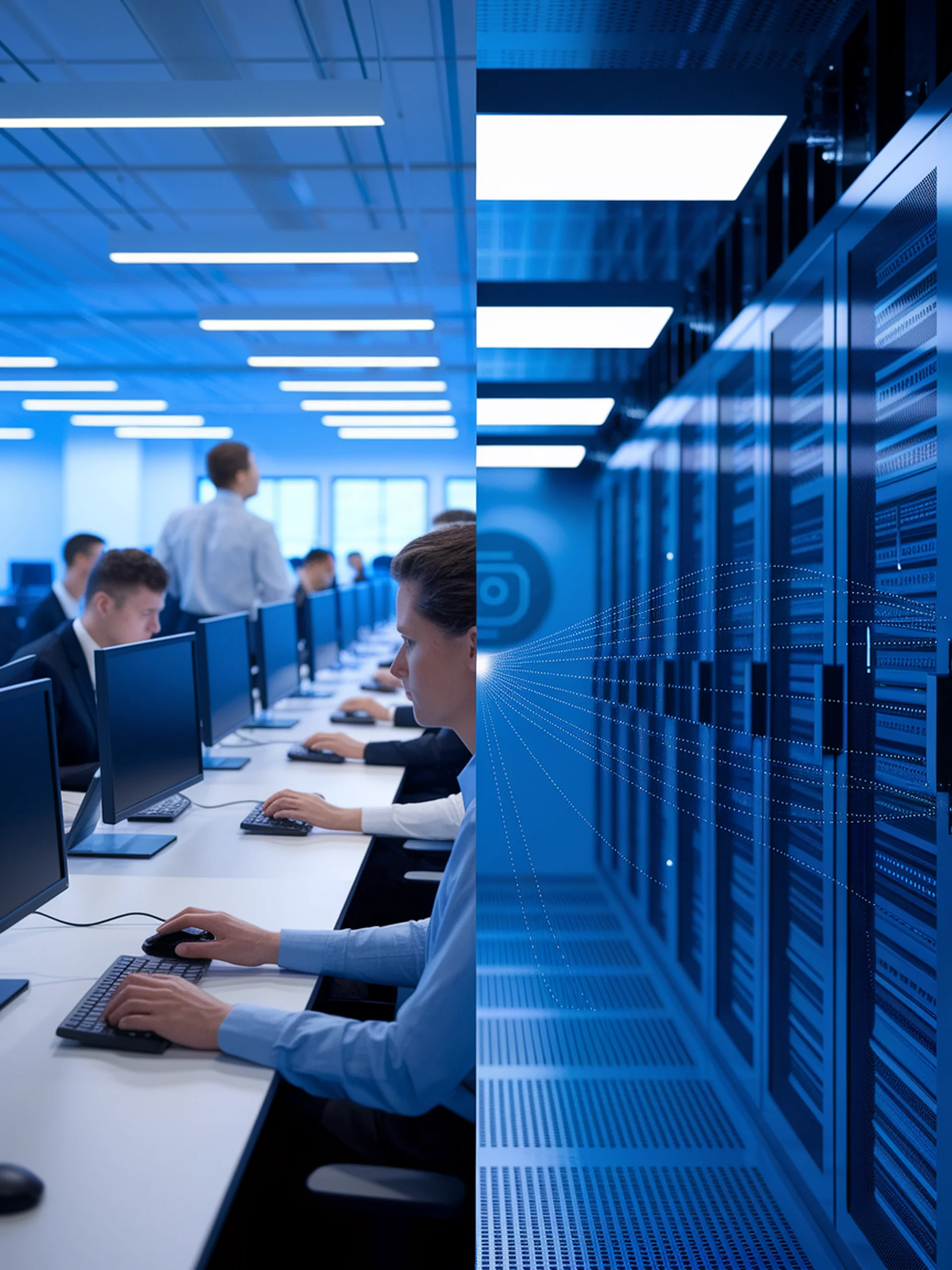
Enhancing LLM Training With Privacy-Preserving Quality Control
A federated approach to filter low-quality data without compromising privacy
FedDQC introduces dynamic quality control for federated instruction-tuning of LLMs, enabling organizations to collaboratively train models while keeping sensitive data local.
- Addresses the challenge of filtering low-quality samples in decentralized training environments
- Implements two-phase quality control mechanisms that work within privacy constraints
- Enables privacy-preserving collaboration between organizations with sensitive instruction data
- Demonstrates improved model performance compared to standard federated learning approaches
This research is crucial for security-conscious organizations seeking to leverage collective data resources without exposing proprietary information or user data, while still maintaining high training standards.
Data Quality Control in Federated Instruction-tuning of Large Language Models