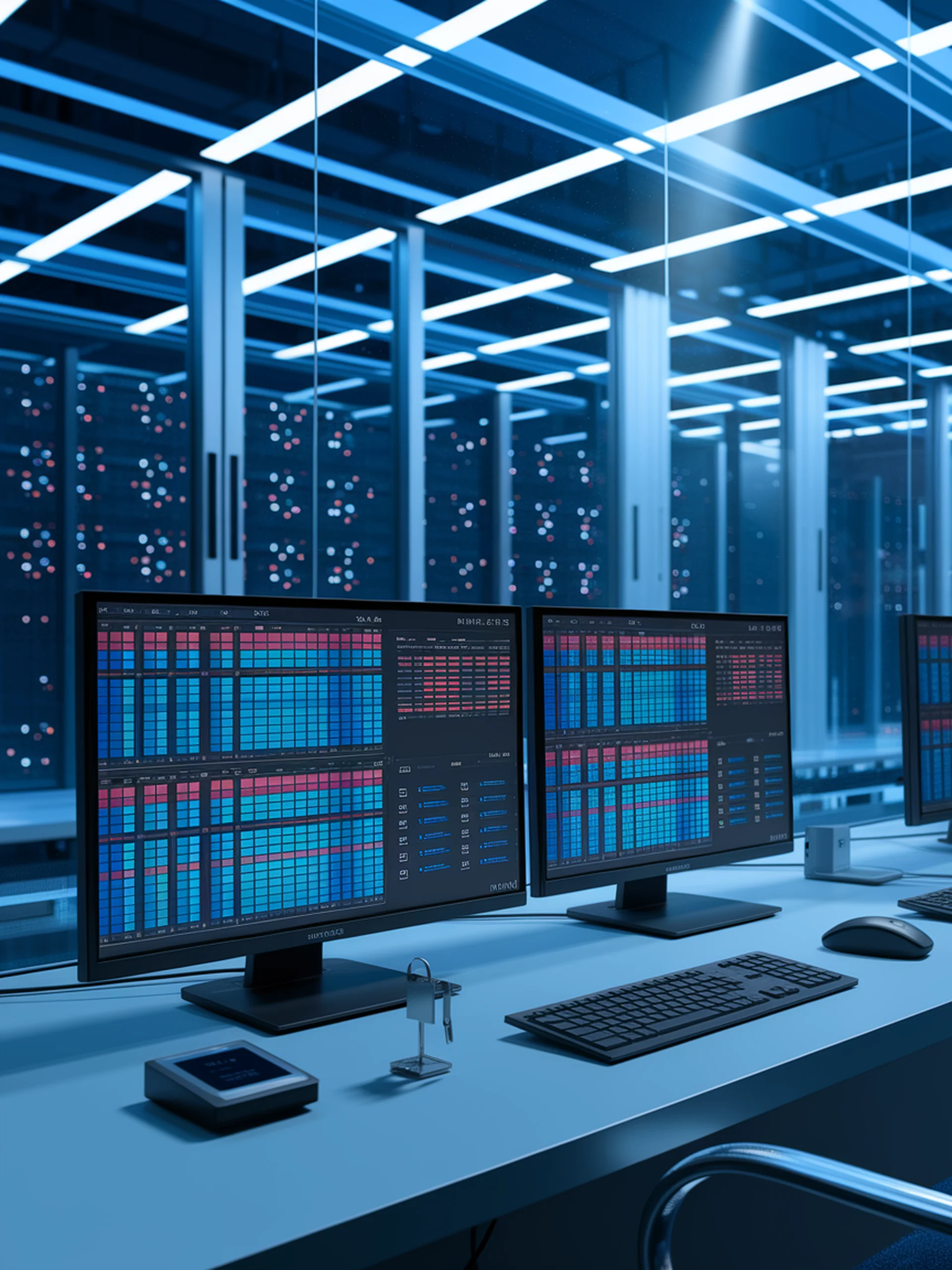
Reimagining Tabular Data Synthesis with LLMs
TabuLa: A Novel Approach to Generate Realistic Tabular Data
TabuLa leverages the power of Large Language Models to generate synthetic tabular data that addresses critical privacy and security concerns in data-intensive industries.
Key Innovations:
- Efficient Architecture: Optimized to overcome the training time and reusability limitations of current LLM-based synthesizers
- Enhanced Privacy Protection: Generates realistic tabular data while preserving sensitive information
- Practical Implementation: Designed for real-world applications where data privacy is paramount
- Industry-Ready Solution: Particularly valuable for security applications requiring synthetic but representative datasets
Security Impact: TabuLa provides organizations with a robust tool to generate high-quality synthetic data, enabling secure data sharing, testing, and analysis without exposing sensitive information or violating privacy regulations.
TabuLa: Harnessing Language Models for Tabular Data Synthesis