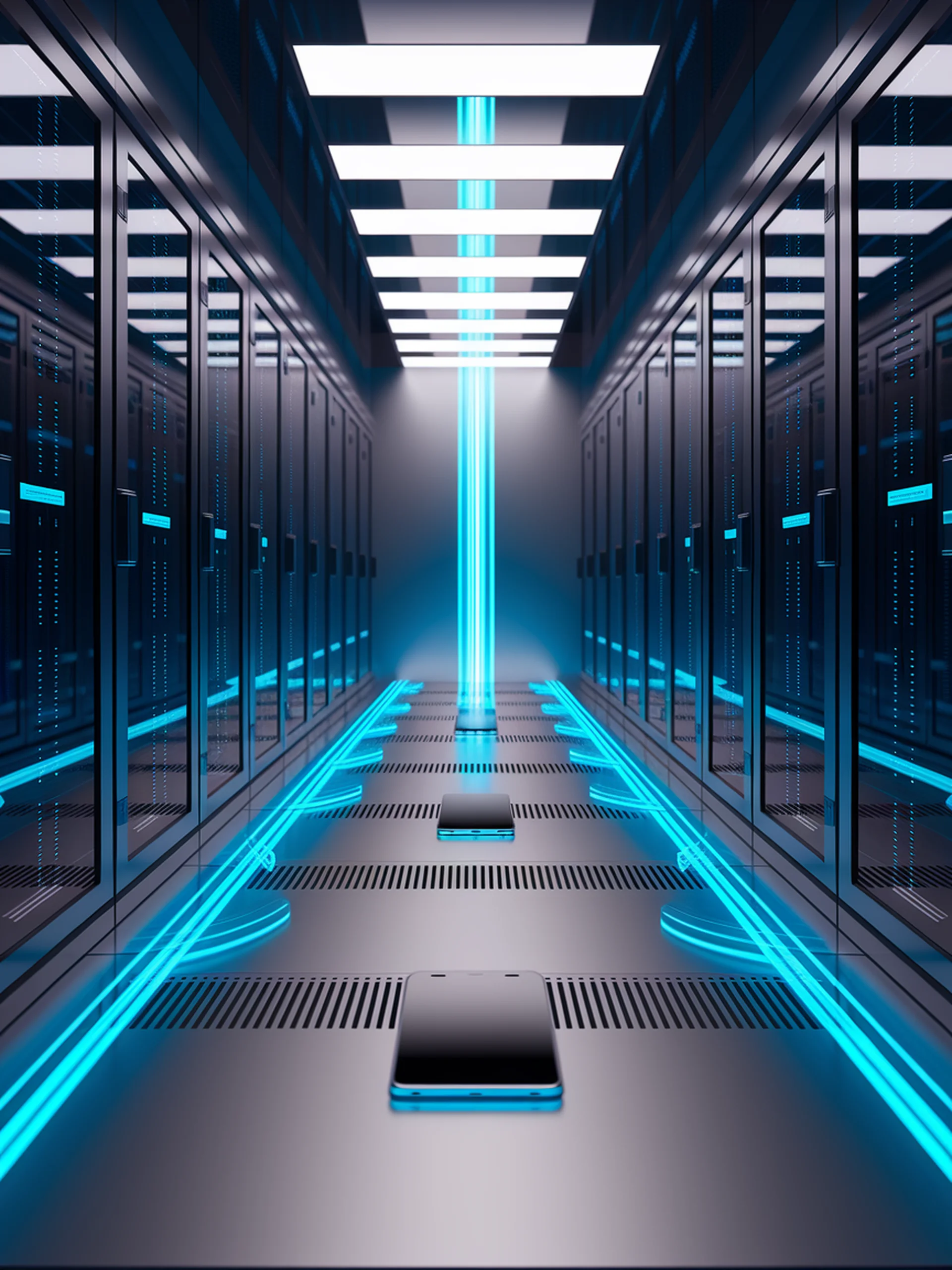
Privacy-Preserving LLM Recommendations
A federated learning approach that protects user data while enabling personalized recommendations
This research introduces a federated framework for LLM-based recommendation systems that addresses critical privacy concerns while maintaining personalization quality.
- Combines federated learning with LLMs to keep sensitive user data on local devices
- Addresses key challenges in federated LLM deployments including client heterogeneity and communication overhead
- Proposes a novel approach that balances privacy protection with recommendation performance
- Establishes a security foundation for LLM-based recommendation systems compliant with data protection regulations
This framework represents a significant advancement in secure AI systems, enabling organizations to leverage powerful LLM capabilities for personalized recommendations without compromising user privacy or violating increasingly strict data protection laws.