses a critical gap in assessing out-of-distribution (OOD) generalization capabilities
- Provides fine-grained annotations for evaluating performance on complex detection tasks
- Enables systematic testing of model resilience across varying environmental conditions
- Particularly valuable for security applications where detection systems must operate reliably in unpredictable environments
This benchmark helps security professionals identify and mitigate potential vulnerabilities in AI-powered surveillance and monitoring systems that might otherwise fail when deployed in real-world conditions that differ from training data.
COUNTS: Benchmarking Object Detectors and Multimodal Large Language Models under Distribution Shifts