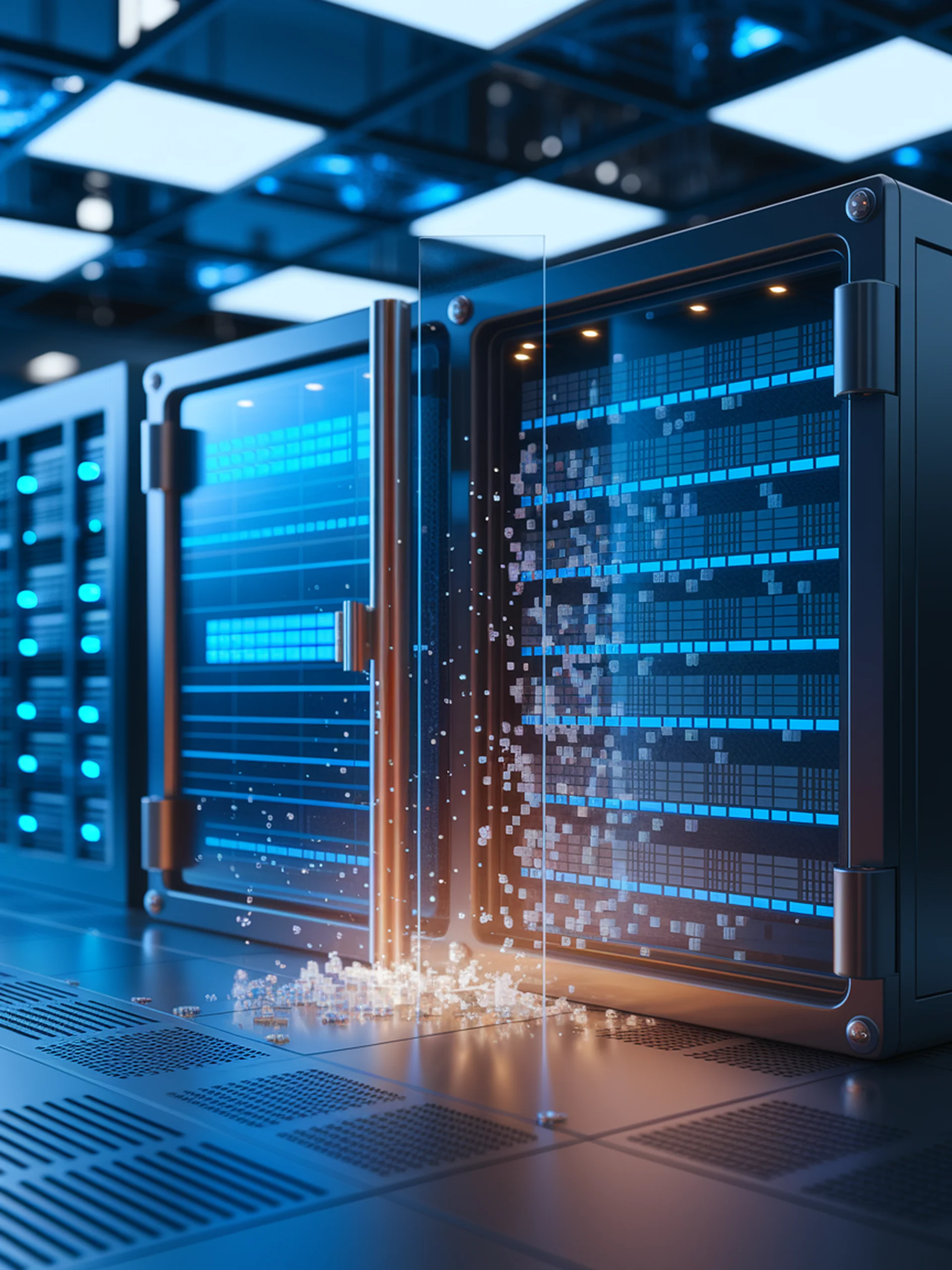
Knowledge Washing in Large Language Models
Safely removing unwanted knowledge while preserving model capabilities
This research introduces Large Scale Knowledge Washing, a novel approach to selectively remove extensive factual knowledge from LLMs without degrading their core capabilities.
- Addresses critical concerns about LLMs memorizing private, toxic, or copyrighted content
- Develops a targeted unlearning method that preserves model fluency and reasoning abilities
- Demonstrates effective removal of specific knowledge domains while maintaining general performance
- Provides a scalable approach to knowledge security in foundation models
For security professionals, this work offers a practical solution to mitigate privacy risks and legal liabilities associated with LLM deployments while maintaining model utility.