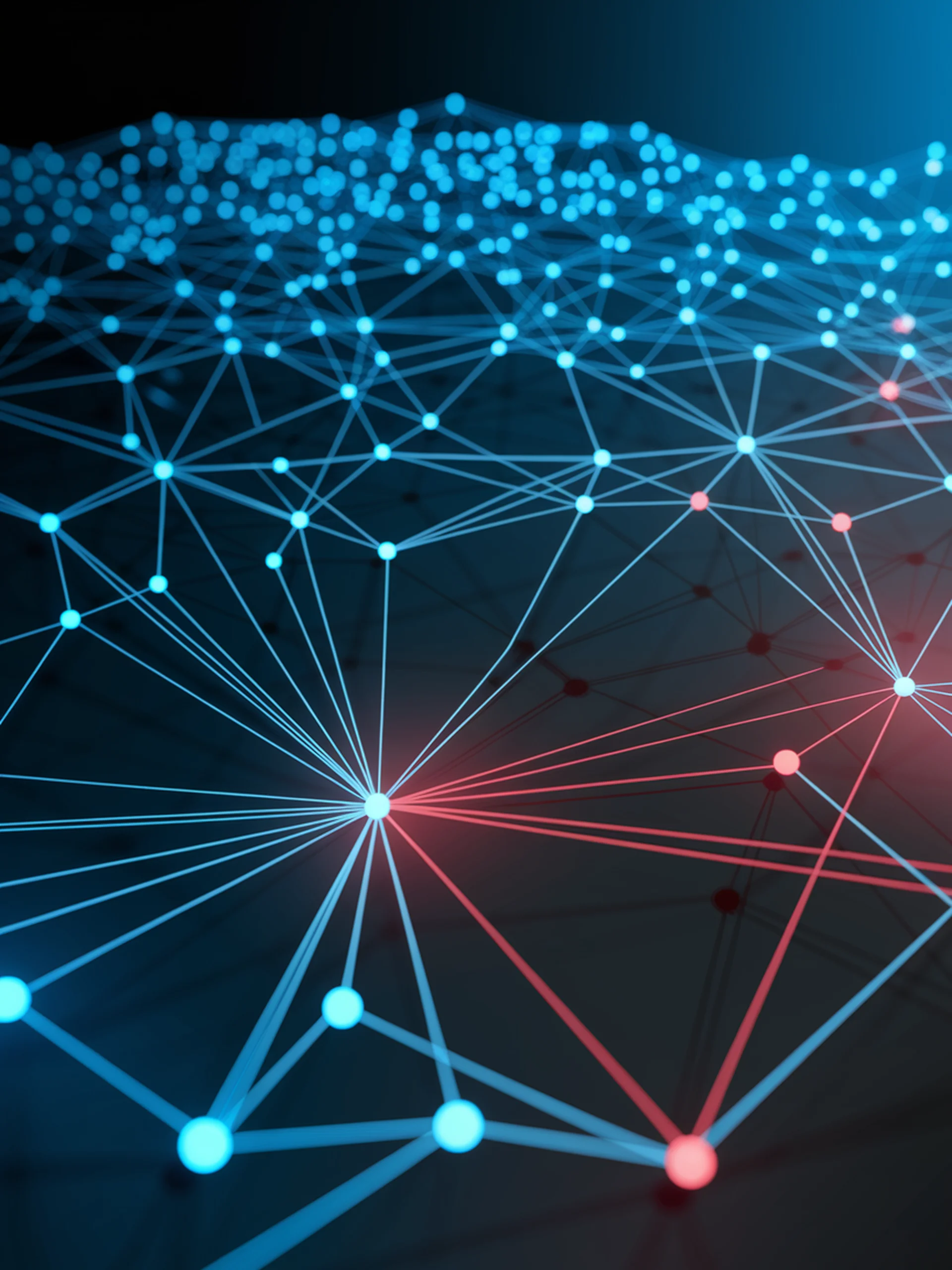
Rethinking LLM Unlearning: The Missing Data Connection
How interconnected data structures impact secure knowledge removal
This research challenges the common assumption that data points to be forgotten from LLMs are independent, introducing PISTOL, a method that addresses the overlooked inter-connectivity of real-world data.
- Demonstrates how contractual relationships create inherent data structures affecting unlearning
- Reveals limitations in current unlearning approaches that treat data as isolated points
- Provides a framework for more effective knowledge removal that respects data relationships
- Highlights critical security and legal implications for responsible AI deployment
For organizations concerned with data privacy and compliance, this research offers crucial insights into developing more robust unlearning techniques that align with real-world data structures.
How Data Inter-connectivity Shapes LLMs Unlearning: A Structural Unlearning Perspective