Safety Engineering for ML-Powered Systems
Research on proactive approaches and methodologies to identify, evaluate, and mitigate safety risks in ML-powered systems through systematic safety engineering practices
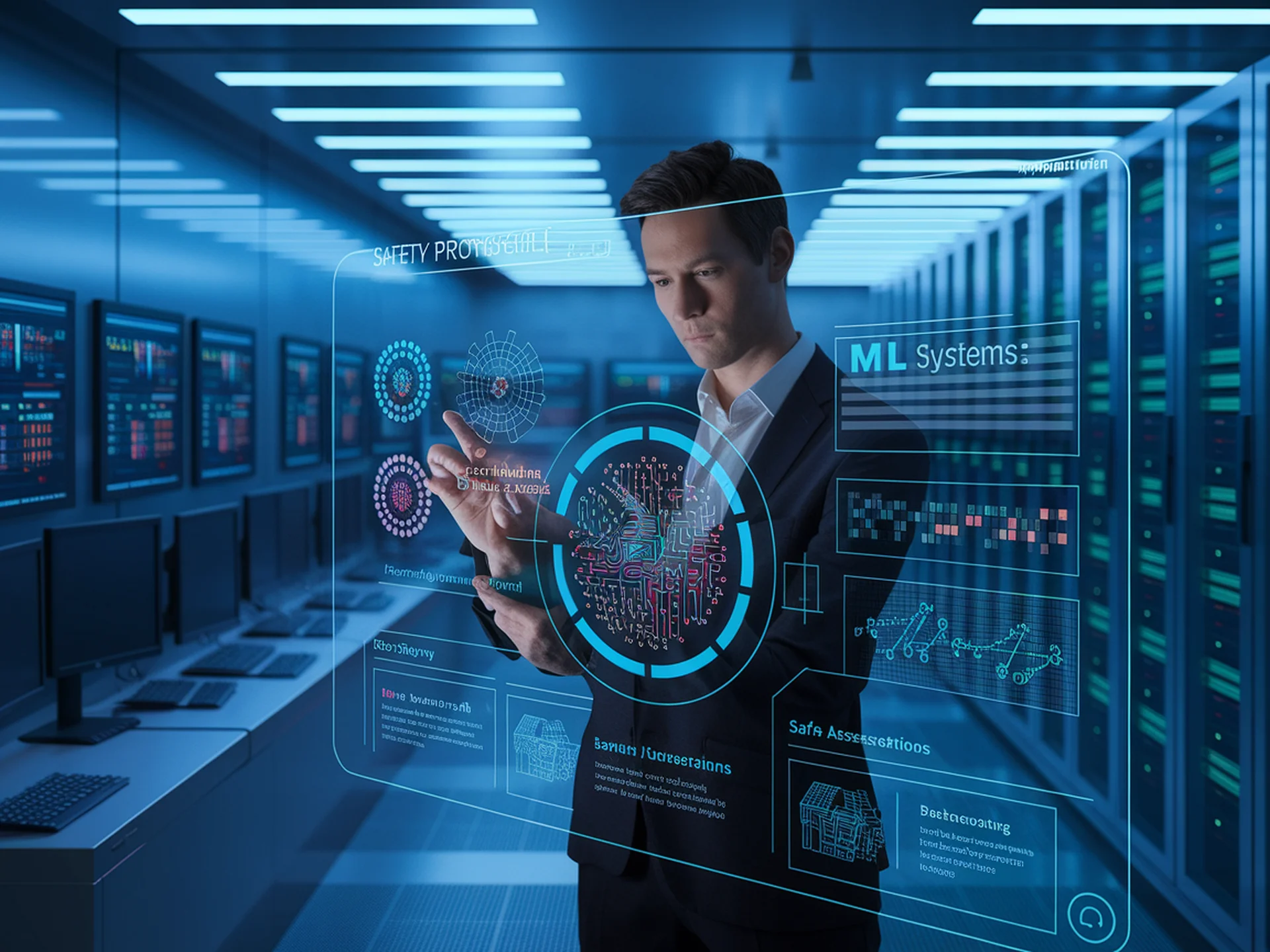
Safety Engineering for ML-Powered Systems
Research on Large Language Models in Safety Engineering for ML-Powered Systems
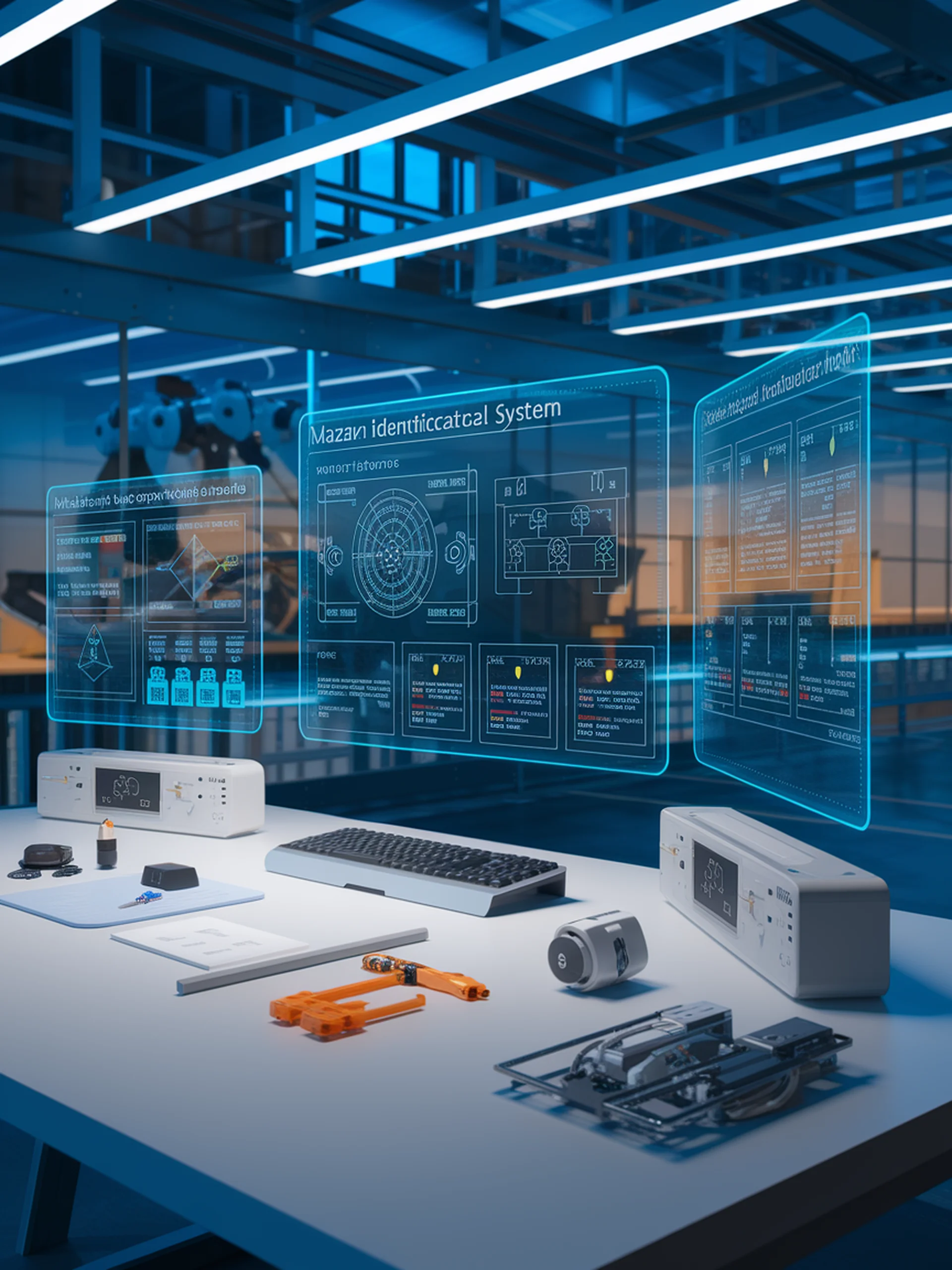
Proactive Safety for ML Systems
Using LLMs to enhance safety engineering for machine learning applications
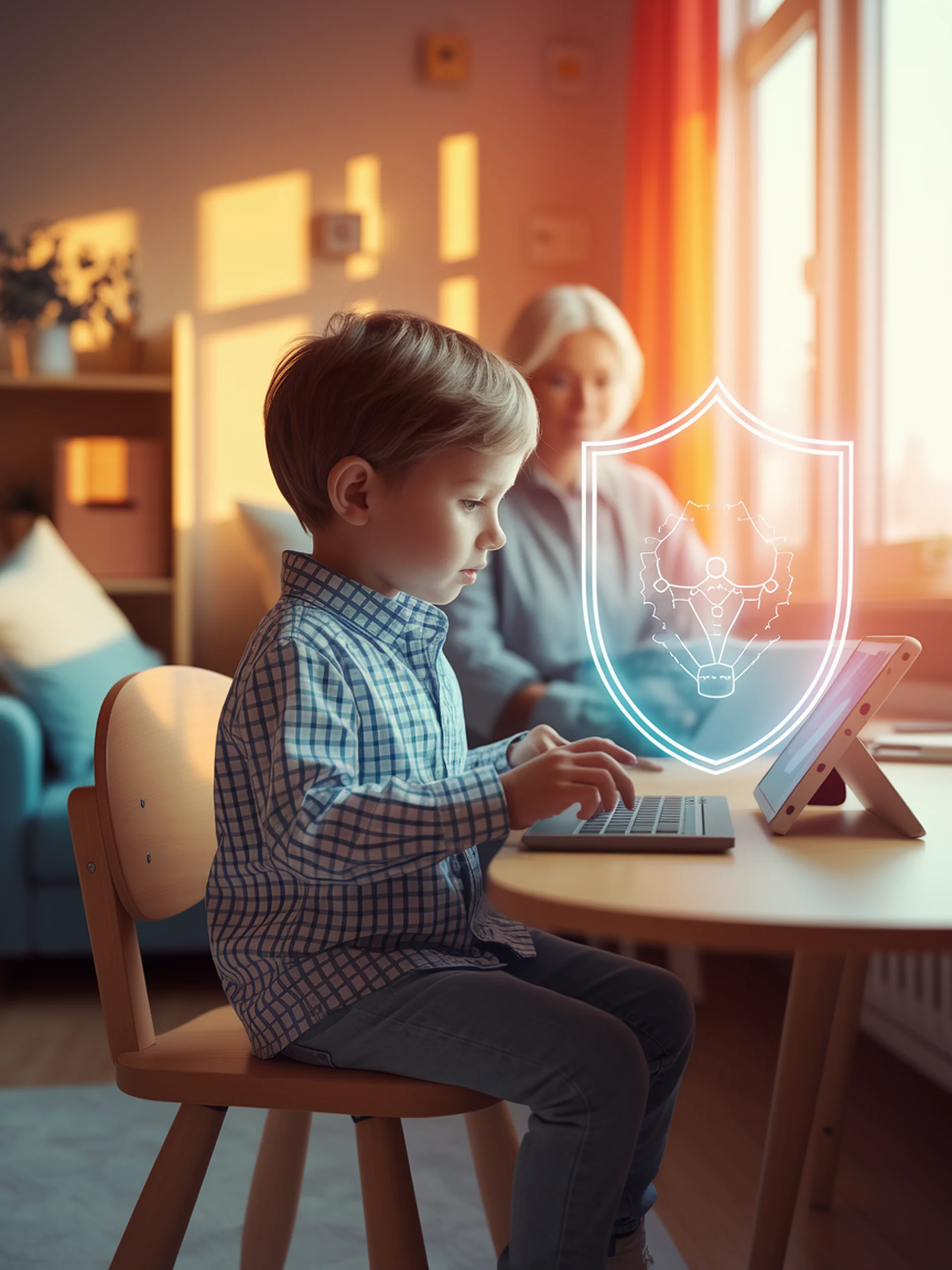
Safeguarding Children in the AI Era
A protection framework for child-LLM interactions
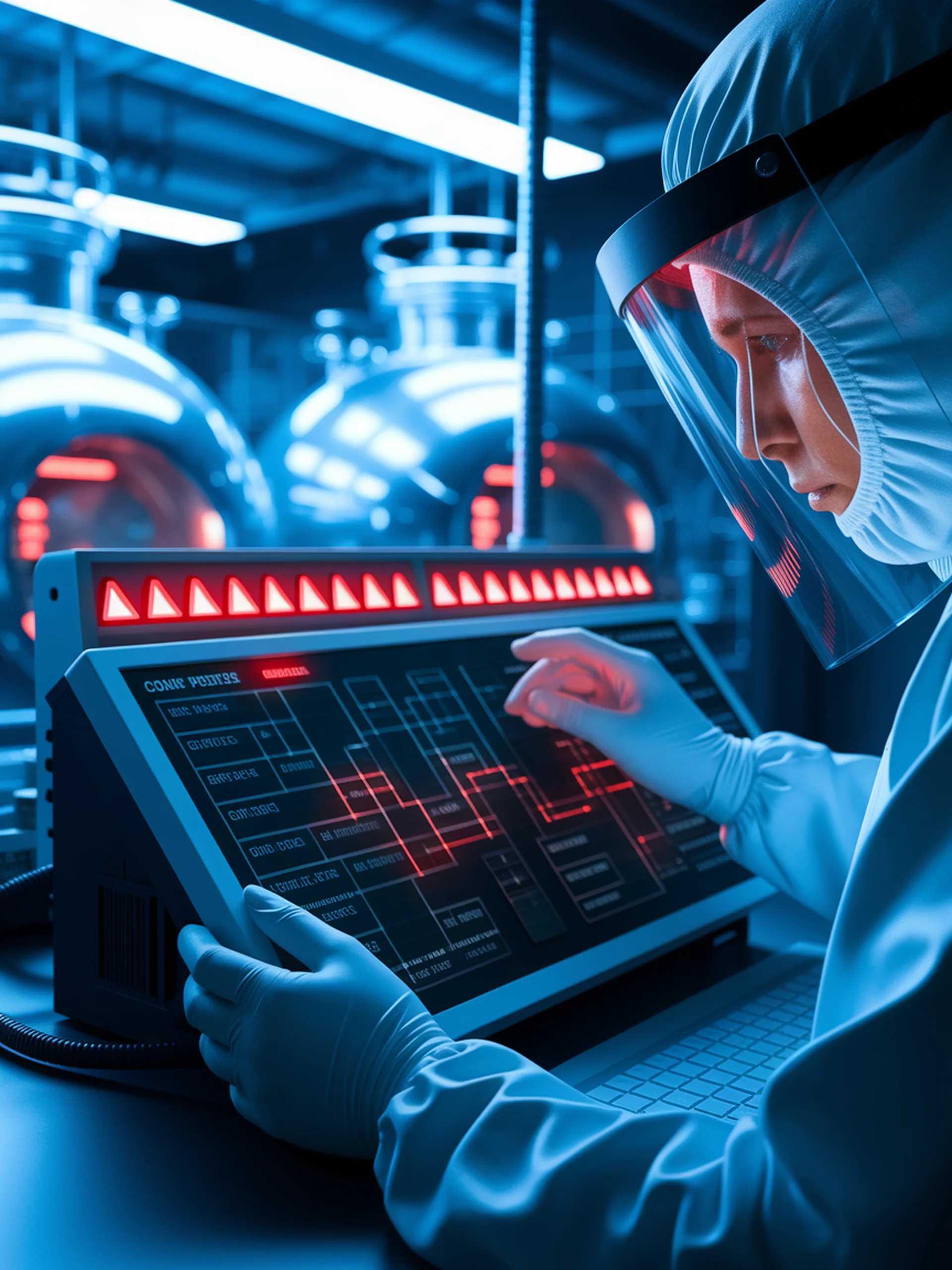
Catastrophic Risks in Autonomous LLM Decision-Making
New evaluation framework reveals security vulnerabilities in CBRN scenarios
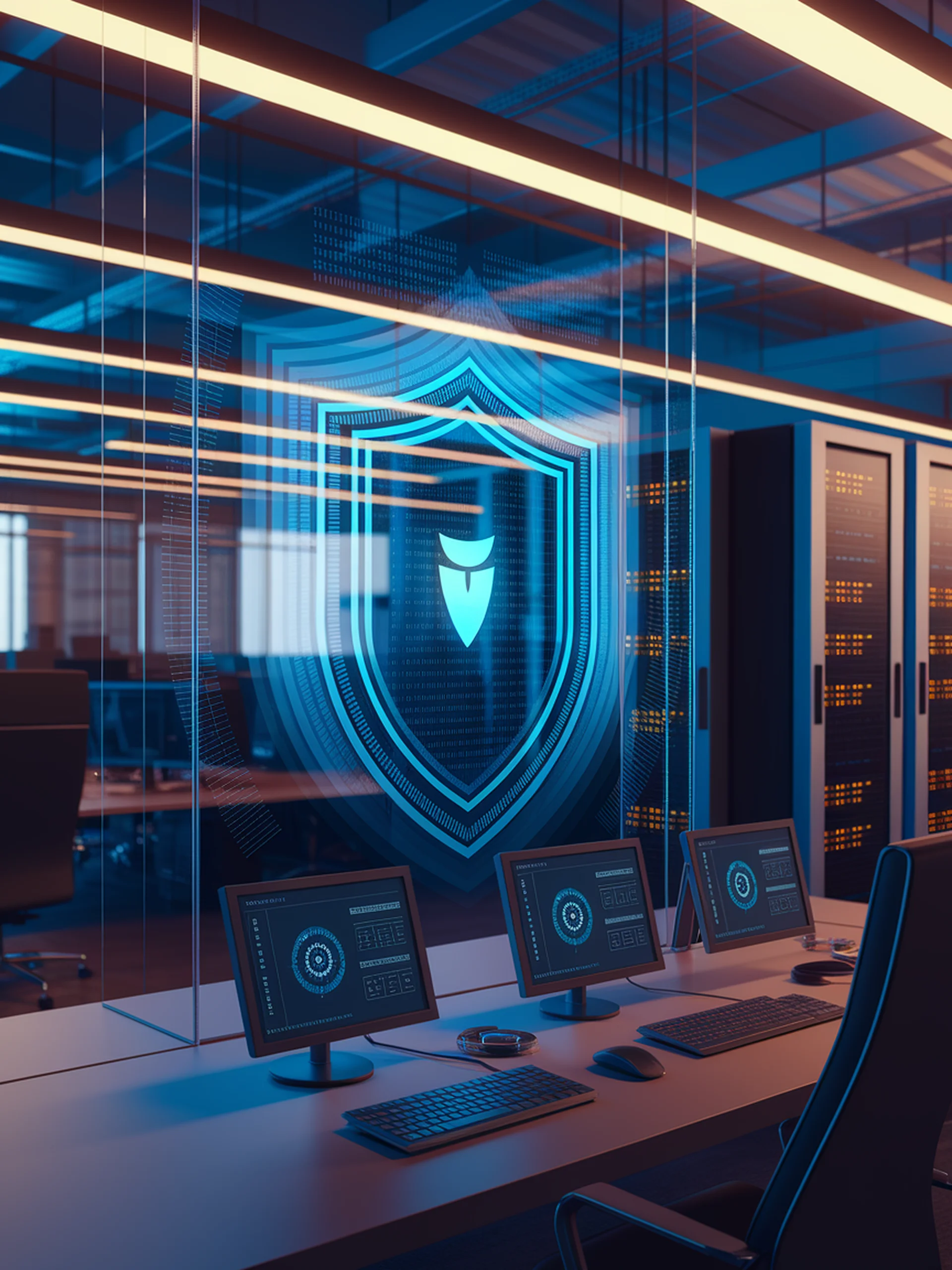
Safeguarding AI Agents in the Wild
AGrail: A Dynamic Safety System for LLM-Based Agents
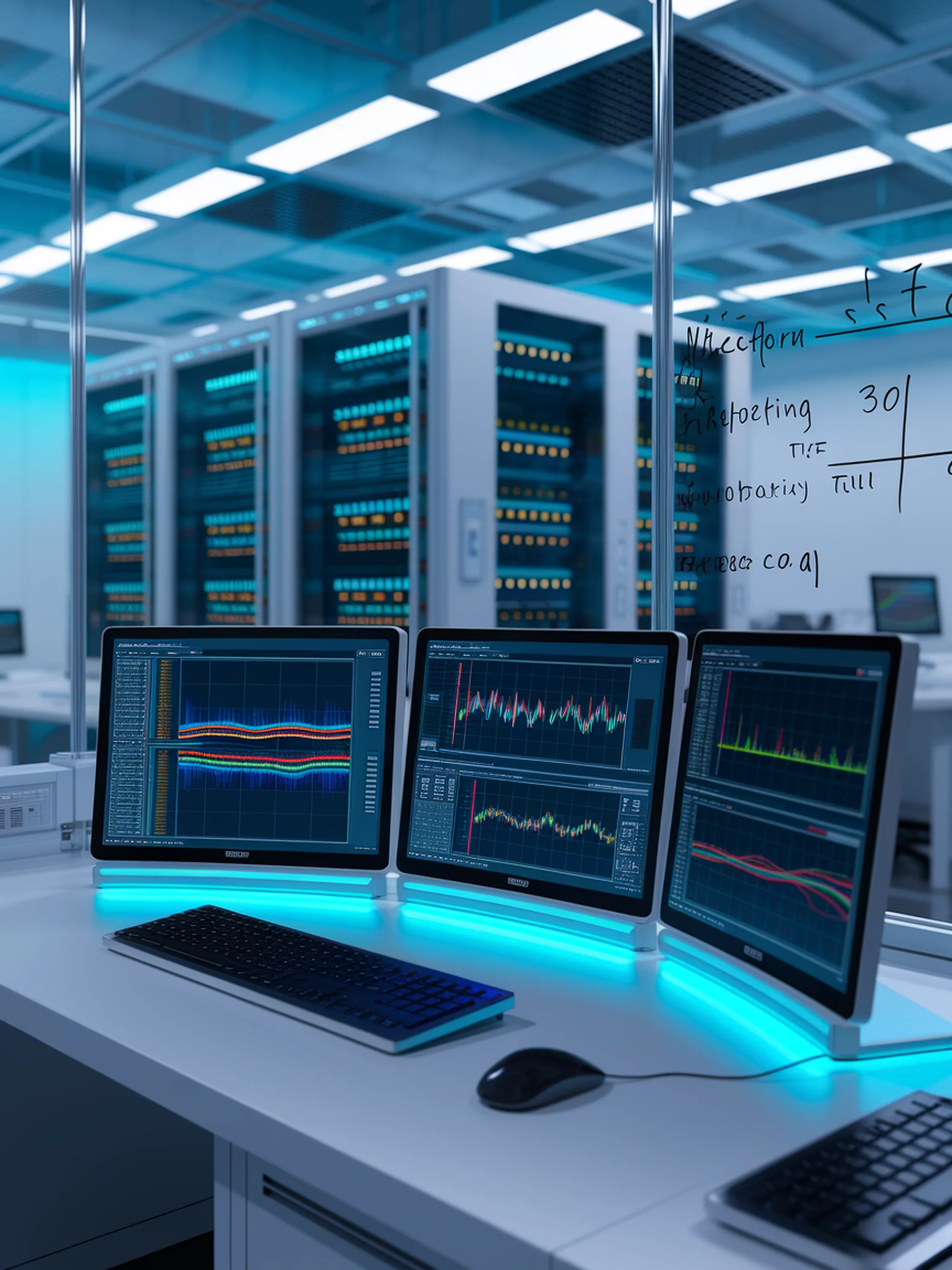
Signal Processing: A New Lens for AI Safety
Securing Generative AI through Signal Processing Principles
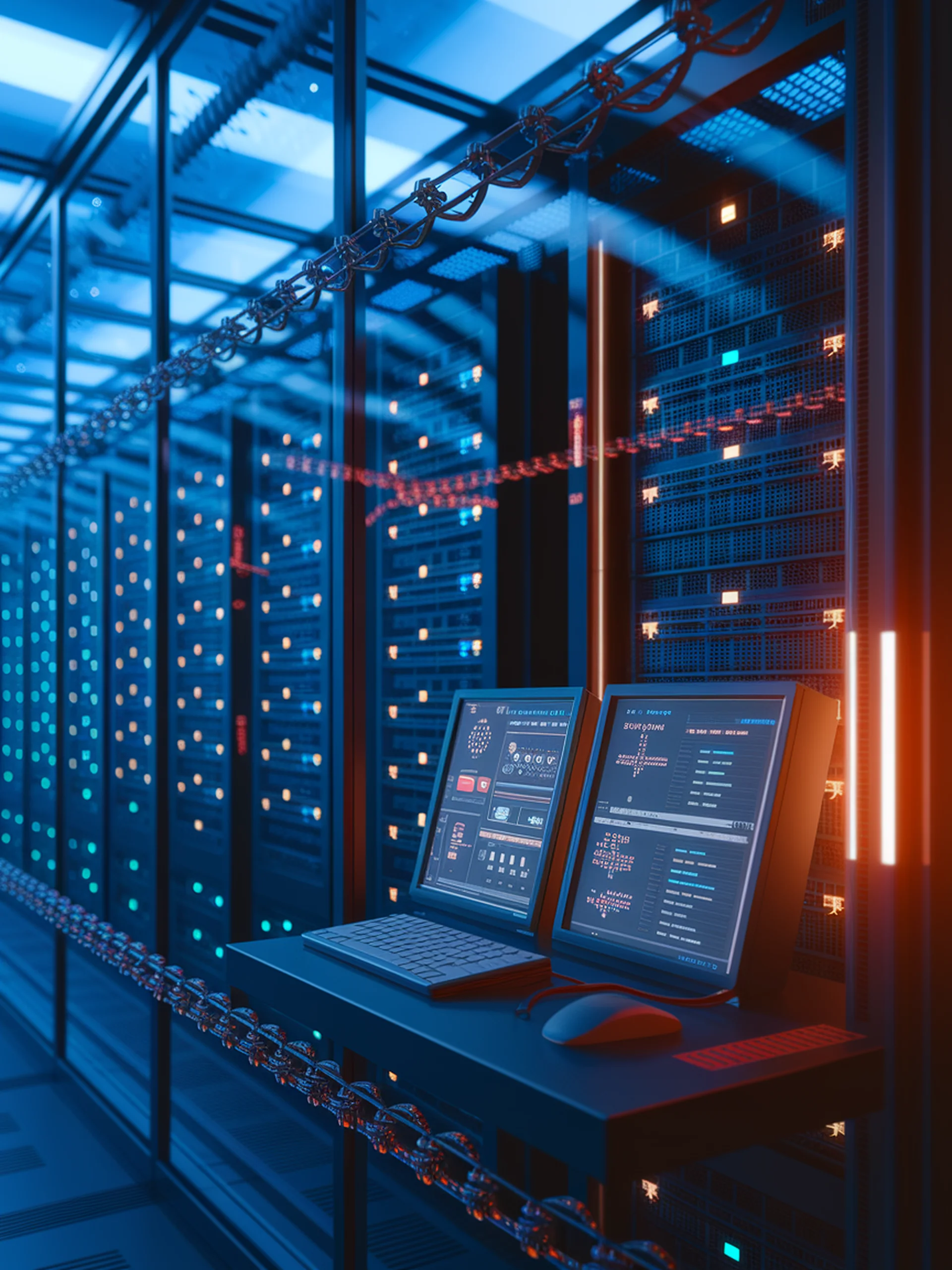
Securing the LLM Supply Chain
Uncovering Hidden Vulnerabilities Beyond Content Safety
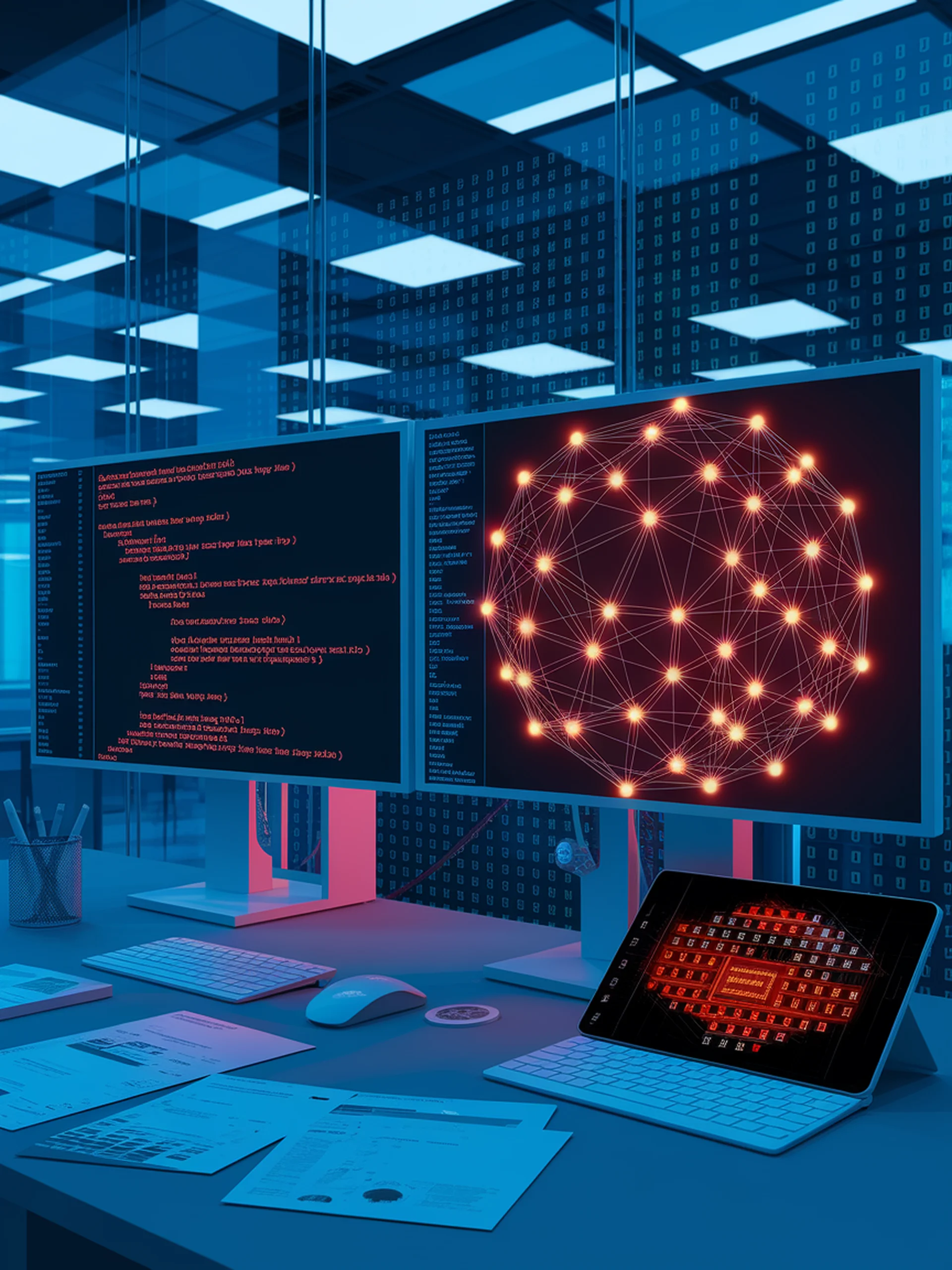
Safety Gaps in Large Reasoning Models
Uncovering security risks in advanced AI systems like DeepSeek-R1
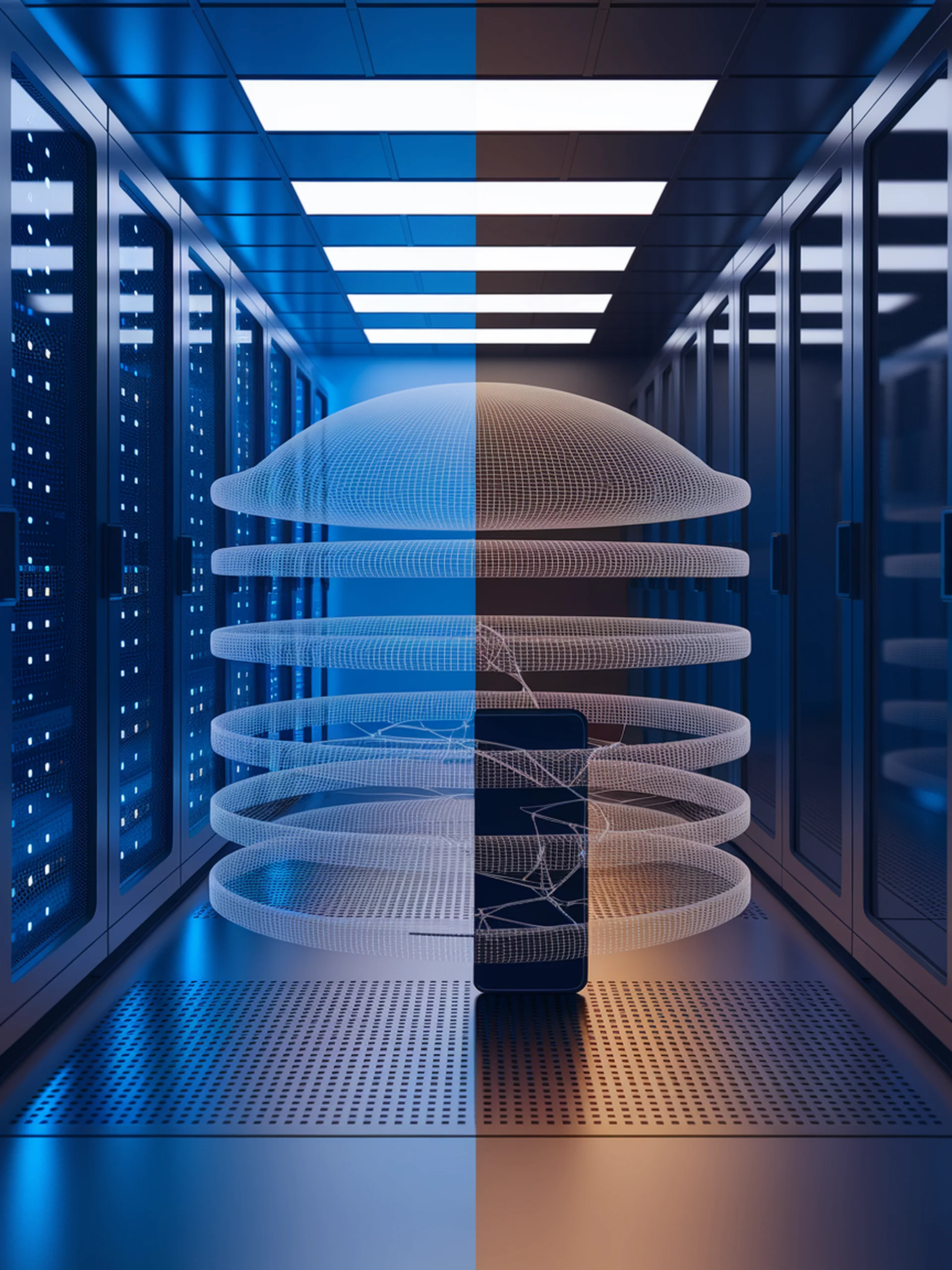
Quantization's Hidden Costs to LLM Safety
How model compression impacts security and reliability
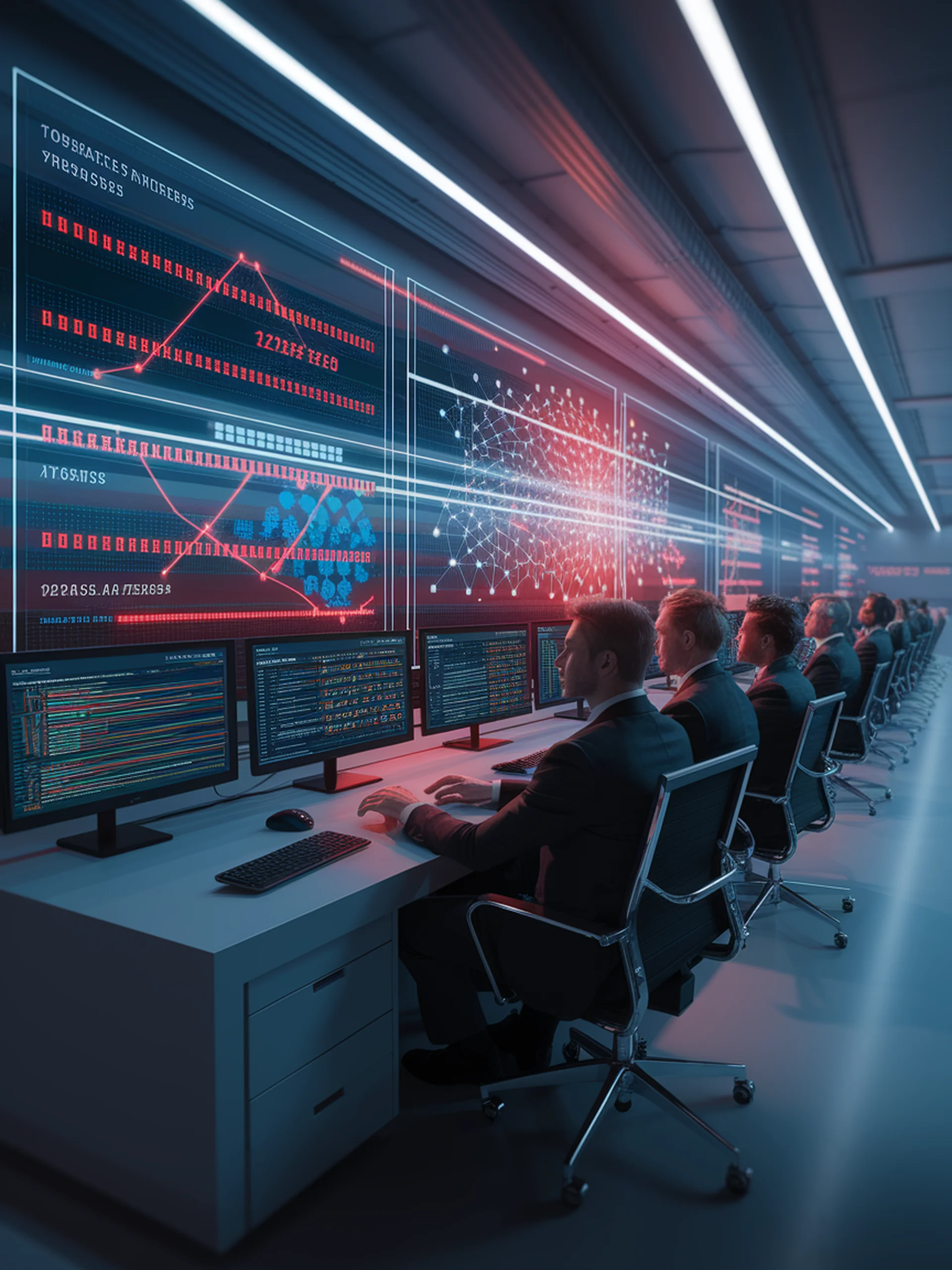
Predicting AI Risks Before They Scale
Forecasting rare but dangerous language model behaviors
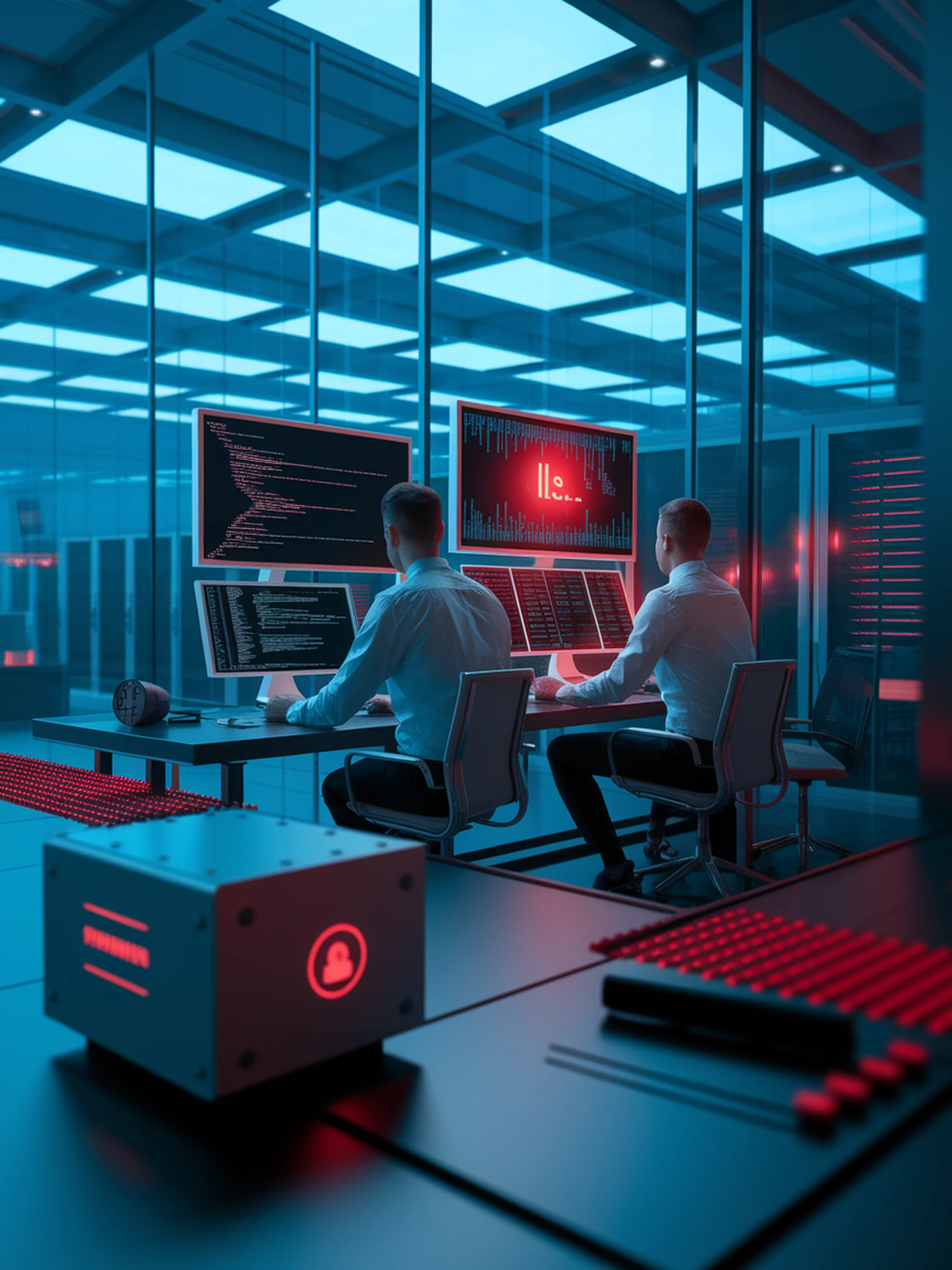
Red Teaming: The Offensive Security Strategy for LLMs
Proactively identifying vulnerabilities to build safer AI systems
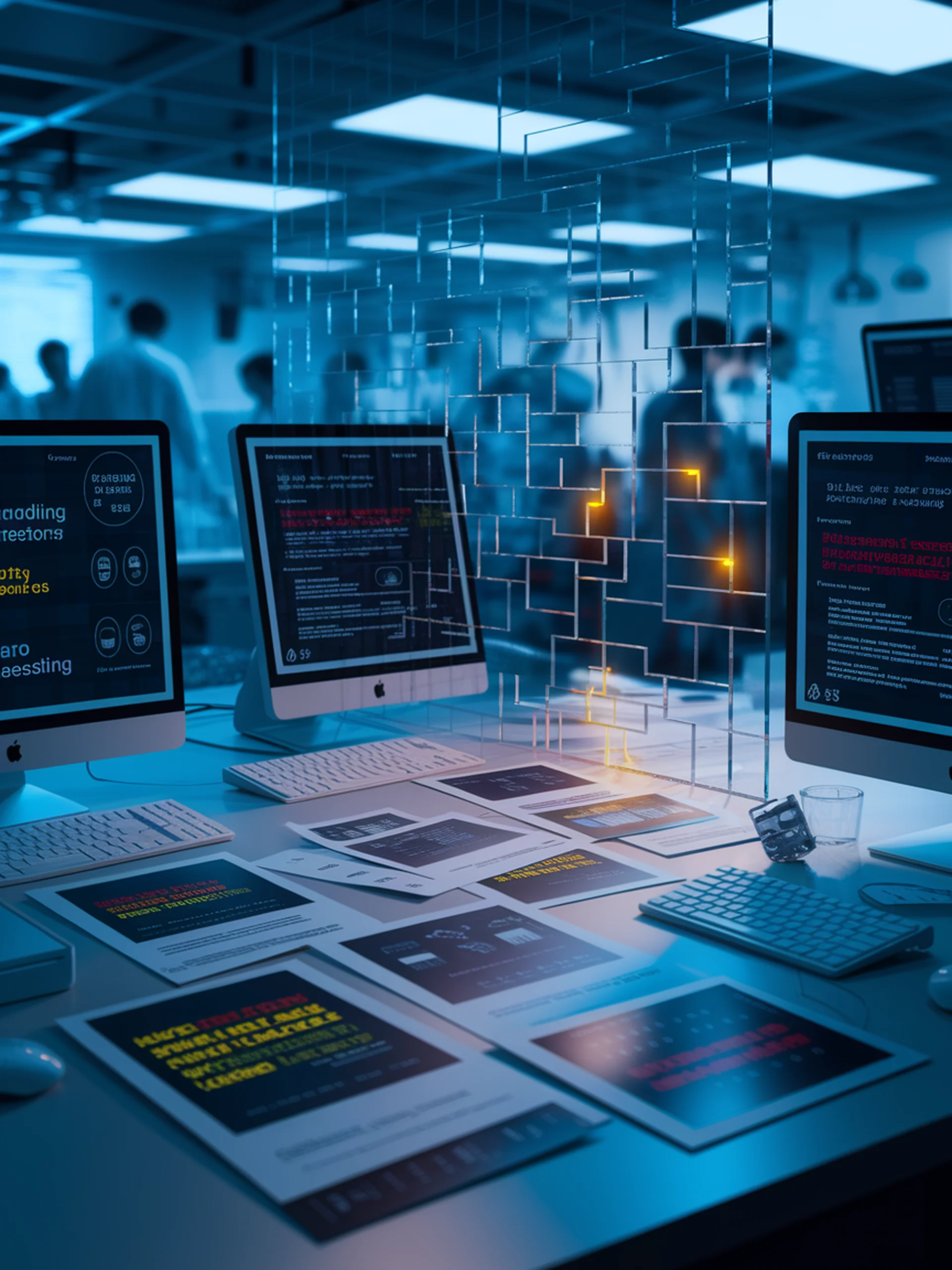
The Fragility of AI Safety Testing
Why Current LLM Safety Evaluations Need Improvement
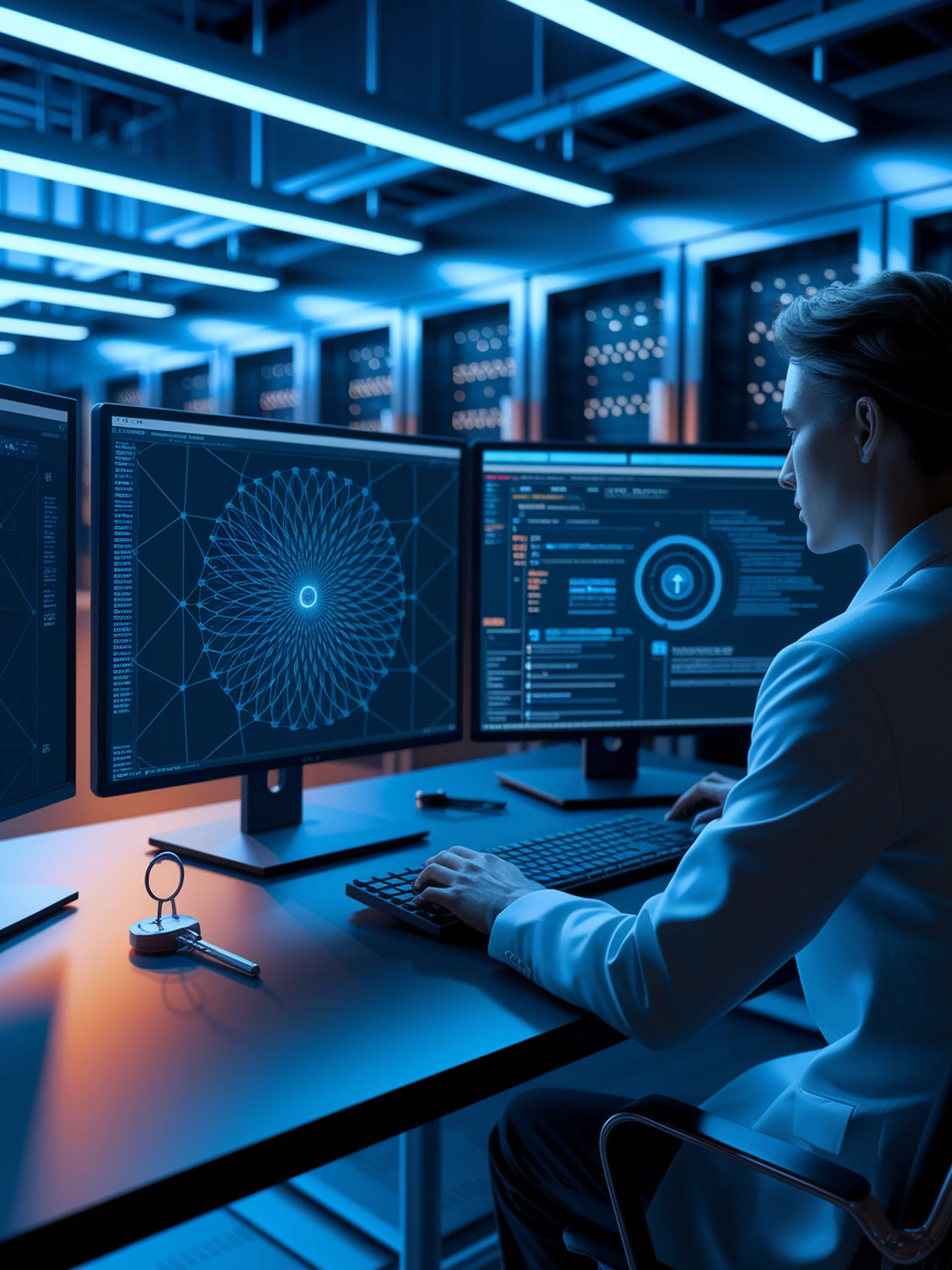
Overfitting in AI Alignment: A Security Challenge
Mitigating risks when training powerful AI systems with weaker supervisors
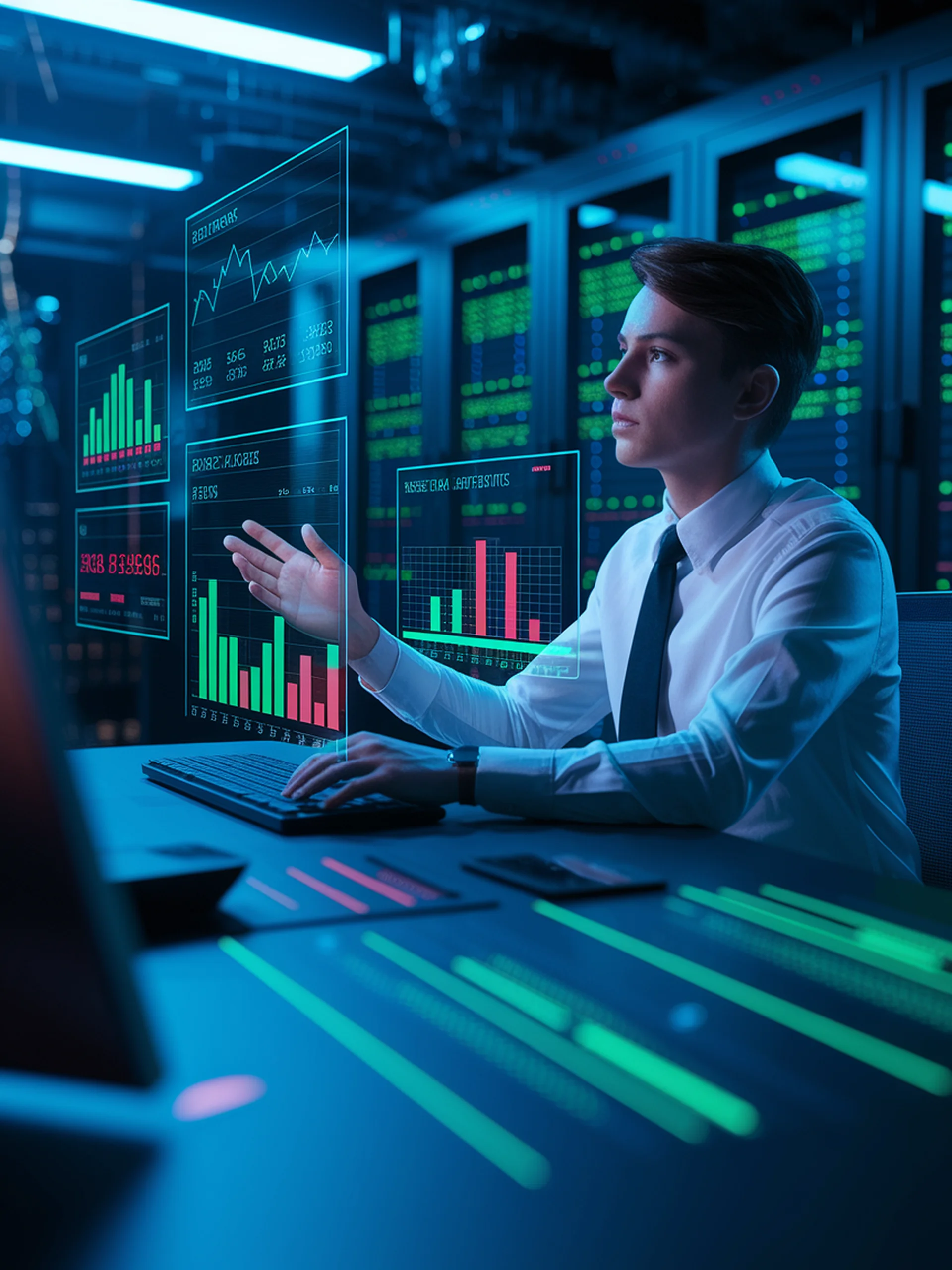
Quantifying AI Risk: Beyond Capabilities
Translating LLM benchmark data into actionable risk estimates
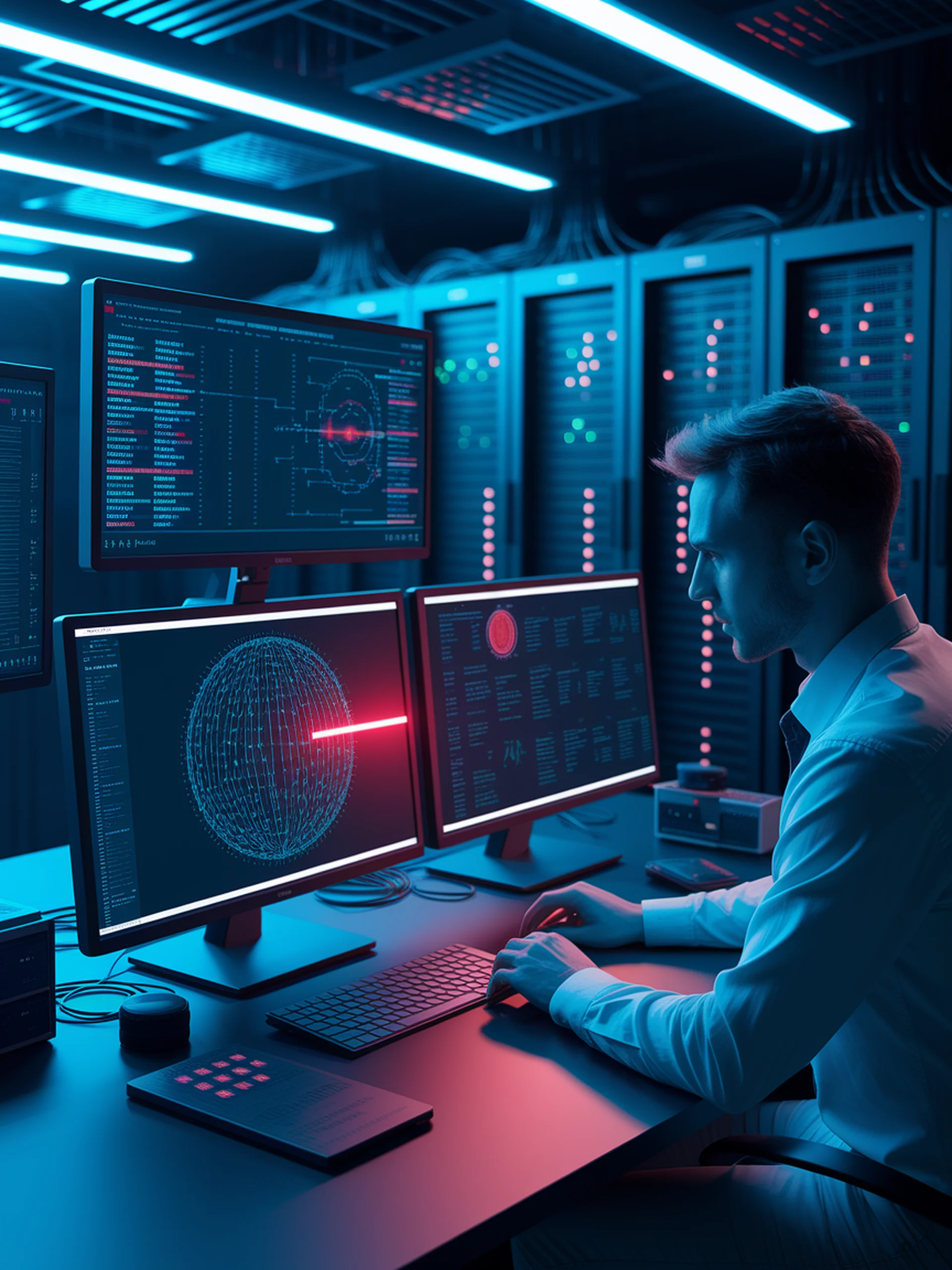
Blind Spots in AI Safety Judges
Evaluating the reliability of LLM safety evaluation systems
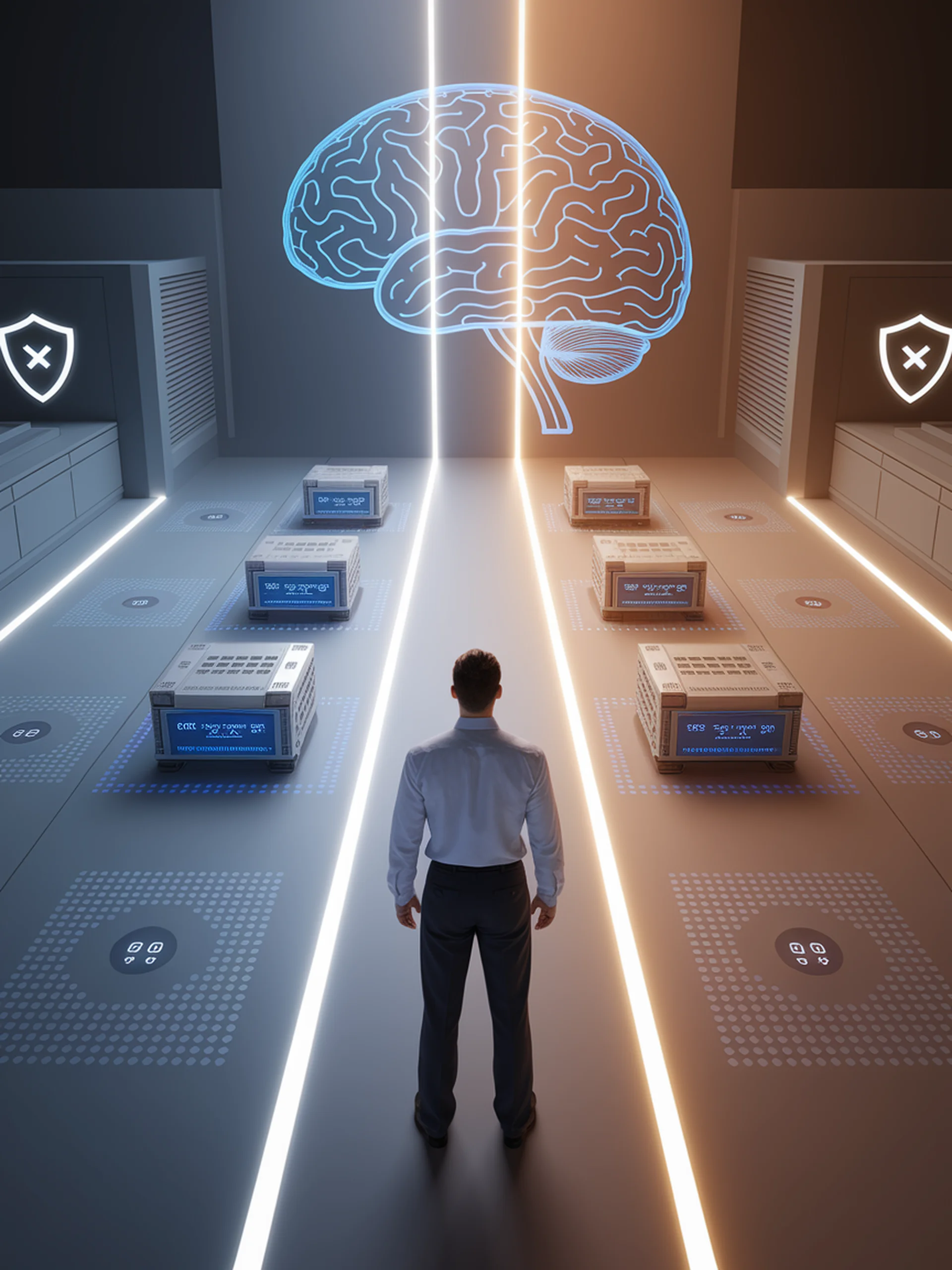
The Case Against AGI
Why Specialized AI Systems Offer Better Security and Value
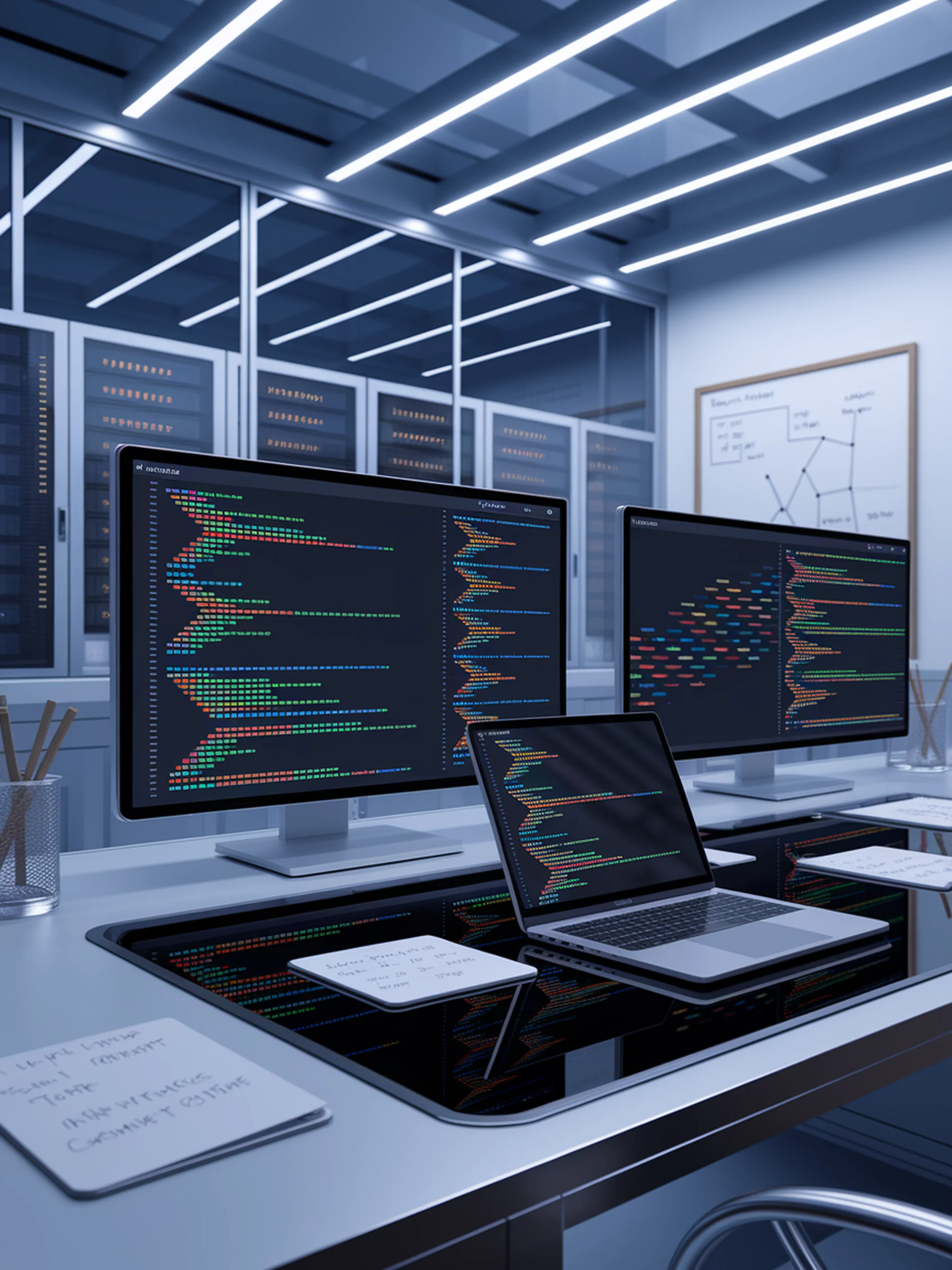
Testing LLM Prompts: The Next Frontier
Automated testing for prompt engineering quality assurance
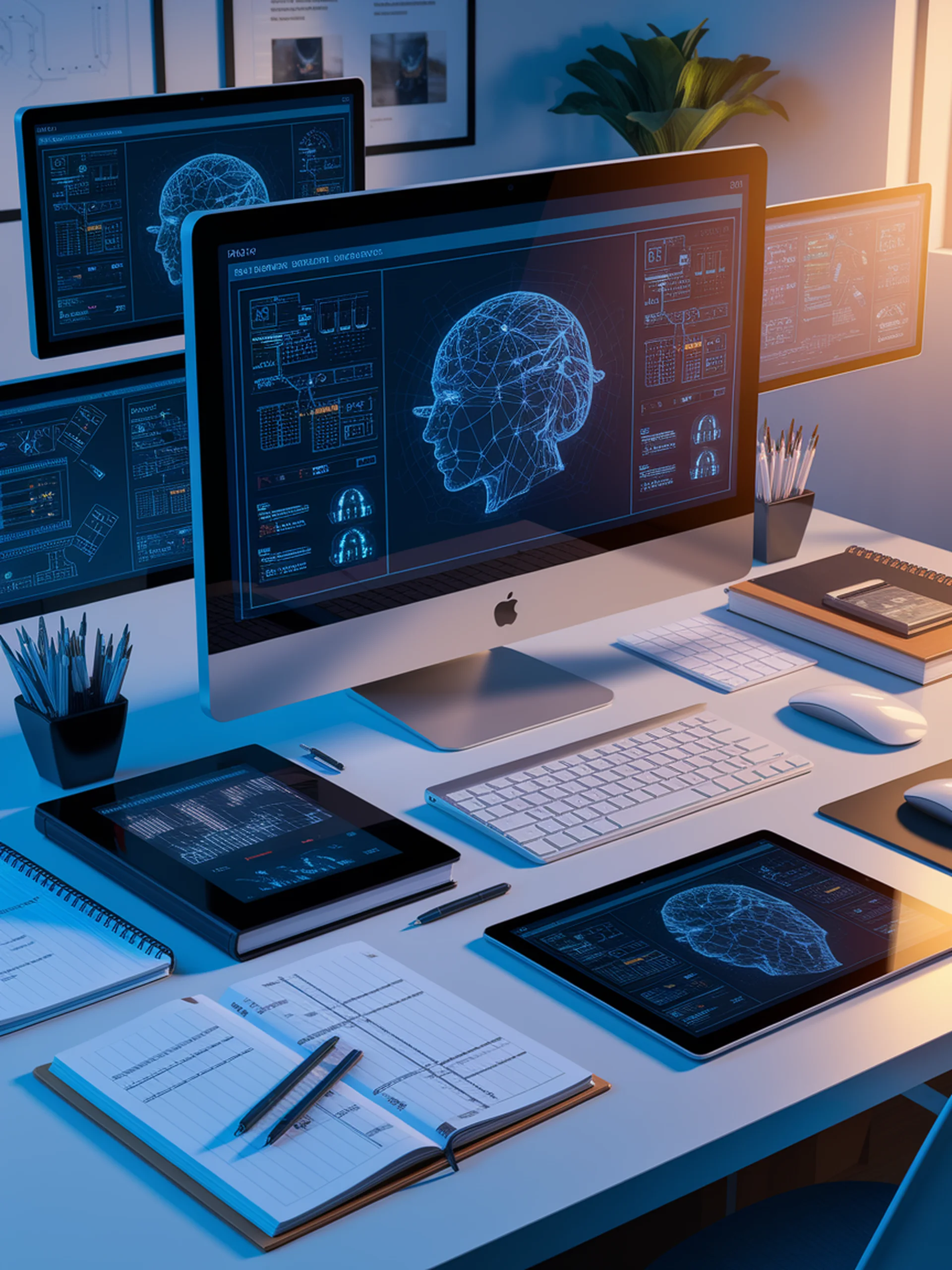
AI-Powered Safety Analysis
Streamlining STPA with Large Language Models
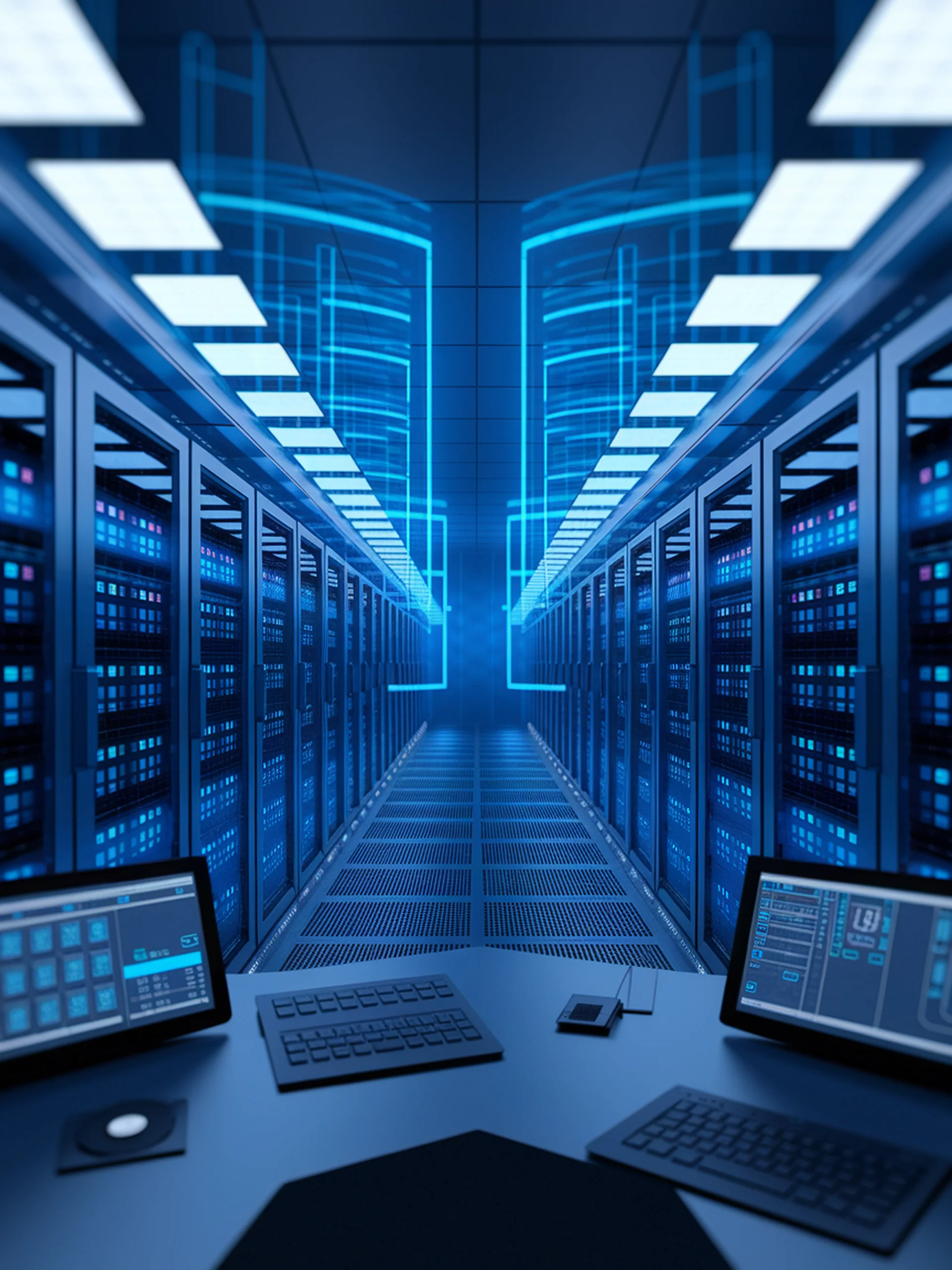
Securing LLMs in the Cloud
Adaptive Fault Tolerance for Reliable AI Infrastructure
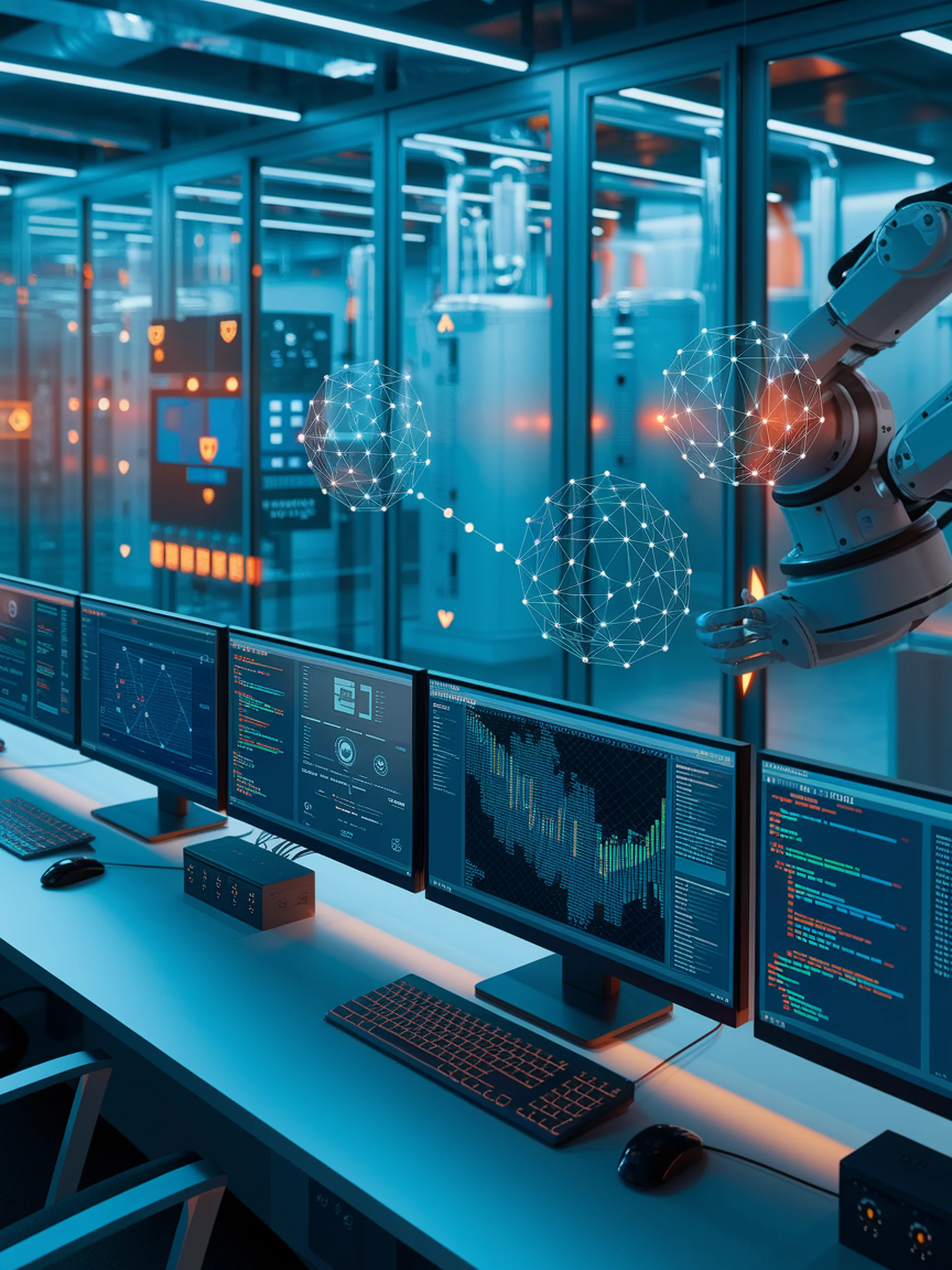
Securing Cyber-Physical Systems with AI
Automated Safety-Compliant LTL Generation Using Large Language Models
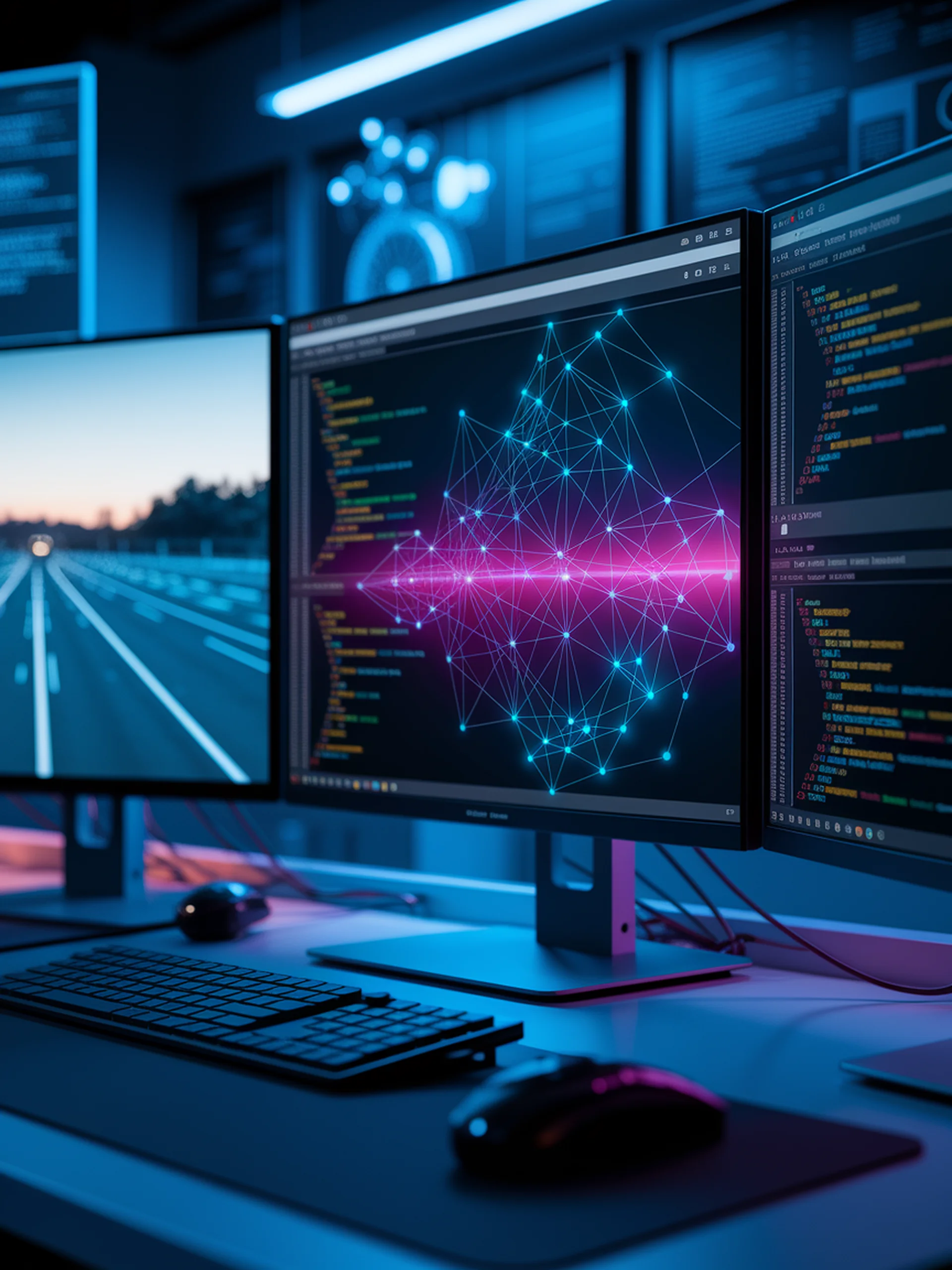
Testing DNNs Without Ground Truth
Using GANs to enable simulator-based testing for safety-critical systems
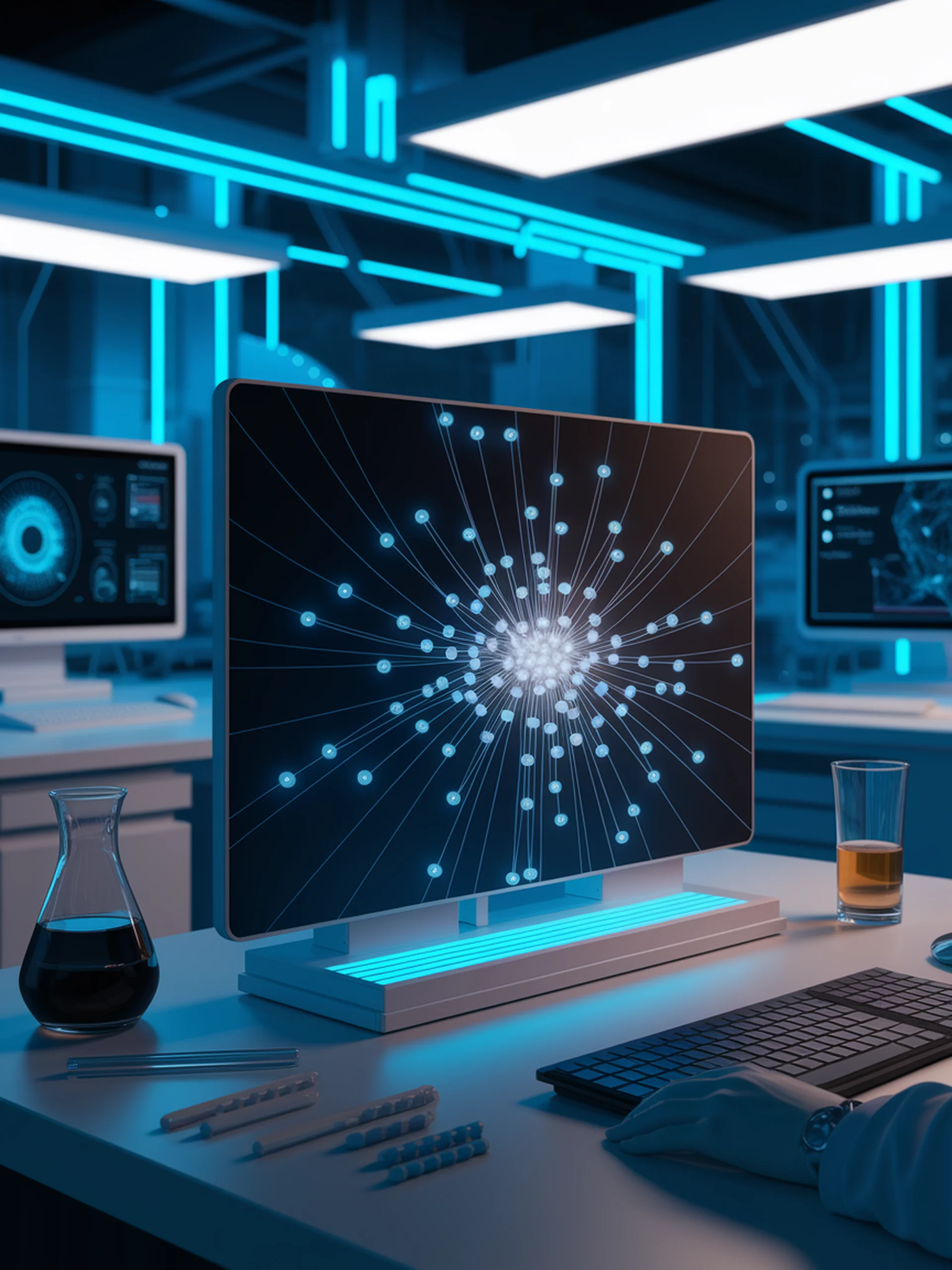
Building Safer LLMs with Sparse Representation Steering
A novel approach to controlling LLM behavior without retraining
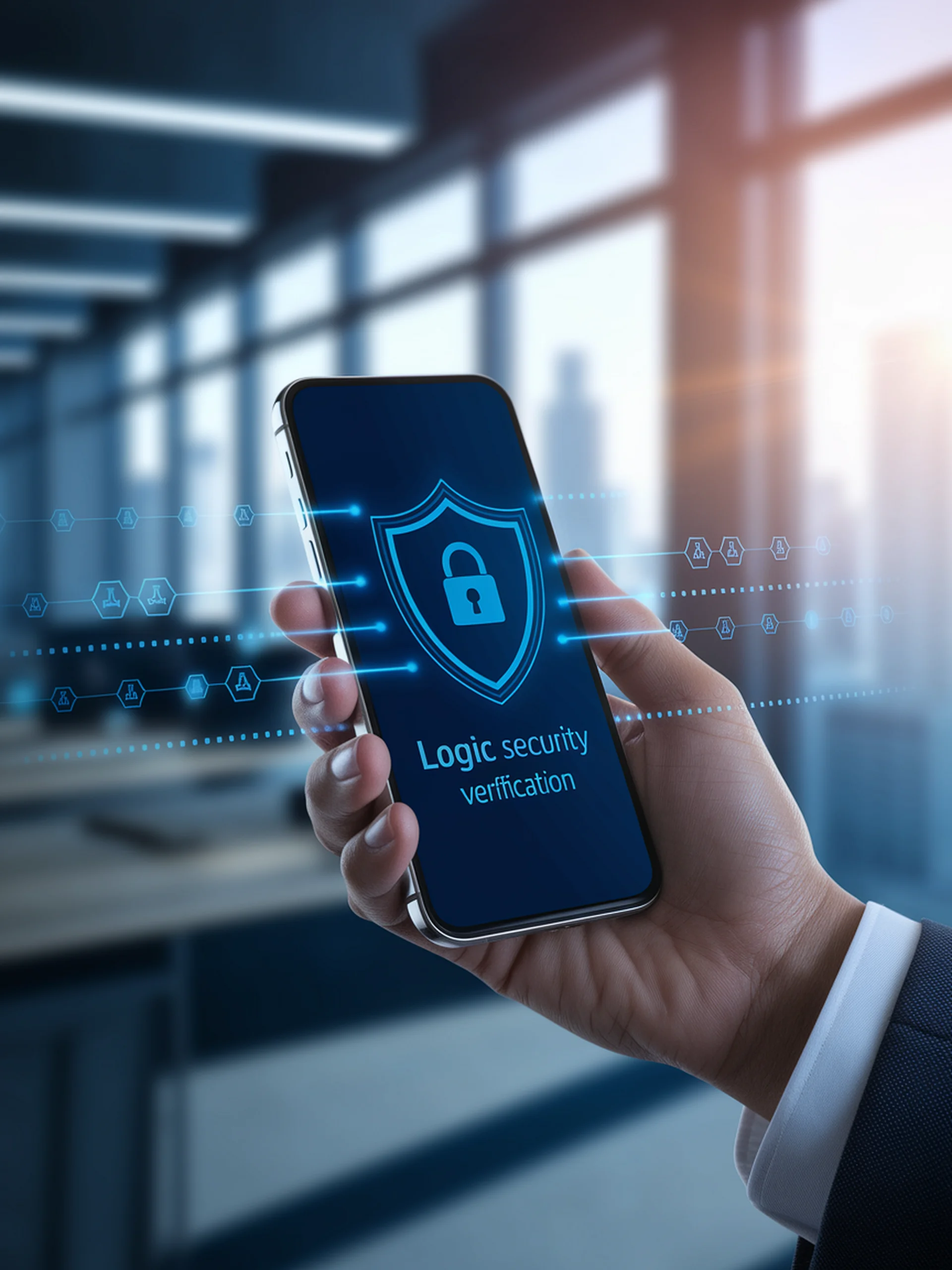
Securing AI Mobile Assistants
Logic-based verification prevents unauthorized or harmful actions
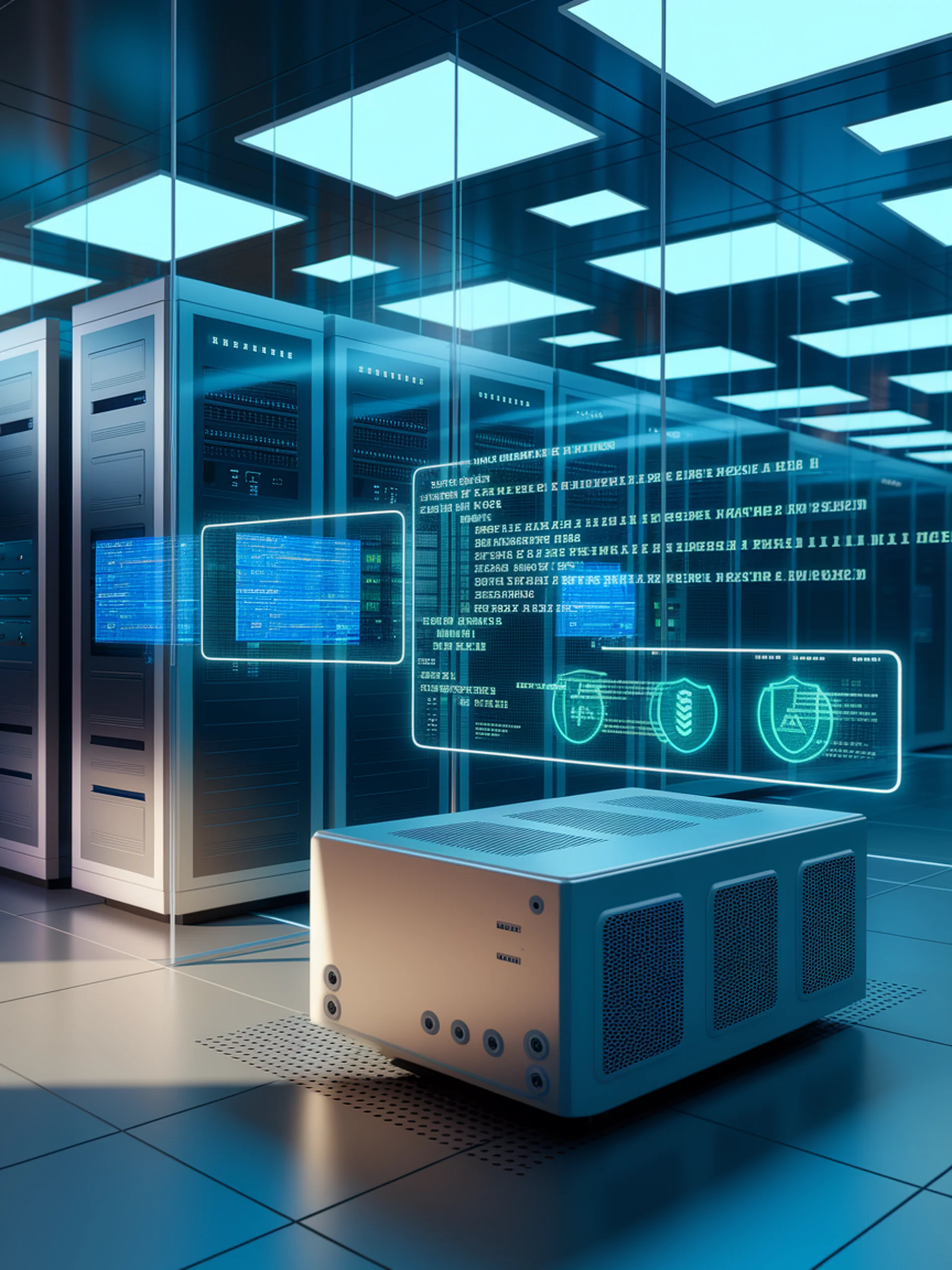
Safer Fine-Tuning for Language Models
Preserving Safety Alignment During Model Adaptation
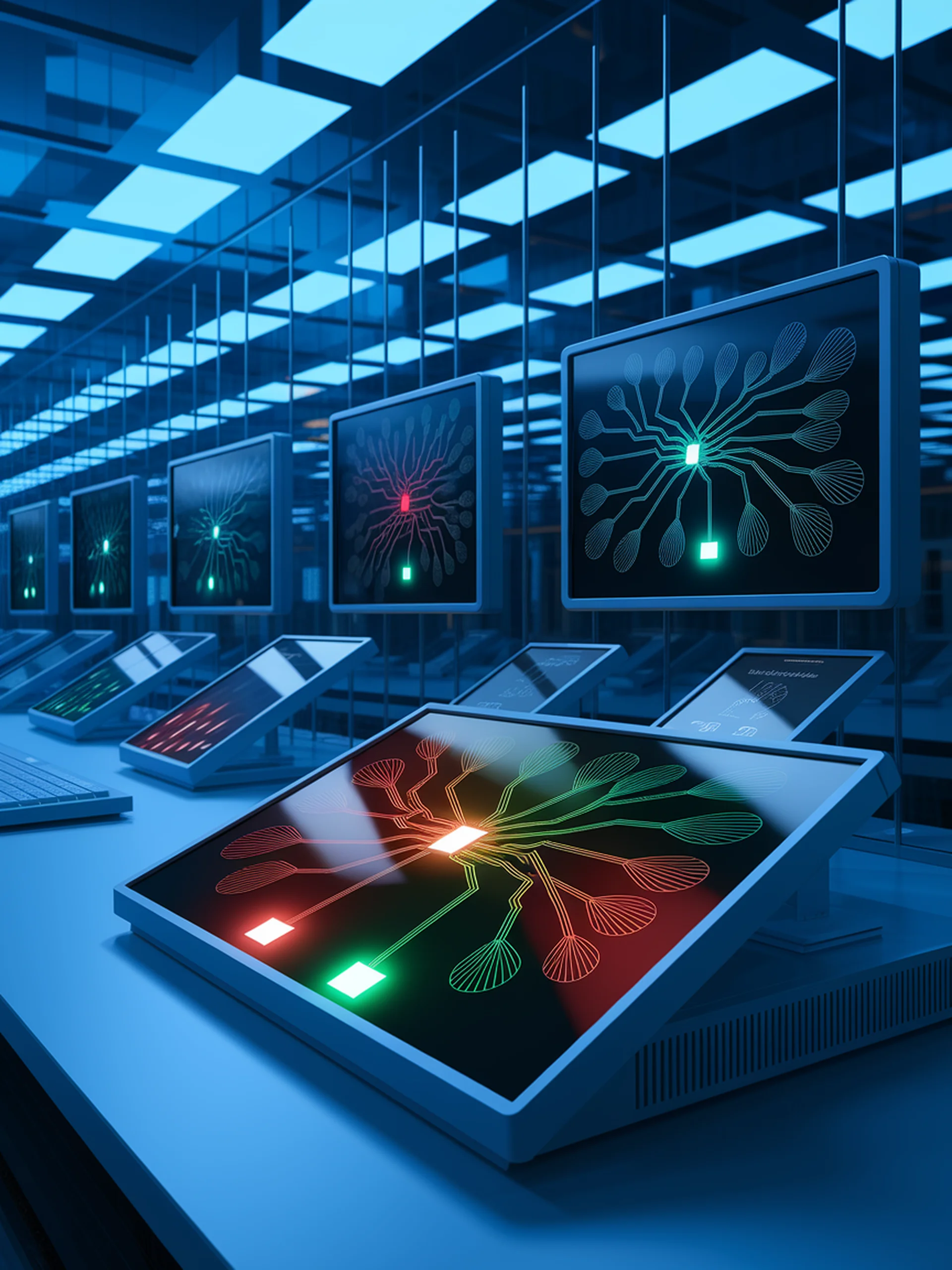
Preventing Harmful AI Content Through Preemptive Reasoning
A novel approach that teaches LLMs to identify risks before generating content
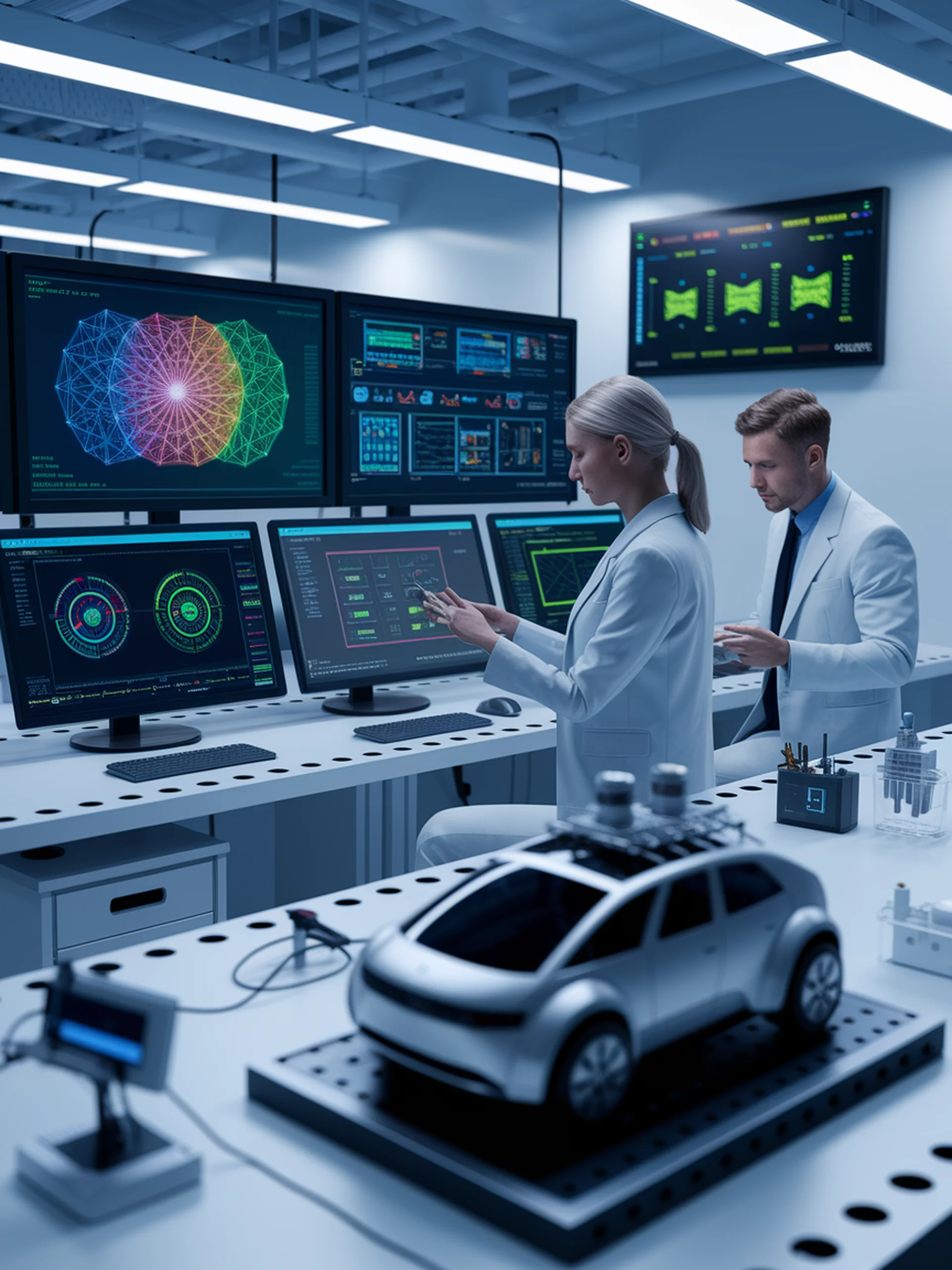