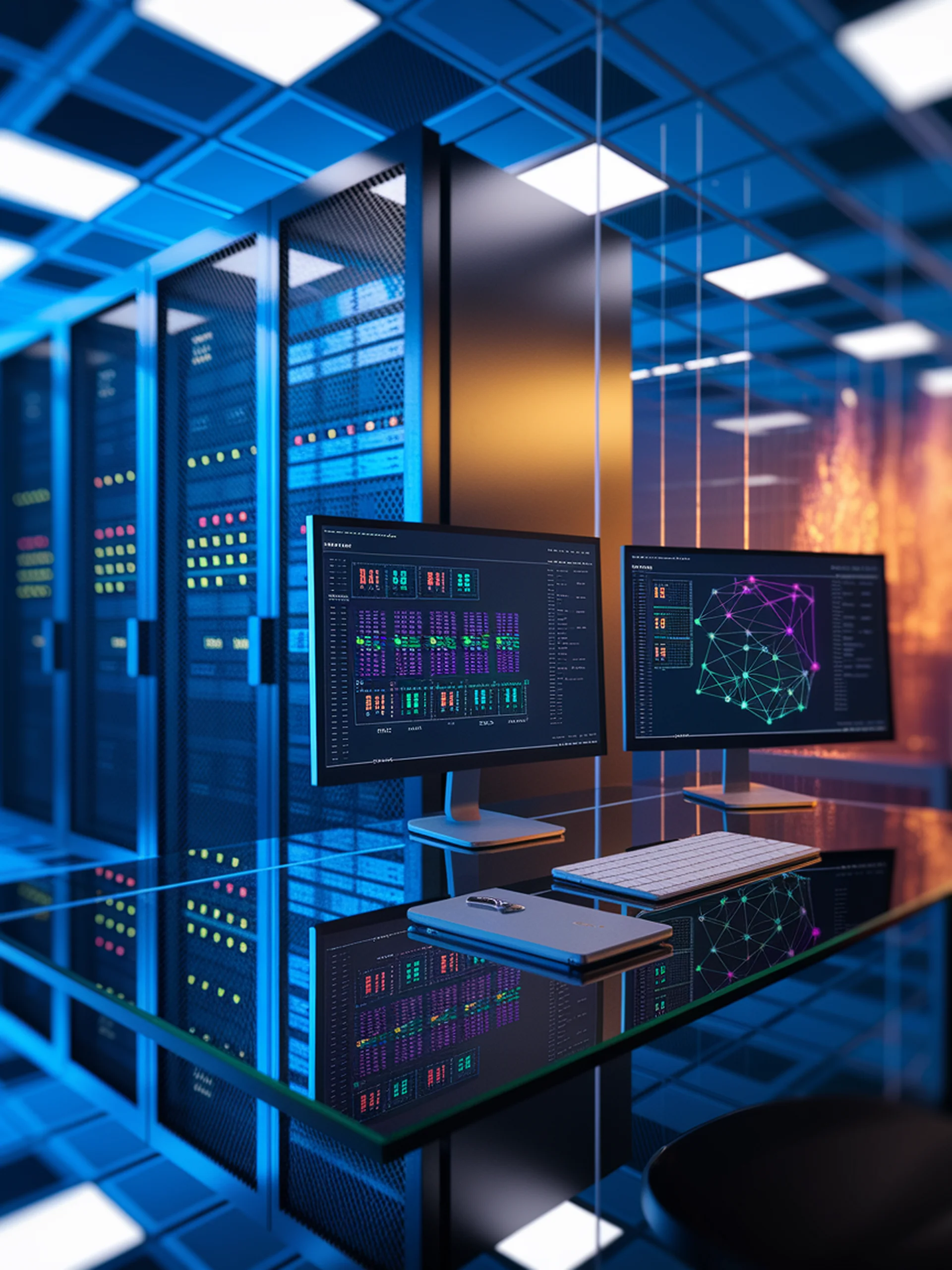
Uncovering the Hidden Memories of LLMs
A New Framework to Measure Privacy Risks in AI Models
This research introduces probabilistic discoverable extraction, a more nuanced approach to measuring how large language models memorize and potentially leak sensitive training data.
- Reveals limitations in current binary (yes/no) extraction methods
- Demonstrates how probabilistic measurement better quantifies memorization risk
- Shows that extraction risk increases with model size but varies across datasets
- Provides a framework for more accurate security assessments of LLMs
This research matters because it gives organizations a more precise tool to evaluate privacy and security vulnerabilities in their AI deployments, helping prevent inadvertent exposure of sensitive information.
Measuring memorization in language models via probabilistic extraction