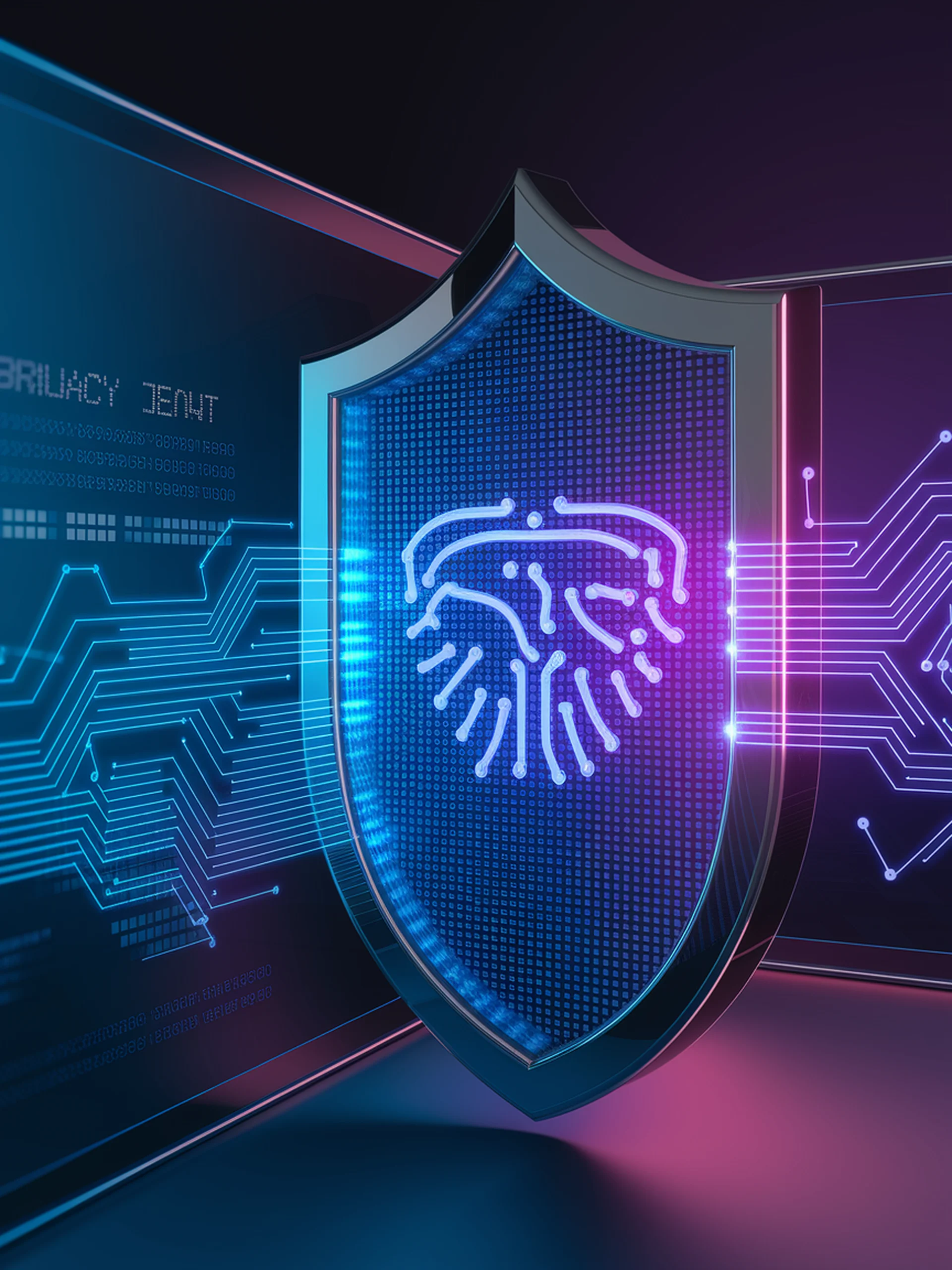
Safeguarding Privacy in Multimodal AI
Introducing MLLMU-Bench: The First Benchmark for Multimodal Model Privacy Protection
This research introduces the first comprehensive benchmark for assessing and mitigating privacy risks in multimodal large language models (MLLMs).
Key innovations:
- Creates MLLMU-Bench, the first benchmark specifically designed for multimodal model unlearning
- Addresses critical gaps in privacy protection for models that process both text and visual data
- Enables measurement and improvement of privacy safeguards without sacrificing model performance
- Provides a standardized framework for evaluating multimodal AI systems' privacy compliance
This work has significant security implications as organizations deploy increasingly sophisticated multimodal AI systems that may inadvertently memorize and expose confidential user information, creating legal and ethical risks.
Protecting Privacy in Multimodal Large Language Models with MLLMU-Bench