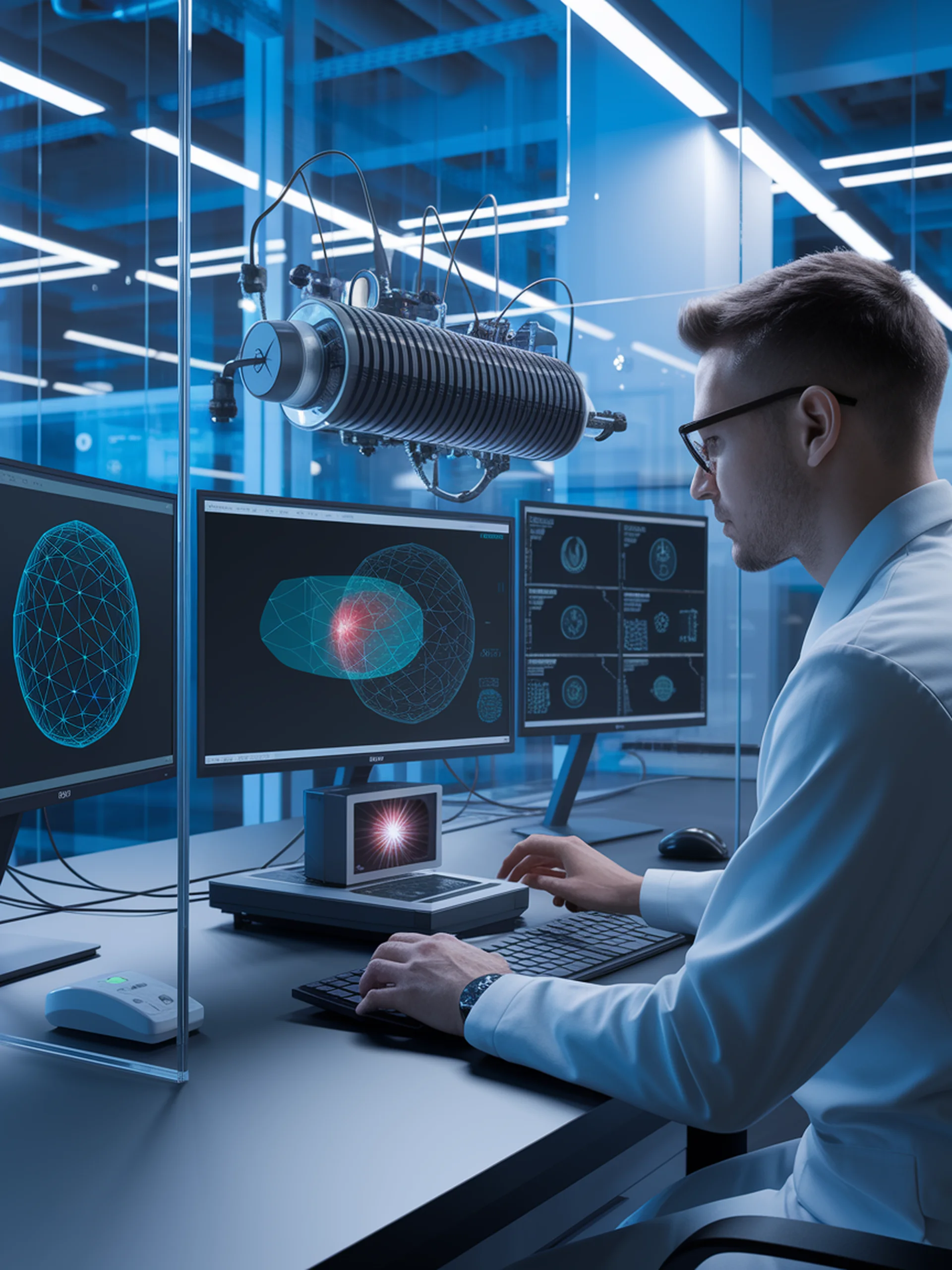
Fighting Visual Hallucinations in AI
A more efficient approach to ensure AI sees what's really there
VASparse introduces a novel decoding algorithm that makes Large Vision-Language Models more trustworthy by reducing hallucinations without sacrificing speed.
- Reduces visual hallucinations by selectively filtering tokens using visual-aware sparsification
- Maintains inference efficiency unlike methods requiring secondary decoding
- Achieves 20.6% reduction in hallucination rate while improving response quality
- Works as a plug-and-play solution compatible with various LVLMs
Security Impact: By ensuring AI outputs remain faithful to visual reality, VASparse addresses a critical security concern in AI systems where hallucinated content could lead to misinformation or unreliable automated decision-making.
VASparse: Towards Efficient Visual Hallucination Mitigation via Visual-Aware Token Sparsification