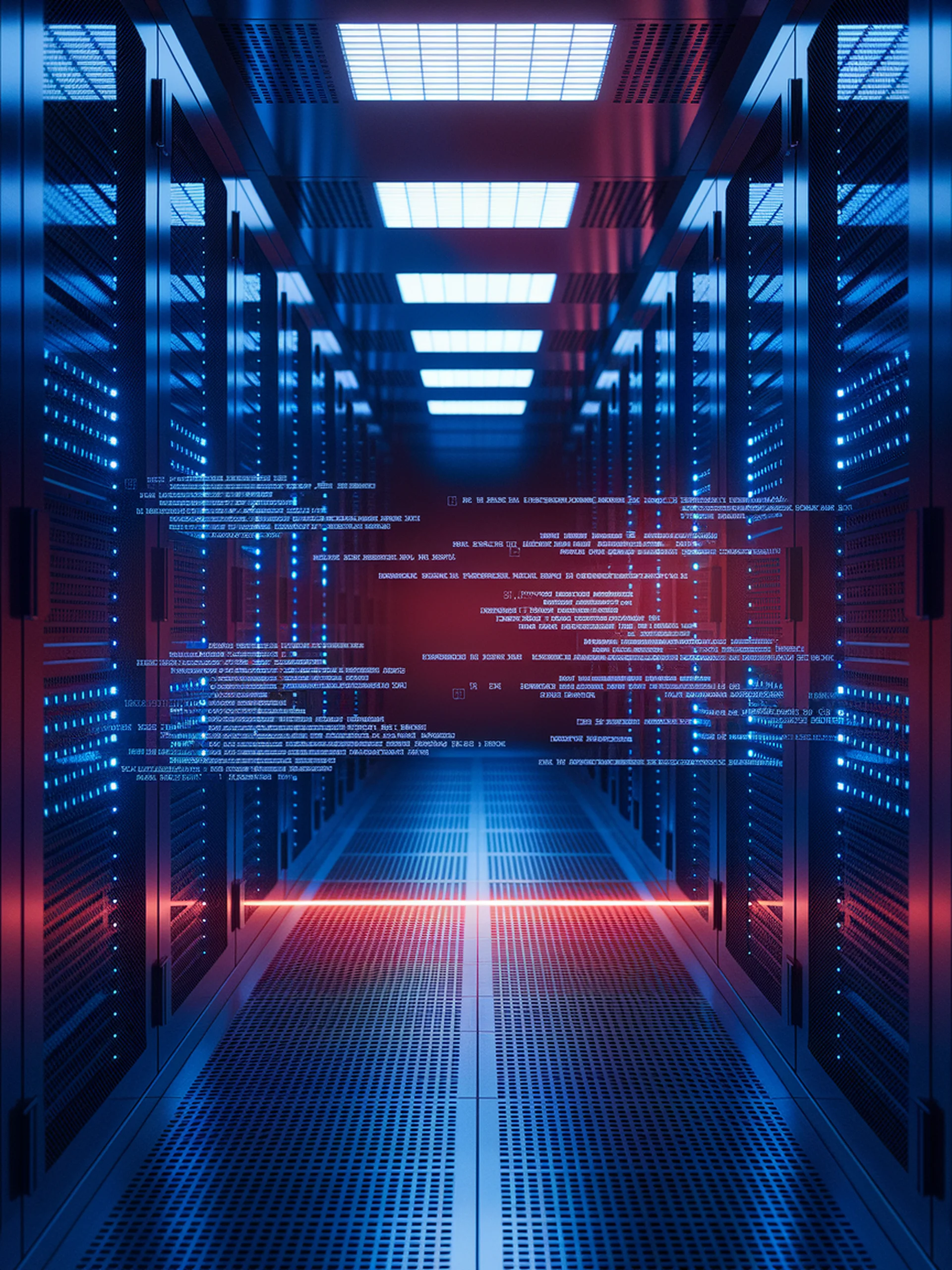
The Instruction-Data Boundary Problem in LLMs
Addressing critical security vulnerabilities in language models
This research identifies the lack of clear boundaries between instructions and data in LLMs as a fundamental security vulnerability, presenting new frameworks to evaluate and address this issue.
- LLMs currently cannot reliably distinguish between instructions and the data they should process
- This vulnerability enables attacks like prompt injections, making LLMs unsuitable for safety-critical tasks
- The paper proposes novel benchmarks to quantify and measure this separation problem
- Highlights the need for structural safety features common in other areas of computer science
For security professionals, this research provides critical insights into an underlying weakness affecting all instruction-tuned LLMs, offering potential pathways toward more secure AI systems.
Can LLMs Separate Instructions From Data? And What Do We Even Mean By That?