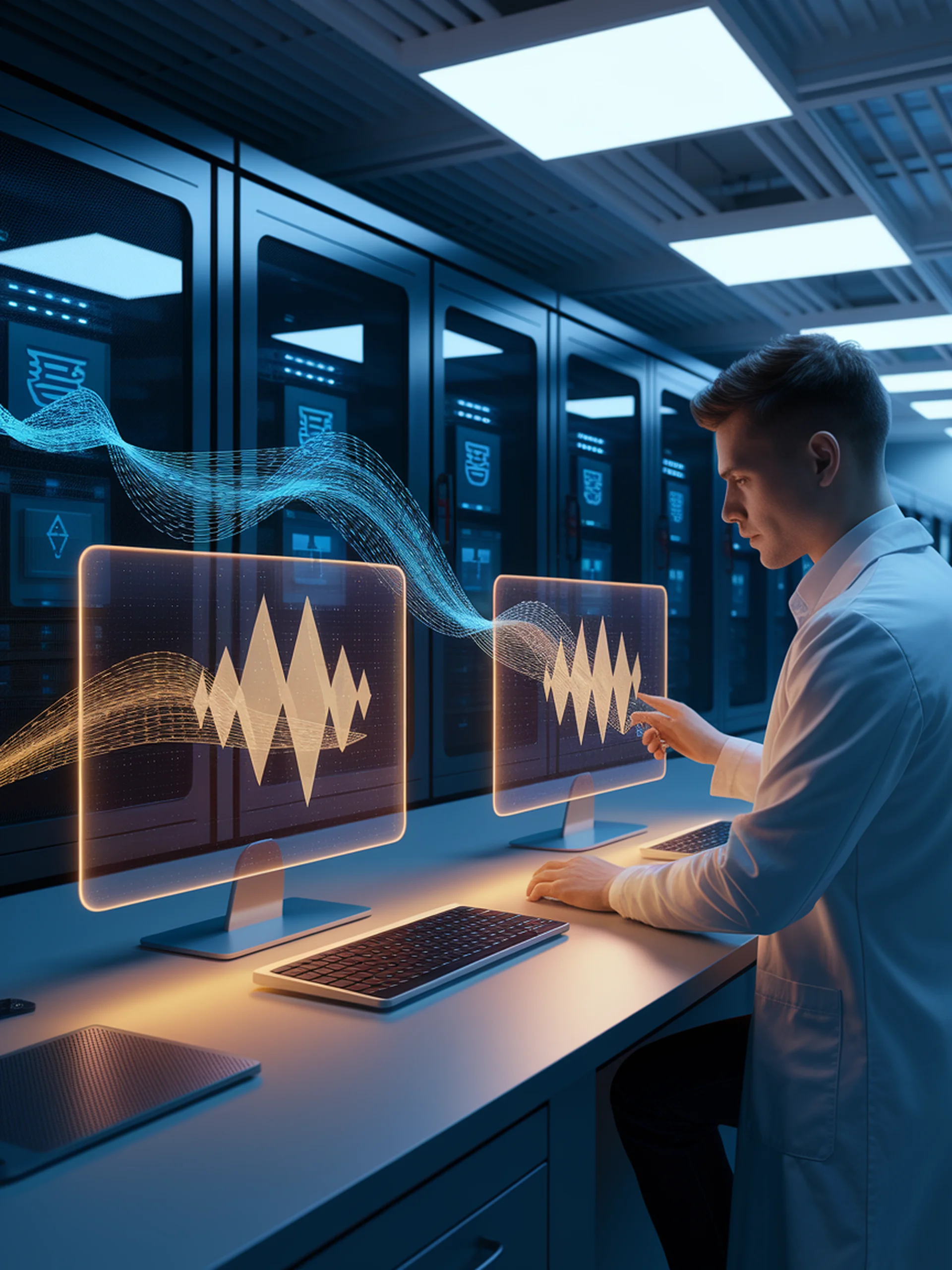
Combating LLM Hallucinations
Using Smoothed Knowledge Distillation to improve factual reliability
This research introduces a novel approach to reduce hallucination in large language models through smoothed knowledge distillation, addressing a critical challenge for deploying LLMs in high-stakes environments.
- Replaces traditional hard labels with smoothed probability distributions from teacher models
- Reduces model overconfidence and better represents uncertainty inherent in language
- Demonstrates improved factual accuracy and reduced hallucination rates
- Provides a practical training method that doesn't require extensive additional resources
From a security perspective, this advancement directly enhances reliability and trustworthiness of LLMs when deployed in sensitive contexts where factual accuracy is essential, reducing potential risks from AI-generated misinformation.
Smoothing Out Hallucinations: Mitigating LLM Hallucination with Smoothed Knowledge Distillation