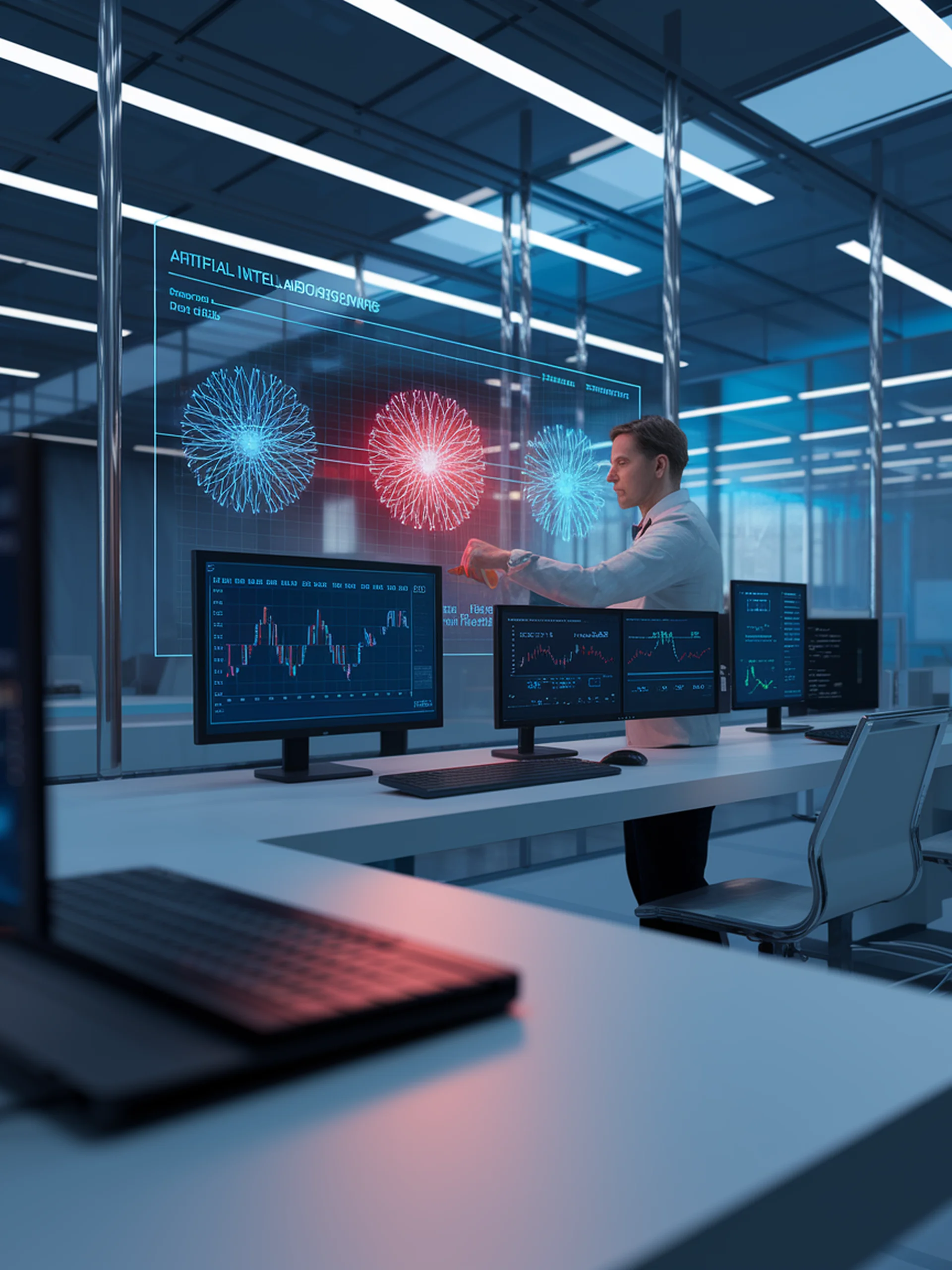
LARS: Learning to Estimate LLM Uncertainty
A trainable approach replacing hand-crafted uncertainty scoring functions
LARS introduces a trainable scoring function for uncertainty estimation in large language models, improving reliability assessment through learning rather than manual design.
- Transforms traditional hand-crafted scoring functions into a learning problem that adapts to model-specific characteristics
- Demonstrates superior performance in identifying uncertain or erroneous LLM outputs across tasks and models
- Provides a unified approach that works effectively across different LLM architectures
- Enhances security and trust by more accurately flagging potentially unreliable generations
This research enables more reliable deployment of LLMs in critical applications by better identifying when model outputs should not be trusted, reducing risks of misleading or harmful content in production systems.