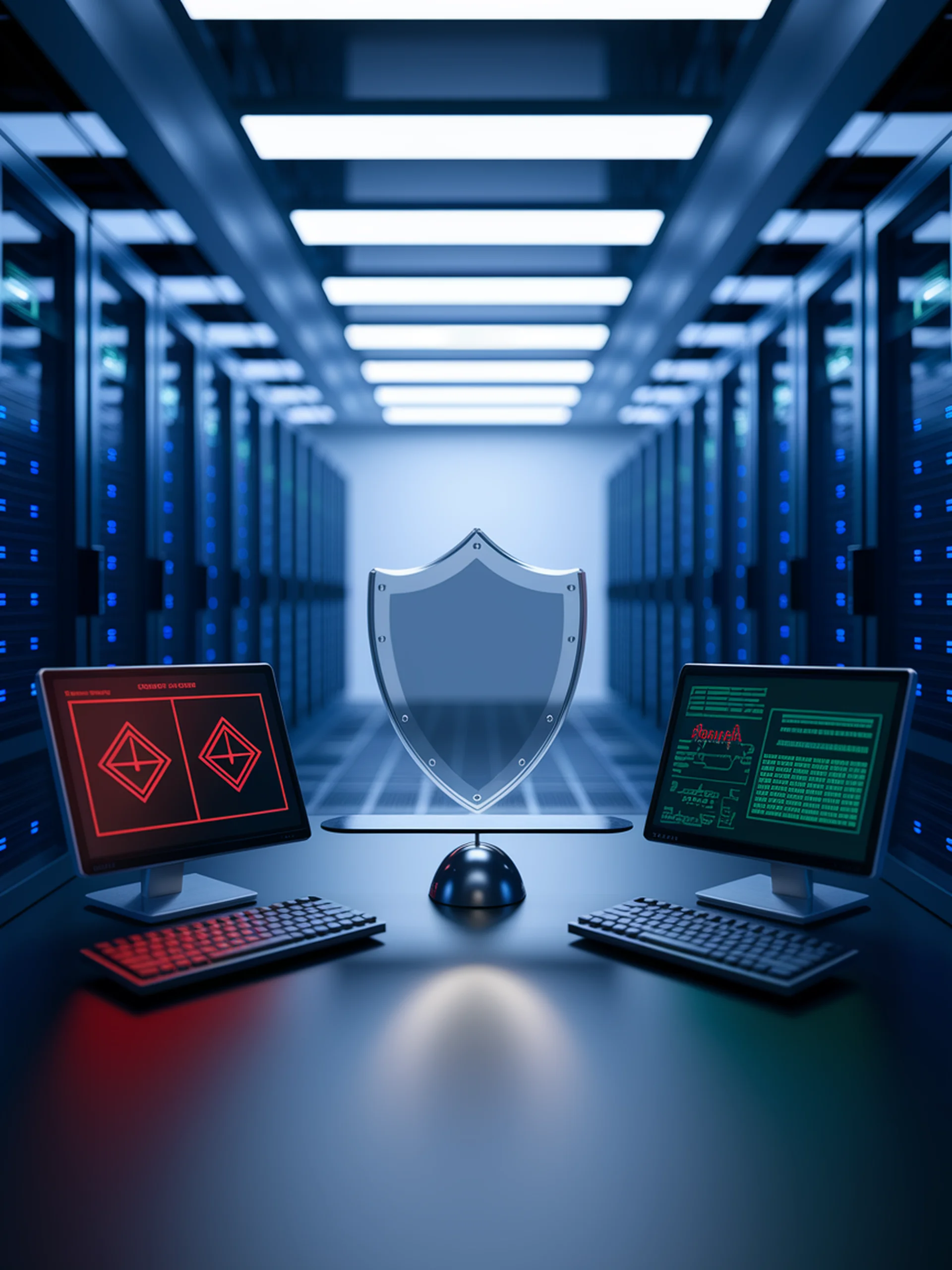
Balancing LLM Defense Systems
Solving the Over-Defense Problem in Prompt Injection Guards
InjecGuard addresses a critical flaw in prompt injection defenses: false flagging of legitimate inputs as attacks.
- Introduces NotInject, a dataset specifically designed to measure over-defensive behaviors in guard models
- Reveals bias toward trigger words causing excessive rejection of benign prompts
- Proposes a novel approach that achieves strong protection while reducing false positives
- Demonstrates improved performance across multiple benchmarks
This research is vital for security teams building reliable AI safeguards that protect against attacks without compromising legitimate user interactions.
InjecGuard: Benchmarking and Mitigating Over-defense in Prompt Injection Guardrail Models