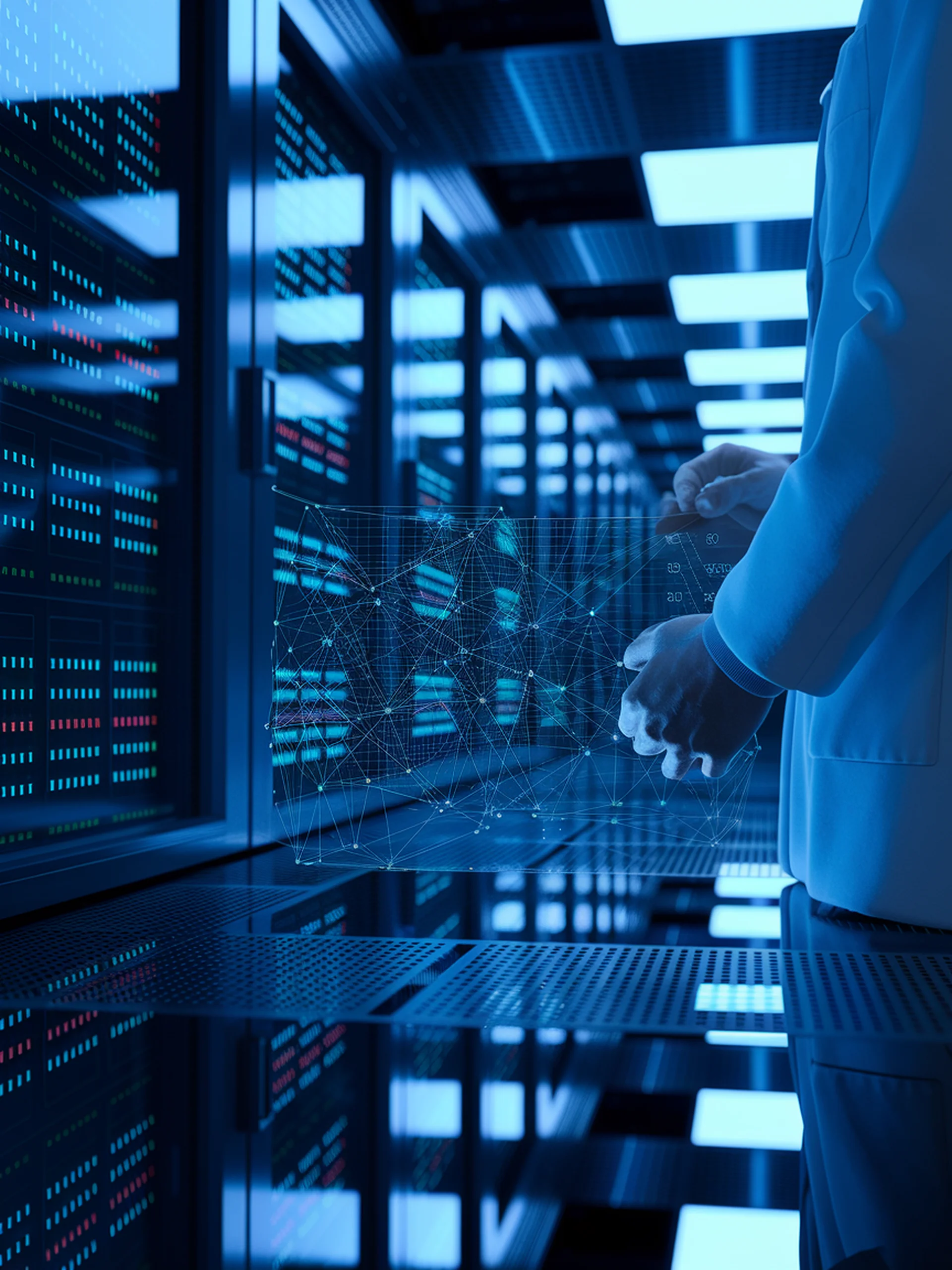
Detecting Pre-Training Data in LLMs
A new, theoretically-sound approach to identifying training data leakage
Min-K%++ is a novel methodology for accurately detecting whether specific text was used to train large language models, addressing critical security concerns.
- Improved Detection: Enhances the state-of-the-art Min-K% approach with theoretical foundations rather than simple heuristics
- Security Applications: Helps identify potential copyright violations in model training data
- Test Integrity: Reduces risks of test data contamination that compromises model evaluation
- Theoretical Soundness: Based on robust mathematical principles rather than ad-hoc methods
This research is vital for the security and privacy of LLM deployments, enabling organizations to verify training data compliance and ensure model evaluations aren't compromised by data contamination.
Min-K%++: Improved Baseline for Detecting Pre-Training Data from Large Language Models