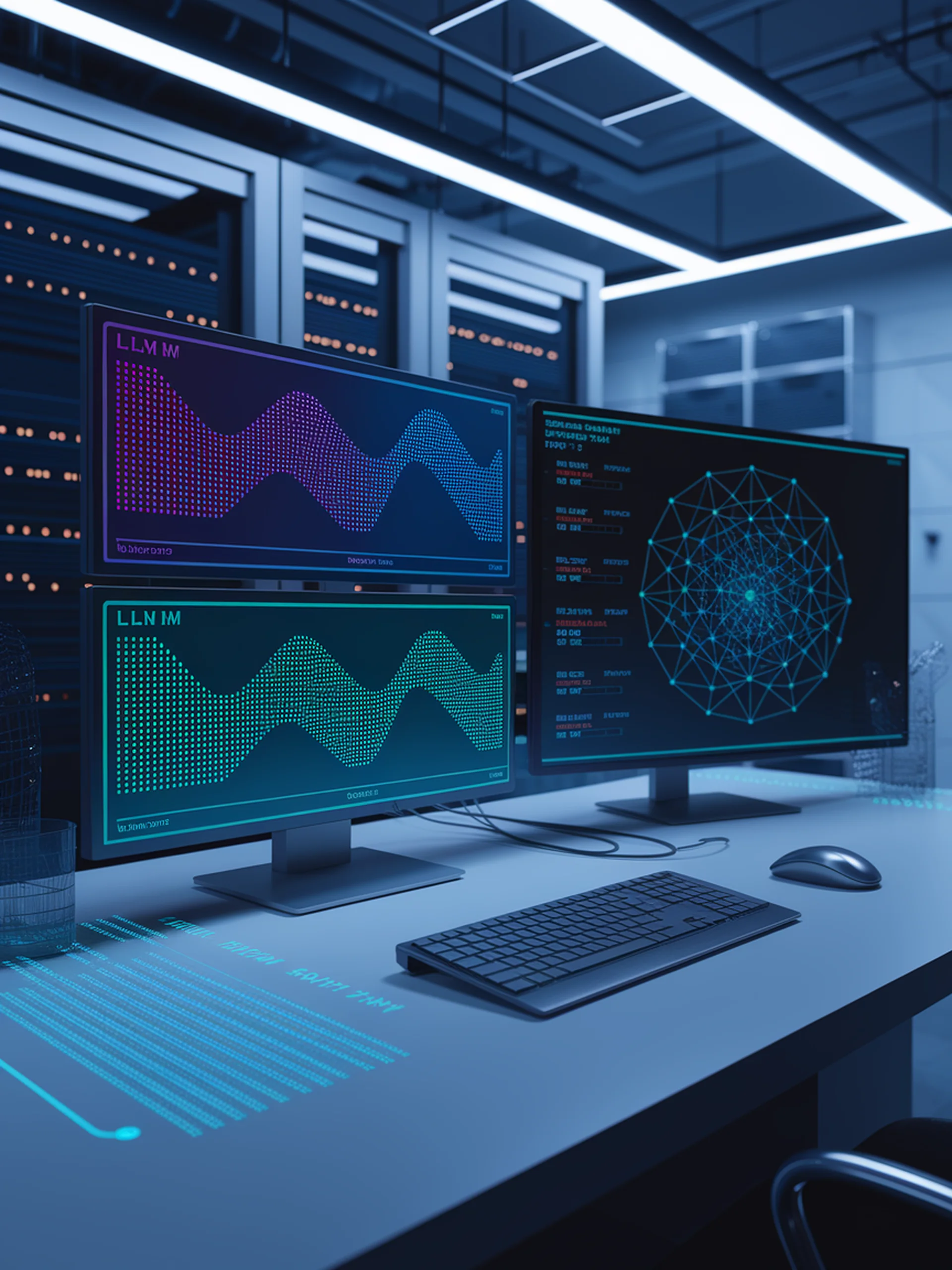
Detecting LLM Hallucinations Without Model Access
New 'Gray-Box' Approach for Analyzing LLM Behavior
This research introduces an innovative transformer-based framework that analyzes LLM output patterns to detect problematic behaviors like hallucinations and data contamination without requiring access to internal model parameters.
- Leverages output signature analysis from LLM-generated tokens
- Offers a practical alternative to "white-box" methods that require internal model access
- Provides a robust approach for verifying LLM reliability in production environments
- Addresses critical security and trustworthiness concerns in deployed LLM systems
This advancement is particularly valuable for security professionals who need to evaluate third-party LLMs where internal access is restricted, helping organizations deploy AI systems with greater confidence in their reliability and safety.
Learning on LLM Output Signatures for gray-box LLM Behavior Analysis