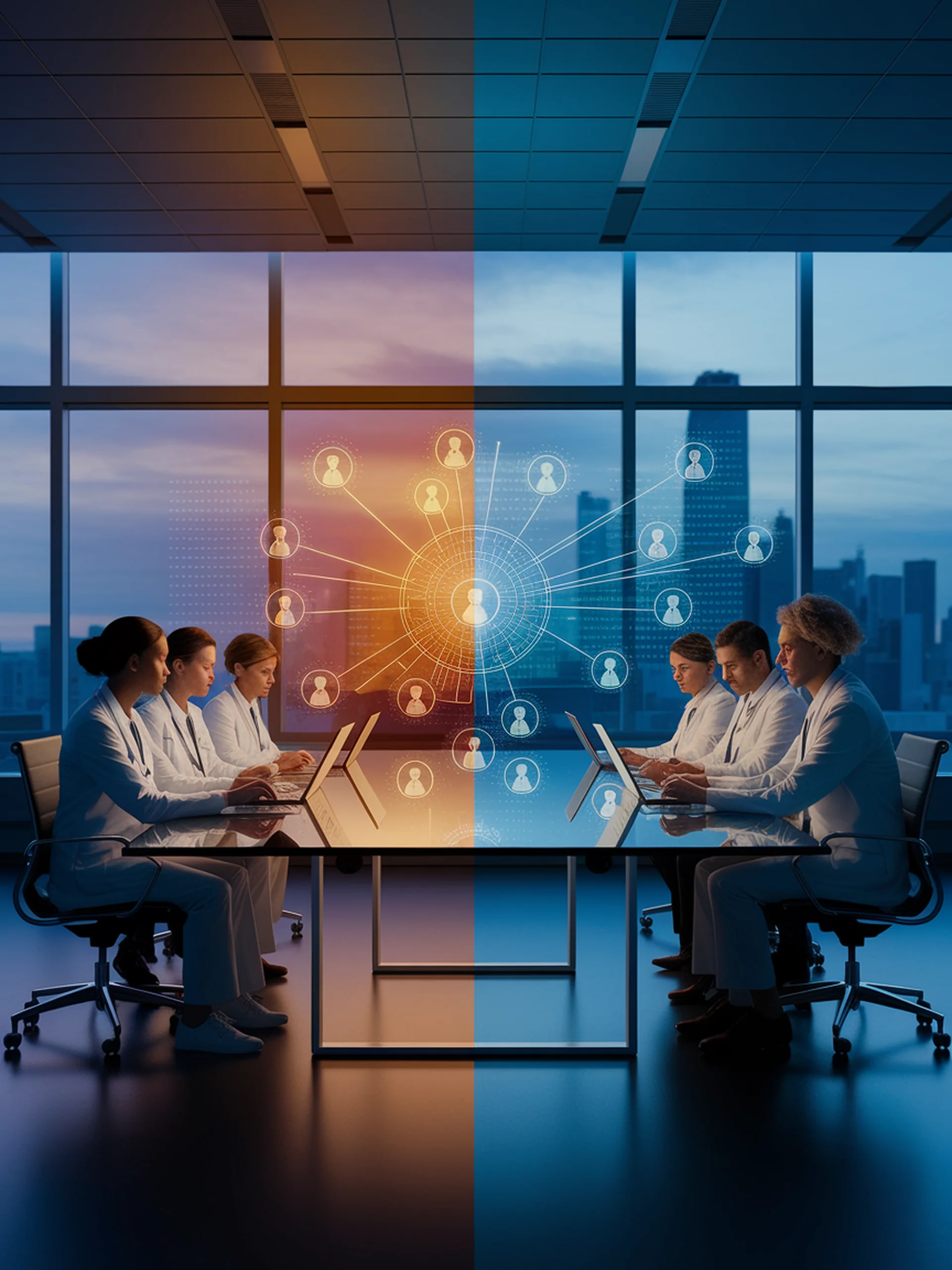
Causality-Guided Debiasing for Safer LLMs
Reducing social biases in AI decision-making for high-stakes scenarios
This research introduces a causality-guided framework to mitigate social biases in large language models, particularly for high-stakes applications like healthcare and hiring.
- Identifies and reduces objectionable dependencies between LLM decisions and social information
- Targets applications where fair AI decision-making is critical for safety and compliance
- Provides a methodology that addresses bias at its causal roots rather than through surface-level interventions
- Demonstrates particular relevance for security contexts where biased AI could lead to discriminatory outcomes
From a security perspective, this approach helps safeguard against discriminatory AI behaviors that could violate regulations, harm vulnerable populations, or create legal liability in sensitive applications.
Learn more: Prompting Fairness: Integrating Causality to Debias Large Language Models