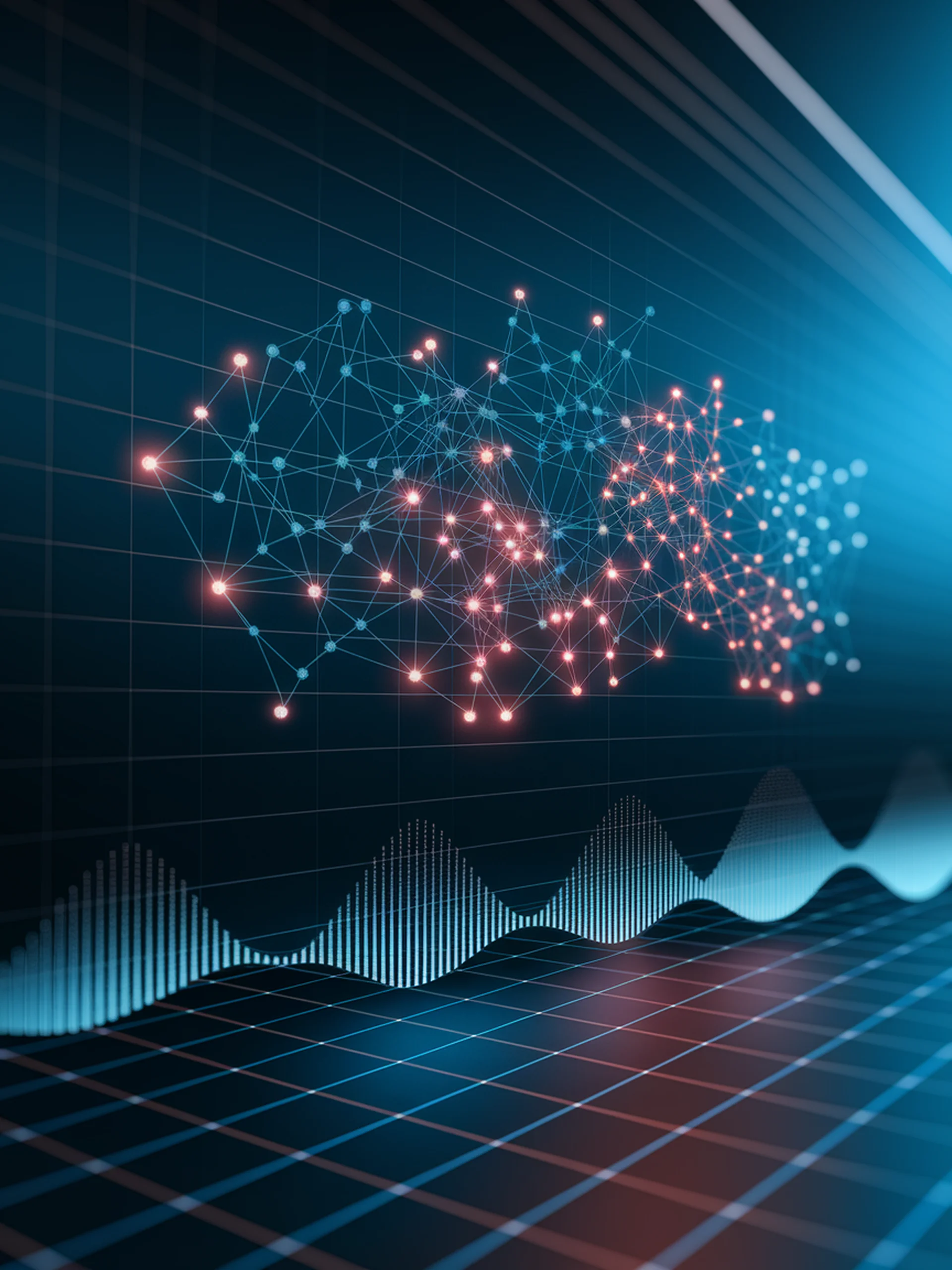
Detecting LLM Hallucinations with Graph Theory
A novel spectral approach to identify when AI systems fabricate information
This research introduces LapEigvals, a new method that leverages graph theory to detect hallucinations in Large Language Models by analyzing the spectral features of attention maps.
- Interprets attention maps as graph structures to identify mathematical signatures of hallucinations
- Provides a more reliable detection mechanism for false information generated by LLMs
- Addresses a critical security challenge in AI deployment for safety-critical applications
- Offers a technical approach that could help organizations implement stronger safeguards against AI misinformation
This methodology is particularly valuable for security applications where LLM reliability is non-negotiable, such as healthcare, finance, and critical infrastructure, helping organizations reduce risk when deploying generative AI technologies.
Hallucination Detection in LLMs Using Spectral Features of Attention Maps