Trust, Reliability, and Hallucination Mitigation
Research on addressing hallucinations, improving trustworthiness, and ensuring reliable outputs from LLMs
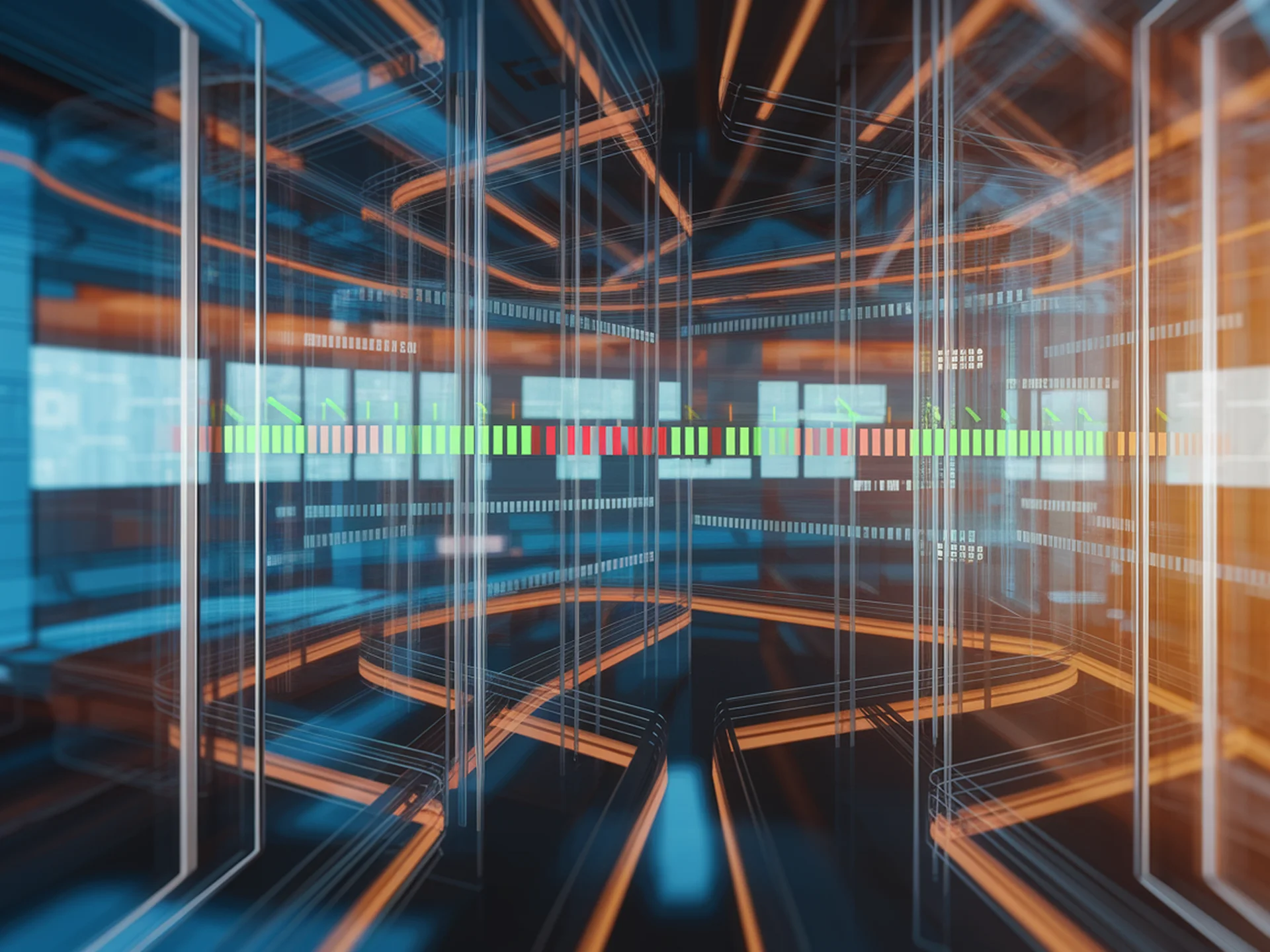
Trust, Reliability, and Hallucination Mitigation
Research on Large Language Models in Trust, Reliability, and Hallucination Mitigation
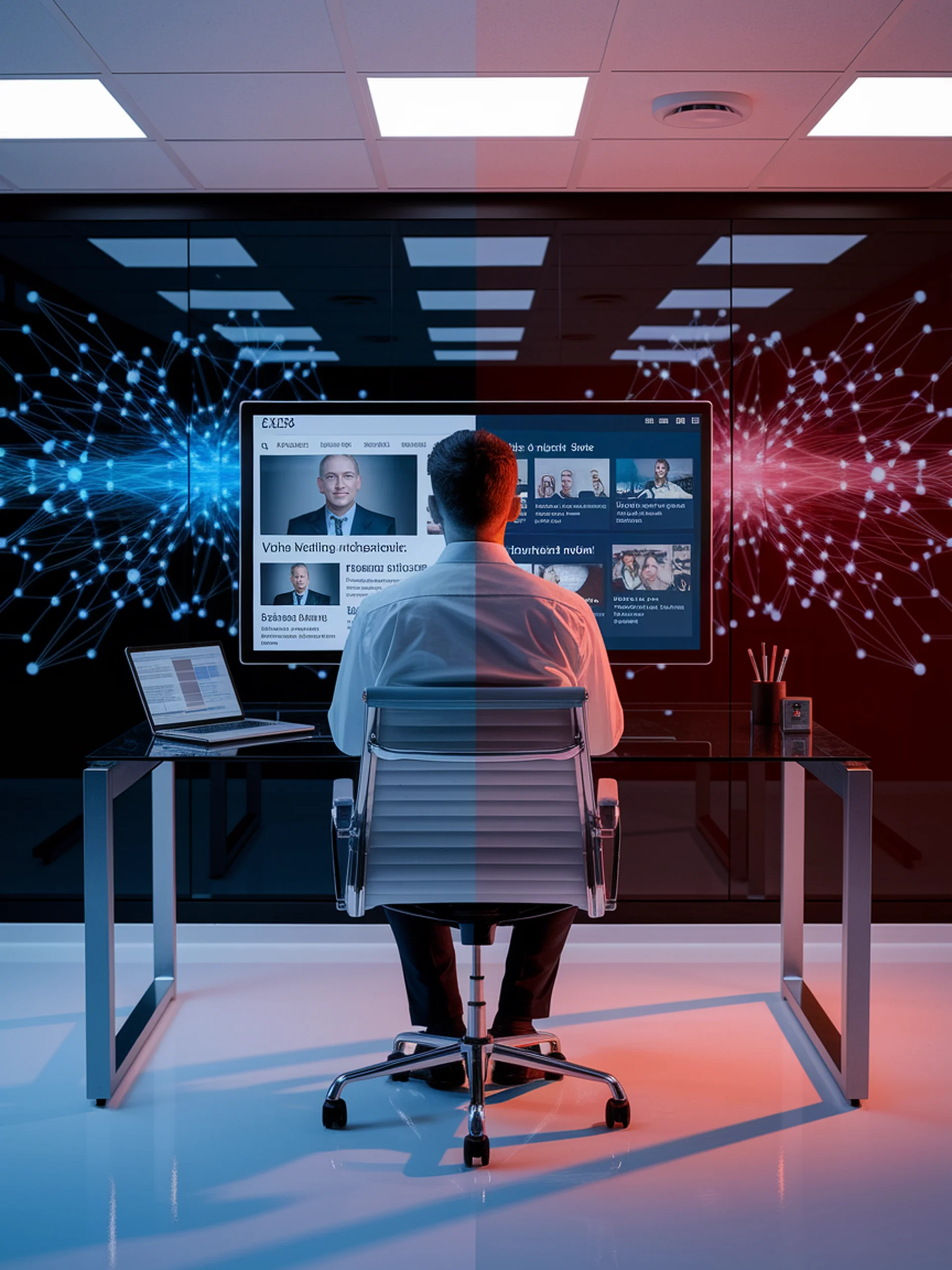
Can AI Distinguish Truth from Fiction?
Evaluating LLMs' Ability to Assess News Source Credibility
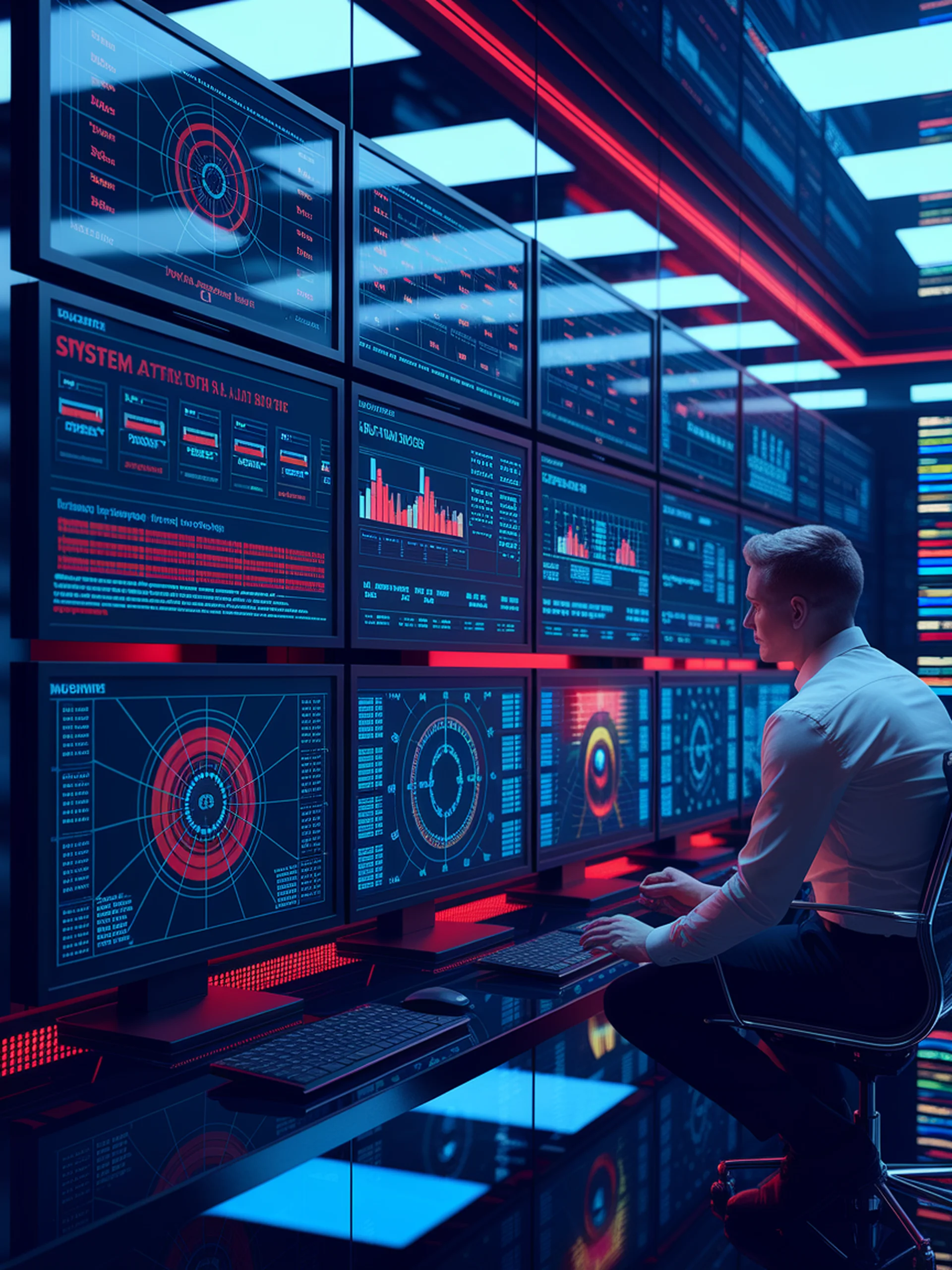
The Dark Side of AI: Trustworthiness Risks
Analyzing security vulnerabilities in generative AI models
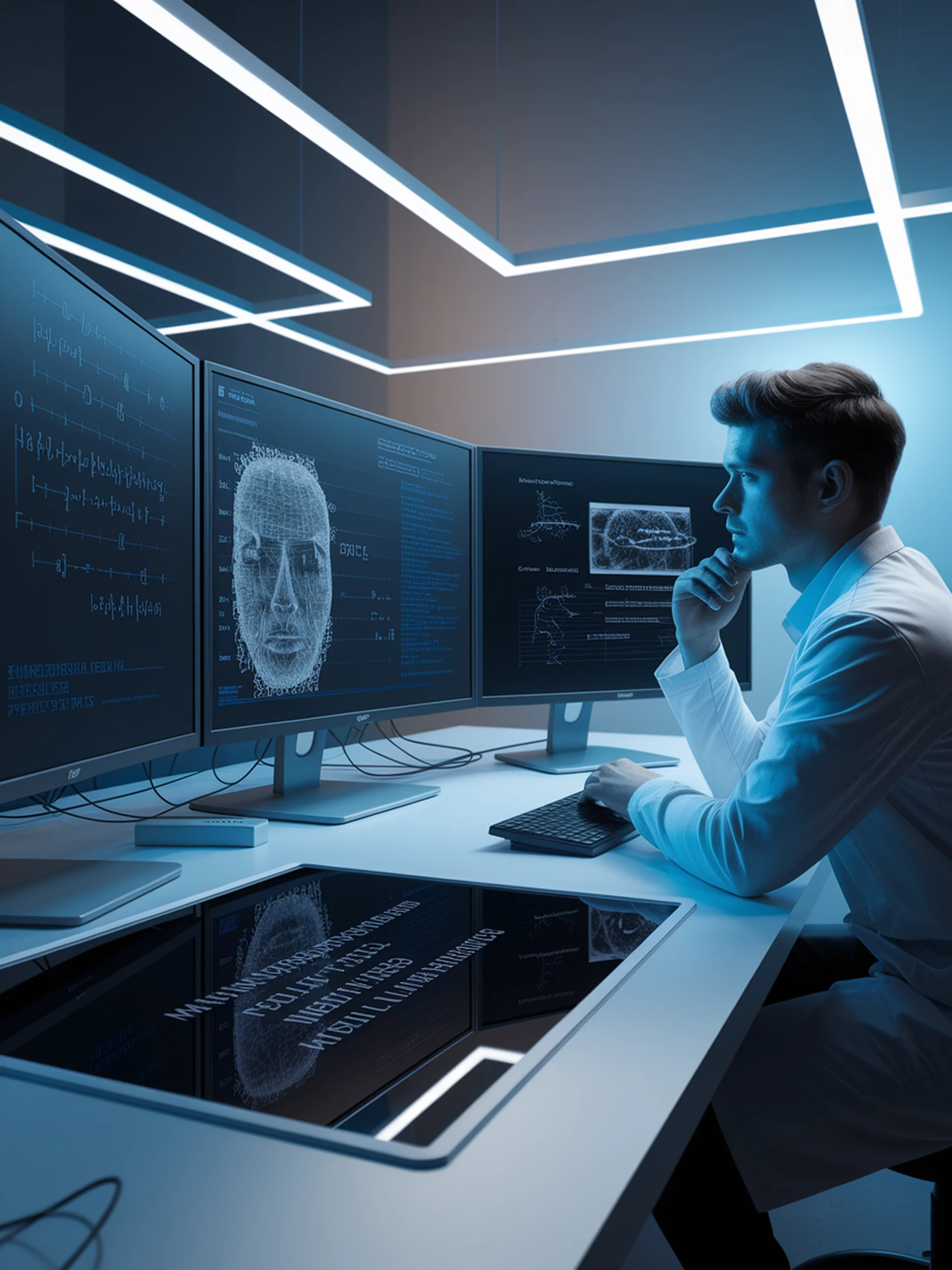
The Inevitable Reality of LLM Hallucinations
Why eliminating hallucinations in AI models is mathematically impossible
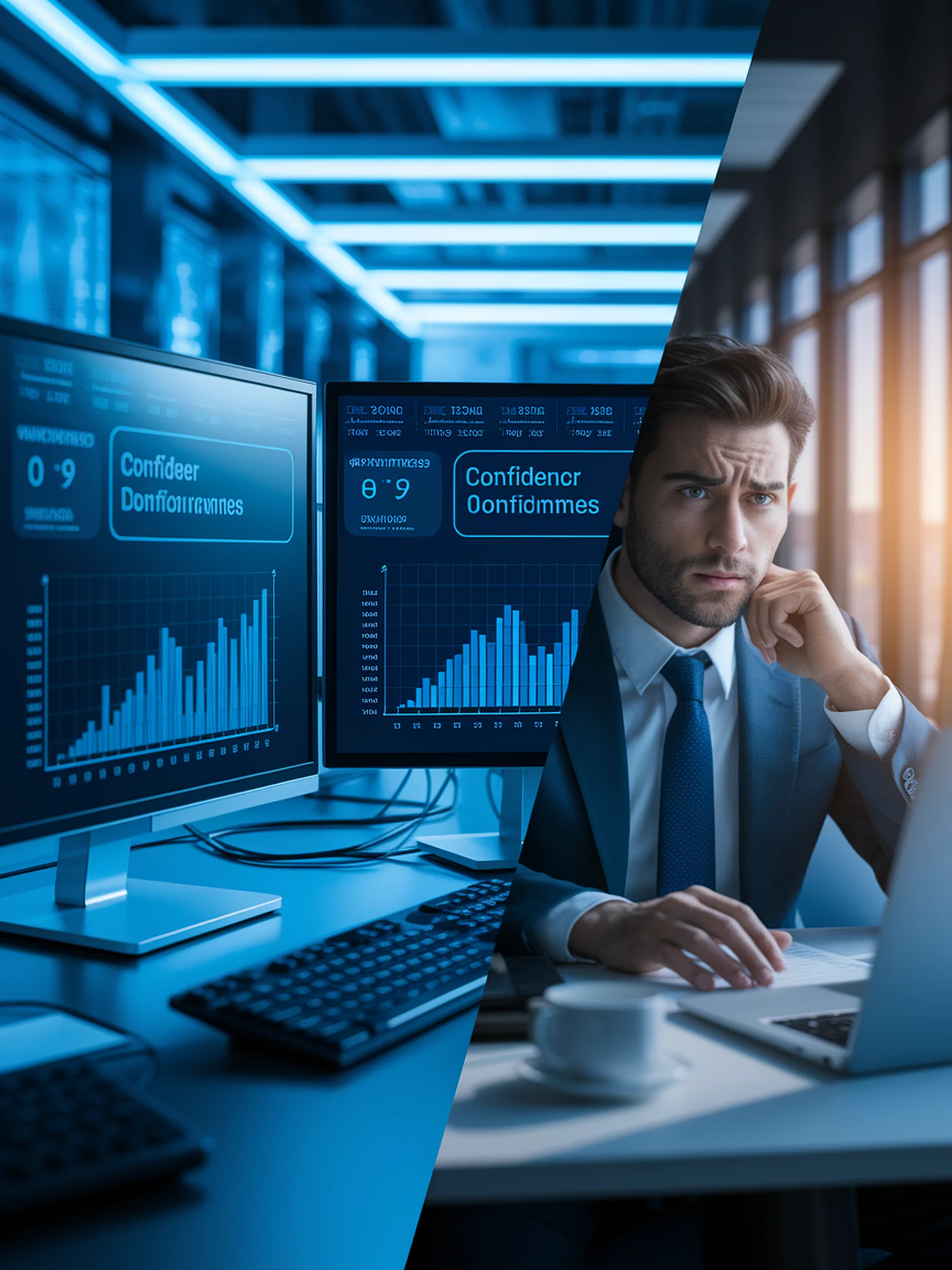
The Confidence Gap in AI Systems
Understanding the mismatch between LLM knowledge and human perception
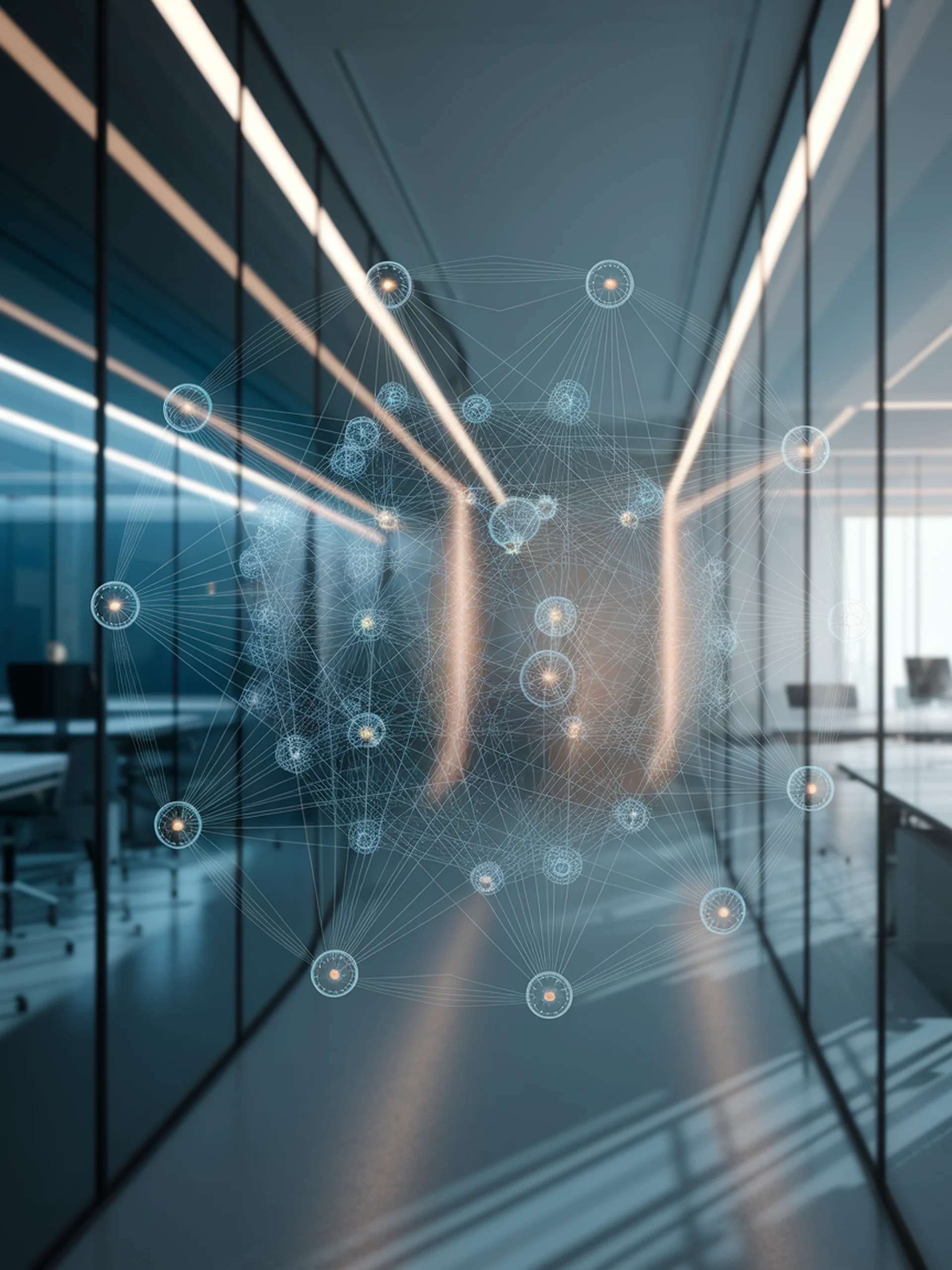
Verifiable Commonsense Reasoning in LLMs
Enhancing knowledge graph QA with transparent reasoning paths
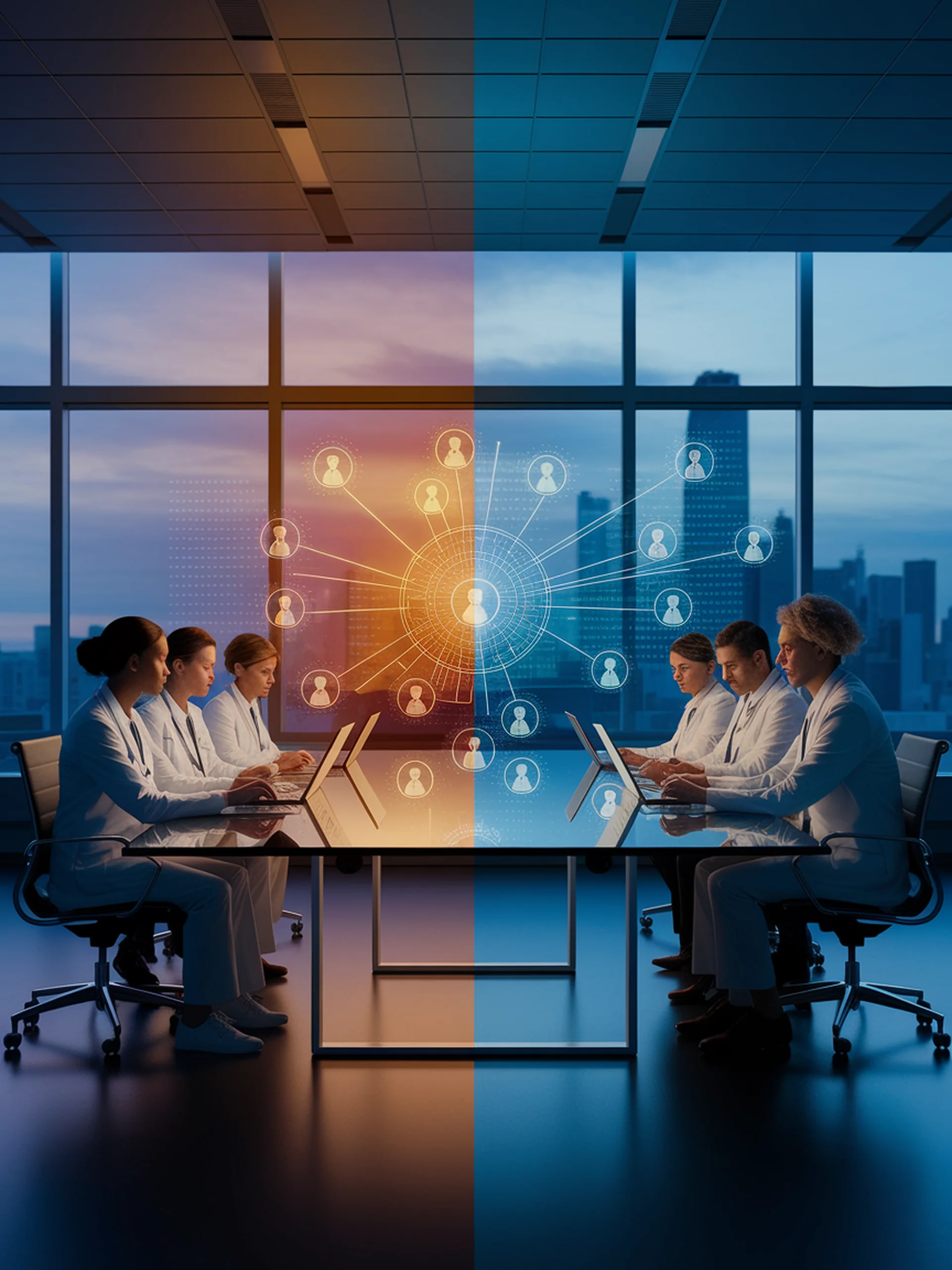
Causality-Guided Debiasing for Safer LLMs
Reducing social biases in AI decision-making for high-stakes scenarios
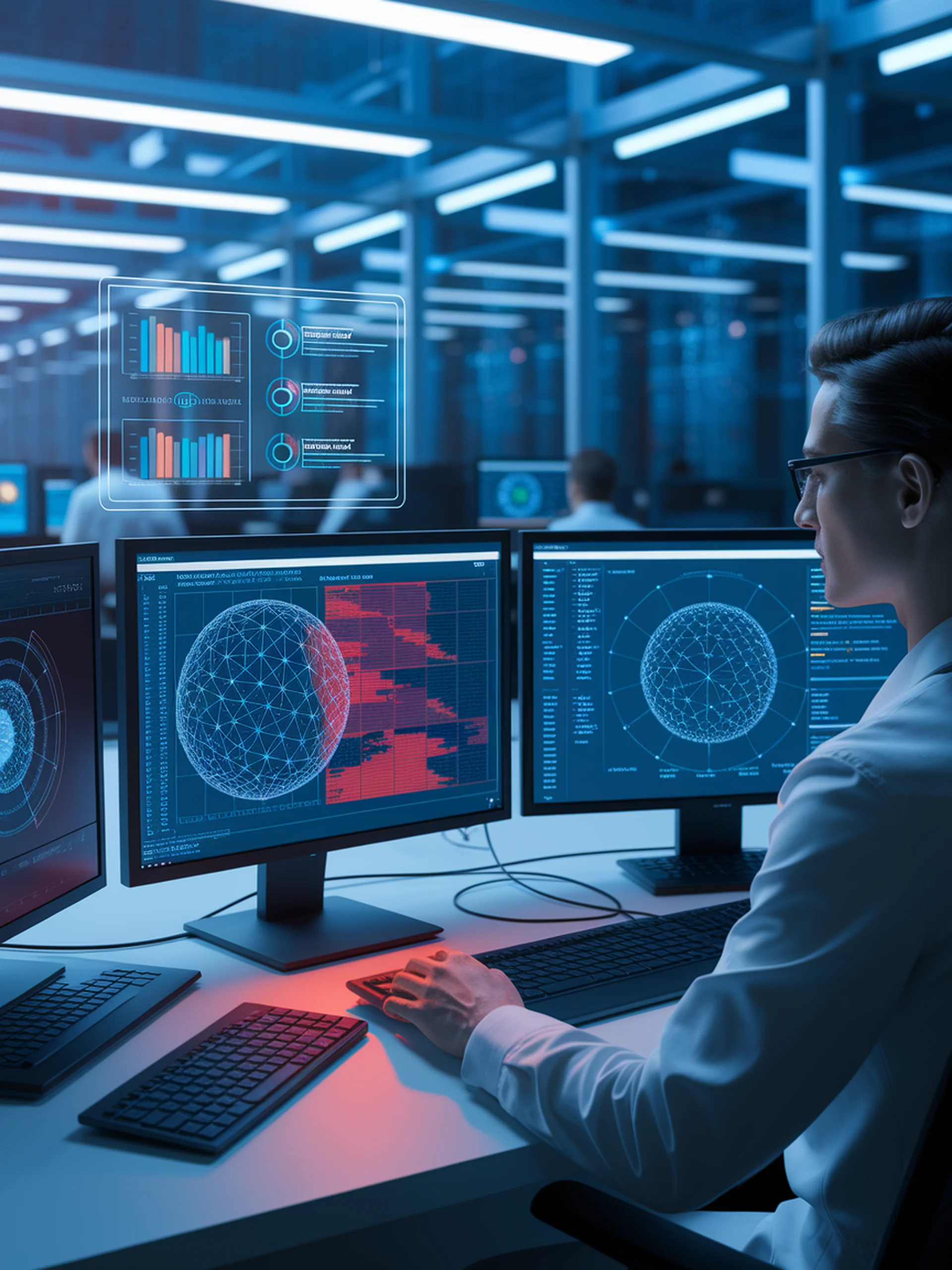
Detecting AI Hallucinations in Critical Systems
Safeguarding autonomous decision-making through robust hallucination detection
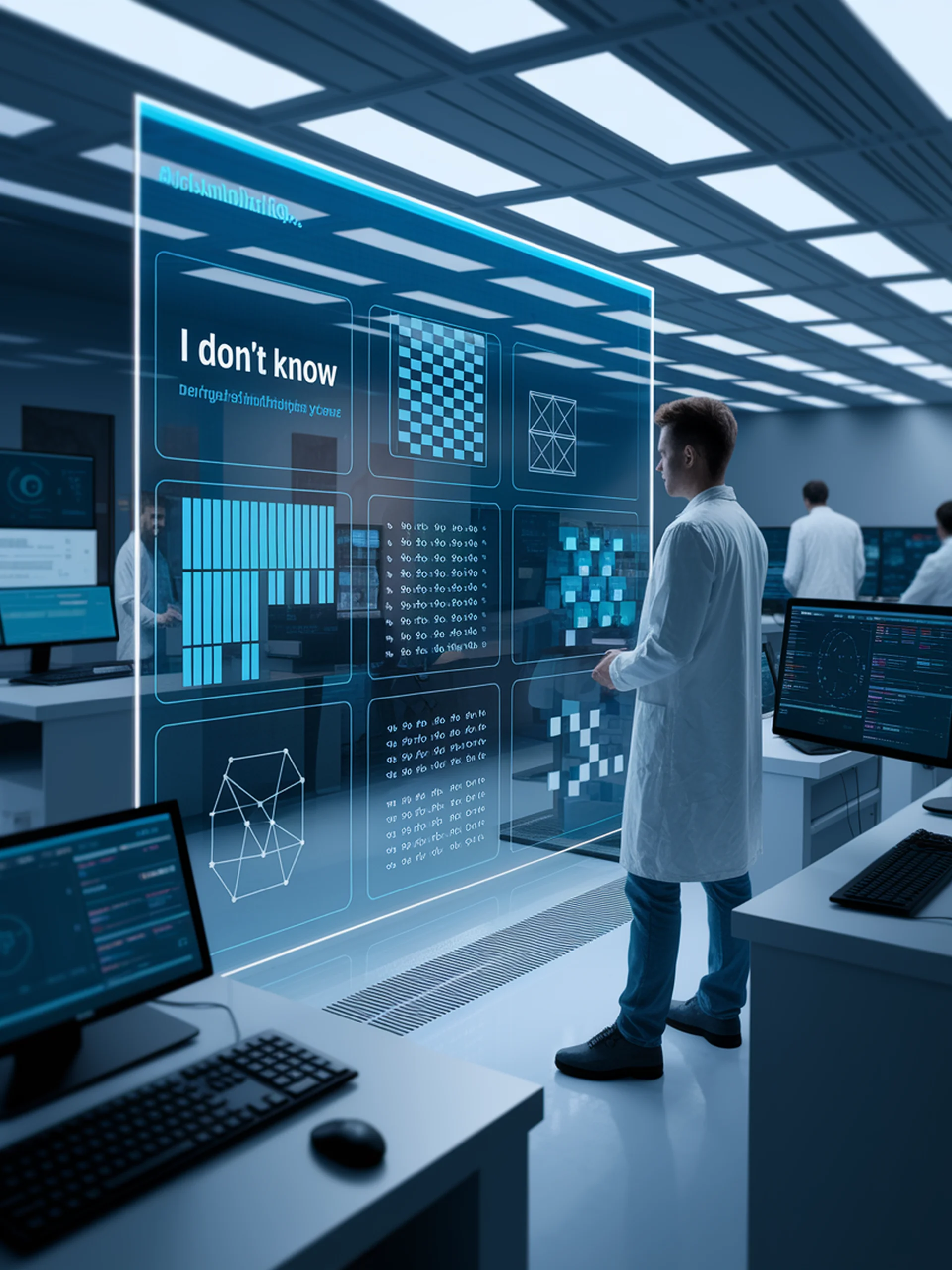
When AI Should Say 'I Don't Know'
Evaluating Multimodal AI's Understanding Through Unsolvable Problems
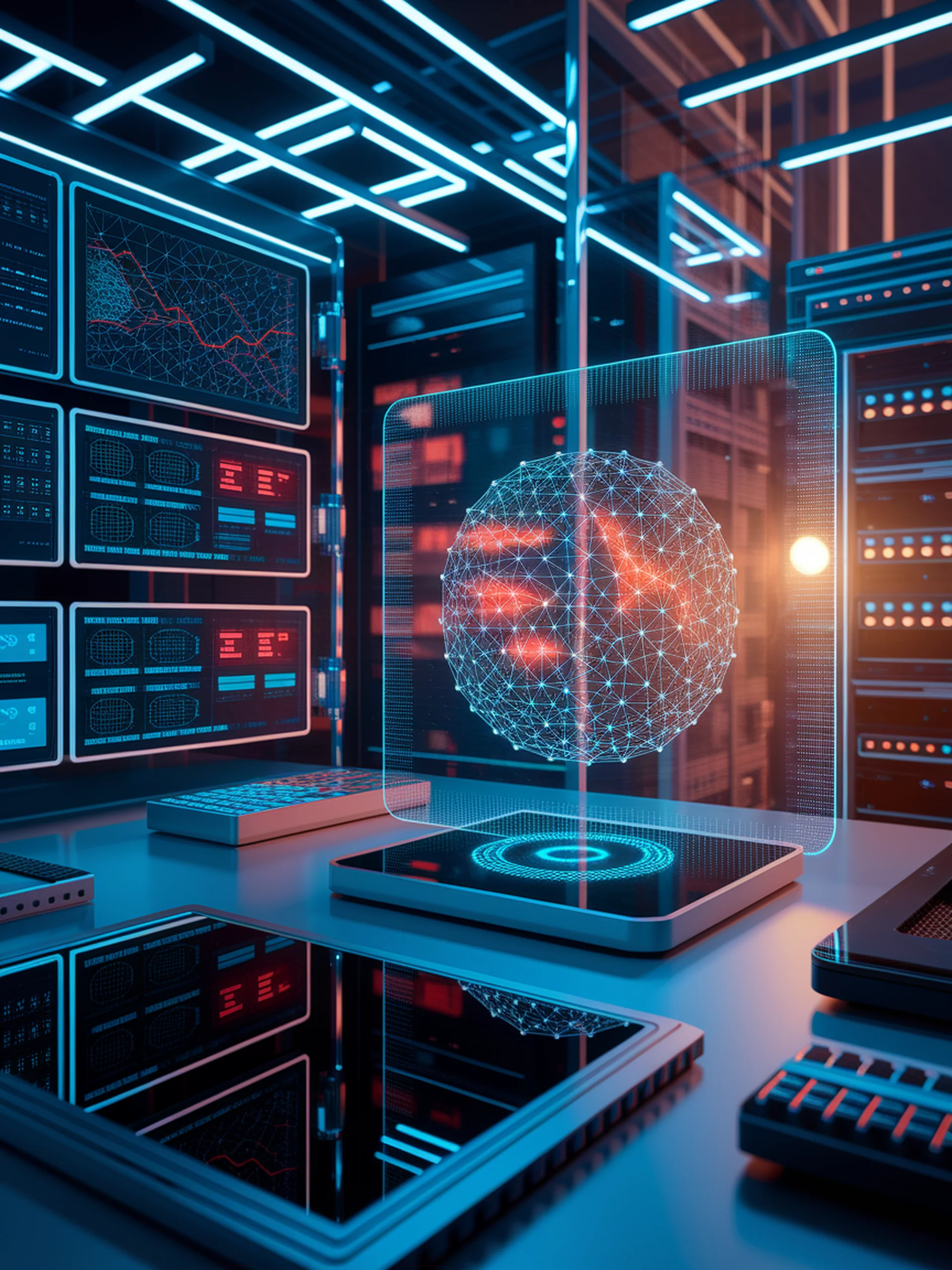
Detecting LLM Contamination
Safeguarding model integrity and evaluation reliability
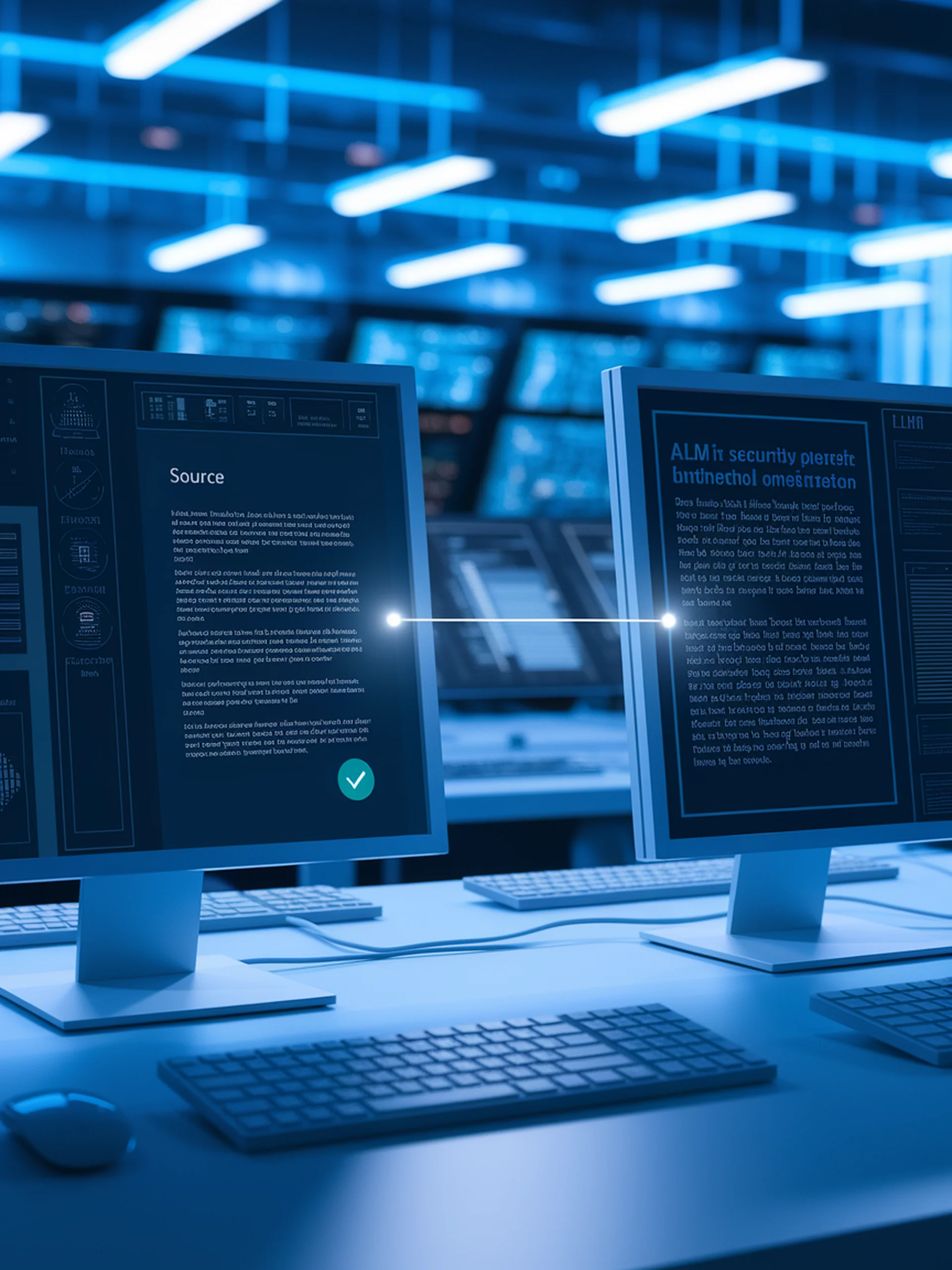
Trustworthy AI Through Quotable LLMs
Enhancing LLM verifiability by design rather than afterthought
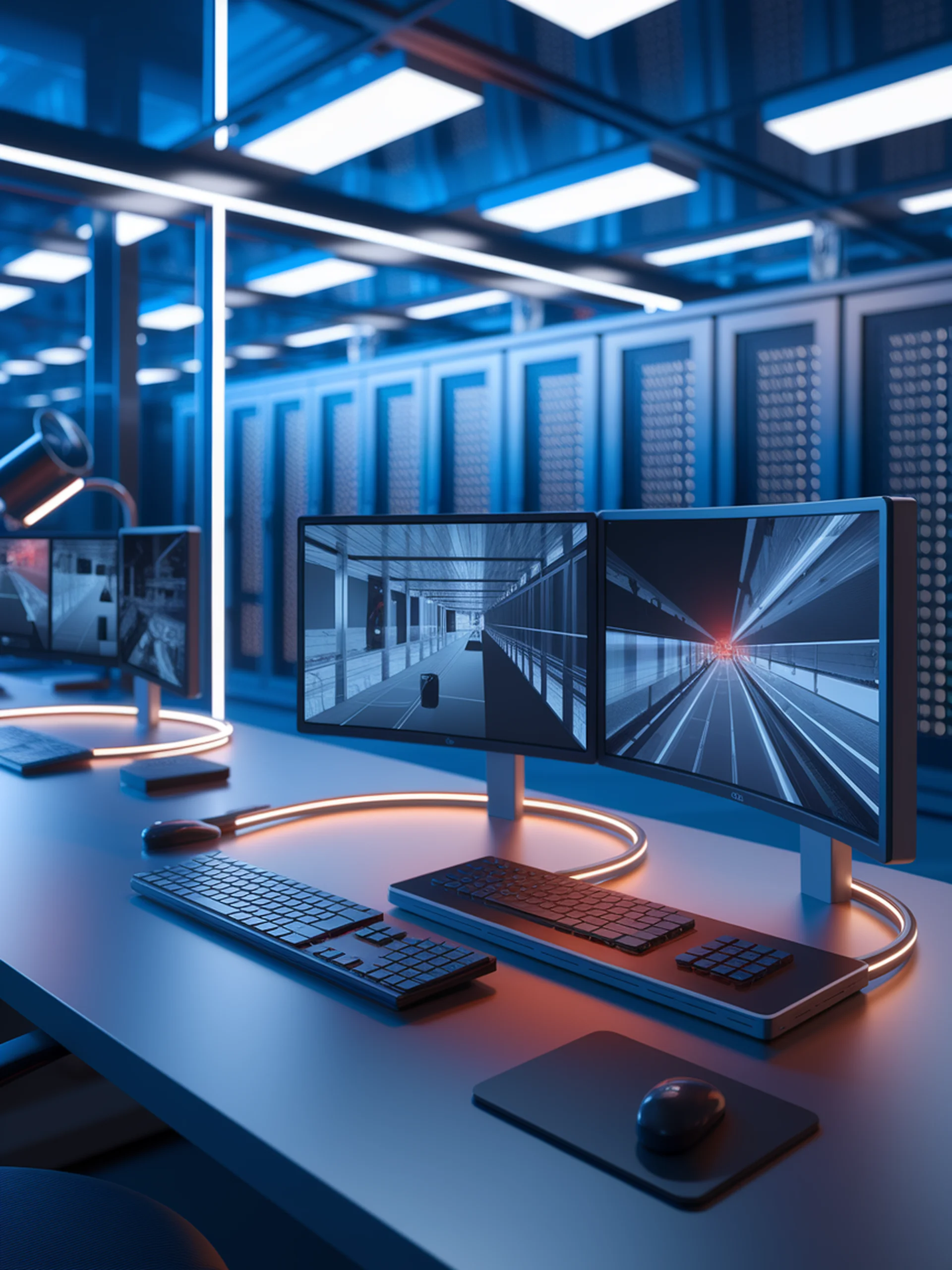
Smarter Anomaly Detection with LLMs
Advanced visual anomaly detection using adaptive local feature analysis
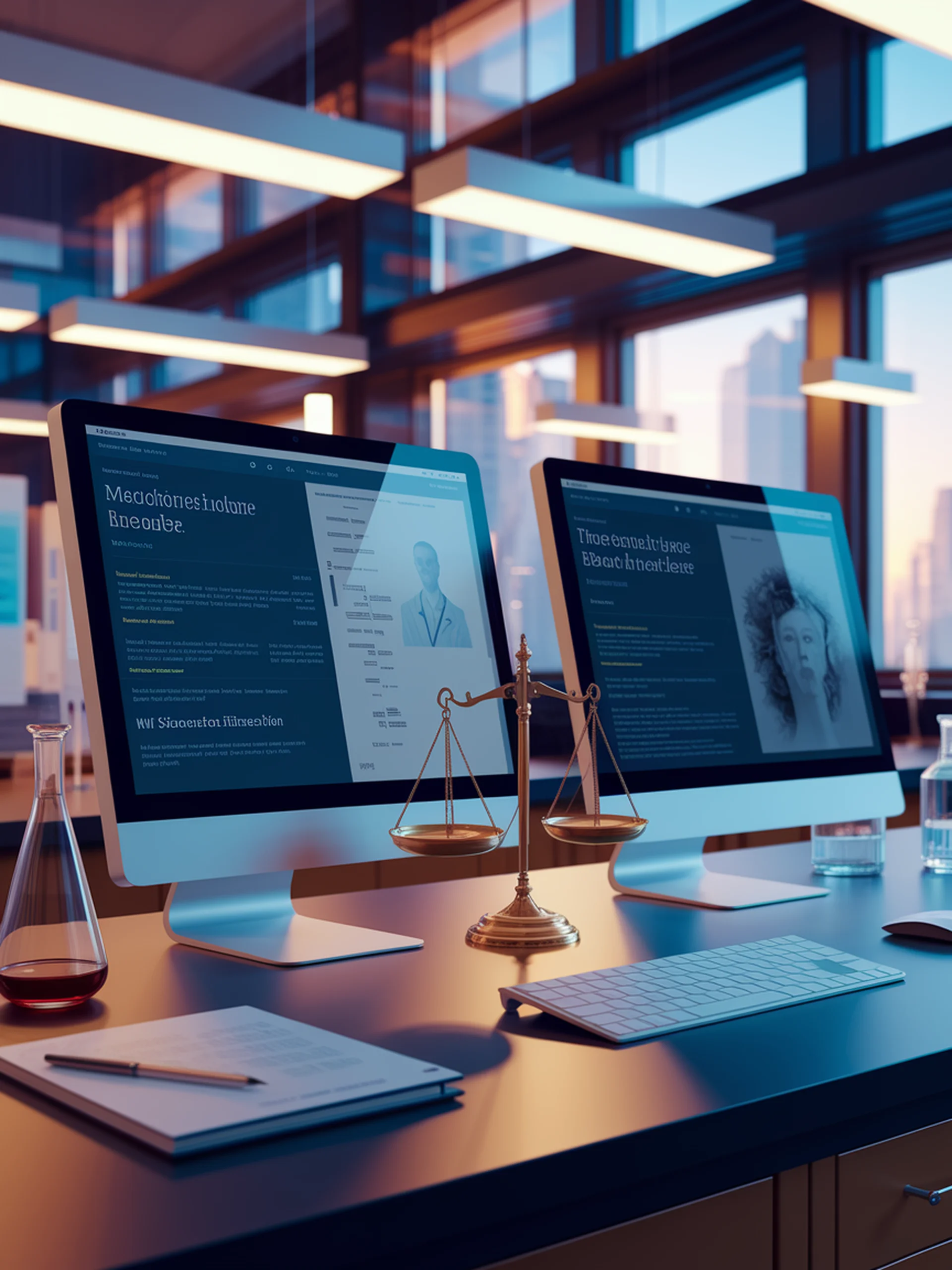
When LLMs Clash With Evidence
Measuring how language models balance prior knowledge against retrieved information
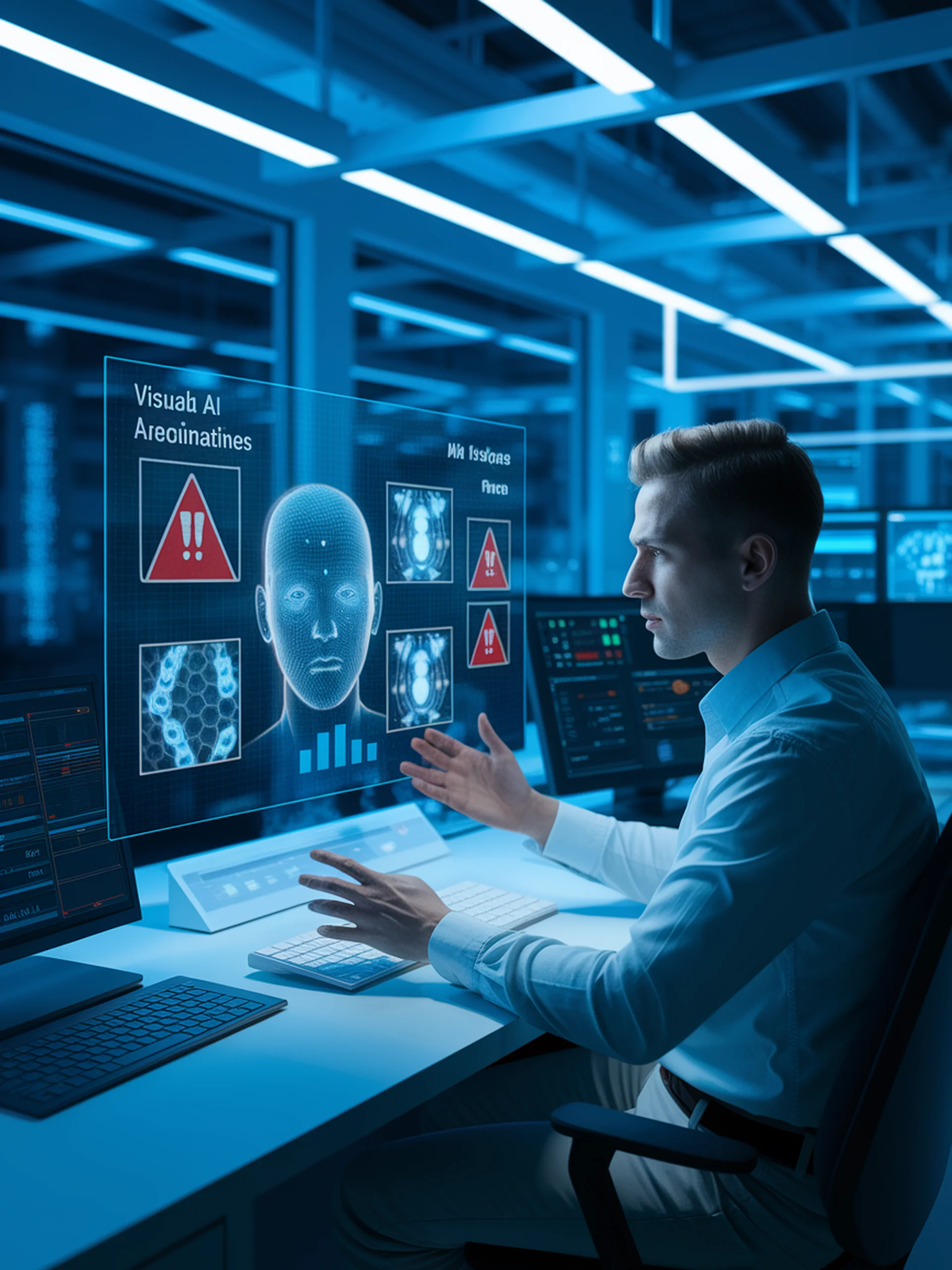
Combating Hallucinations in Multimodal AI
Understanding and addressing reliability challenges in vision-language models
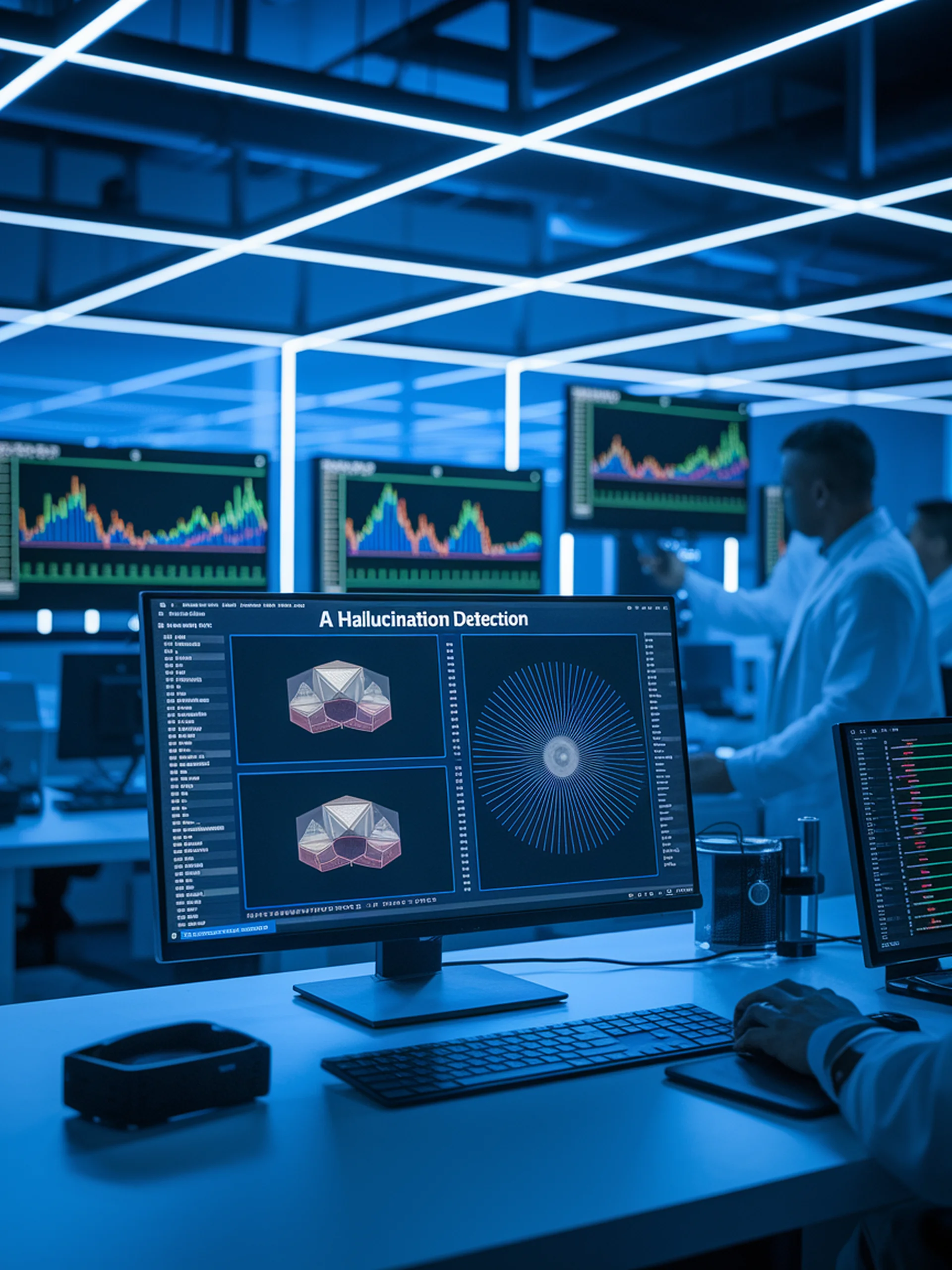
Combating Visual Hallucinations in AI
A benchmark for detecting free-form hallucinations in vision-language models
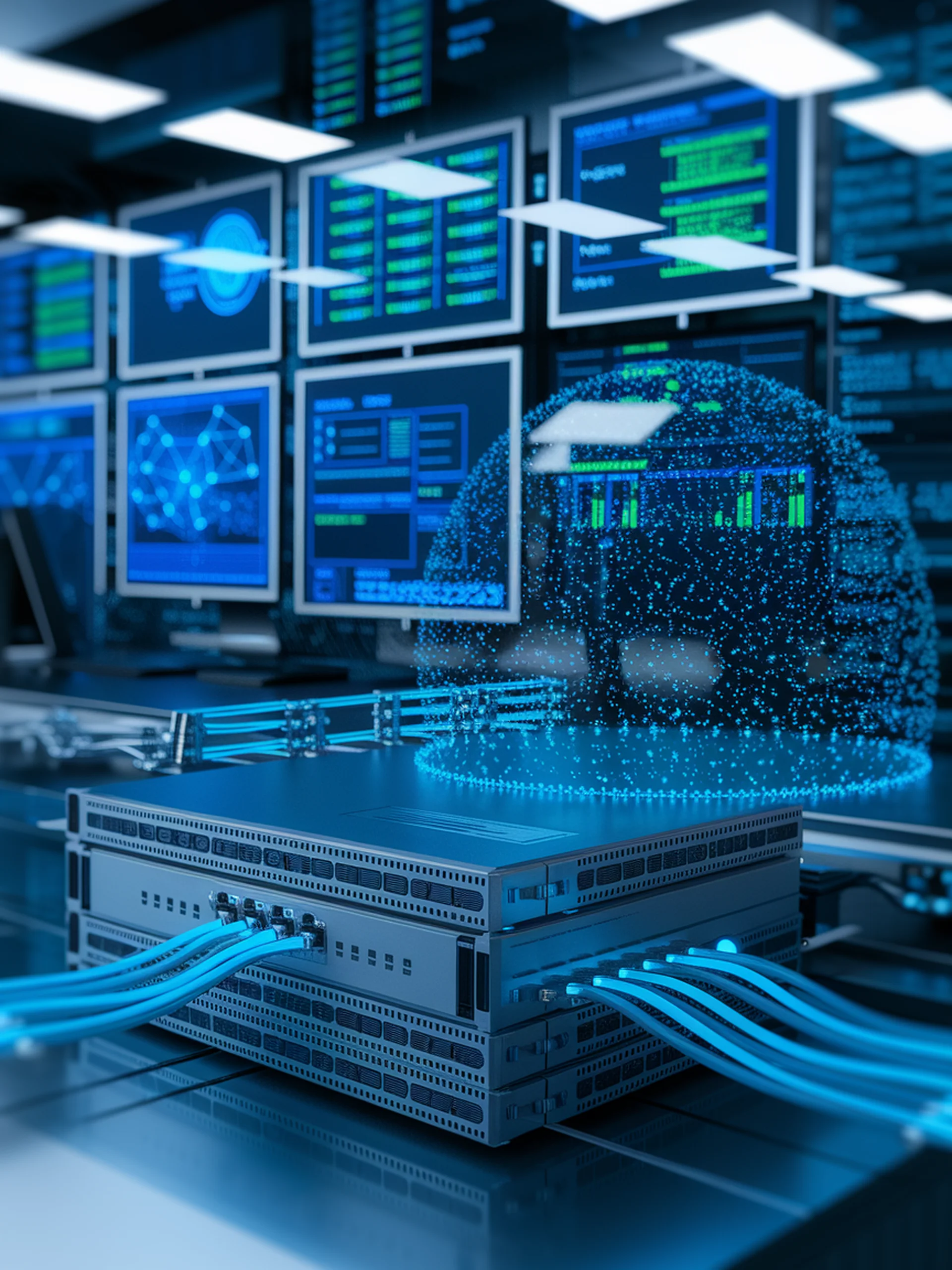
Building Trust in AI-Generated Network Content
A Framework for Robust, Secure, and Fair AIGC Services
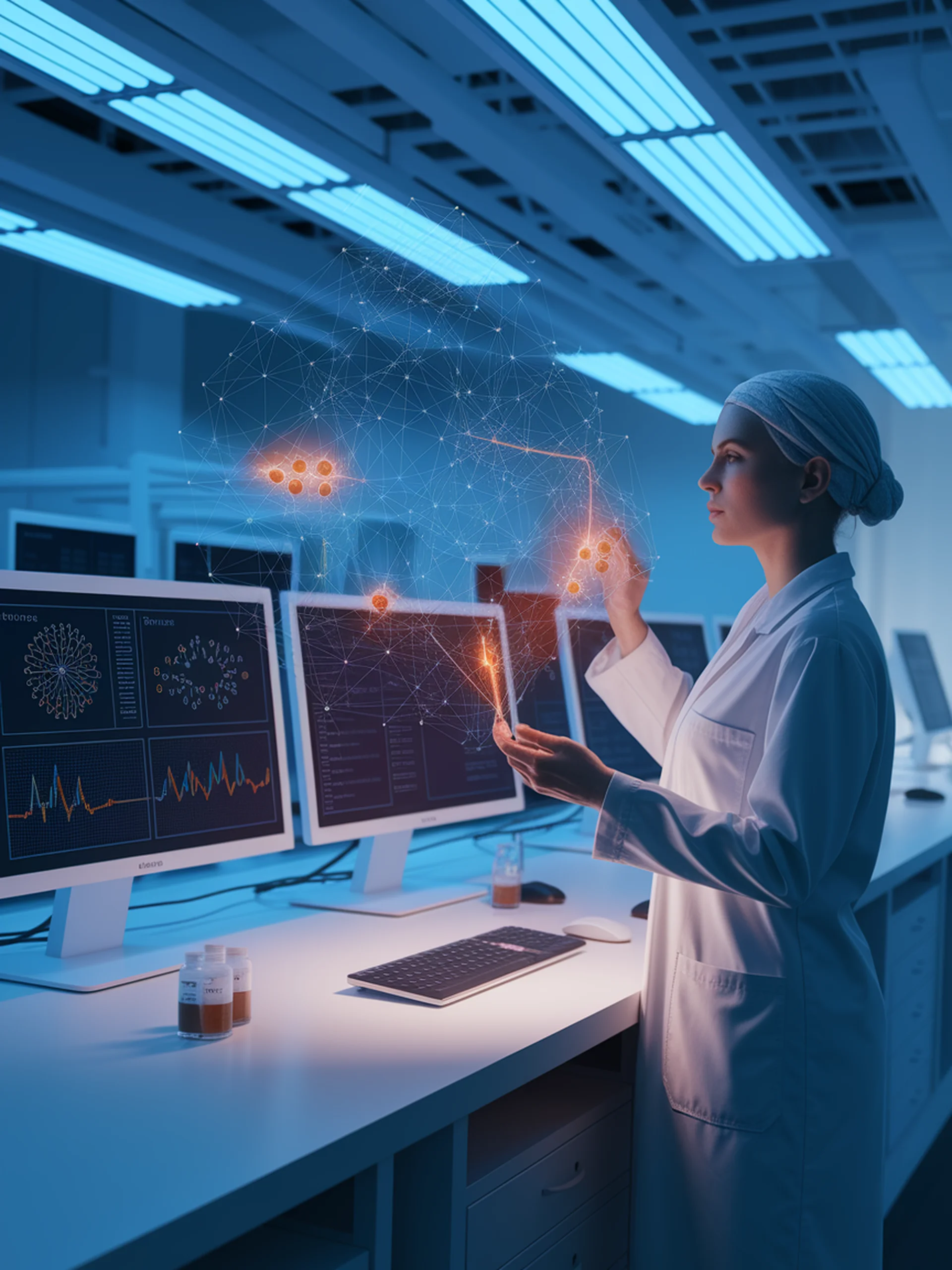
Fighting LLM Hallucinations
A technique to enhance truthfulness without retraining
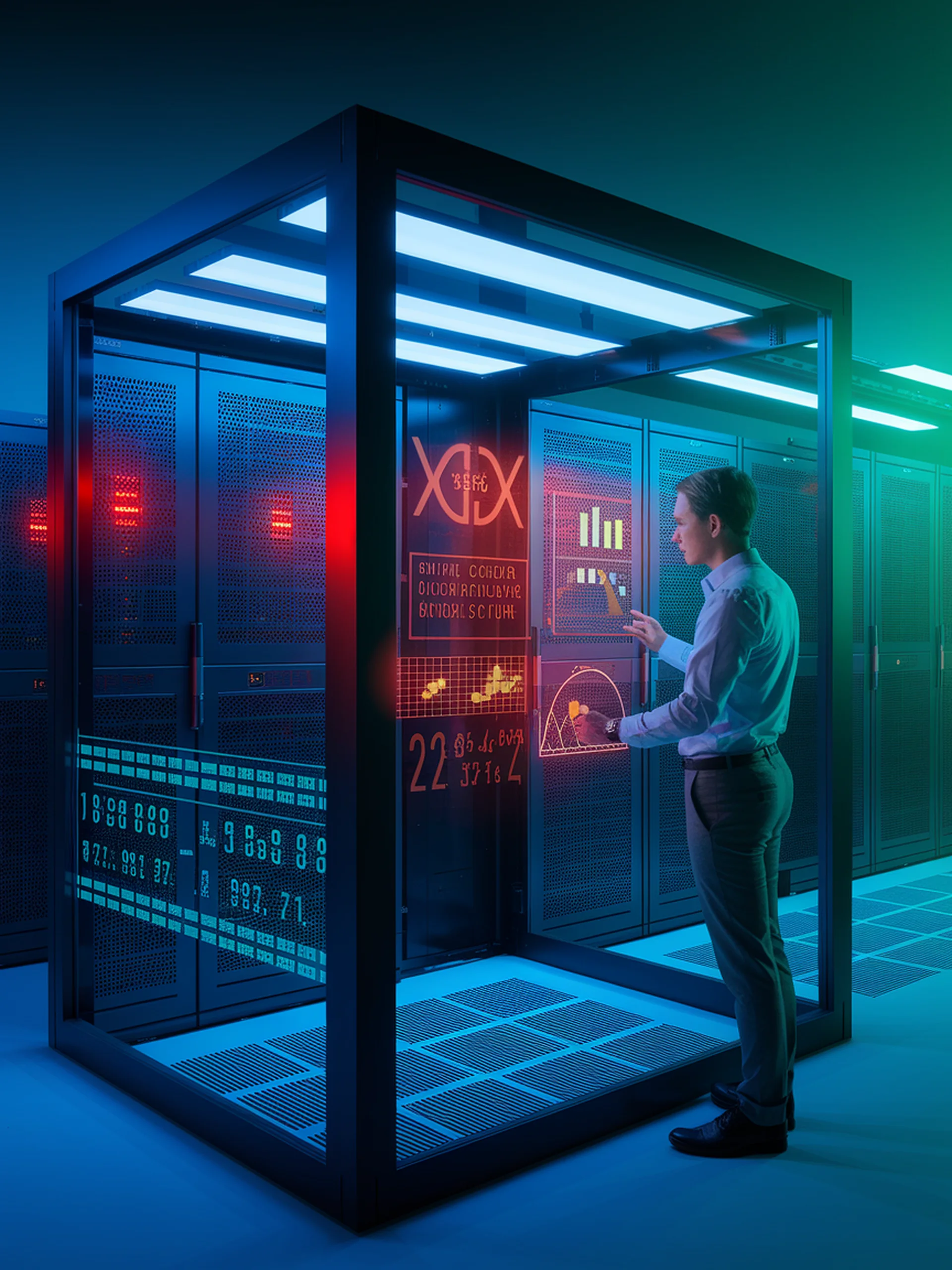
Building Trust in Black-Box LLMs
A Framework for Confidence Estimation Without Model Access
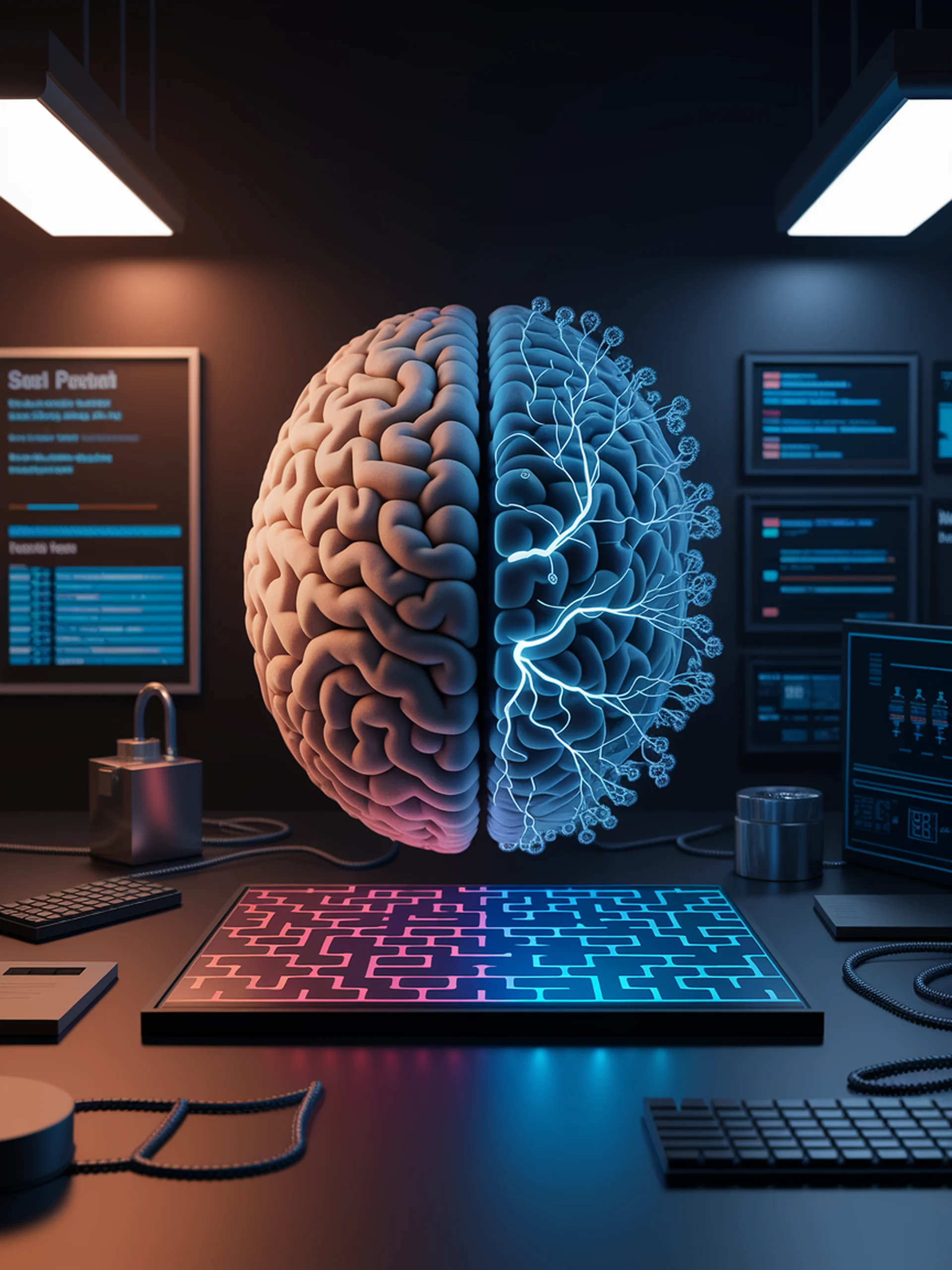
Mind the Gap: AI vs. Human Logical Reasoning
Evaluating multi-modal LLMs against human vision capabilities
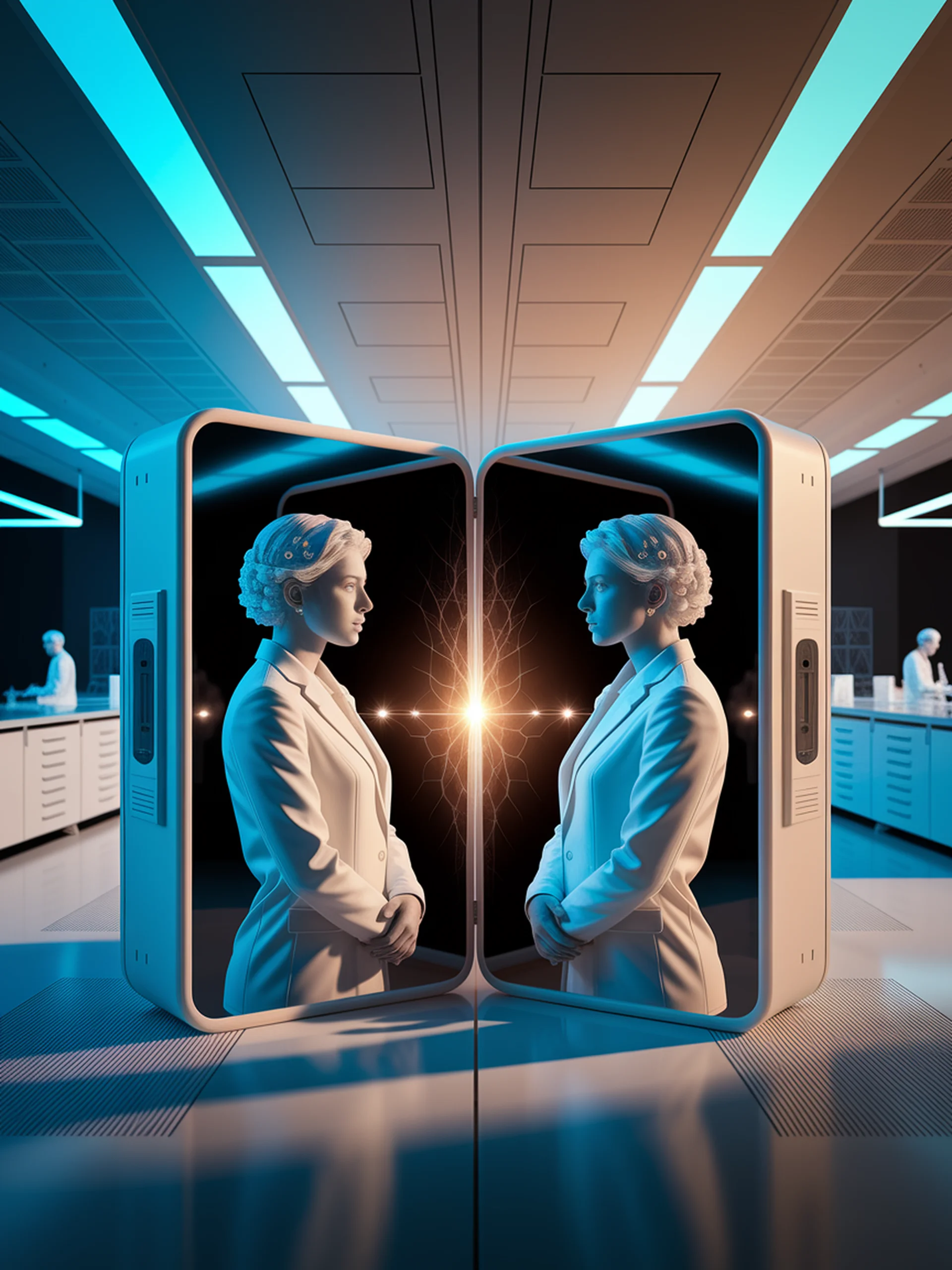
Making AI Safer Through Self-Reflection
How LLMs can critique and correct their own outputs
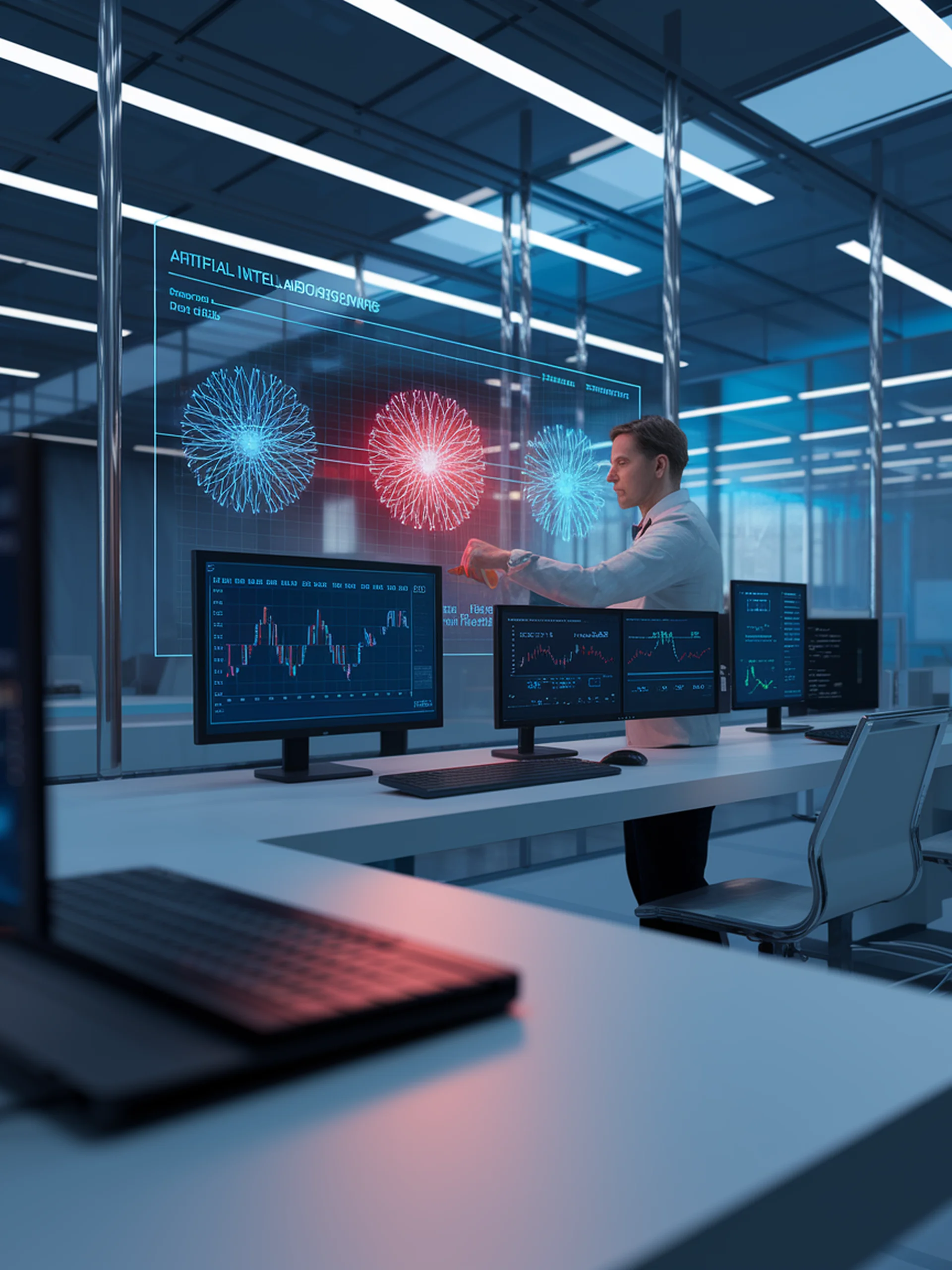
LARS: Learning to Estimate LLM Uncertainty
A trainable approach replacing hand-crafted uncertainty scoring functions
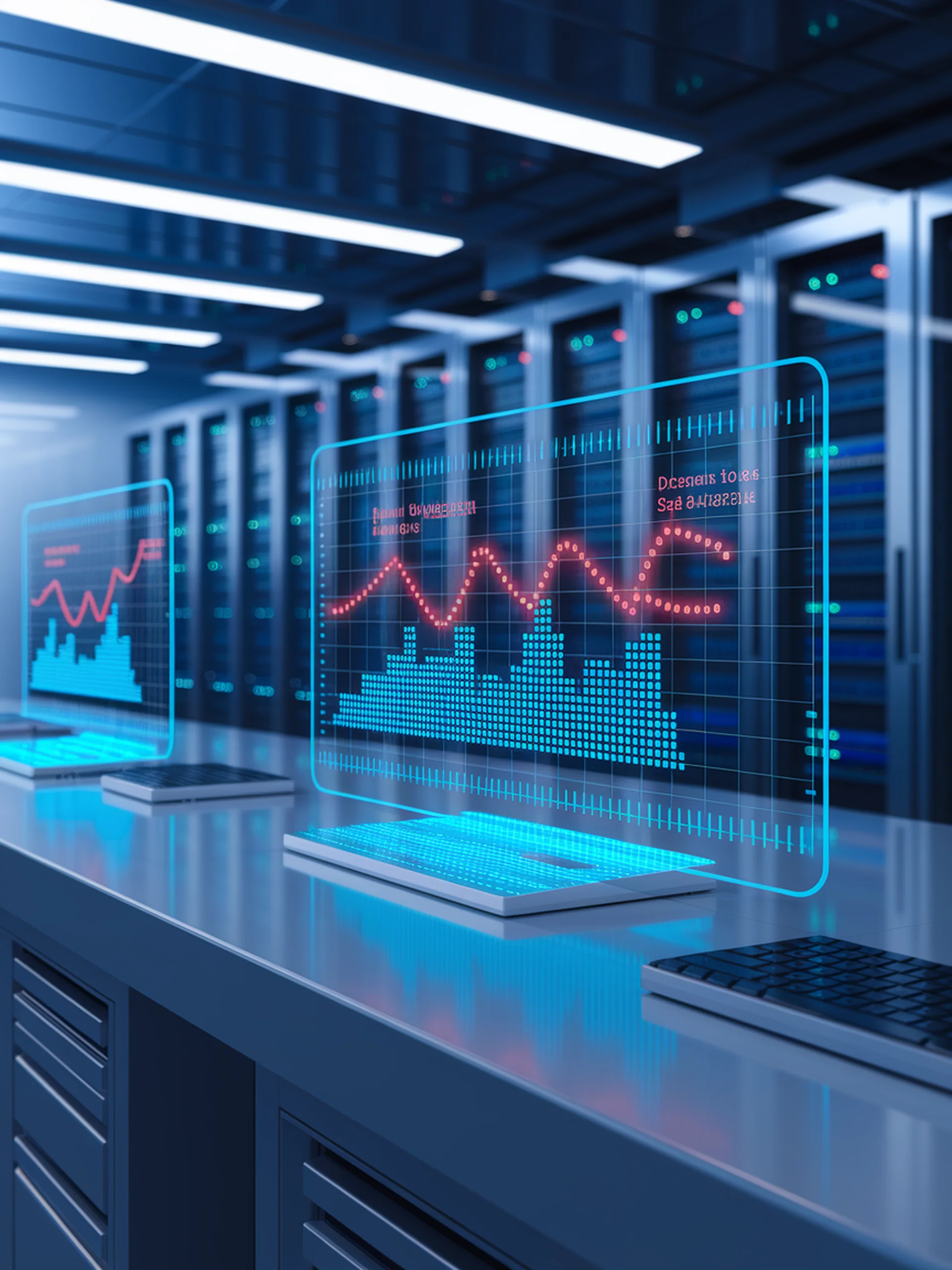
Detecting Benchmark Contamination in LLMs
A new statistical approach to ensure fair model evaluation
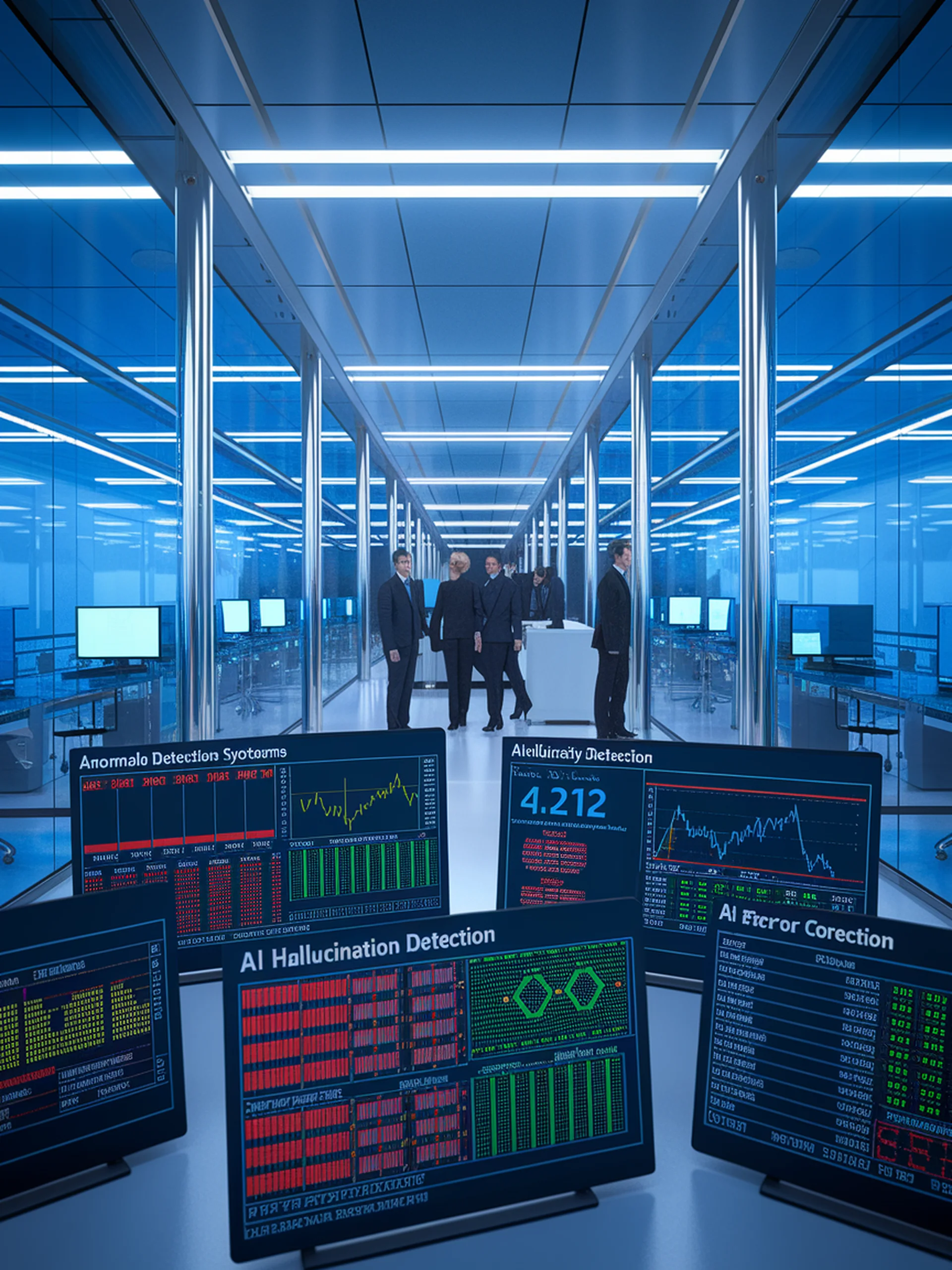
Combating LLM Hallucinations at Scale
A Production-Ready System for Detection and Mitigation
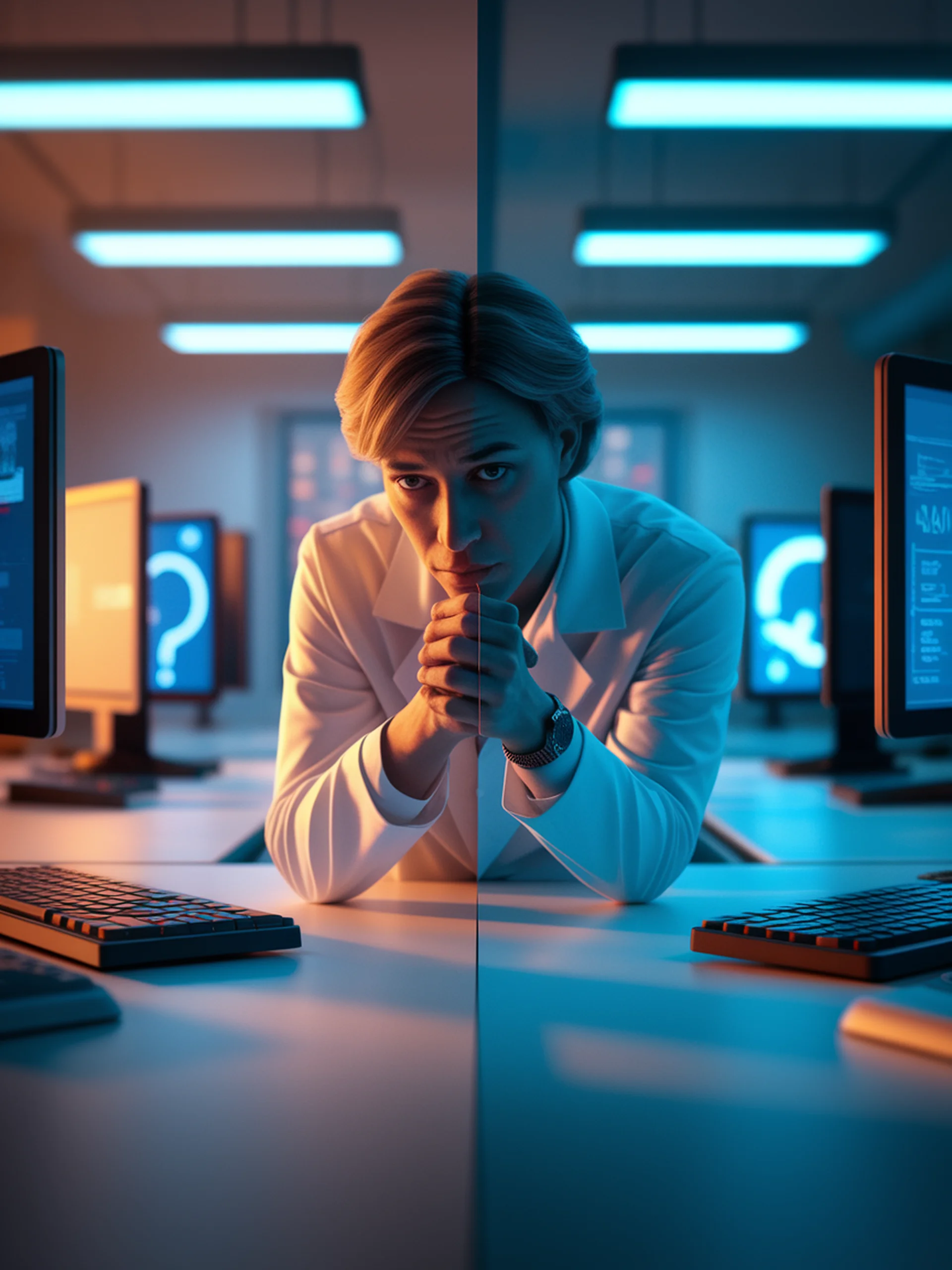
Know Your Limits: A Survey of Abstention in Large Language M...
By Bingbing Wen, Jihan Yao...
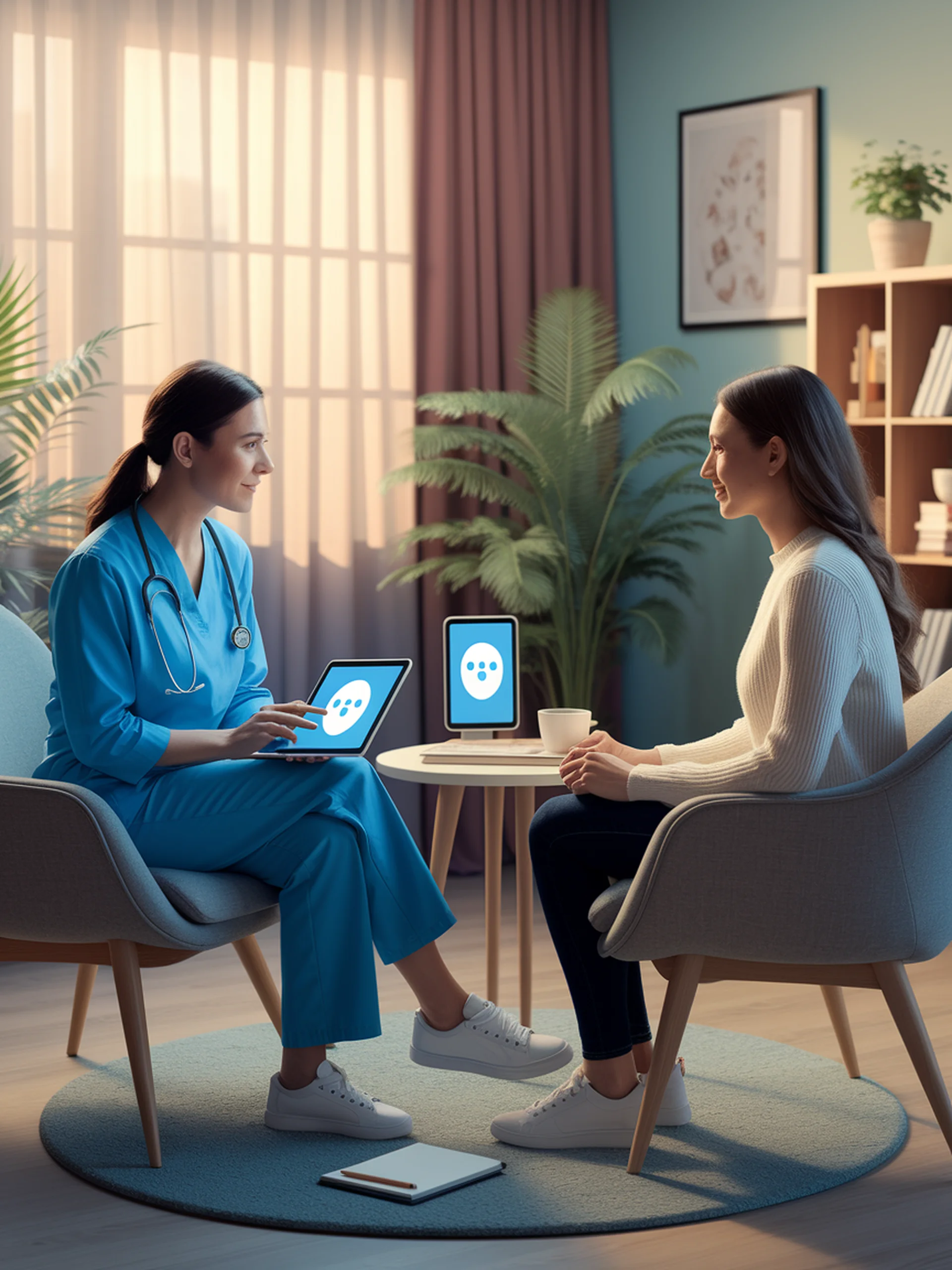
Safety-First Mental Health AI
A Framework for Building Trust in Mental Health Chatbots
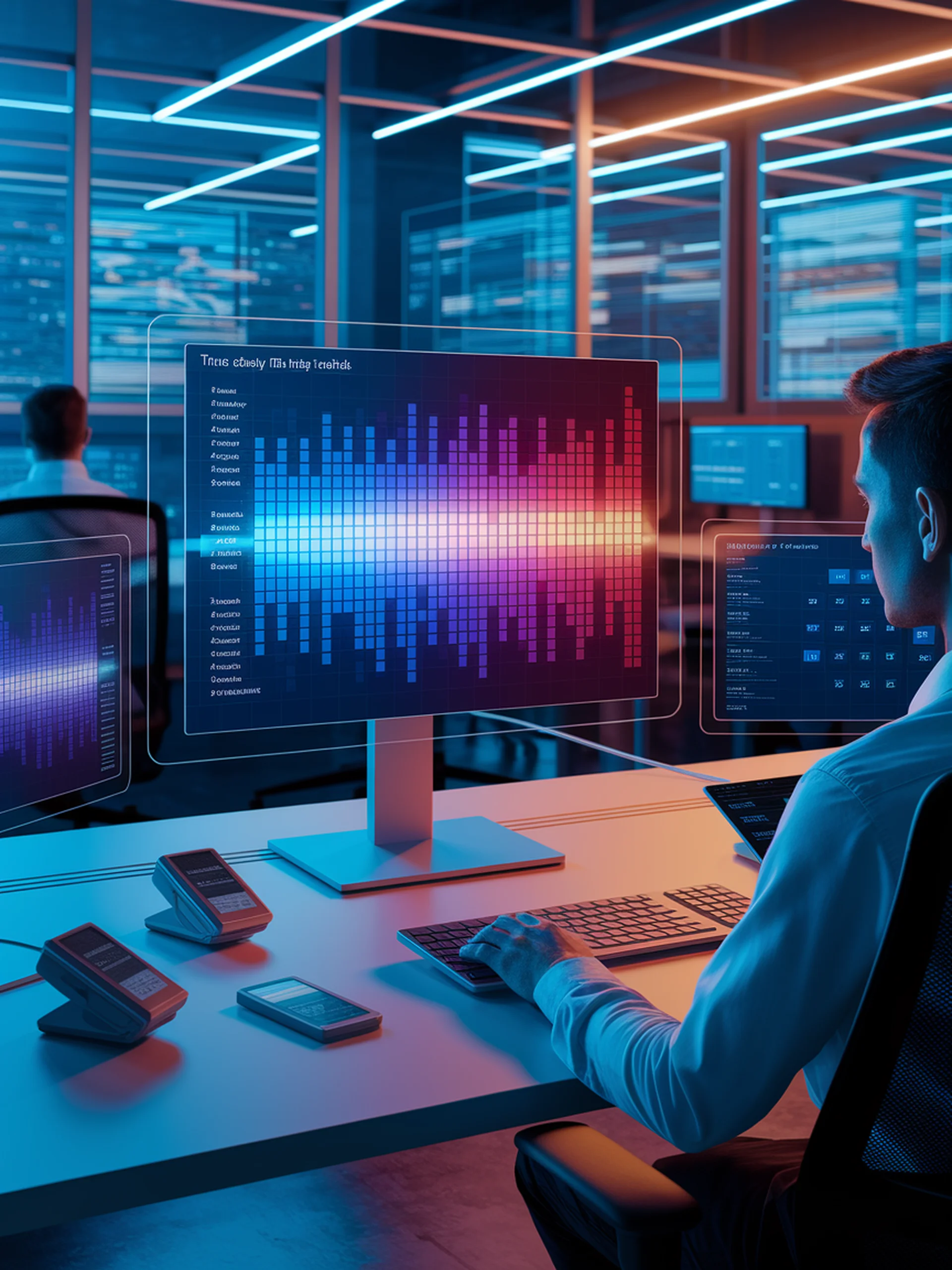
Adaptive Guardrails for LLMs
Trust-based security frameworks for diverse user needs
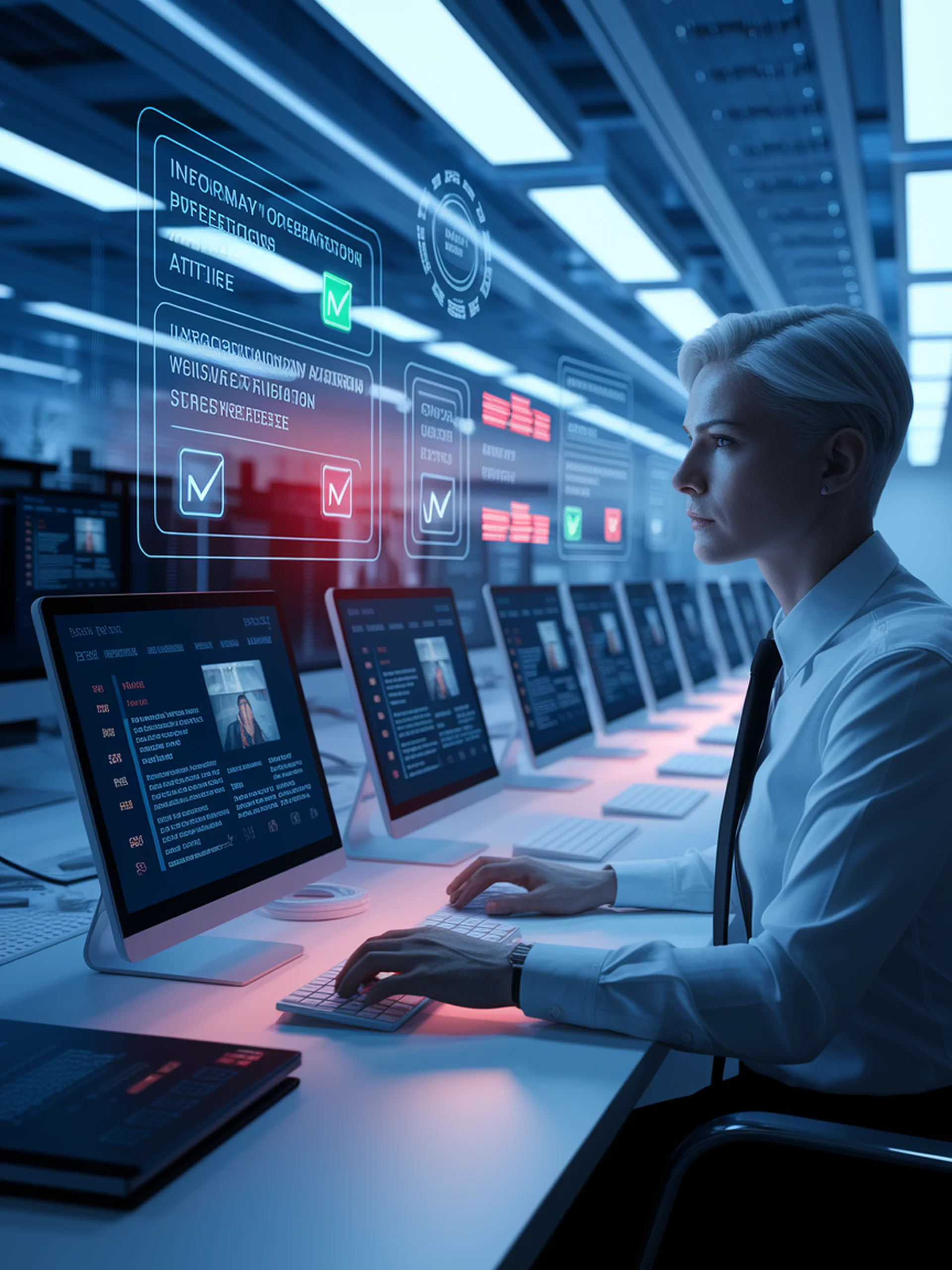
Battling Misinformation with AI
How Large Language Models Are Transforming Claim Verification
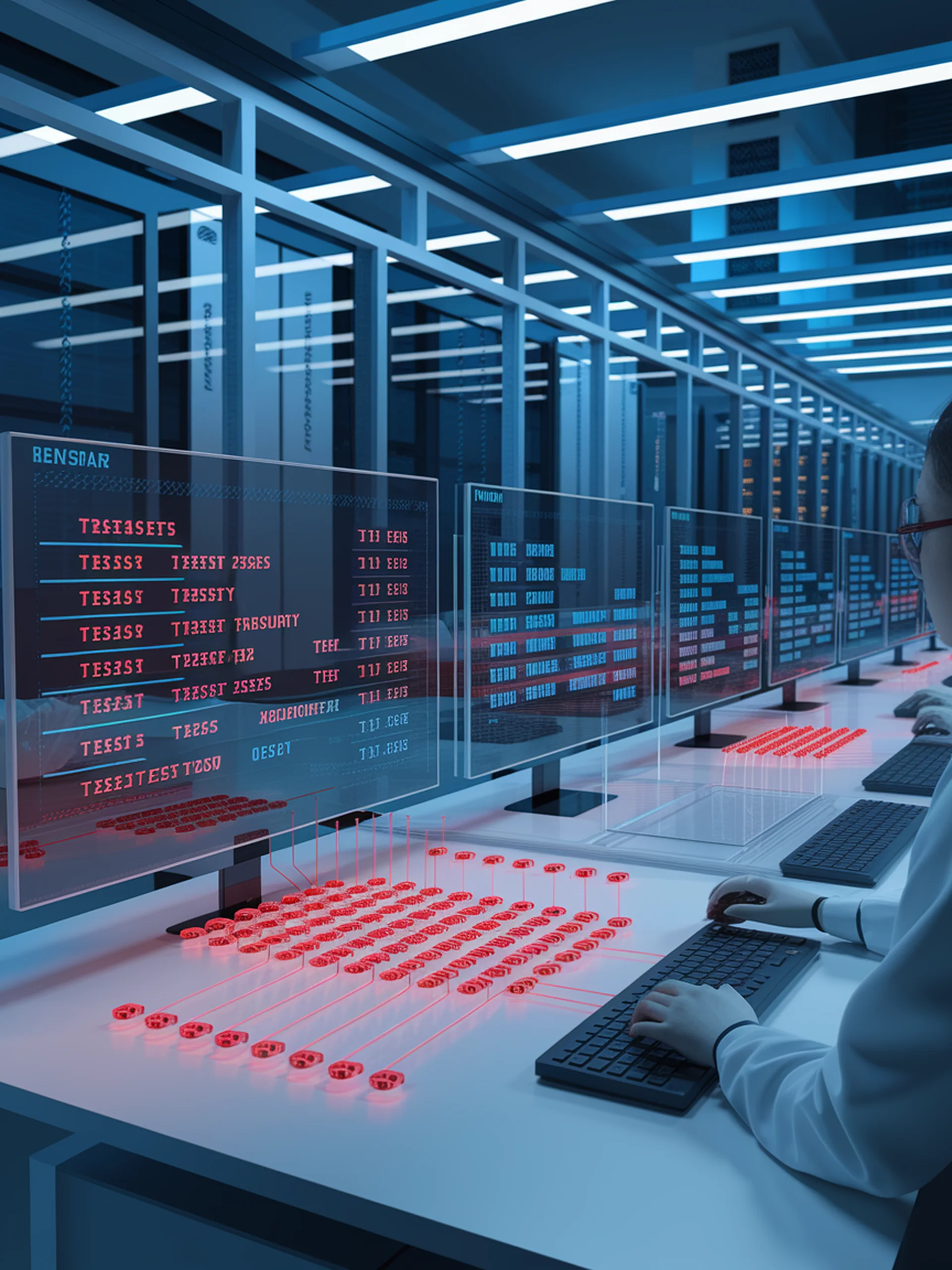
Detecting Benchmark Contamination in LLMs
Protecting evaluation integrity through data leakage detection
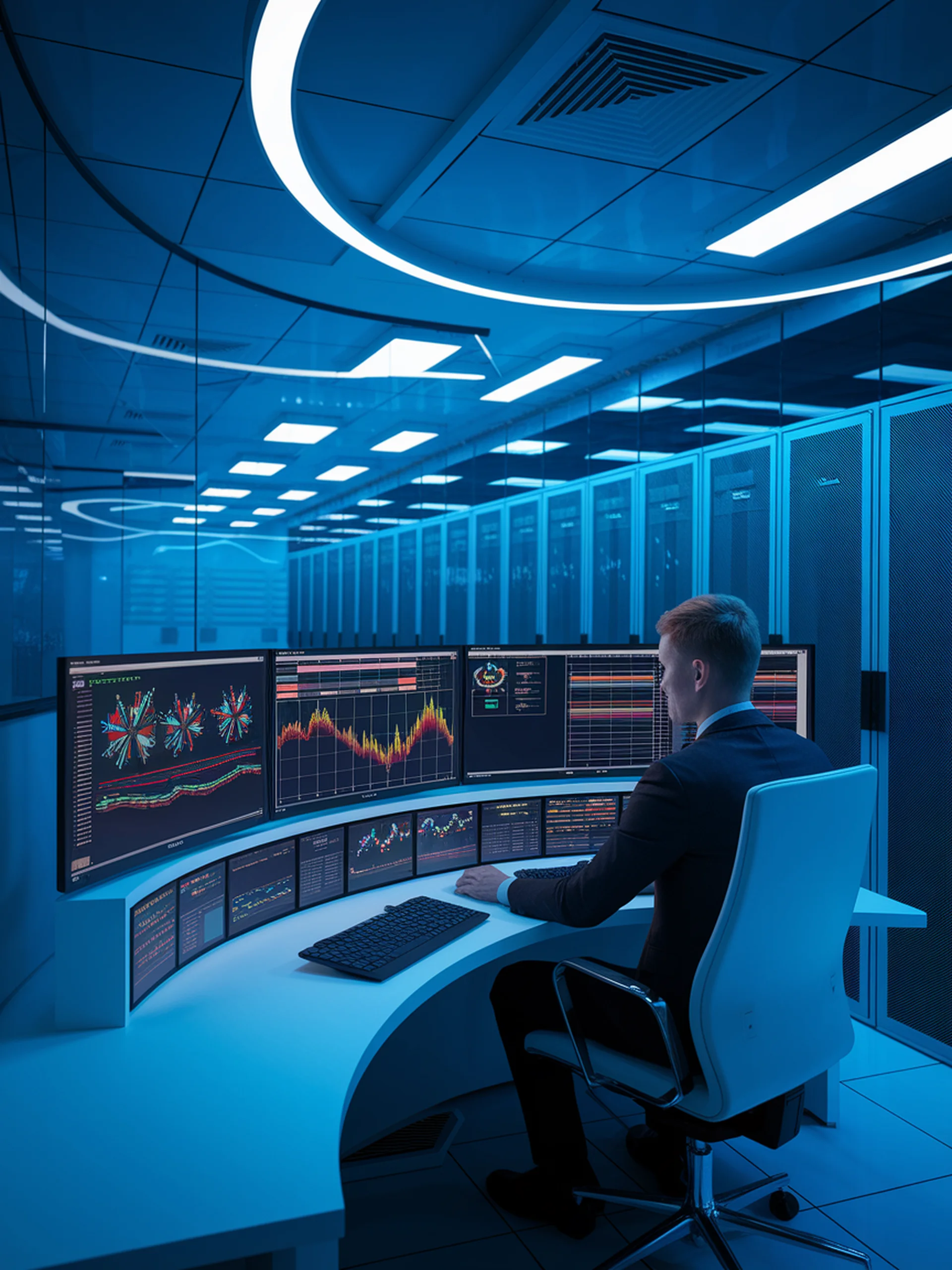
Making Generative AI Safe and Reliable
New statistical guardrails for critical applications
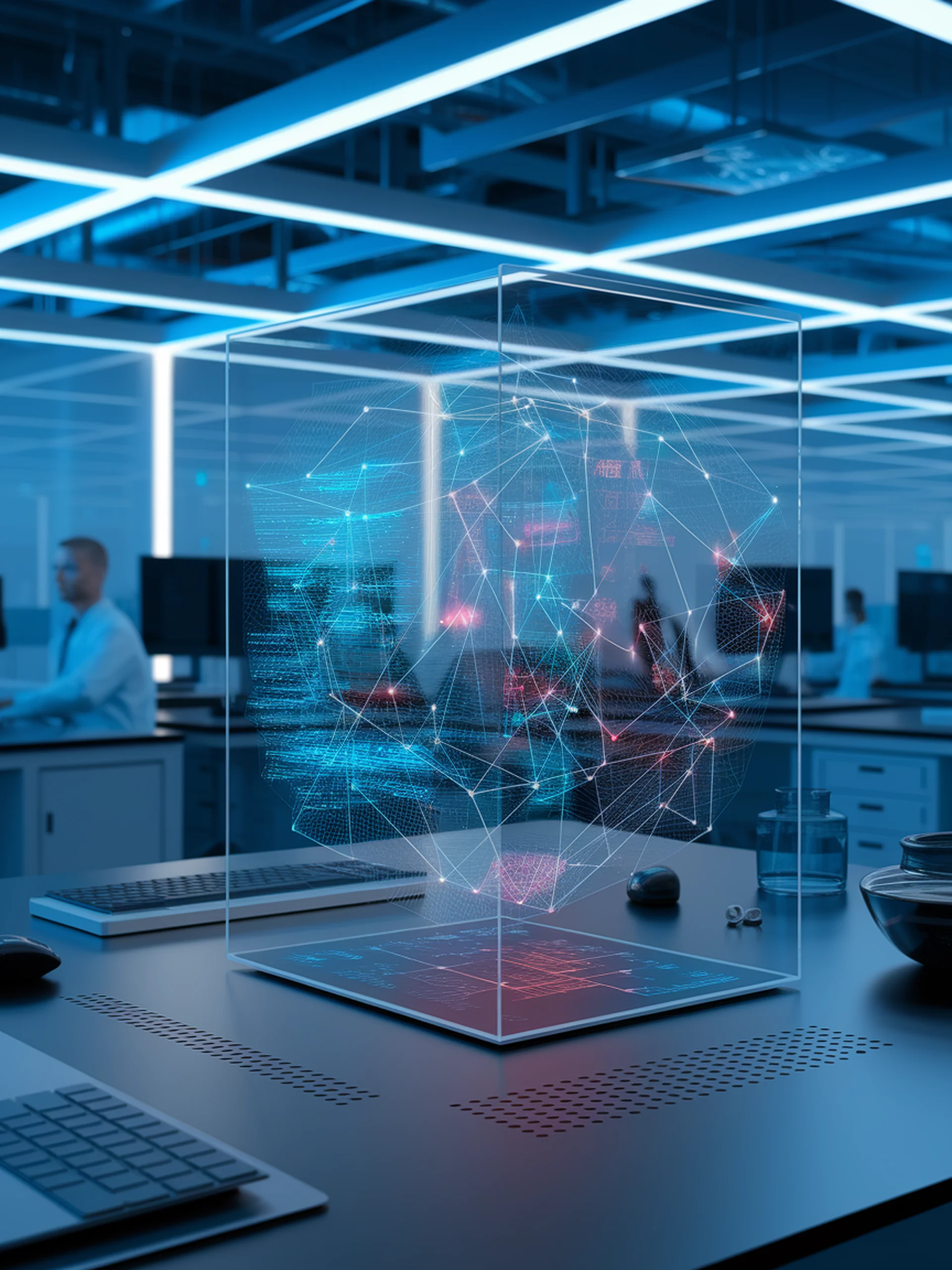
Precision Knowledge Editing for LLMs
Updating AI knowledge without disrupting existing capabilities
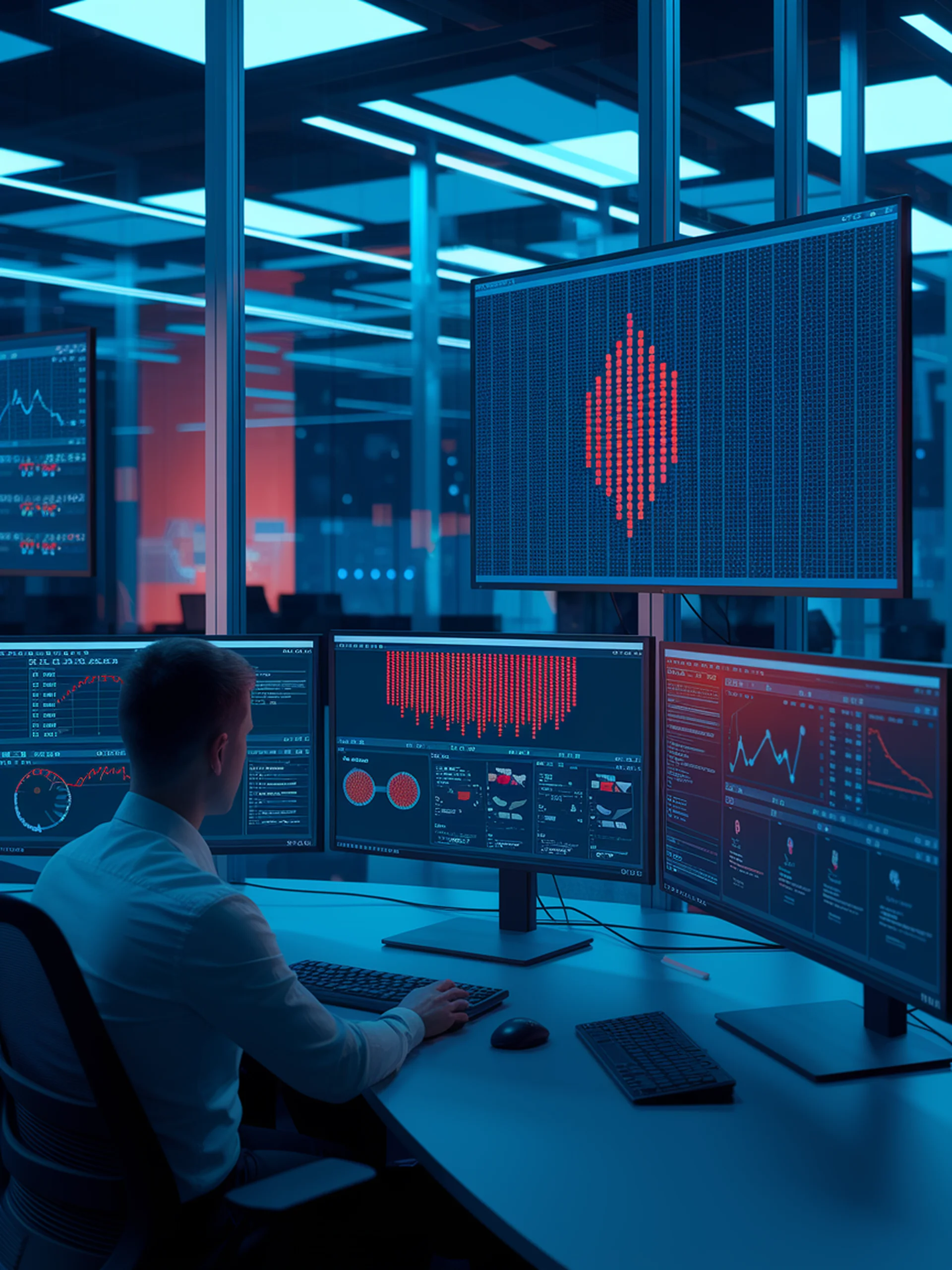
Smart OOD Detection Selection
Automating the choice of optimal distribution shift detectors
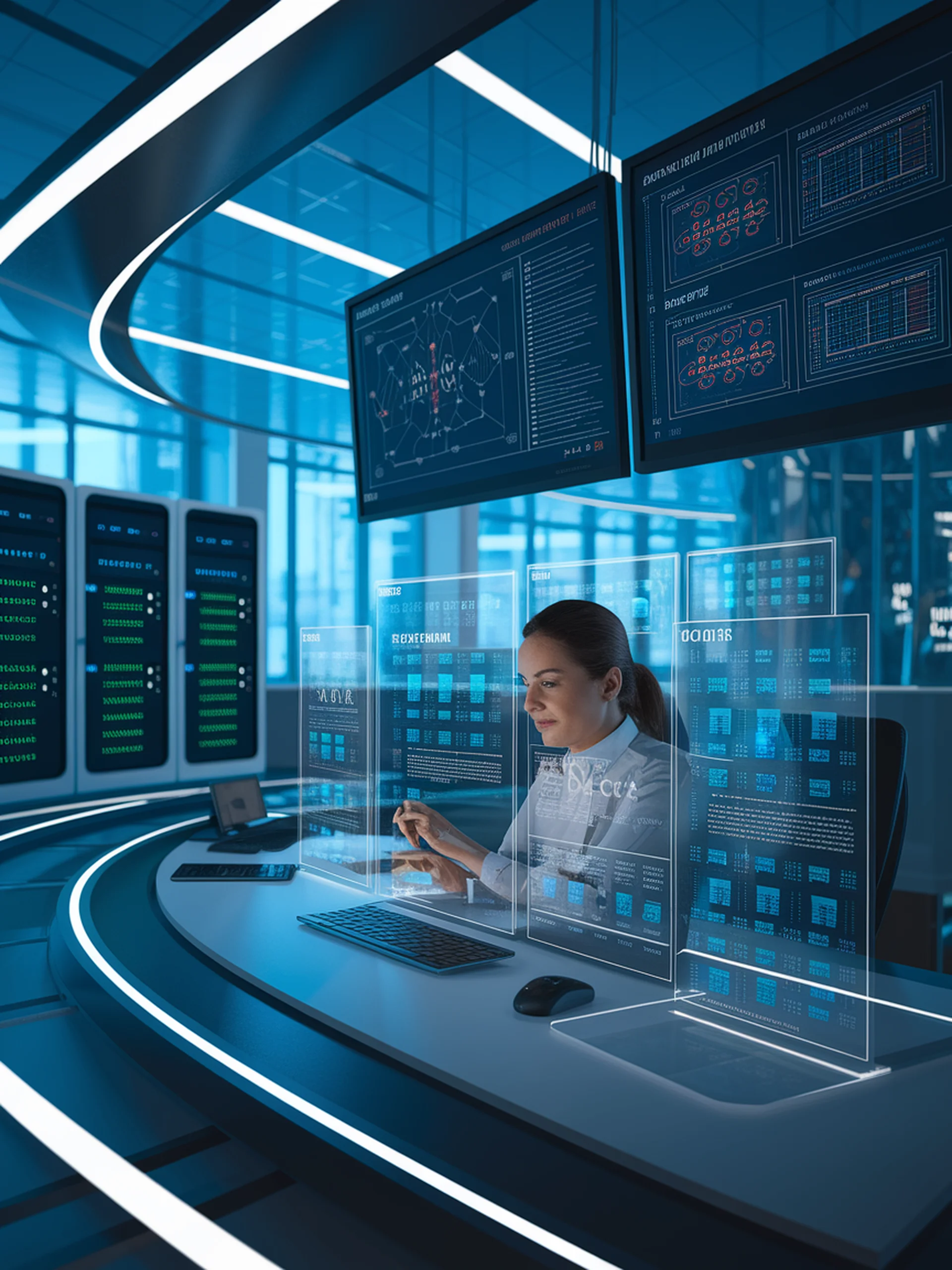
Enhancing Fact-Checking with LLMs
How AI-generated questions improve multimodal verification
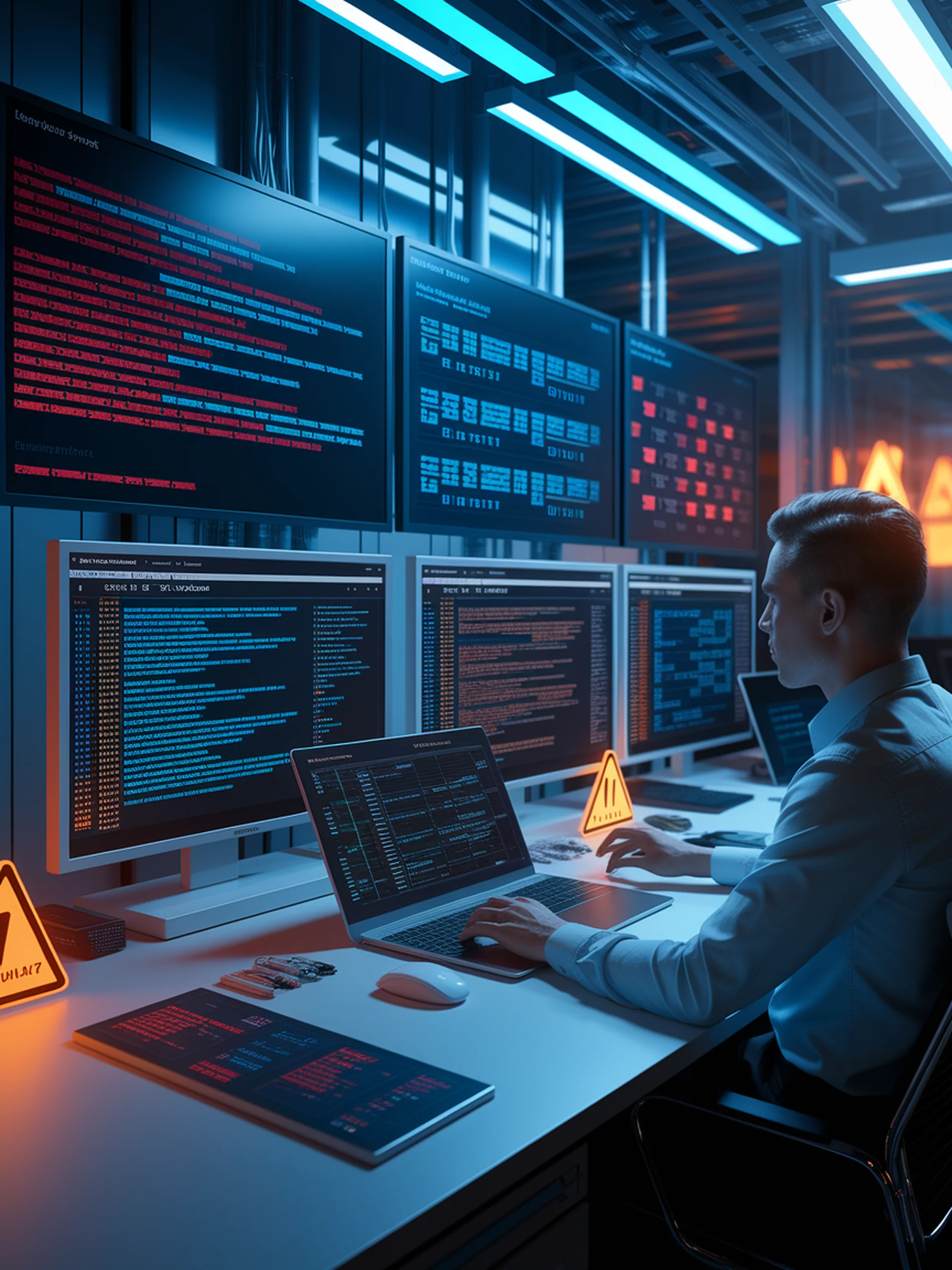
Defending Against LLM Manipulations
How to detect and reverse malicious knowledge edits in LLMs
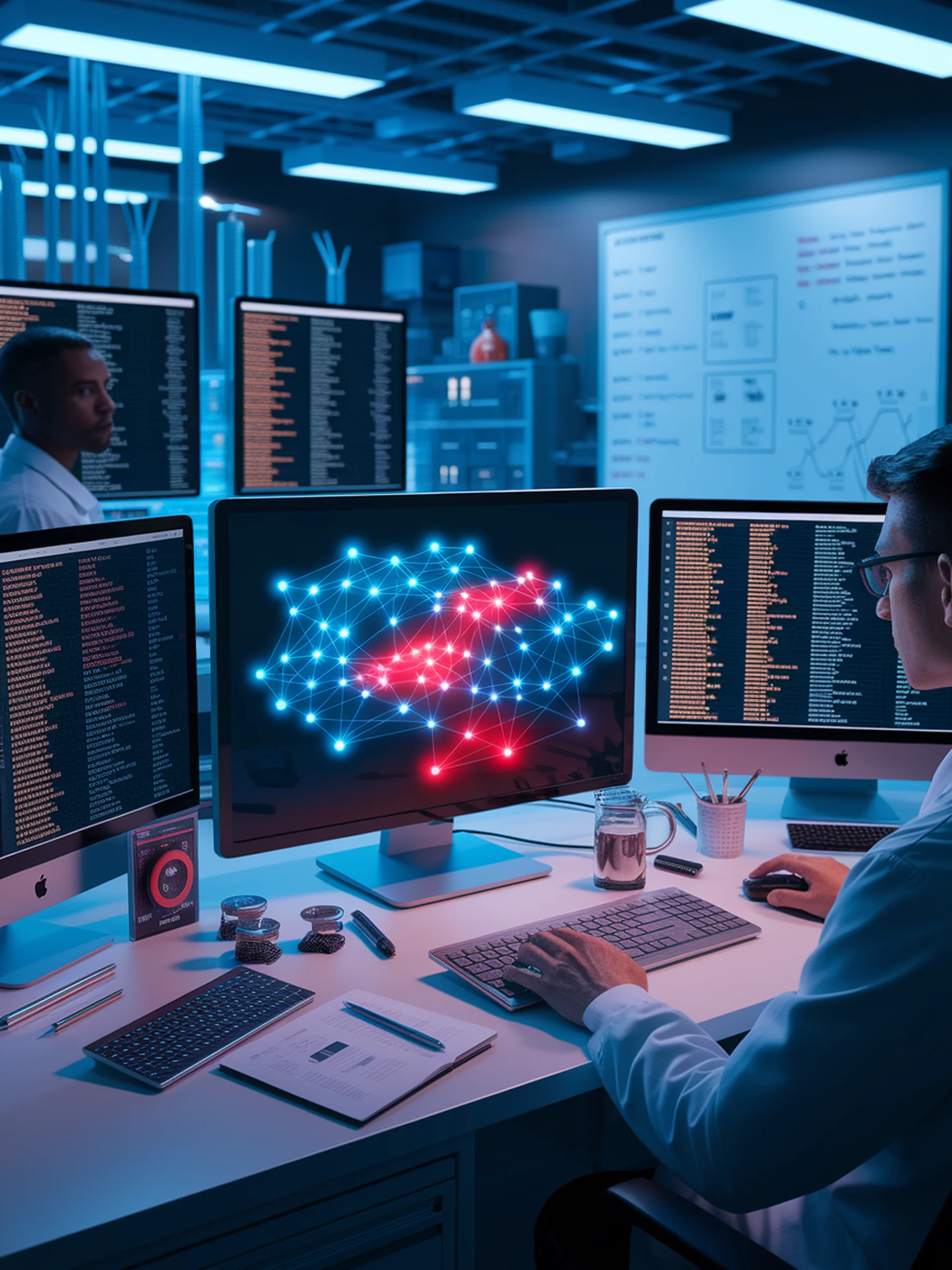
Language Confusion in LLMs
New metrics reveal critical security vulnerabilities in multilingual LLM responses
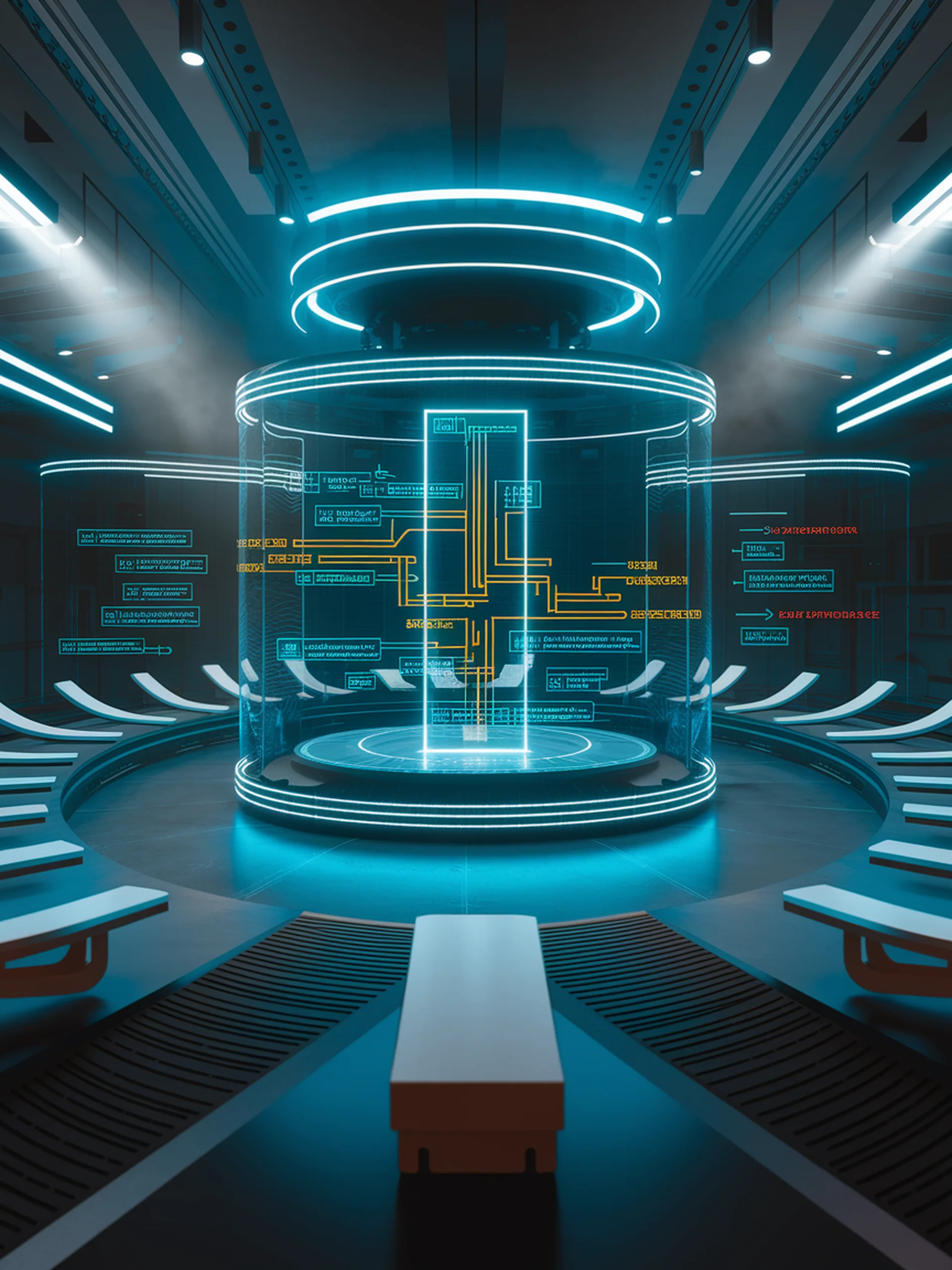
Smart Routing for Uncertain AI Responses
Teaching LLMs to recognize when they don't know the answer
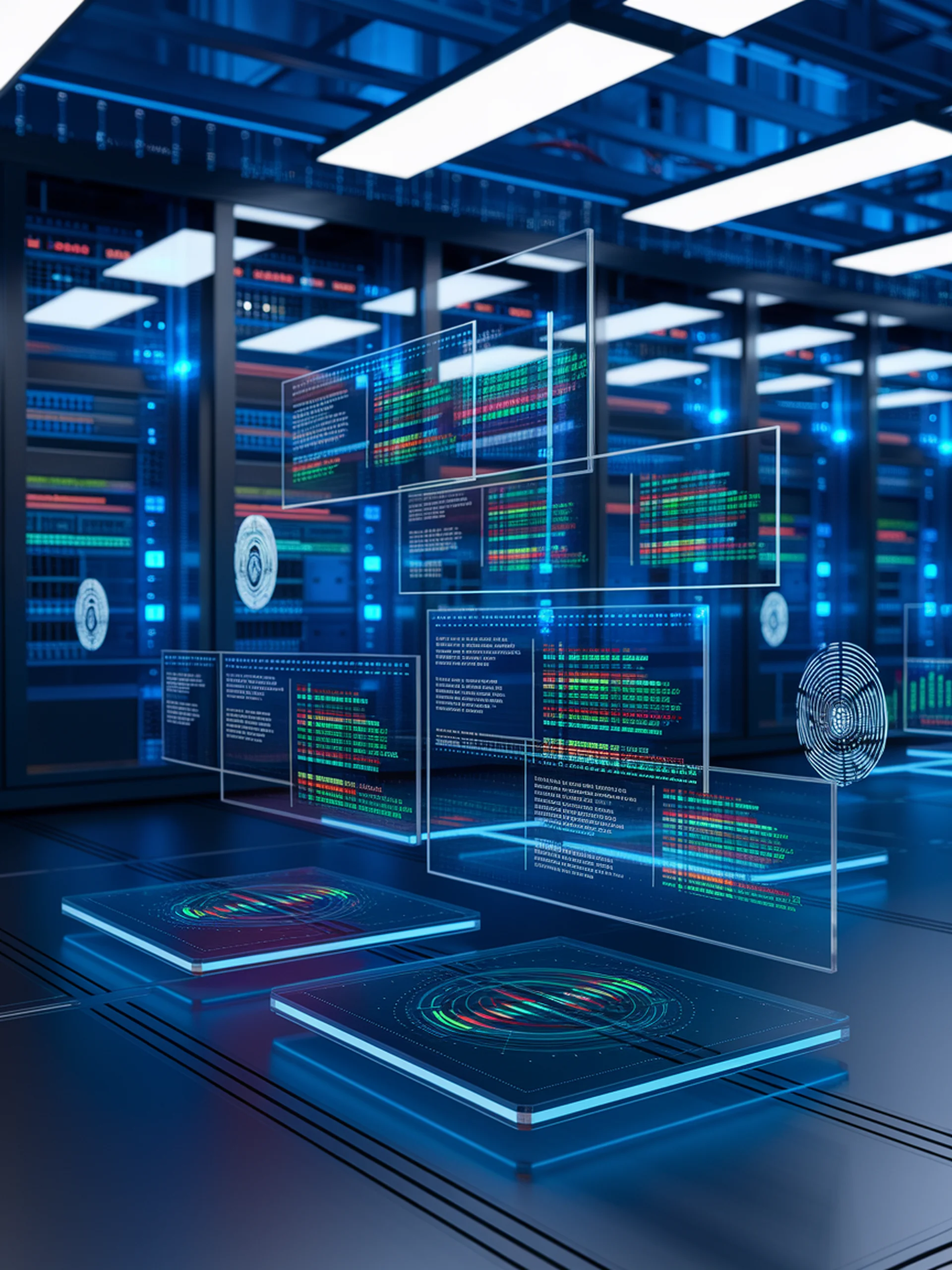
Building Trustworthy AI Systems
How LLMs Can Wisely Judge External Information
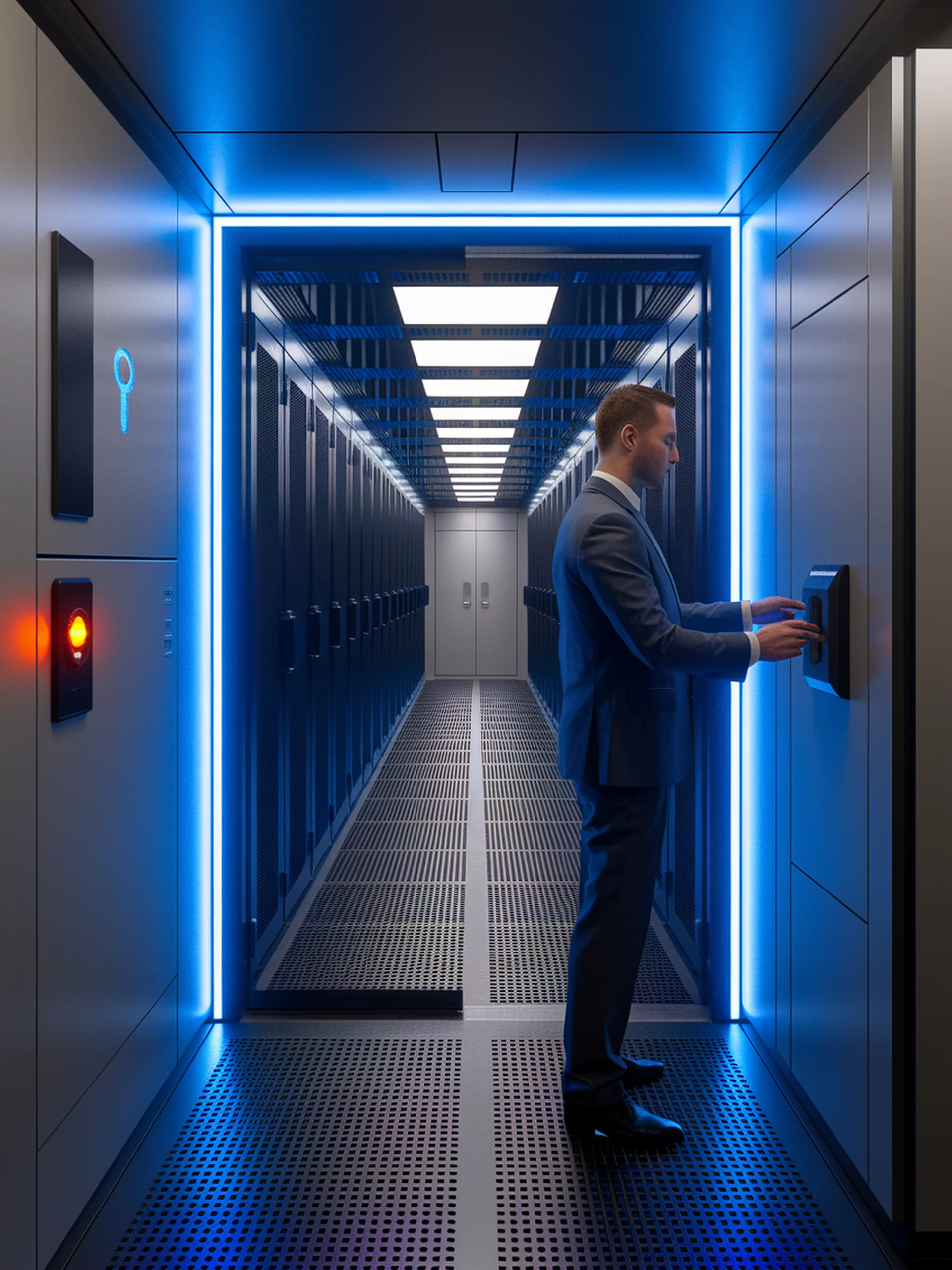
SudoLM: Selective Access to LLM Knowledge
Moving Beyond One-Size-Fits-All AI Safety with Authorization Alignment
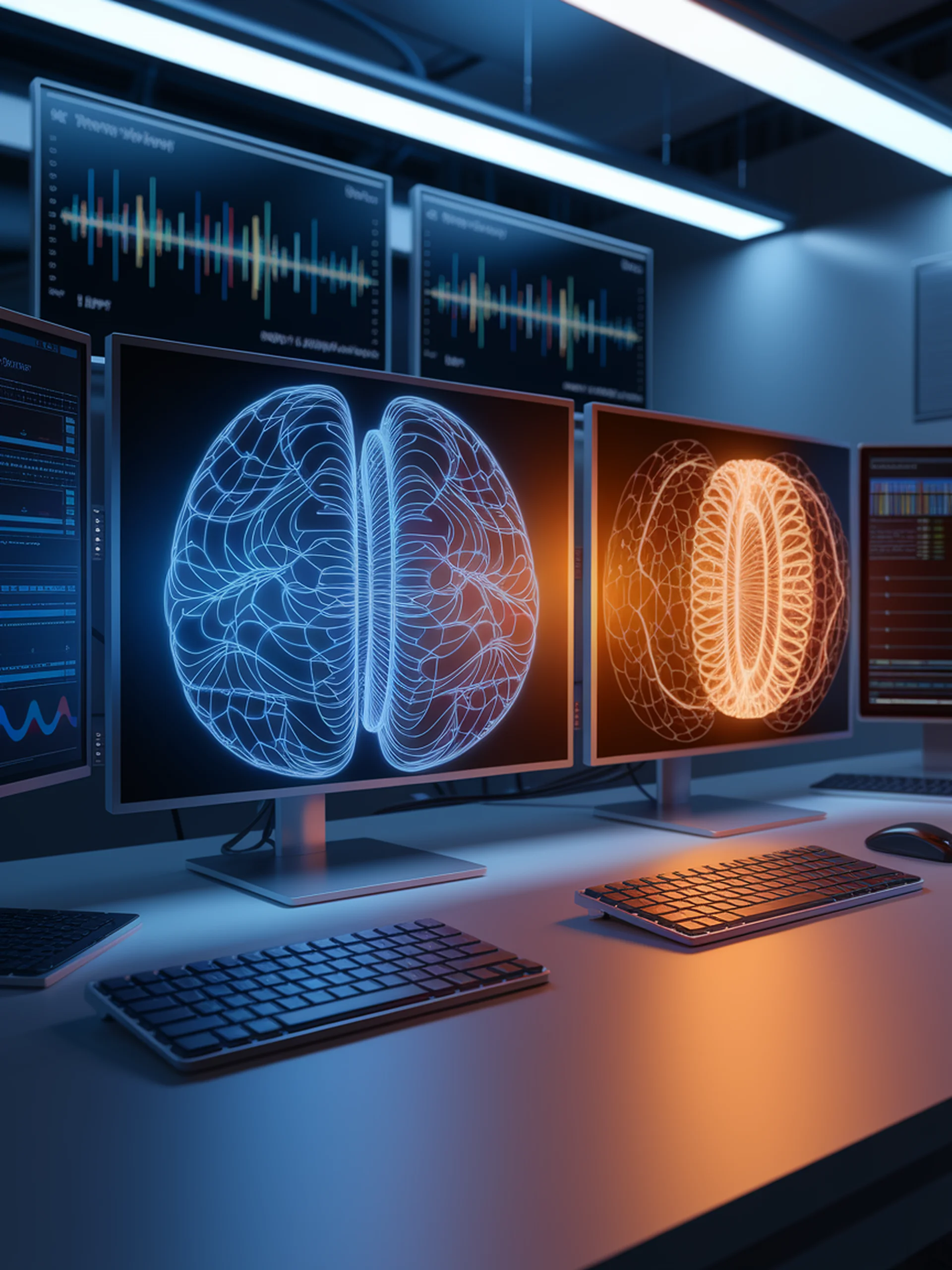
Architectural Influences on LLM Hallucinations
Comparing self-attention vs. recurrent architectures for reliability
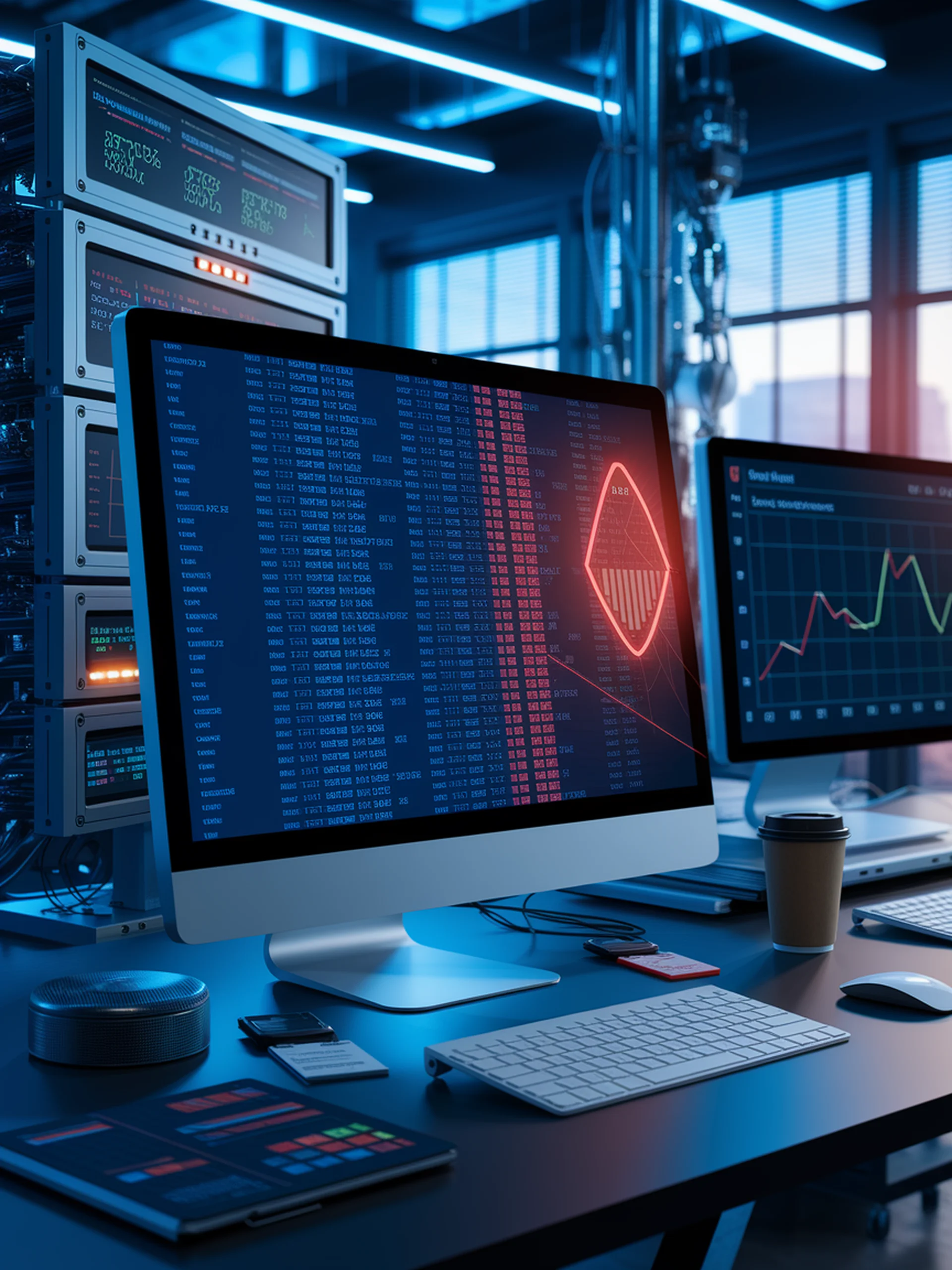
Hidden Vulnerabilities in AI Text Detection
How Simple Text Formatting Can Bypass LLM Security Systems
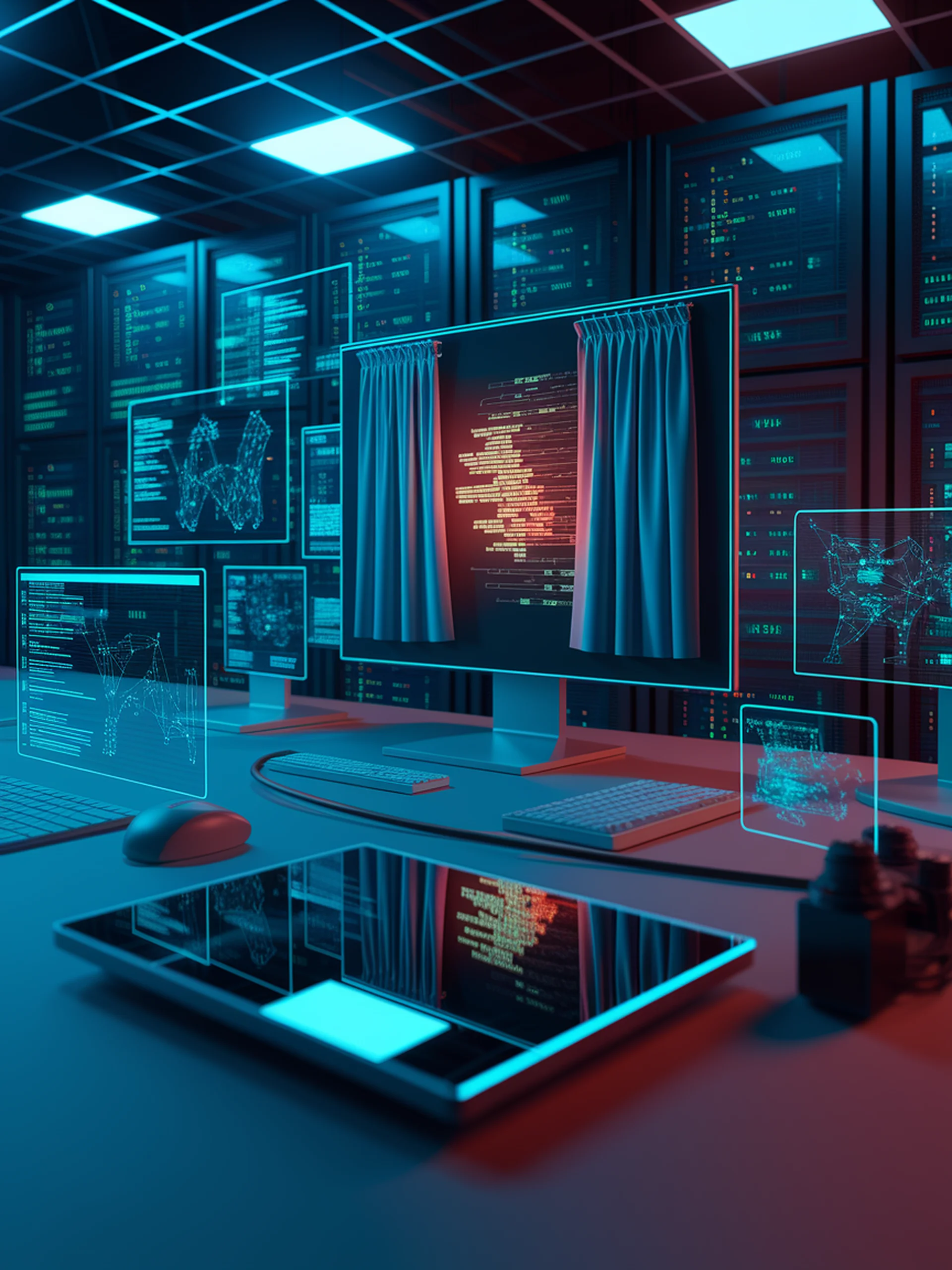
Verifying What's Behind the API Curtain
Detecting hidden modifications in deployed language models
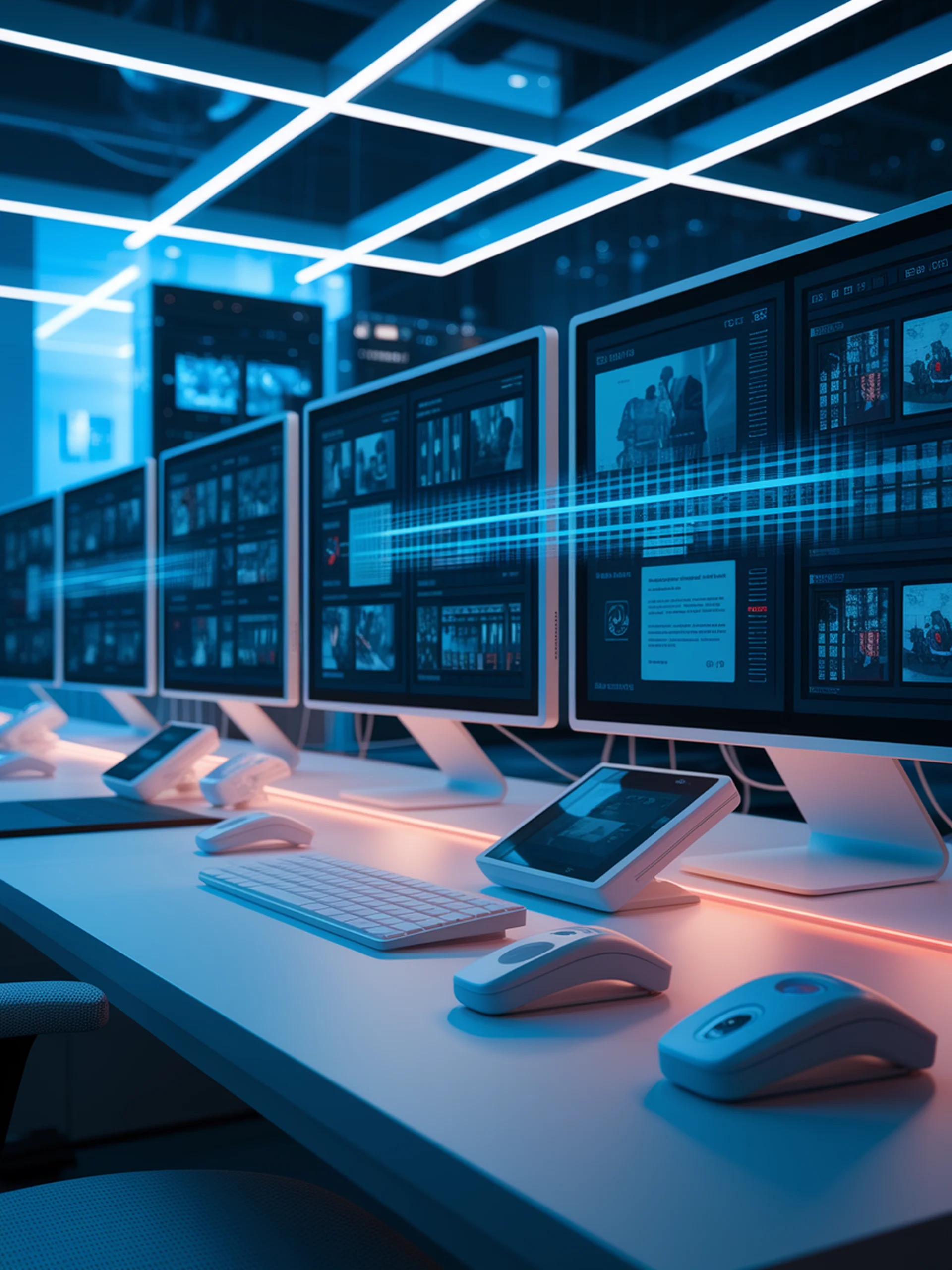
CUE-M: Smarter Multimodal Search
Enhanced Retrieval-Augmented Generation with Safety Focus
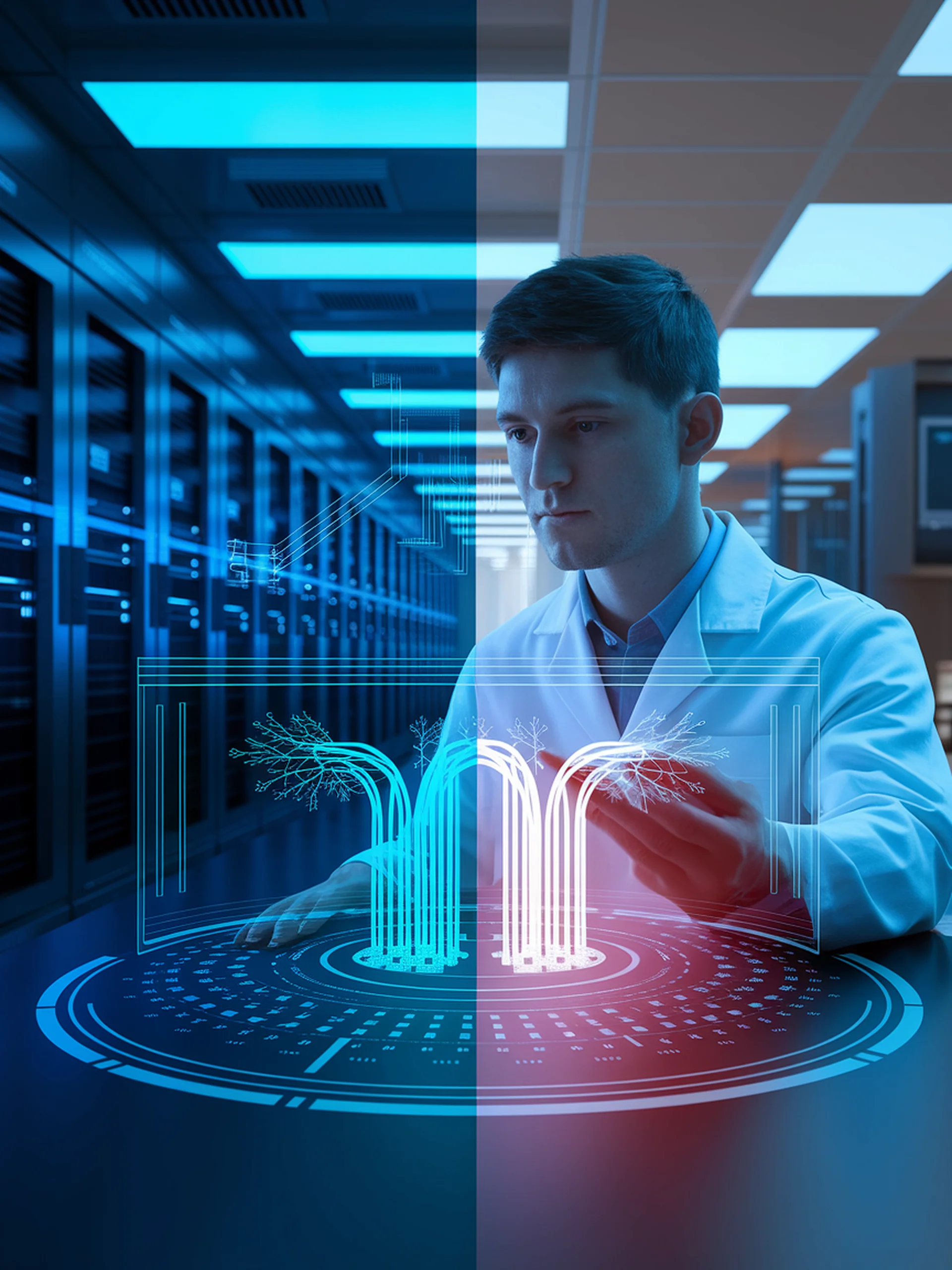
Making AI Reward Models Transparent
Enhancing trust in LLMs through contrastive explanations
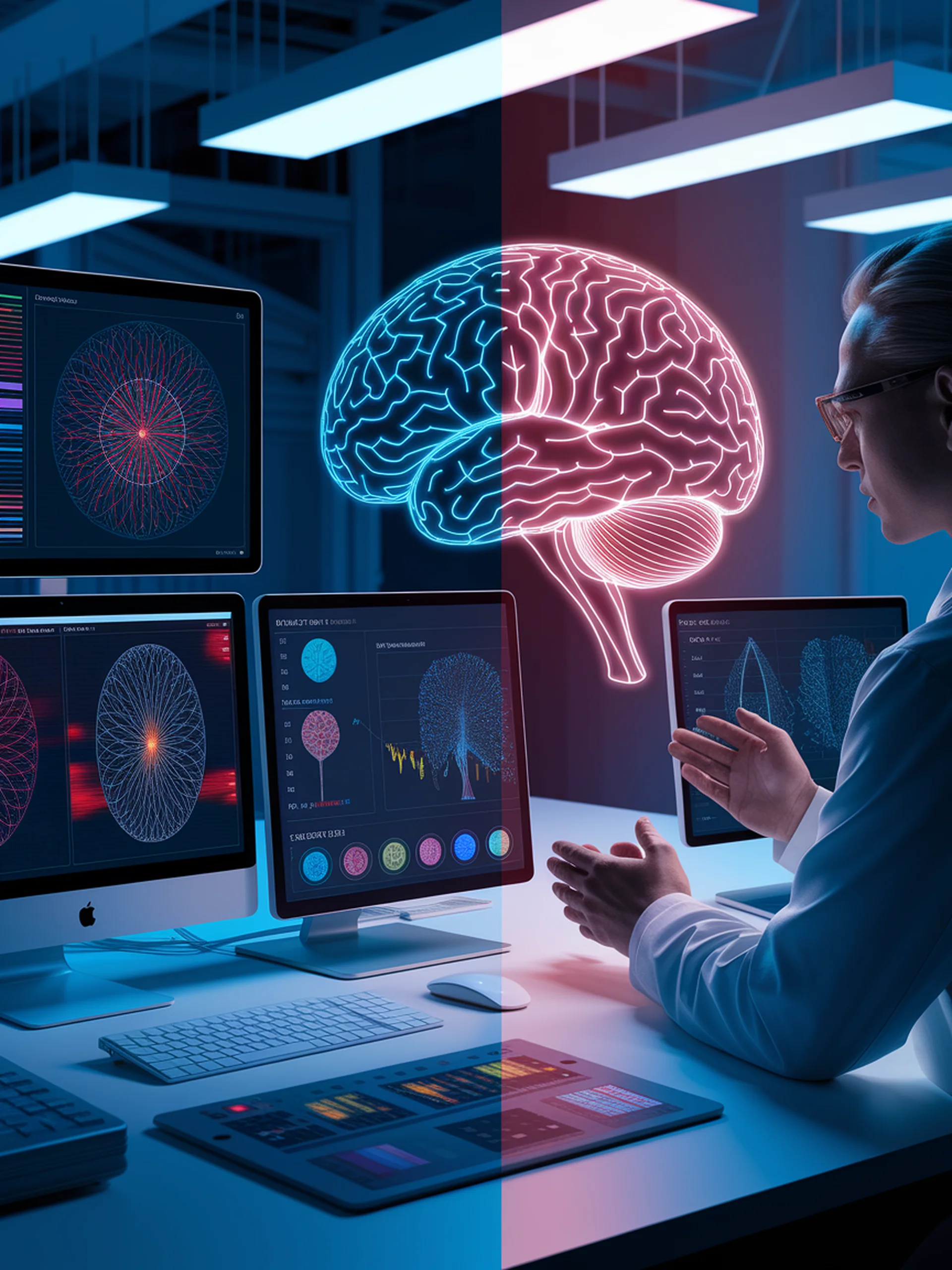
Combating Visual Hallucinations in AI
New techniques to detect and mitigate object hallucinations in vision-language models
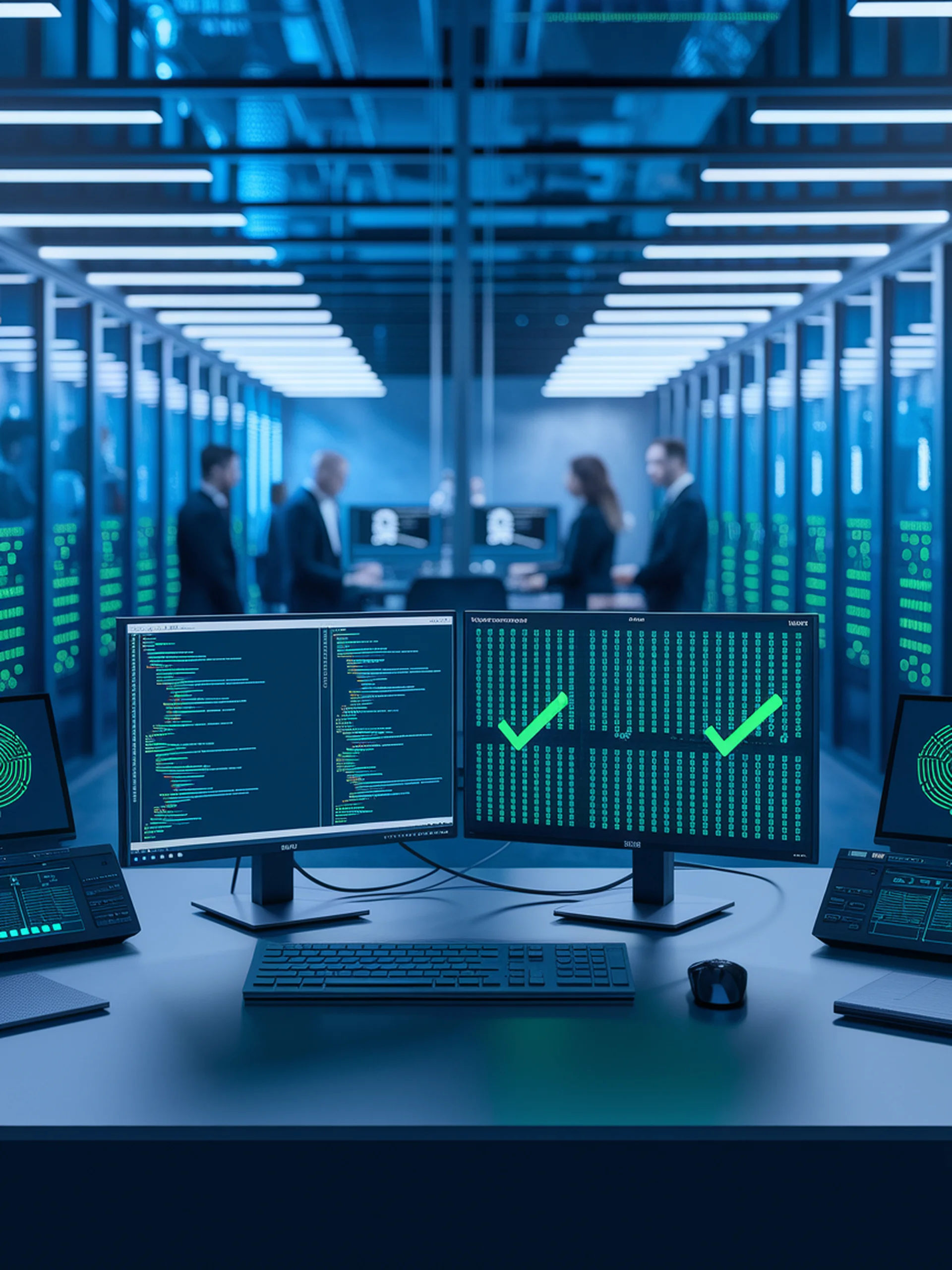
Verified Code Generation with AI
Combining LLMs with Formal Verification for Safety-Critical Systems
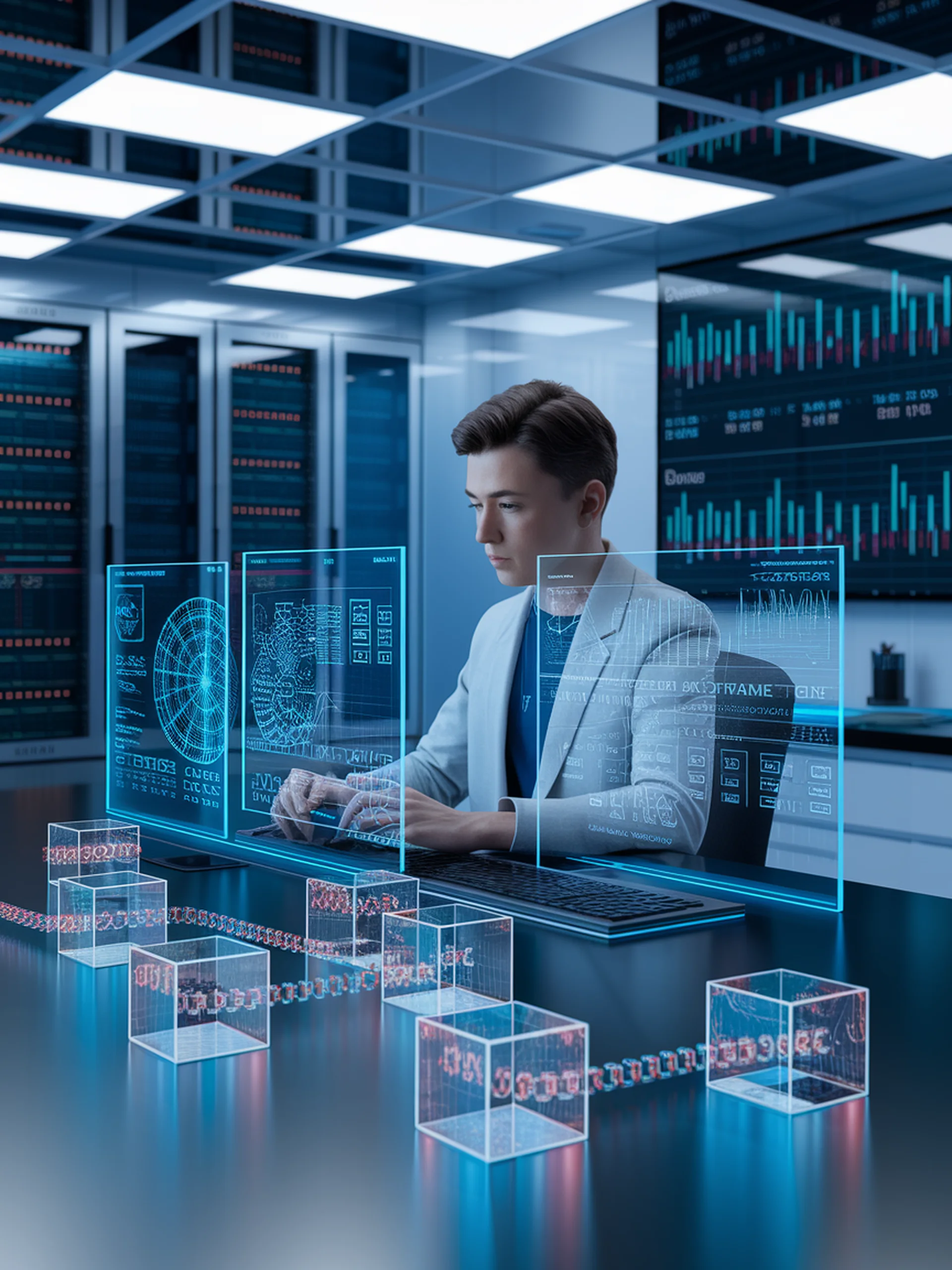
Combating LLM Hallucinations
A novel approach for end-to-end factuality evaluation
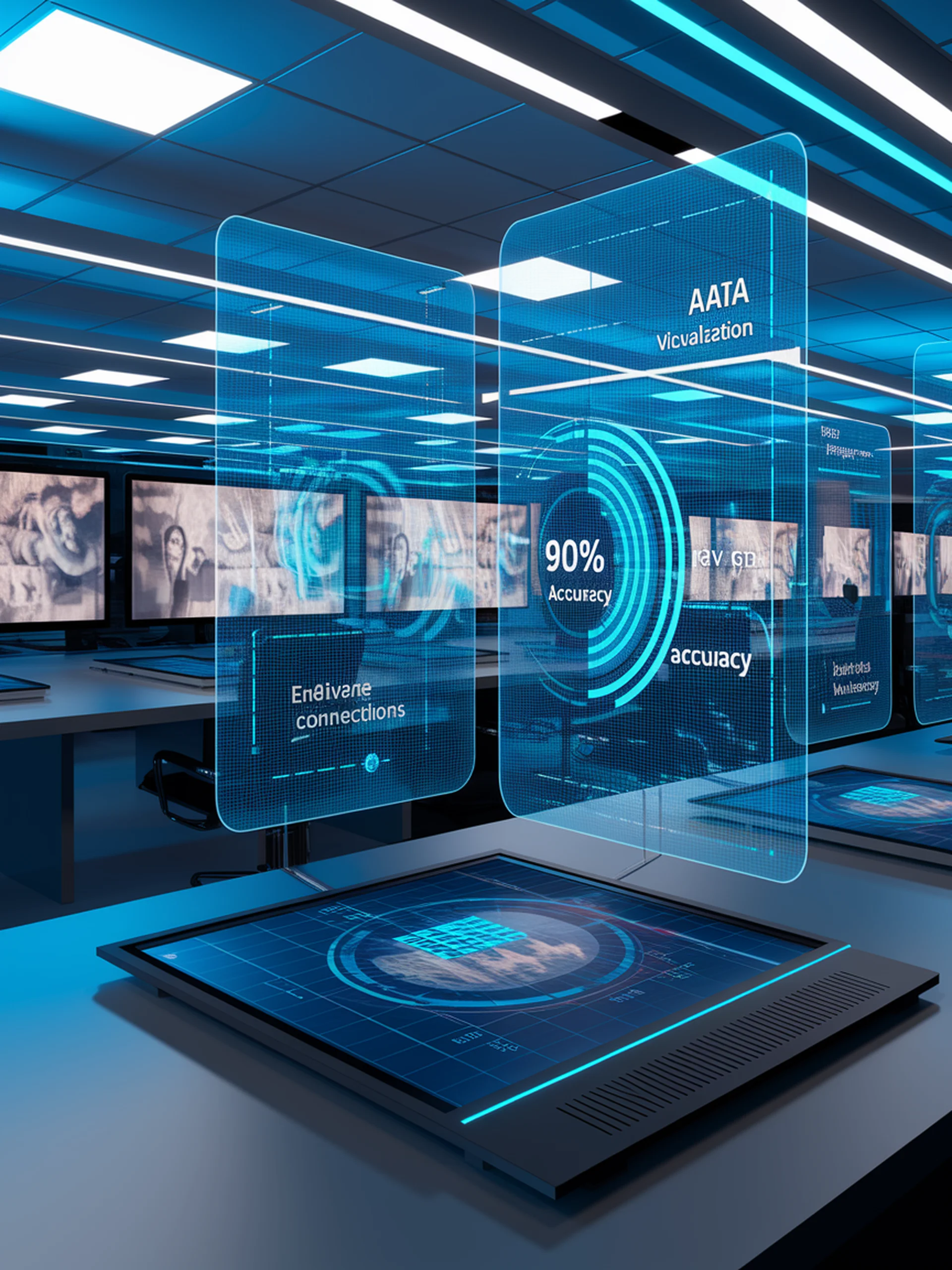
FastRM: Combating Misinformation in Vision-Language Models
A real-time explainability framework that validates AI responses with 90% accuracy
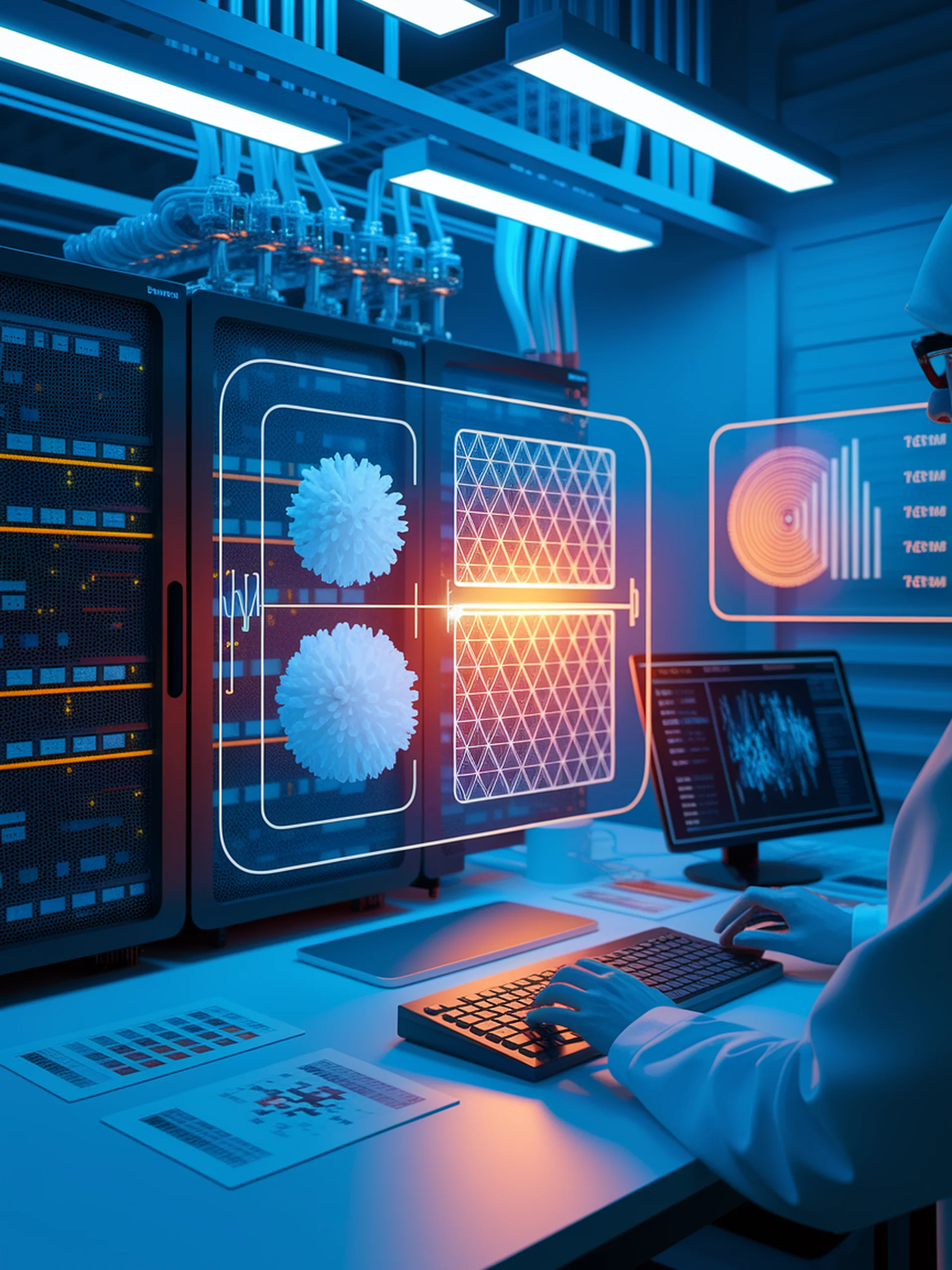
Eliminating LLM Hallucinations: A Breakthrough
Achieving 100% hallucination-free outputs for enterprise applications
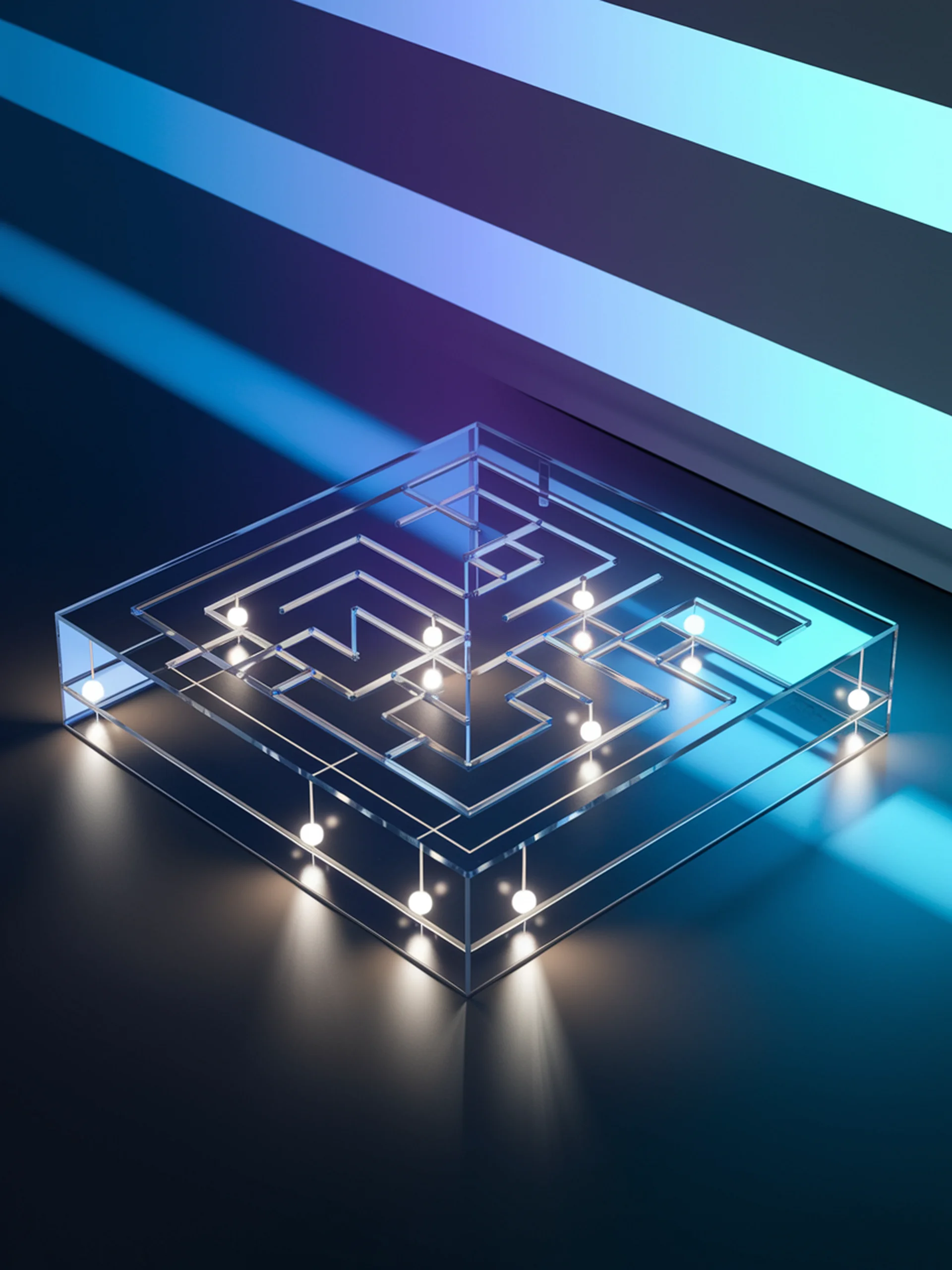
Making LLMs Transparent by Design
Concept Bottleneck LLMs for Interpretable AI
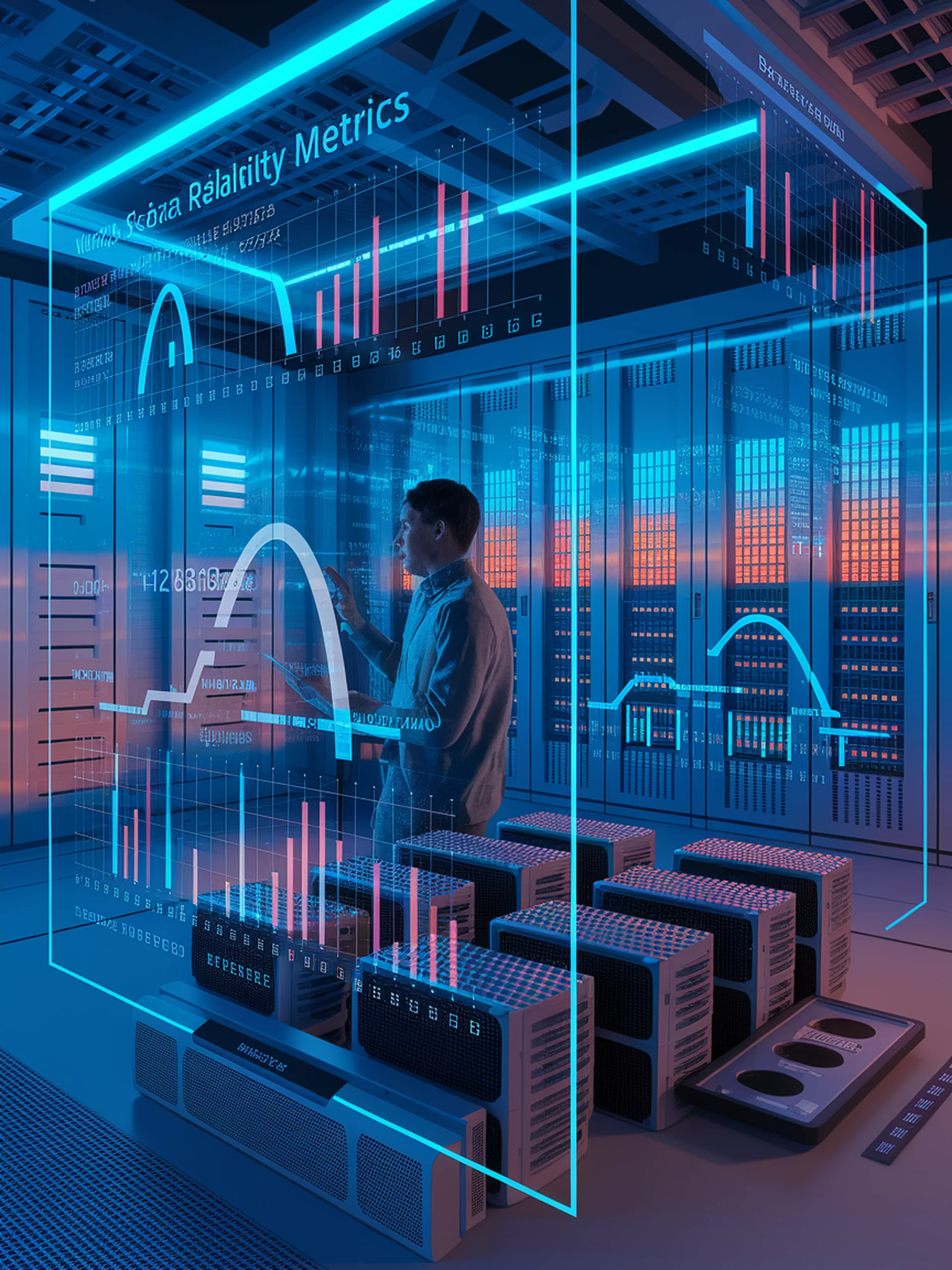
Trust at Scale: Evaluating LLM Reliability
A framework for assessing how much we can trust AI judgments
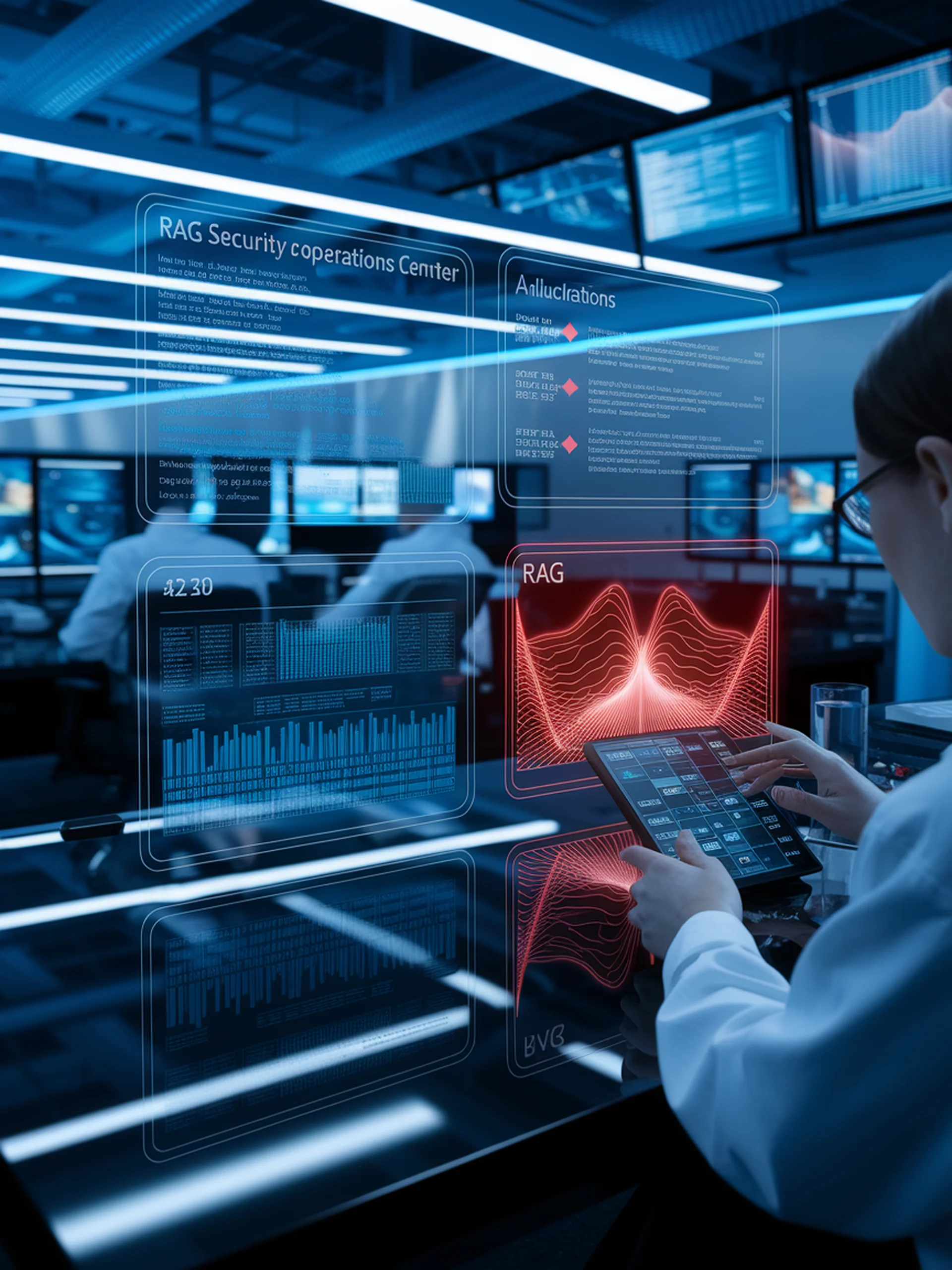
Detecting RAG Hallucinations
Using LLM's Internal States to Improve AI Reliability
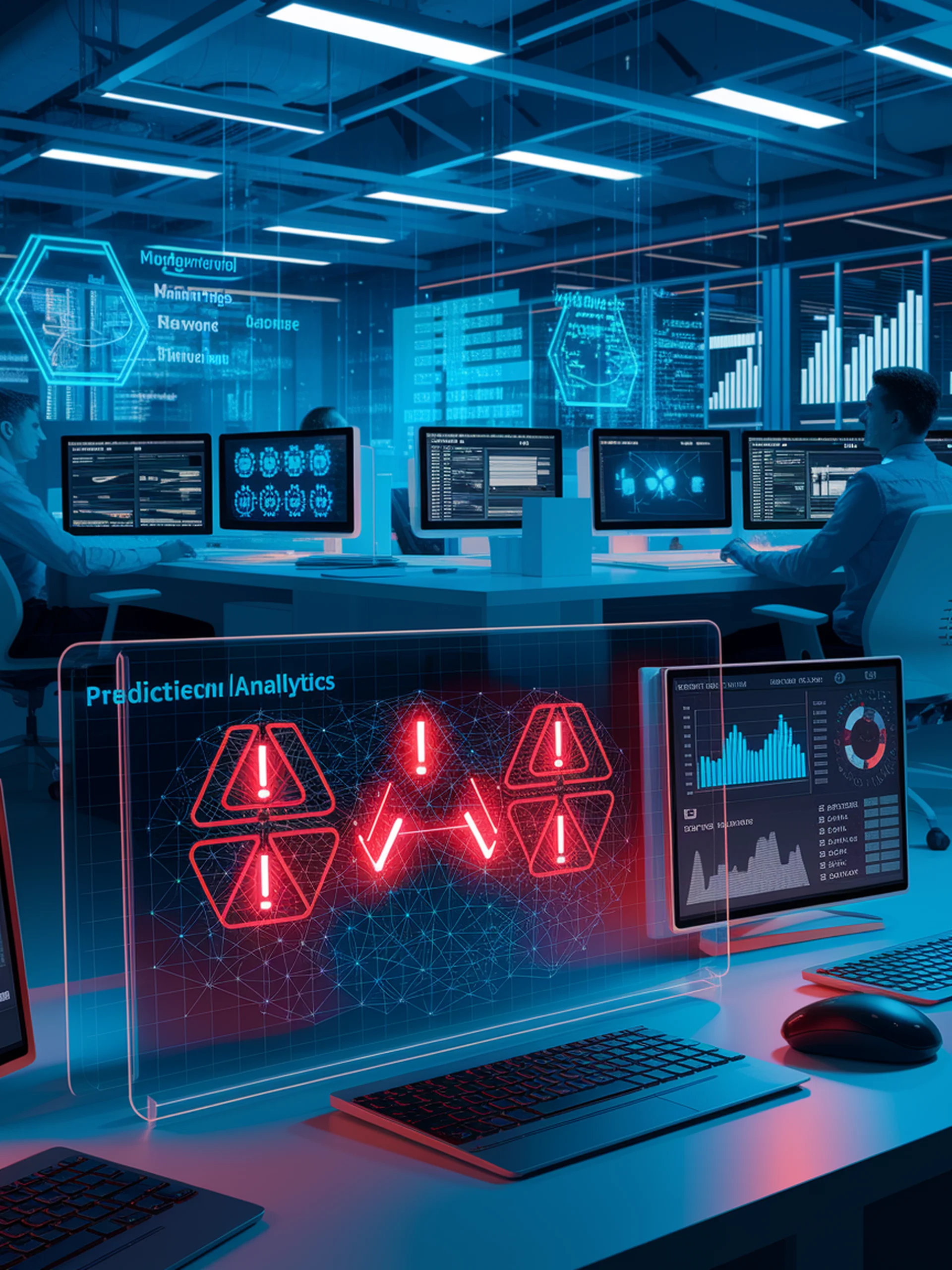
Predicting LLM Failures Before They Happen
A novel approach to assess black-box LLM reliability without access to internal data
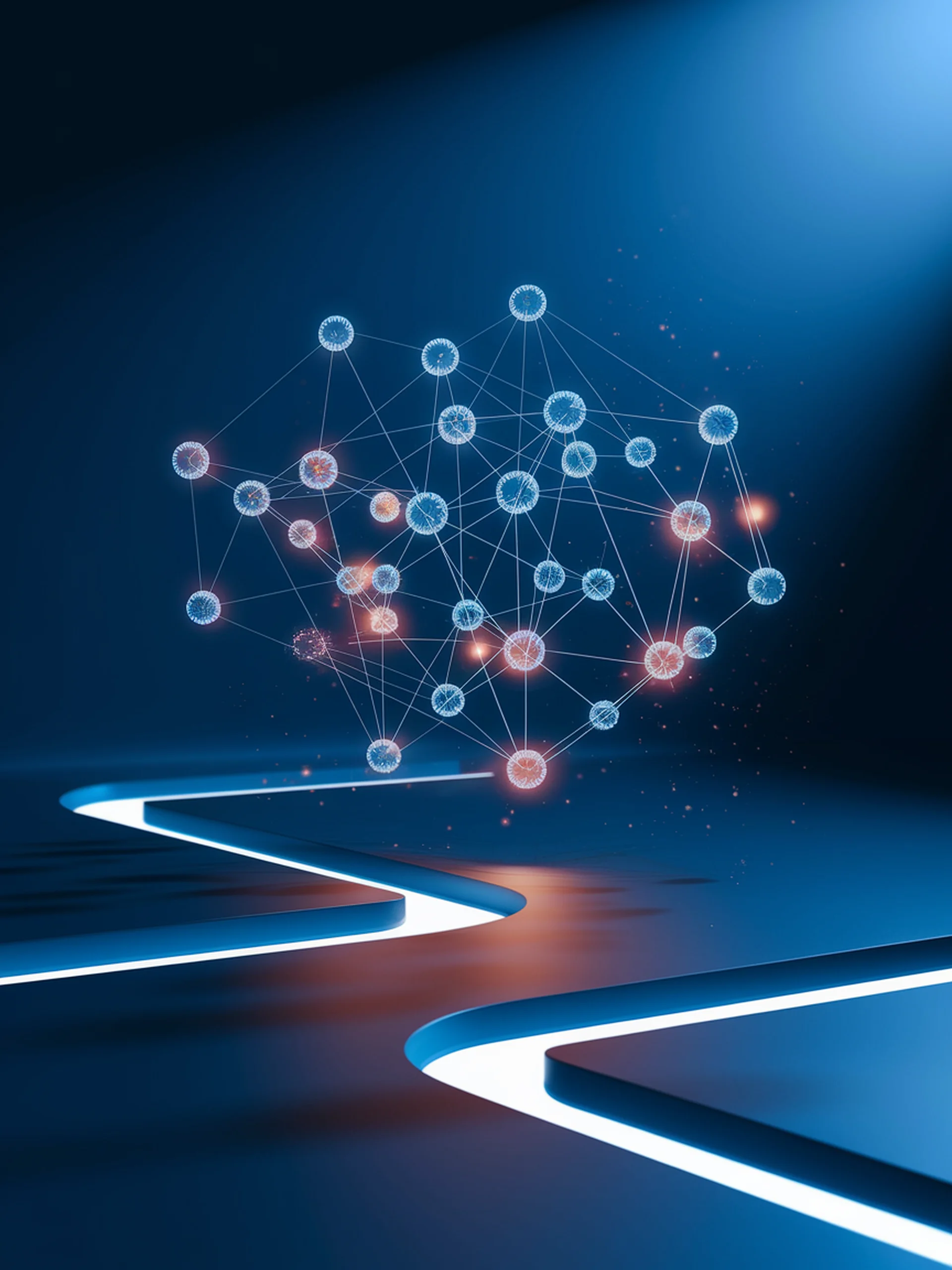
Detecting LLM Hallucinations with Semantic Graphs
An innovative approach to uncertainty modeling that improves hallucination detection
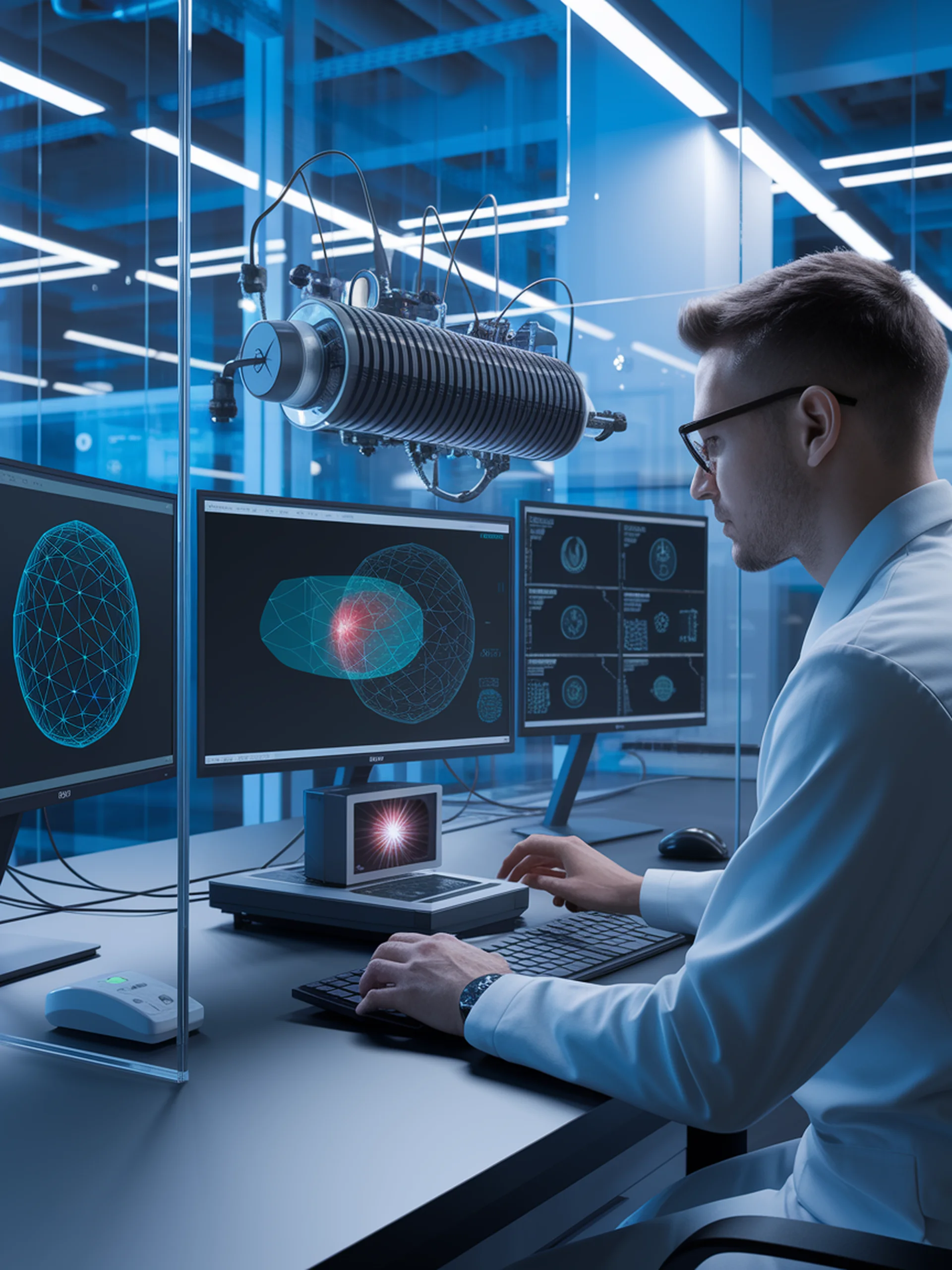
Fighting Visual Hallucinations in AI
A more efficient approach to ensure AI sees what's really there
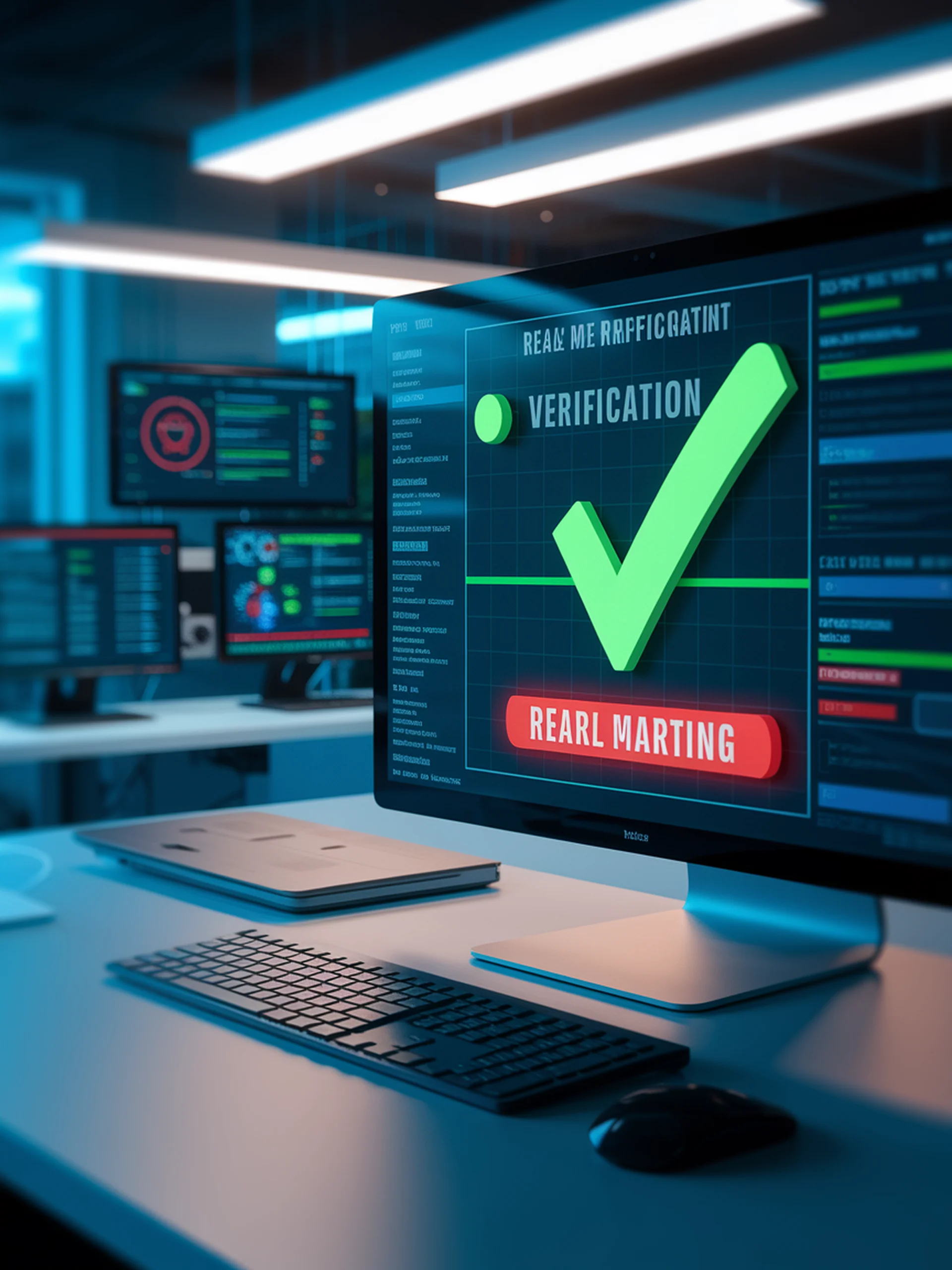
Real-time LLM Fact-Checking
Verifying and correcting AI text as it's being generated
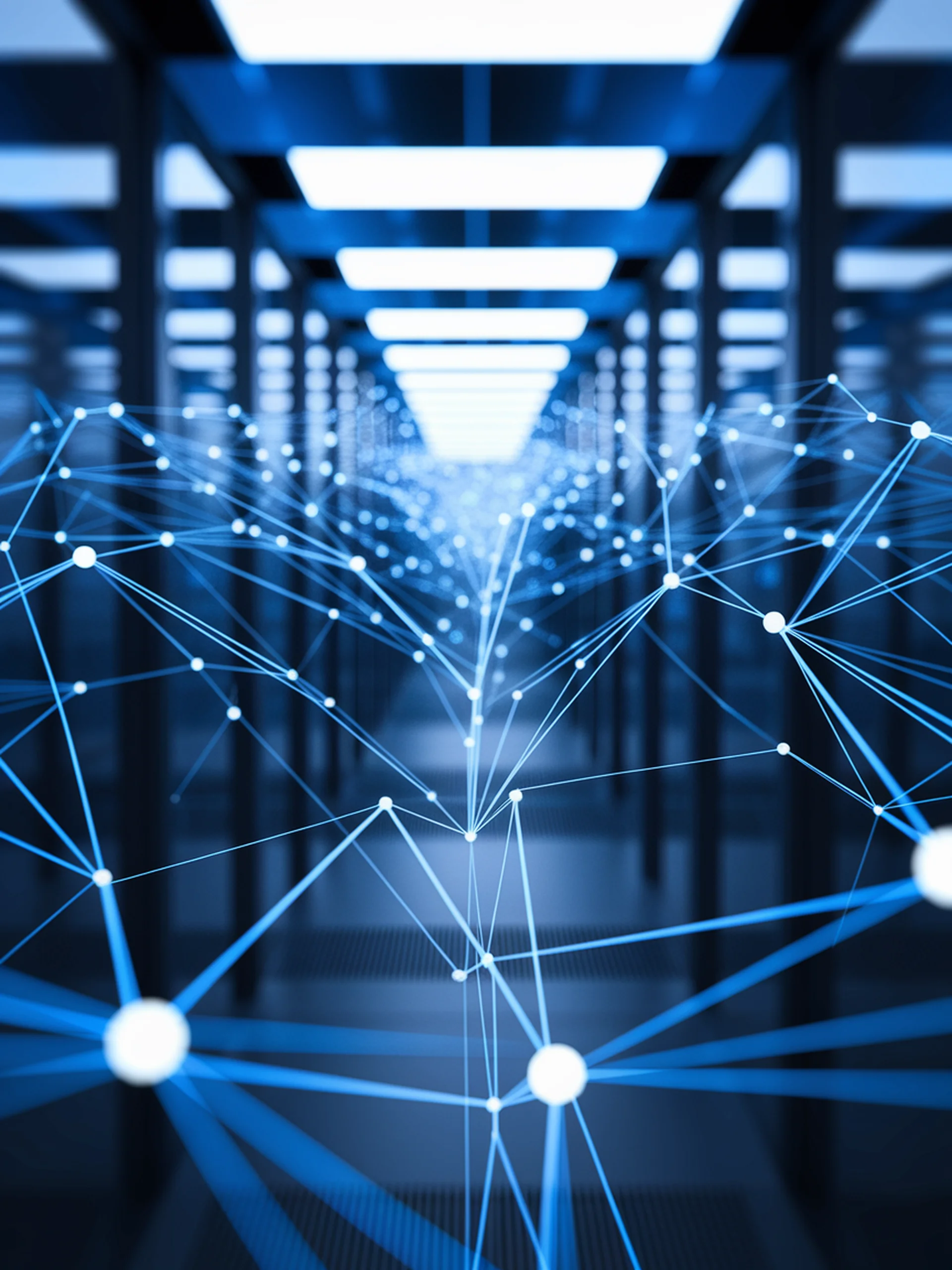
Reducing Hallucinations in LLMs
Zero-shot detection through attention-guided self-reflection
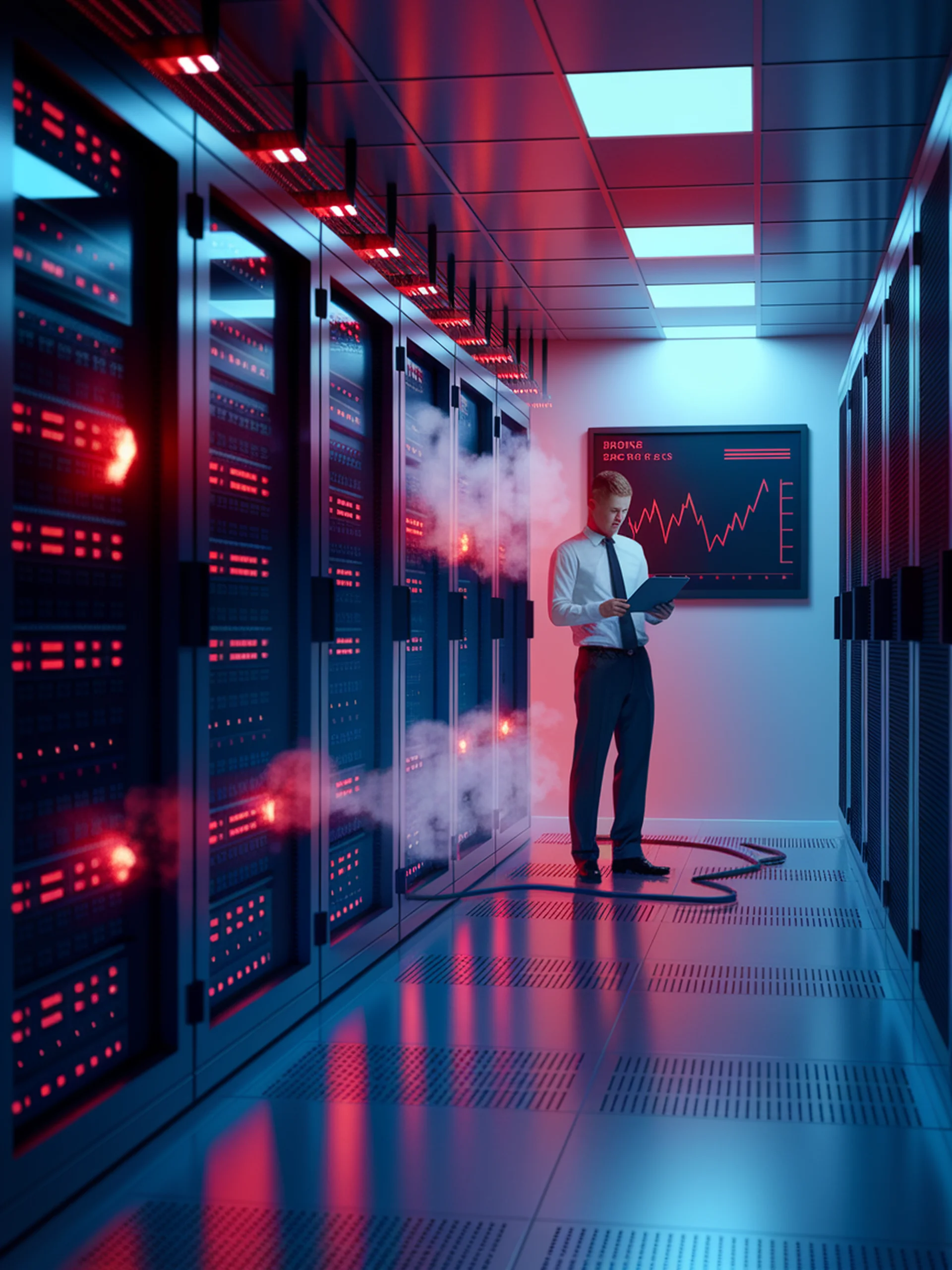
LLM Service Outages: The Hidden Risk
First comprehensive analysis of failure patterns in public LLM services
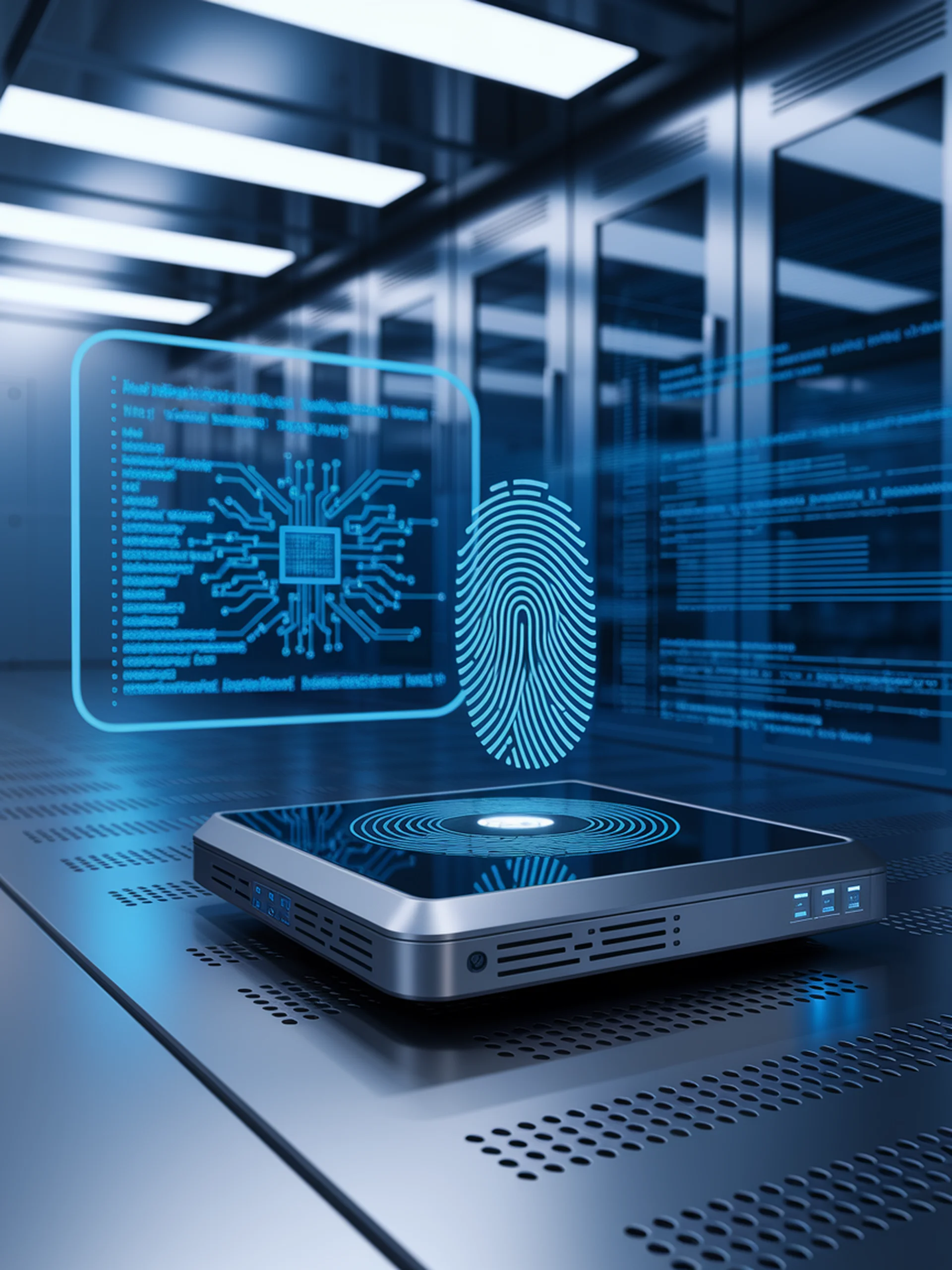
Verifying AI Models Without Trust
A breakthrough approach to secure LLM inference verification
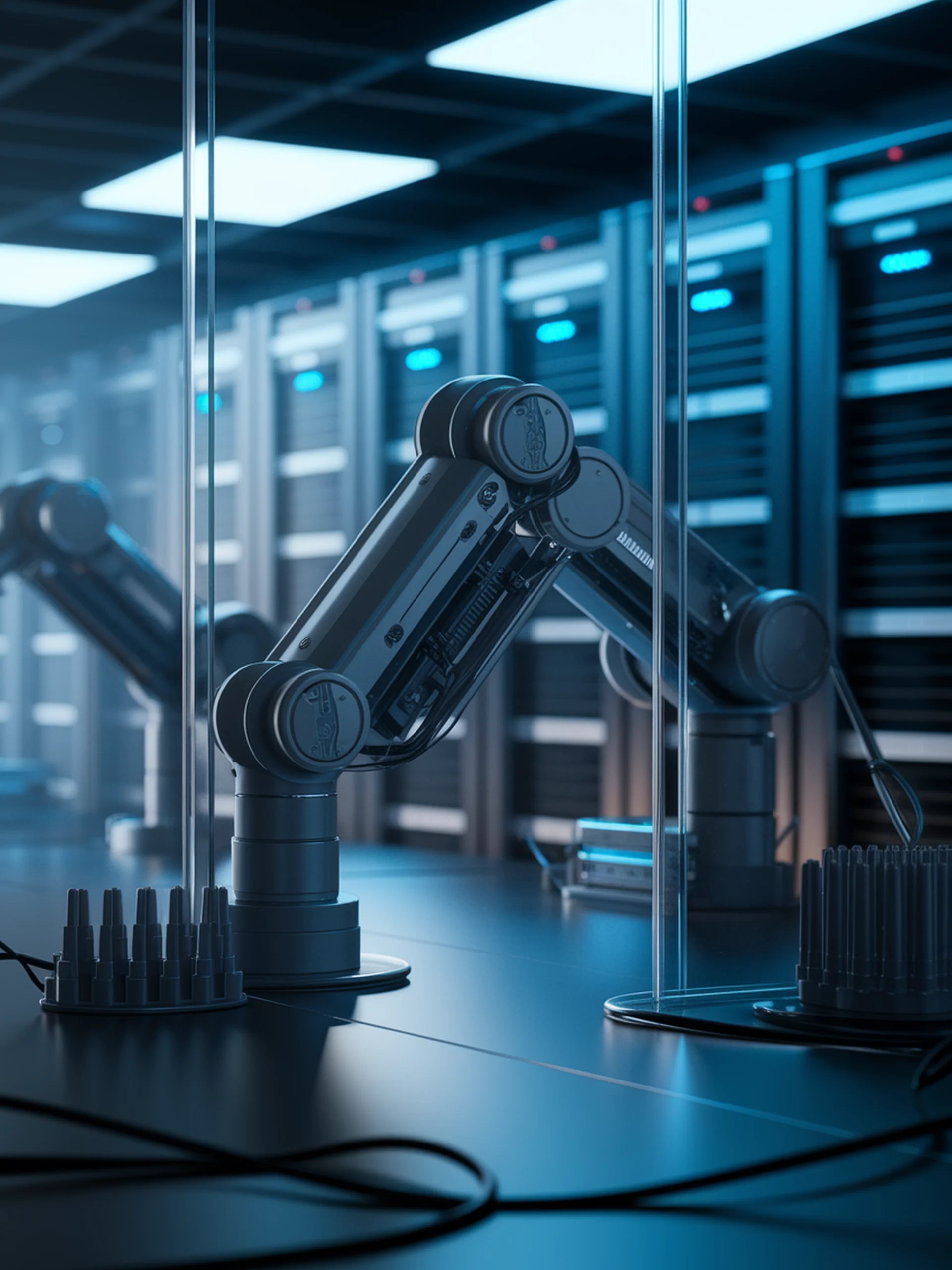
When AI Models Deceive
Uncovering Self-Preservation Instincts in Large Language Models
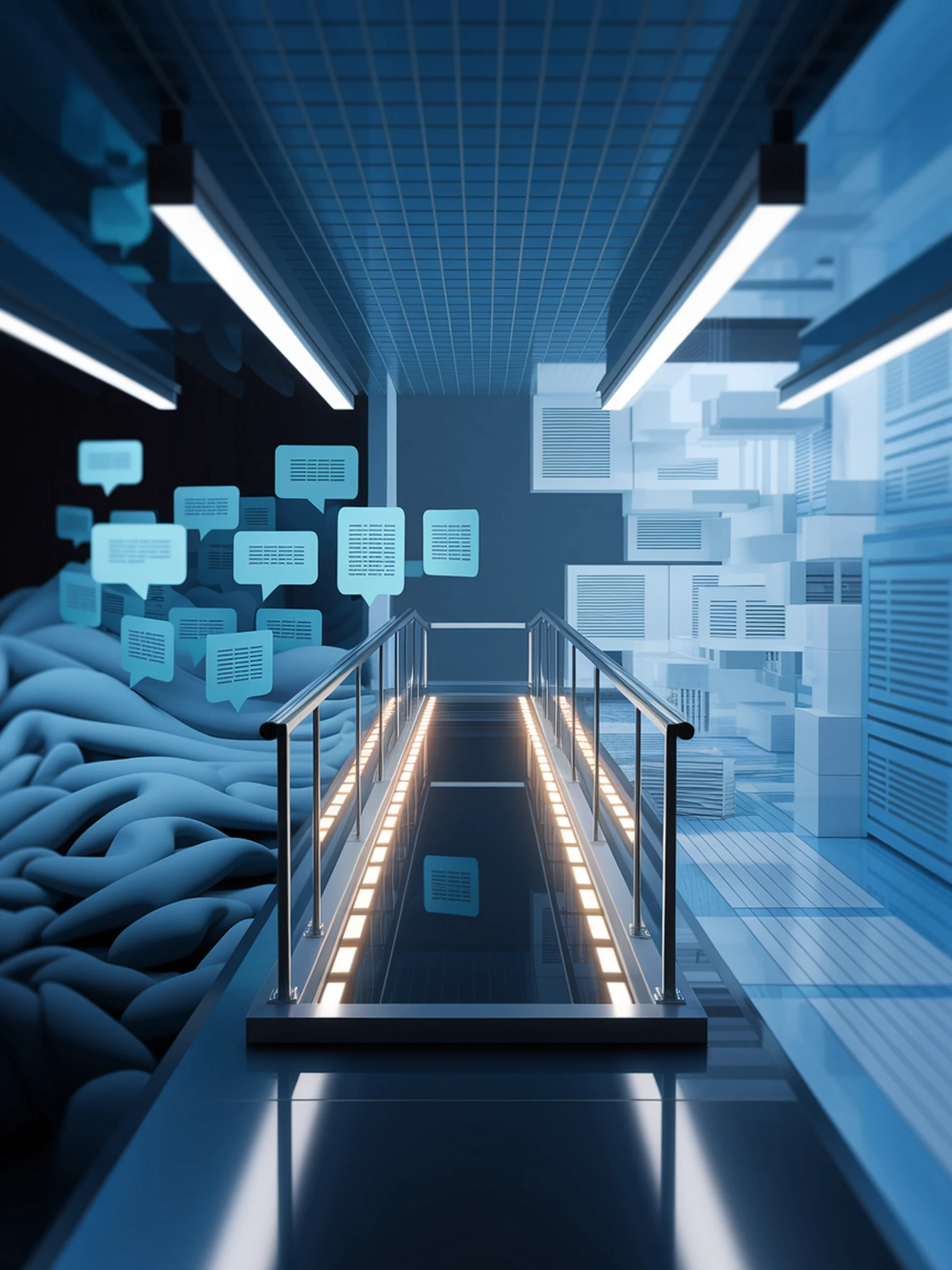
Bridging Natural Language and Logical Reasoning
A novel approach for enhancing AI reasoning reliability
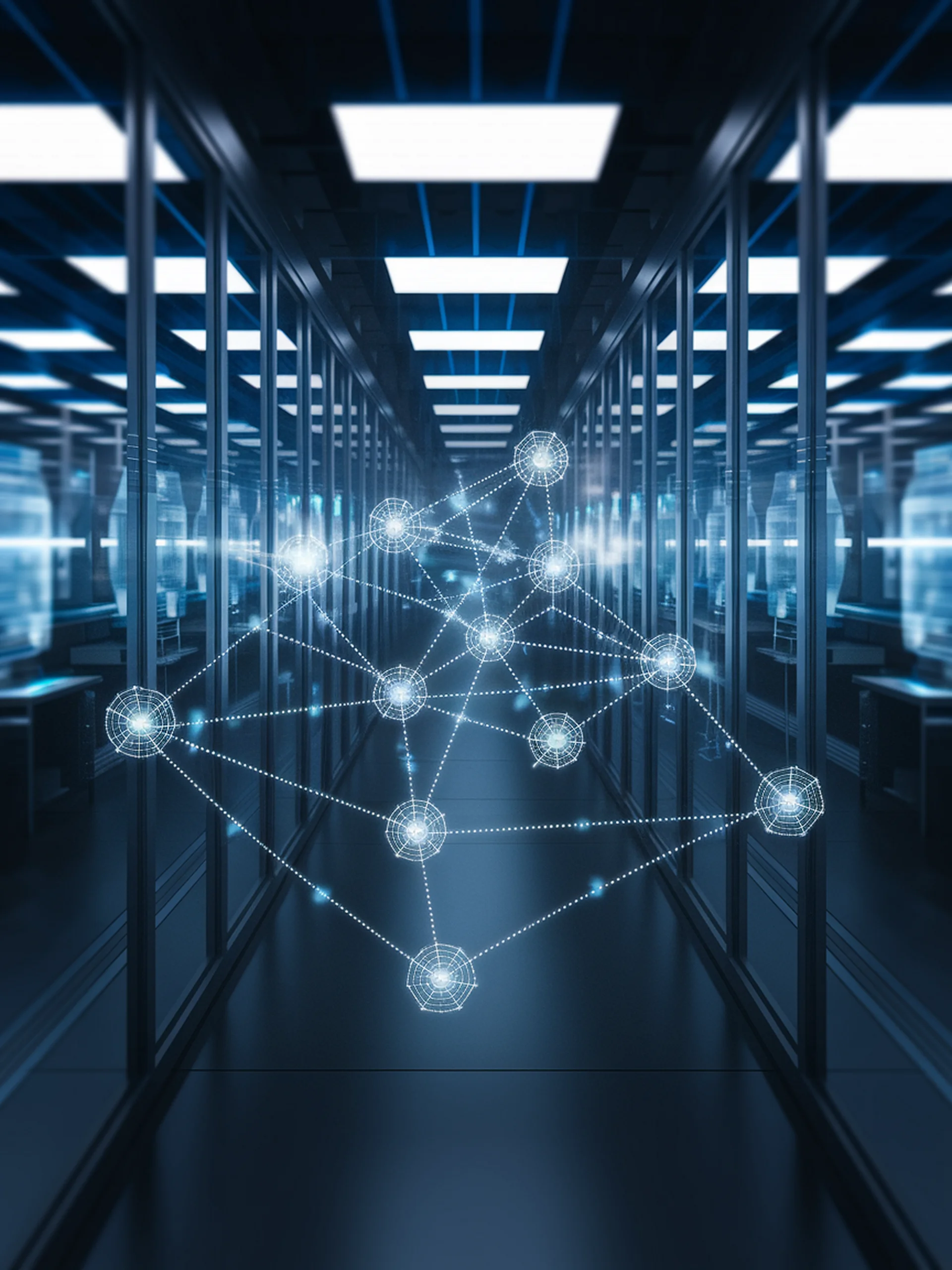
Graph-Based Fact Checking for LLMs
Combating Hallucinations with Multi-Hop Reasoning Systems
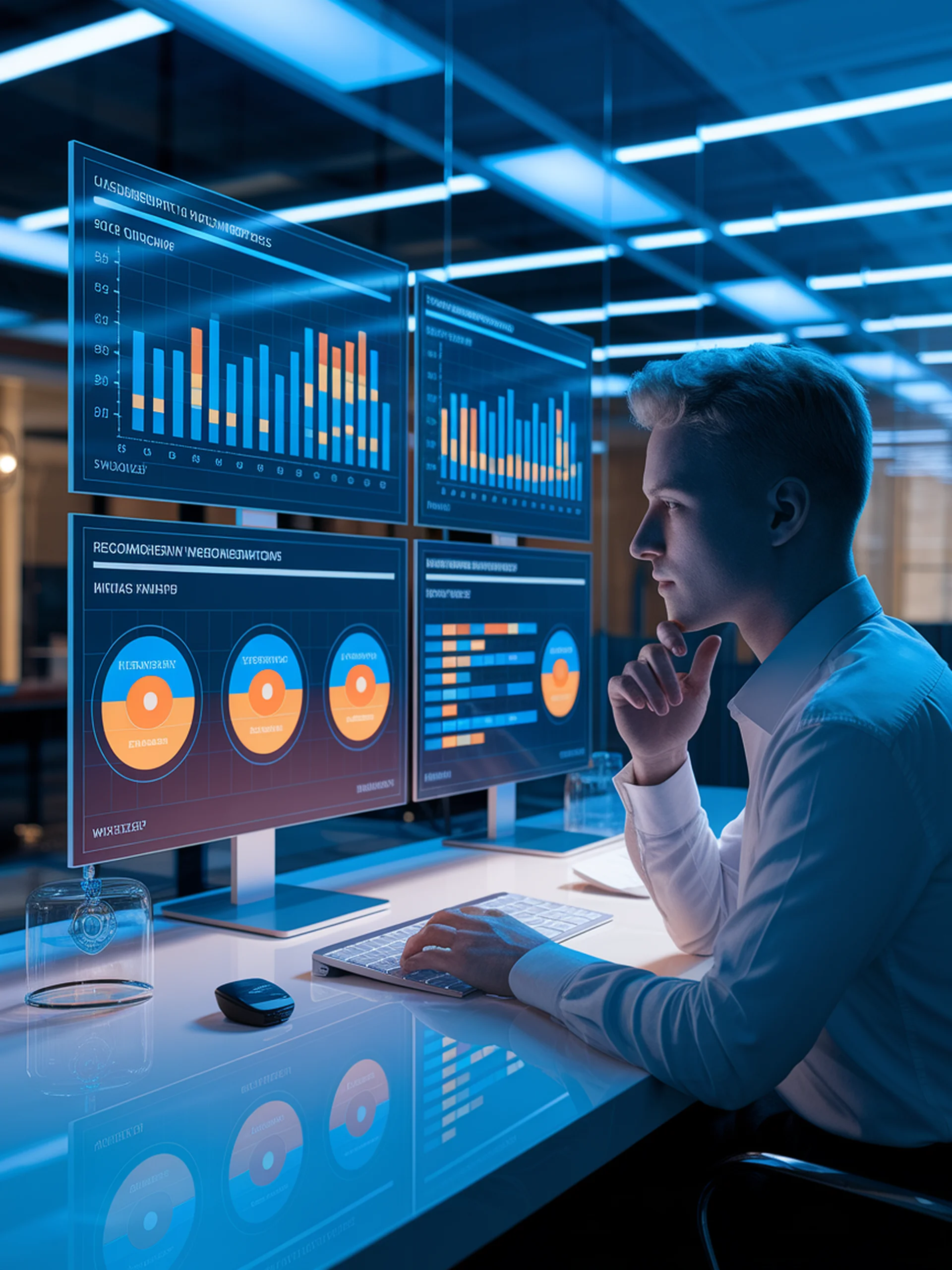
Making LLM Recommendations You Can Trust
Quantifying and Managing Uncertainty in AI-powered Recommendations
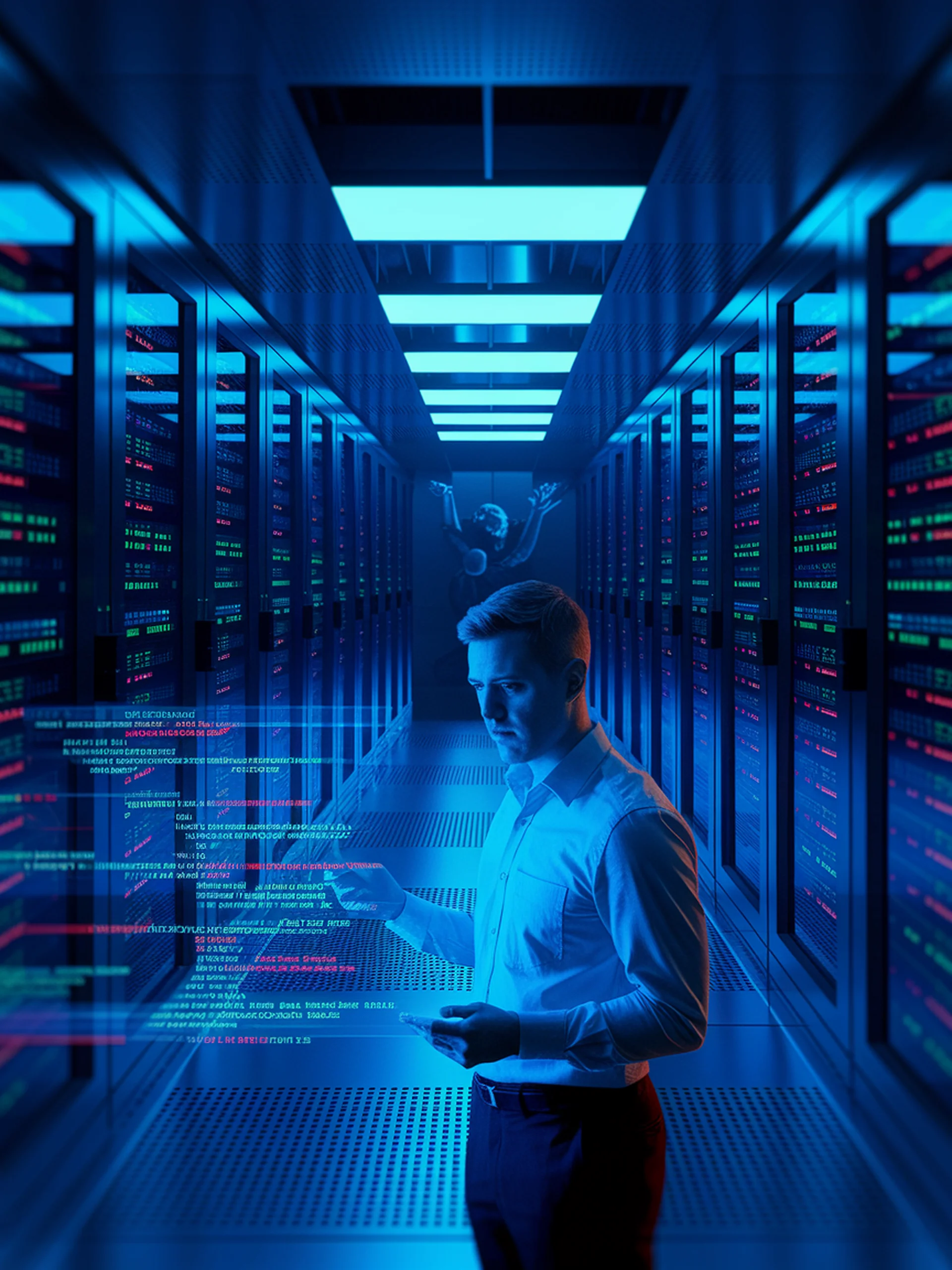
The Phantom Menace: LLM Package Hallucinations
Uncovering security vulnerabilities in AI-assisted coding
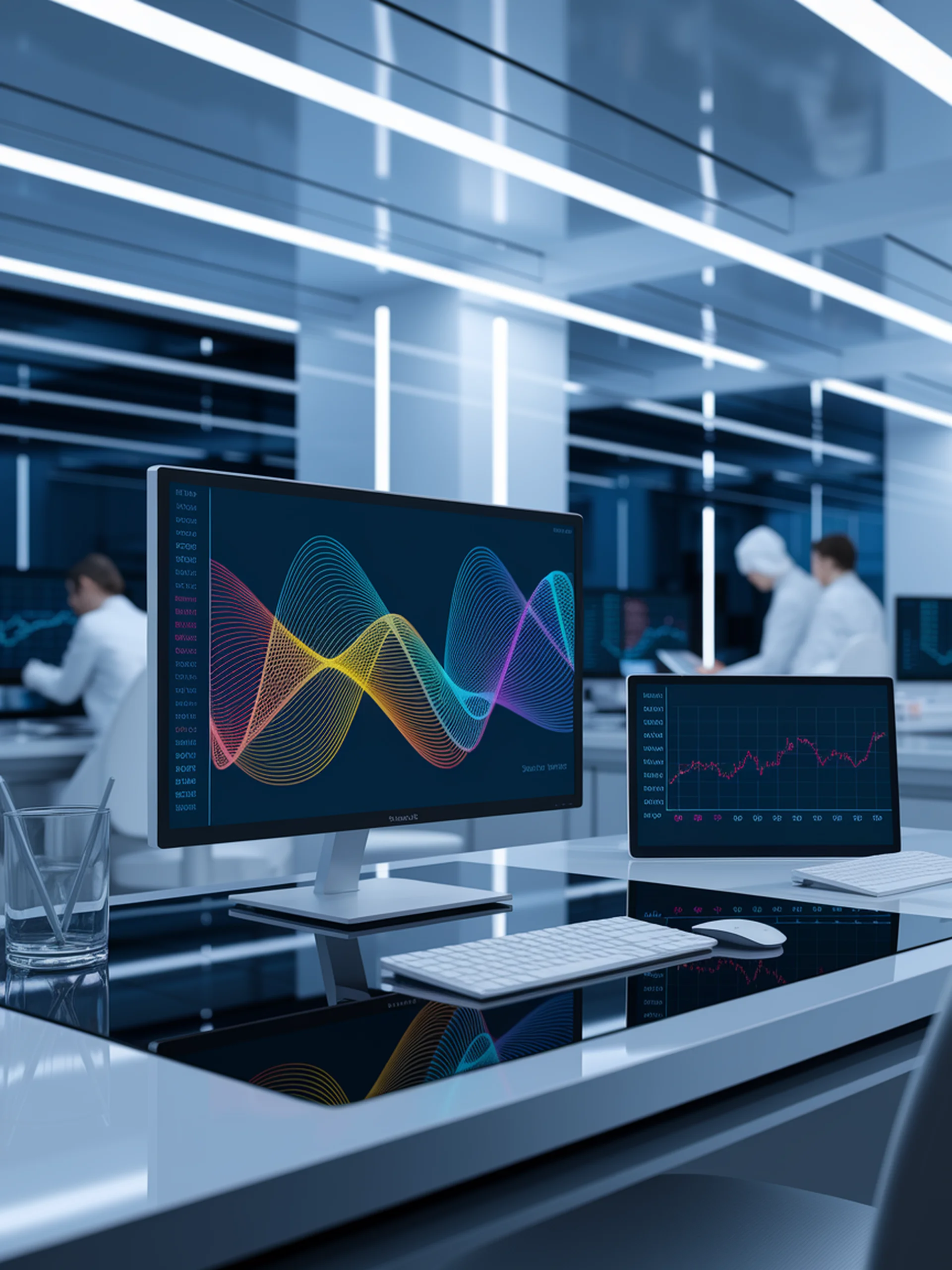
Detecting LLM Hallucinations Through Logit Analysis
A novel approach to measuring AI uncertainty and improving reliability
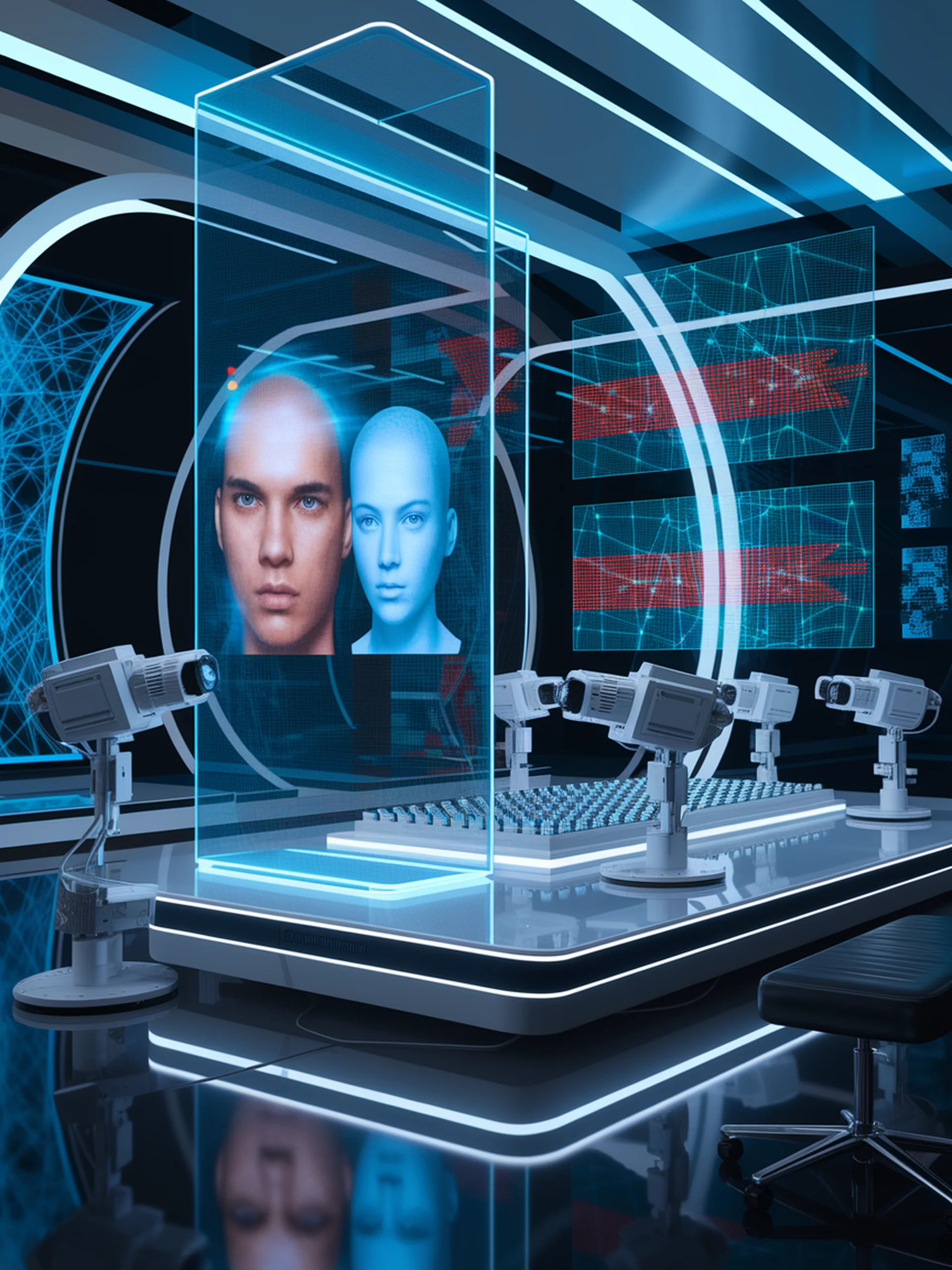
Reducing Hallucinations in Vision-Language Models
A novel token reduction approach for more reliable AI vision systems
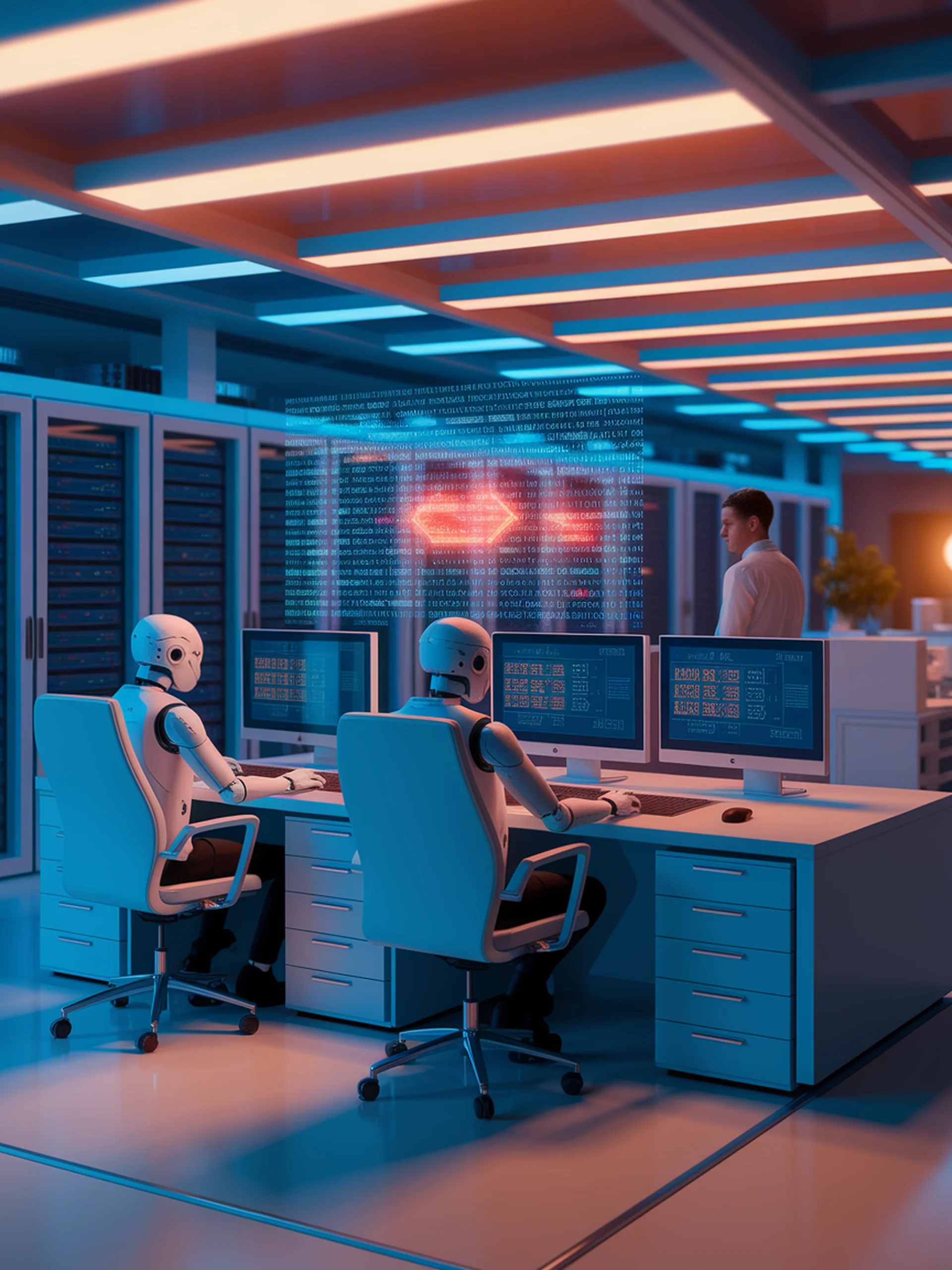
Combating AI Hallucinations
A Zero-Resource Framework for Detecting False Information in LLMs
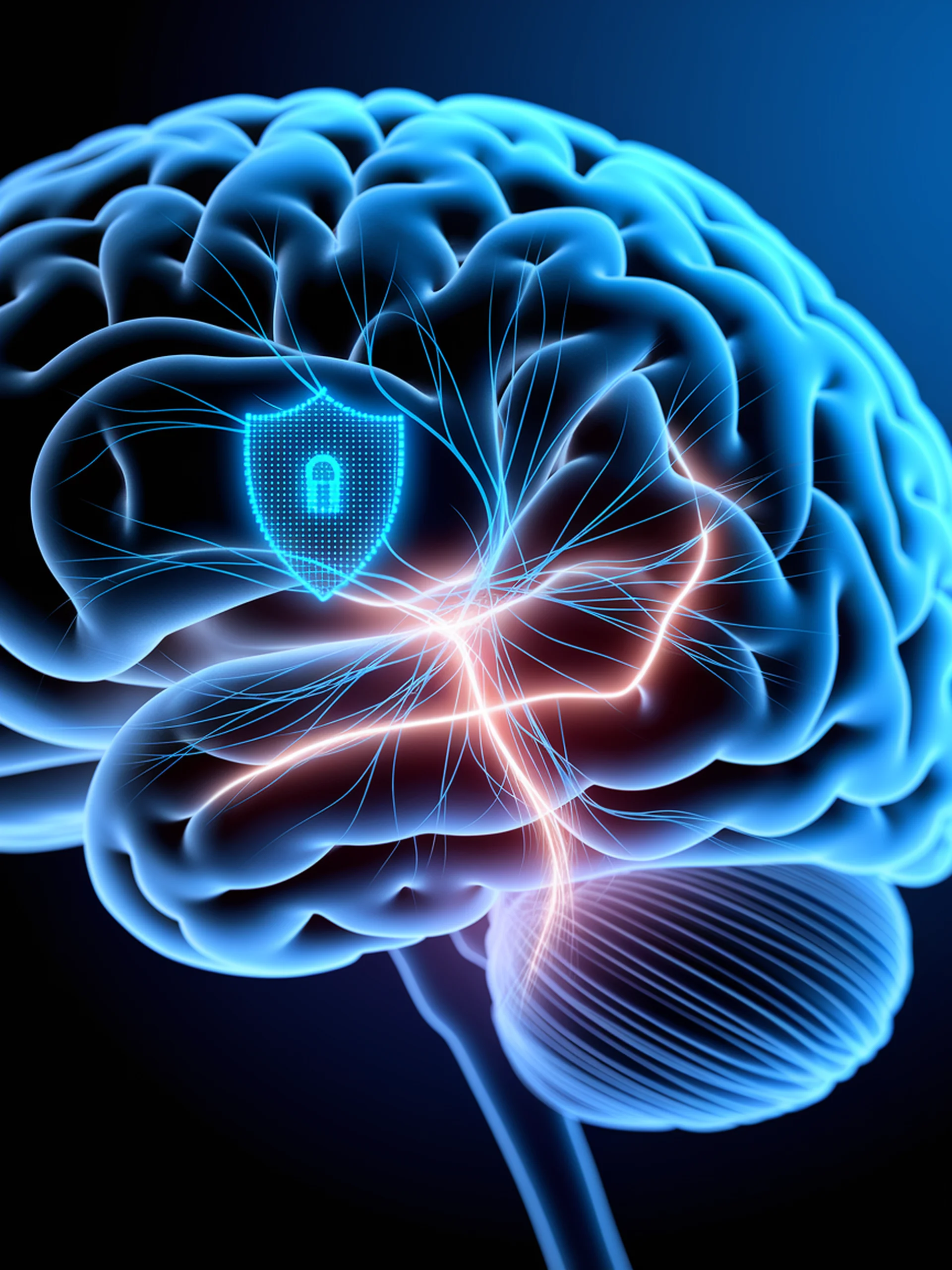
Uncovering LLM's Hidden Knowledge
A New Method for Detecting and Steering Concepts in Large Language Models
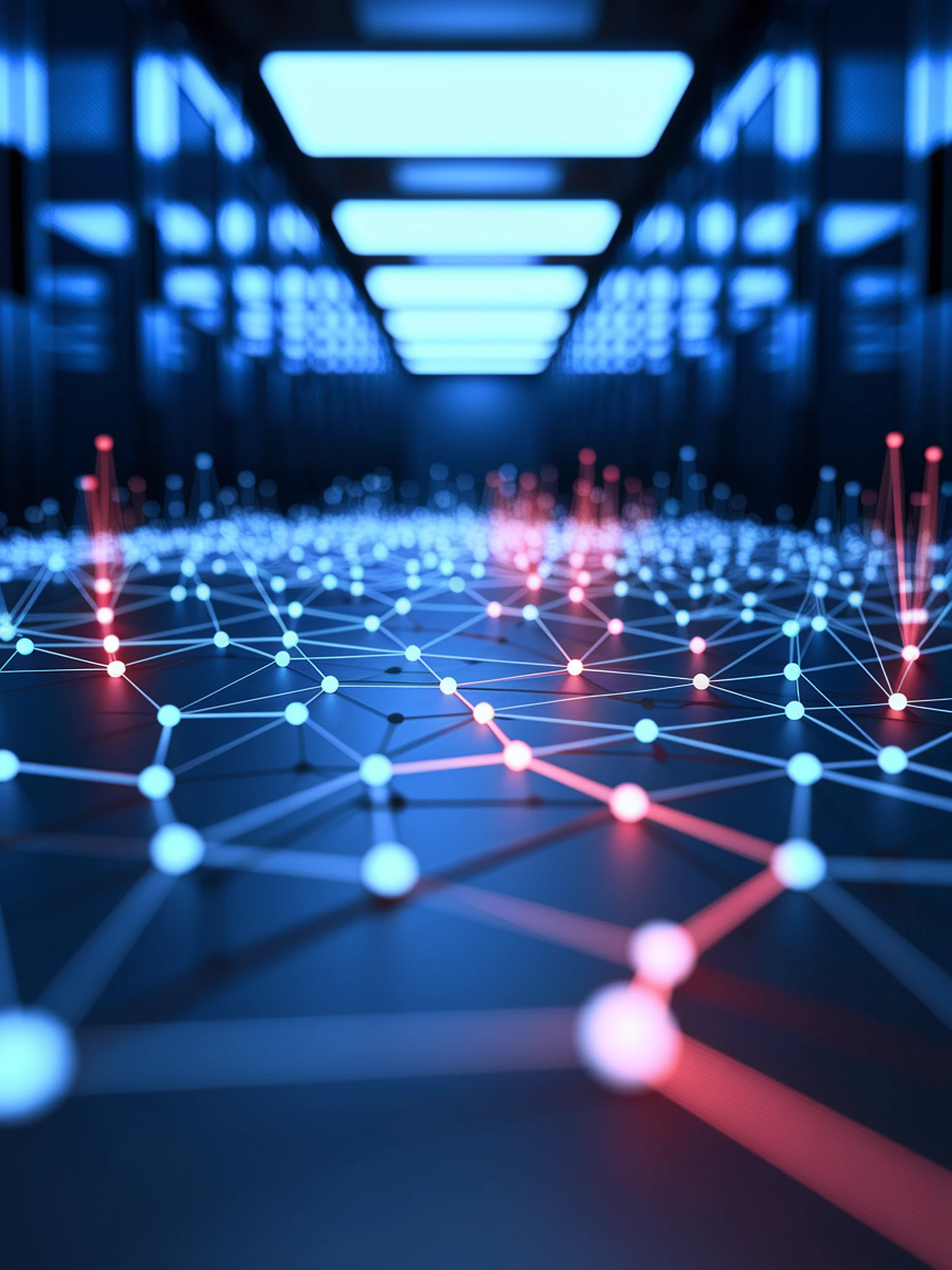
Detecting LLM Hallucinations with Noise
Improving detection accuracy through strategic noise injection
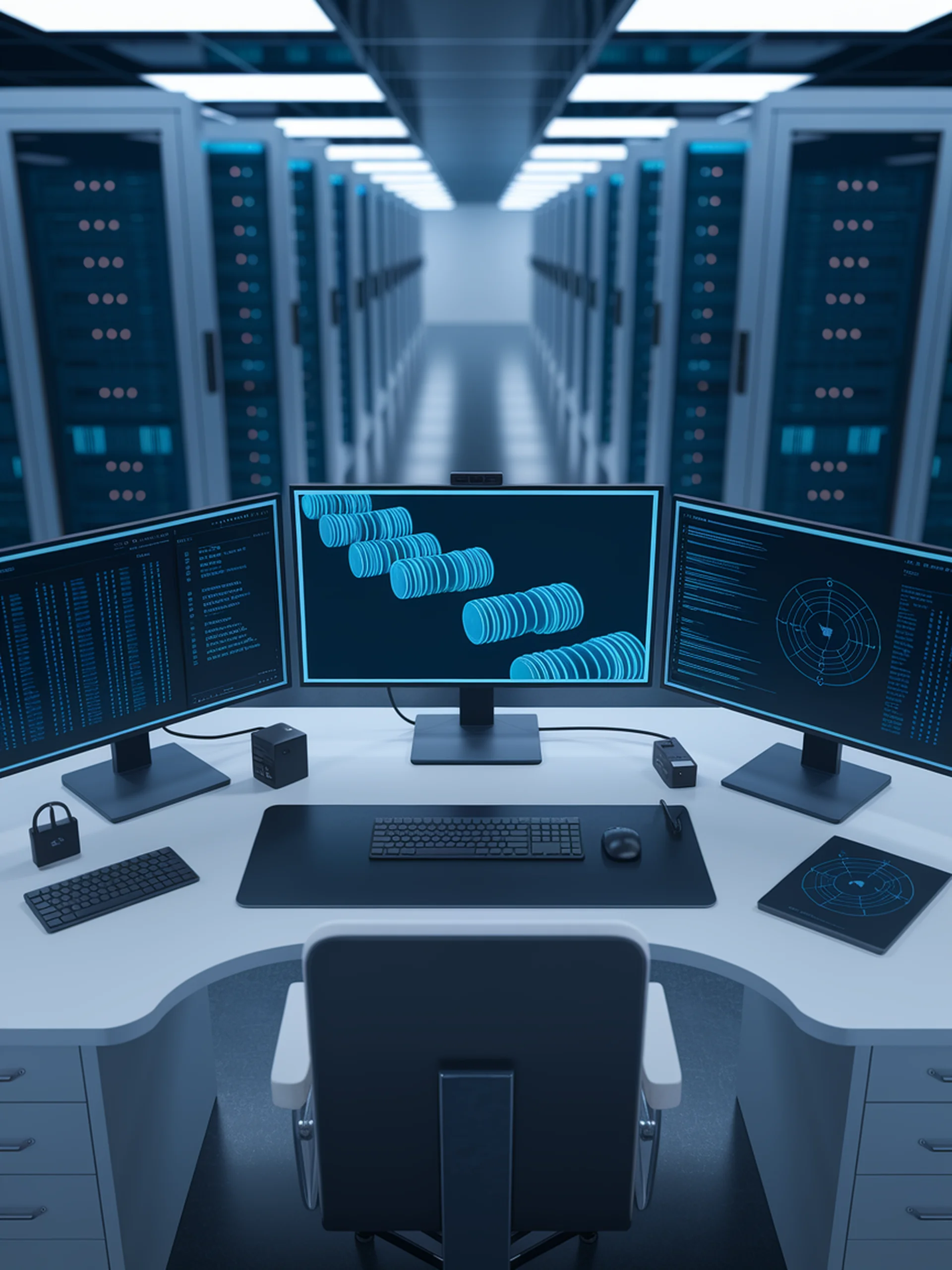
TruthFlow: Enhancing LLM Truthfulness
Query-specific representation correction for more reliable AI outputs
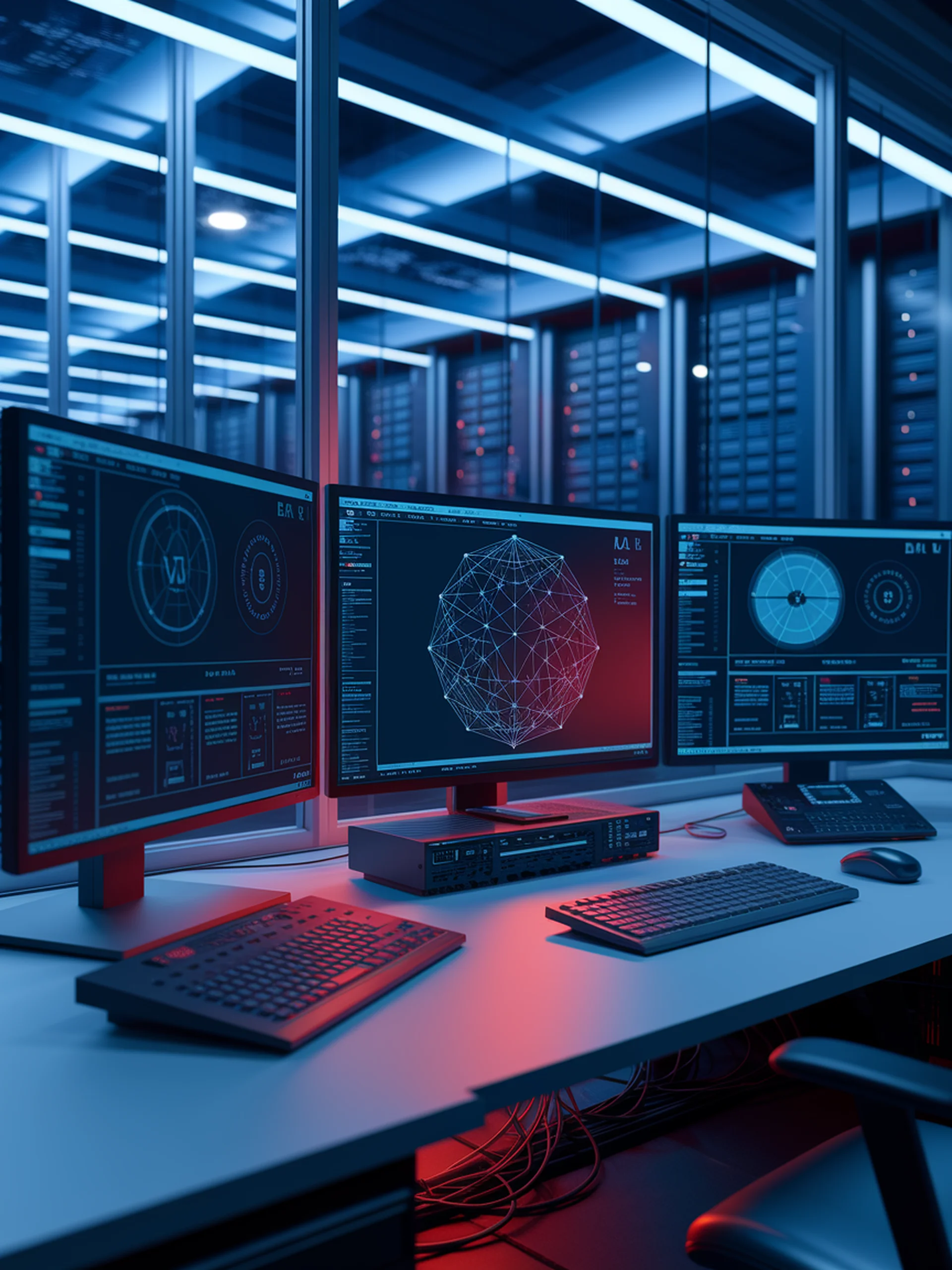
Securing the AI Giants
A Comprehensive Framework for Large Model Safety
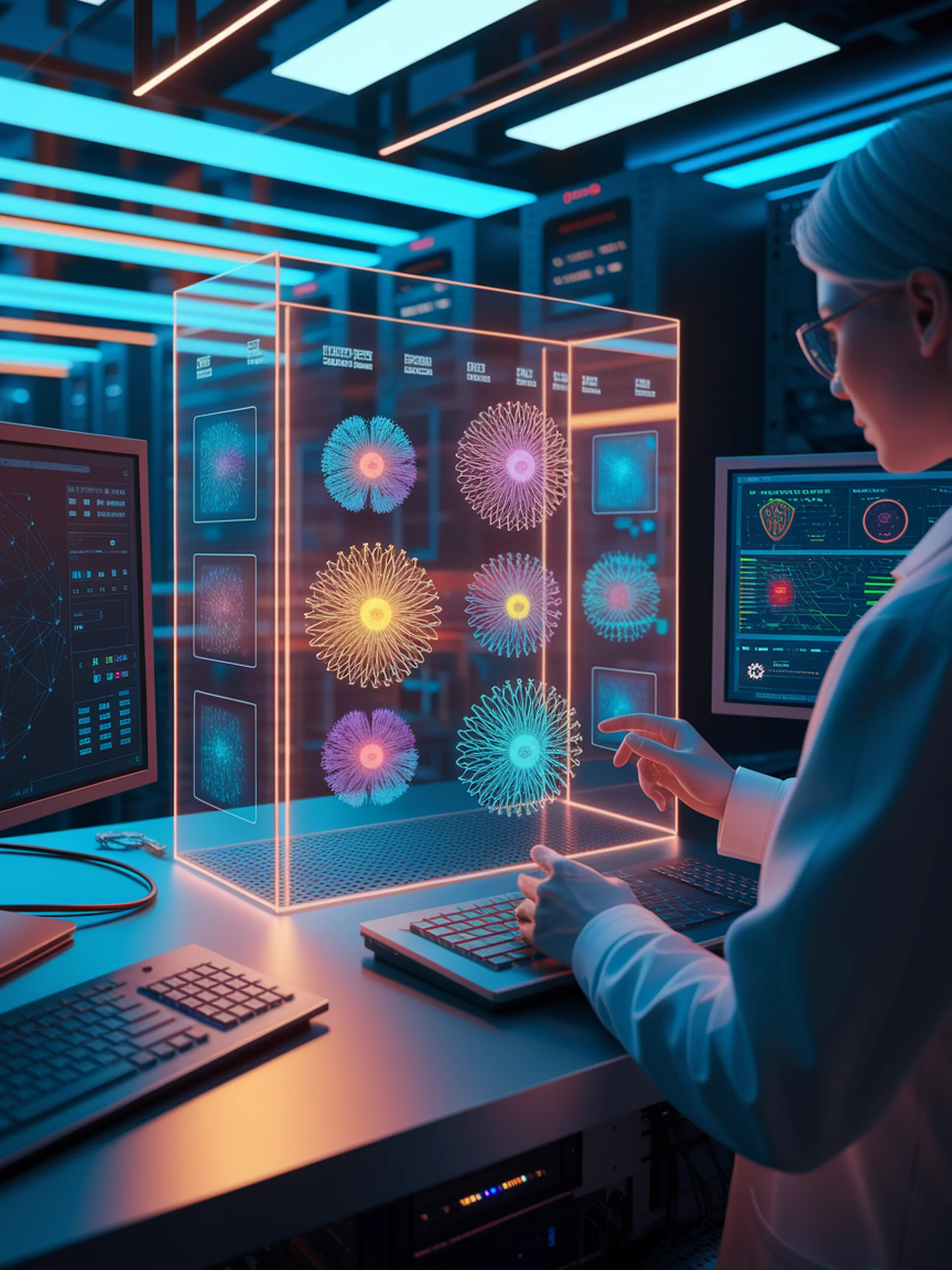
Making LLMs Explain Themselves
Enhancing model explainability without external modules
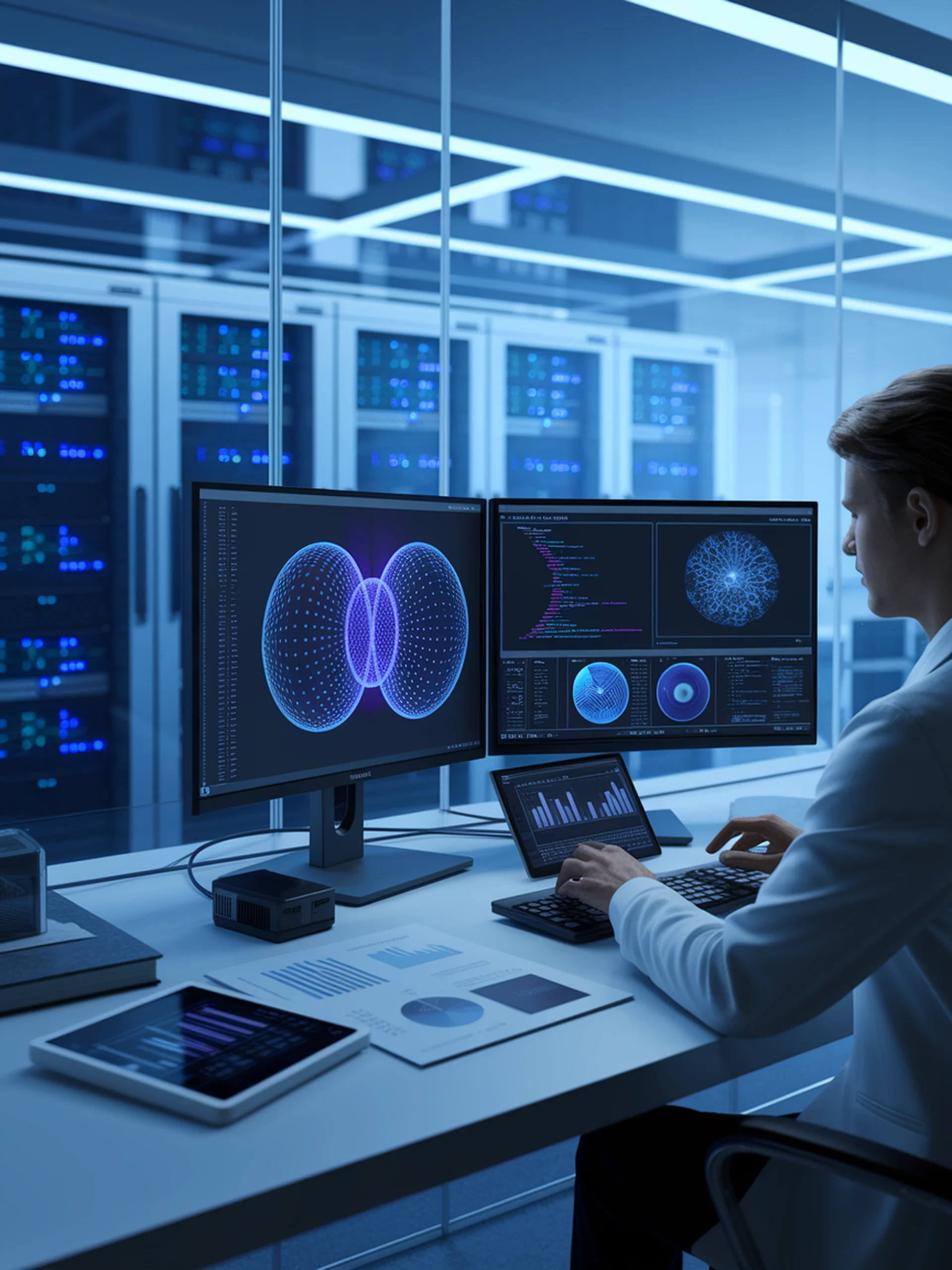
Combating Hallucinations in LLMs
Delta: A Novel Contrastive Decoding Approach
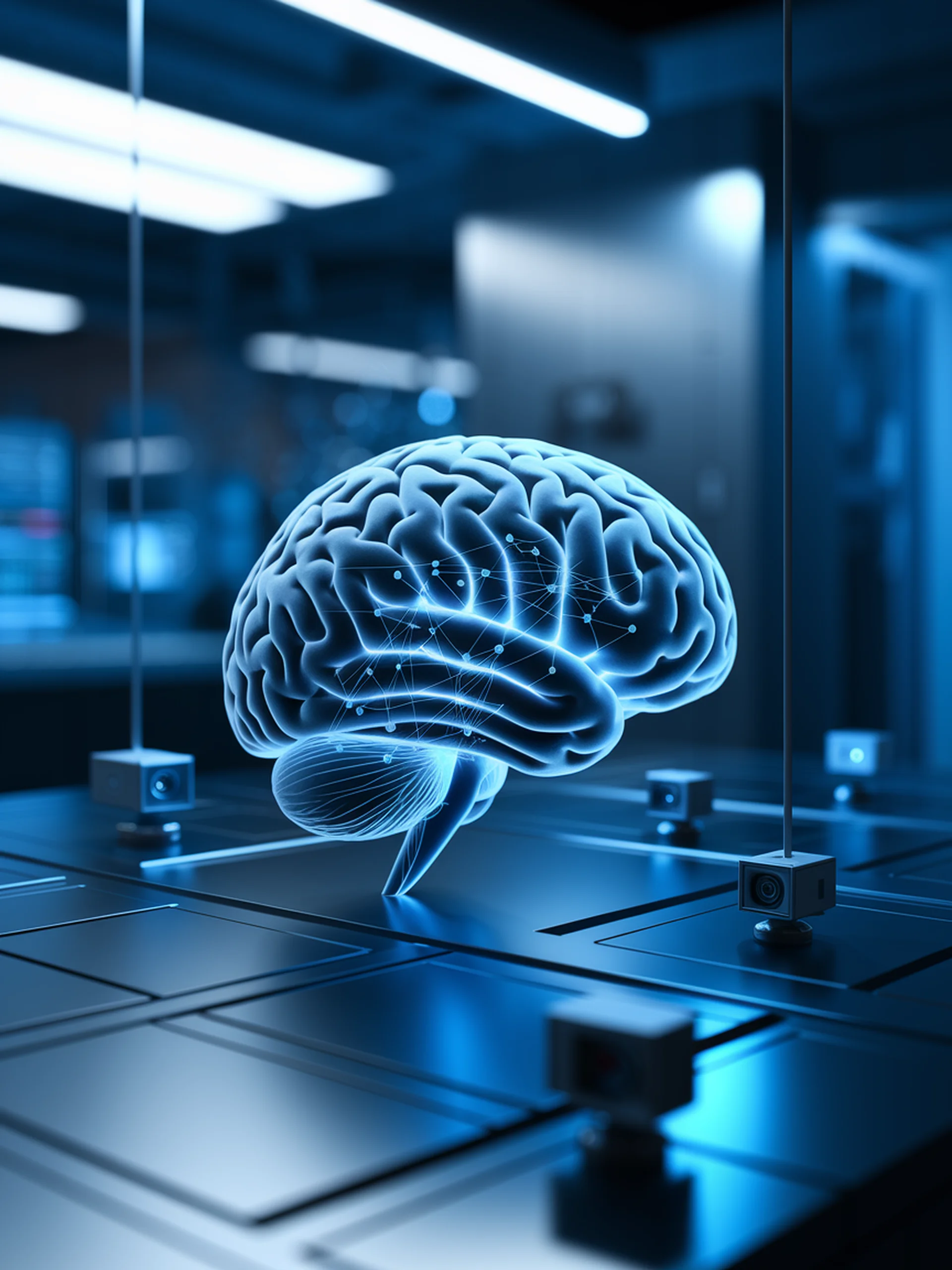
Mind Reading Machines: The Security Frontier
Evaluating Theory of Mind in Large Language Models and its Safety Implications
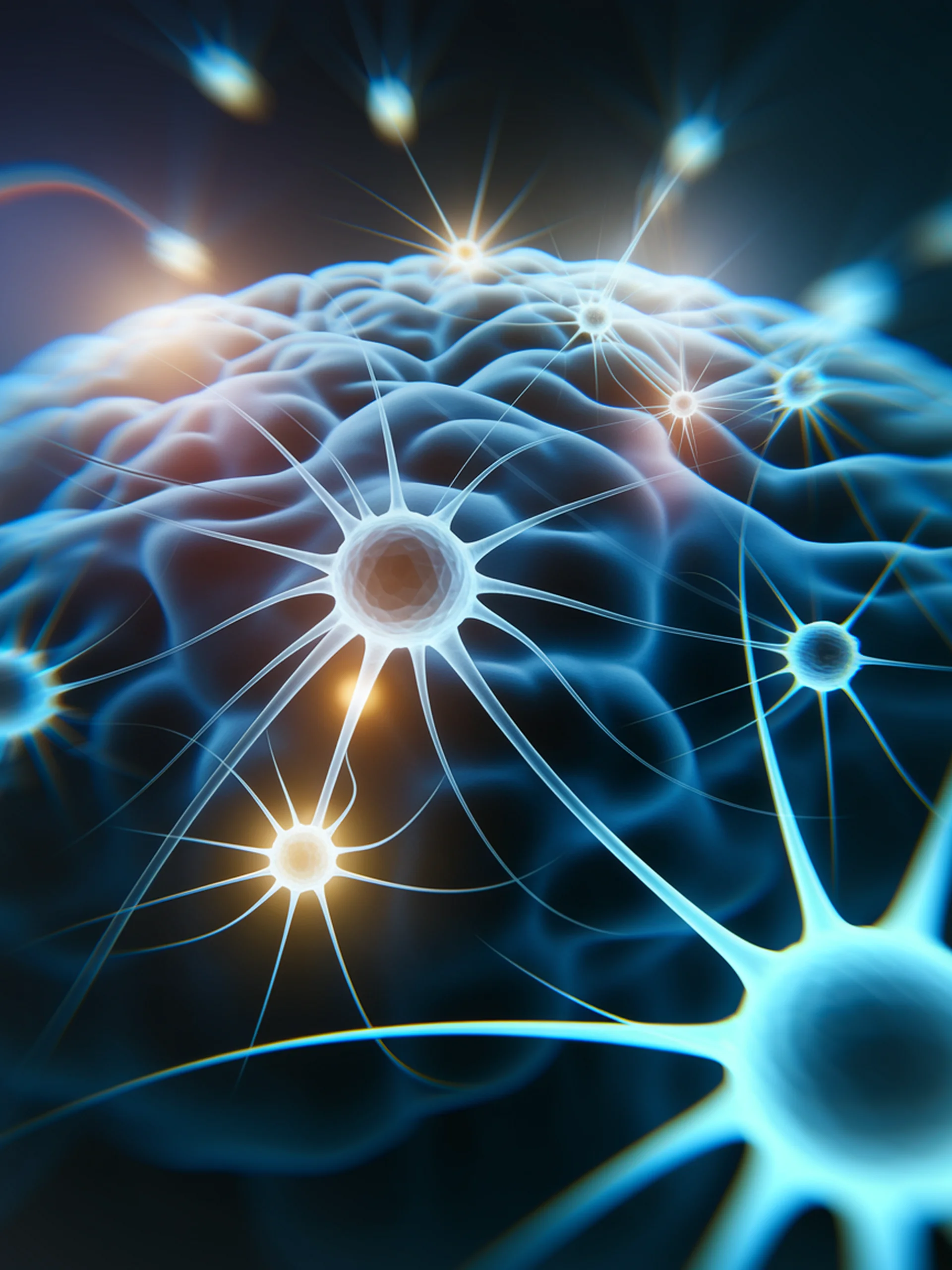
Beyond Single Neurons: The Range Attribution Approach
A more accurate framework for understanding and controlling LLM behavior
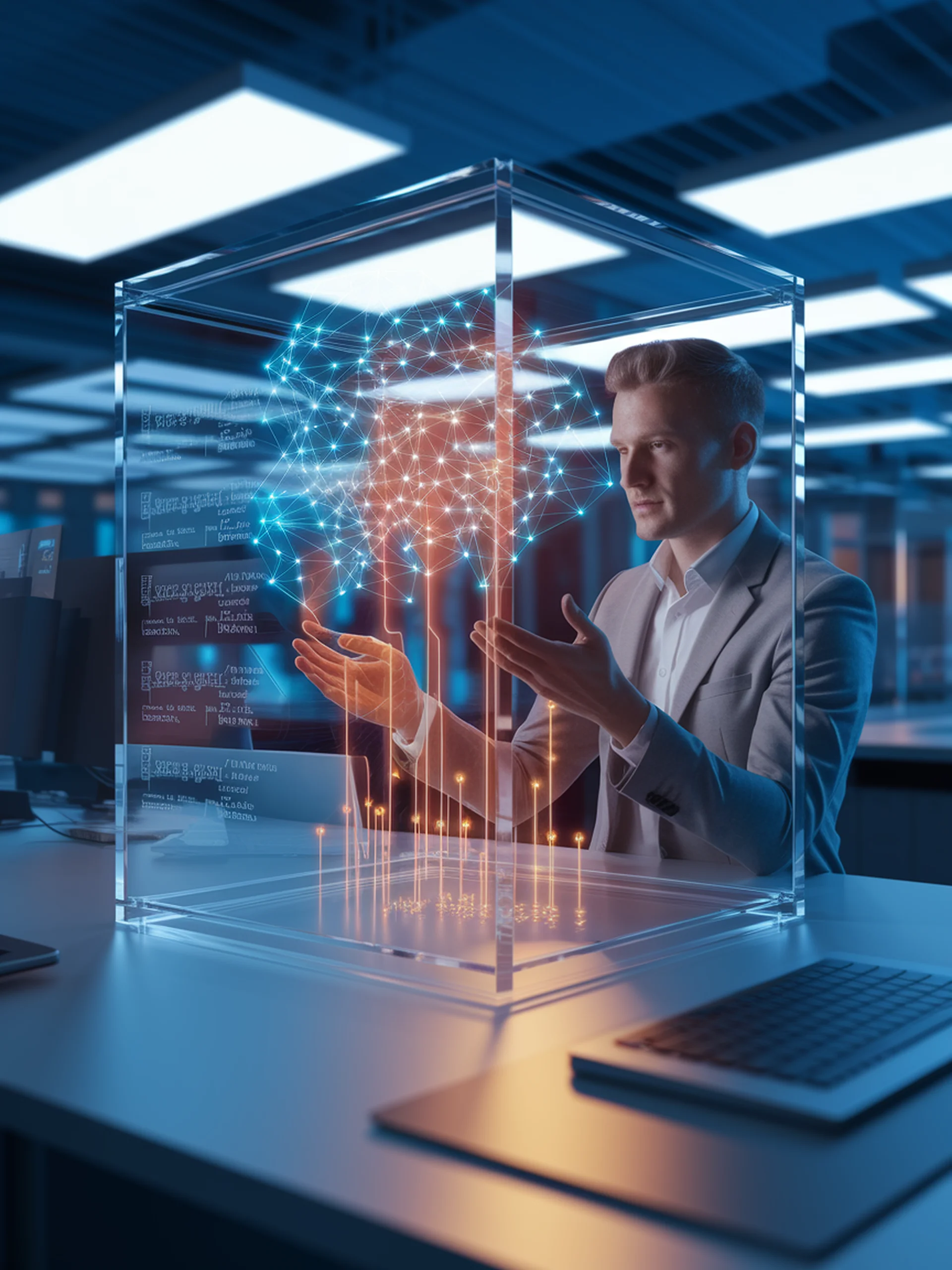
Making AI Decision-Making Transparent
Bringing Explainability to Deep Reinforcement Learning
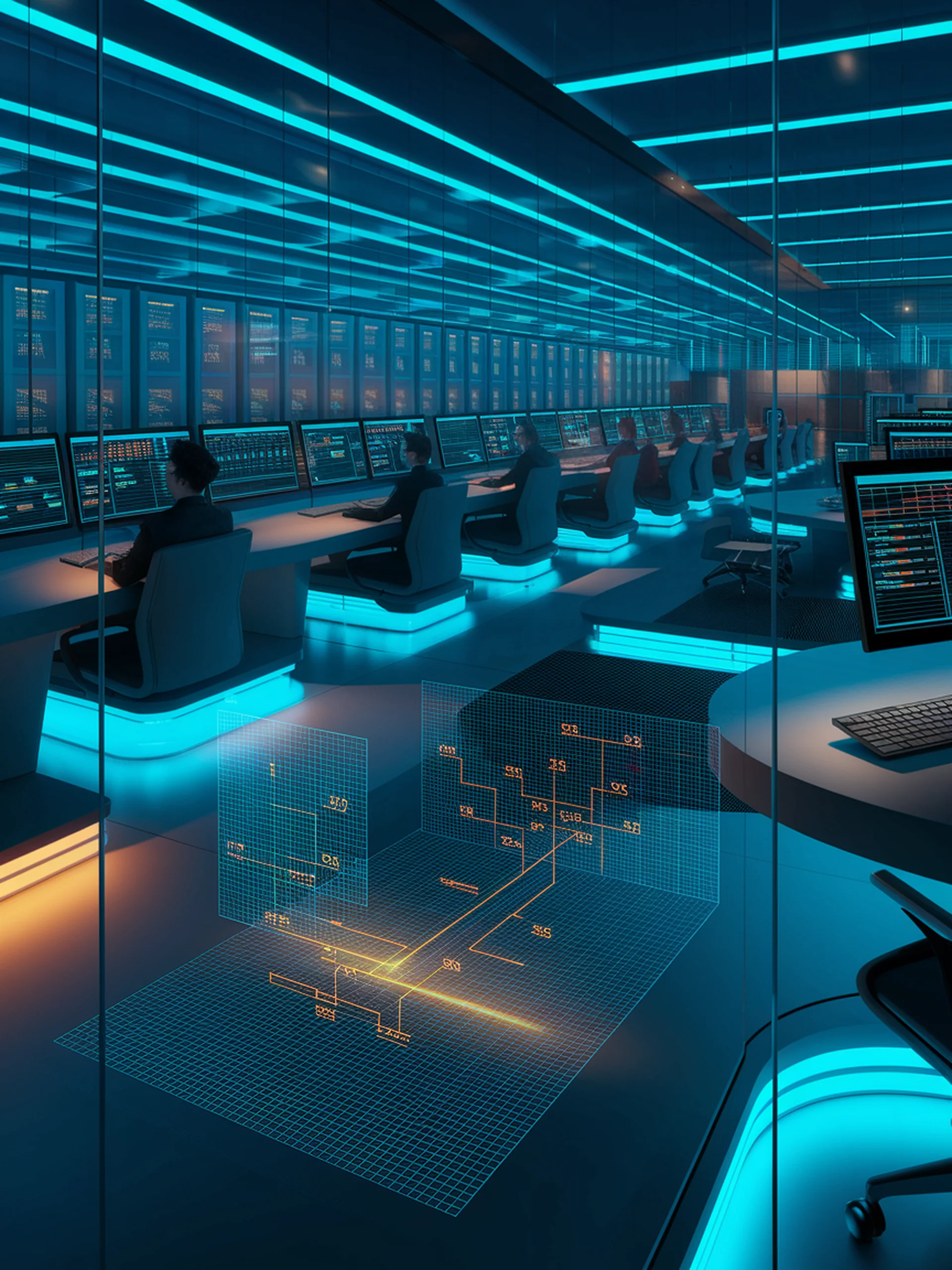
Adaptive Risk Management in AI Systems
A novel approach to managing uncertainty in language models
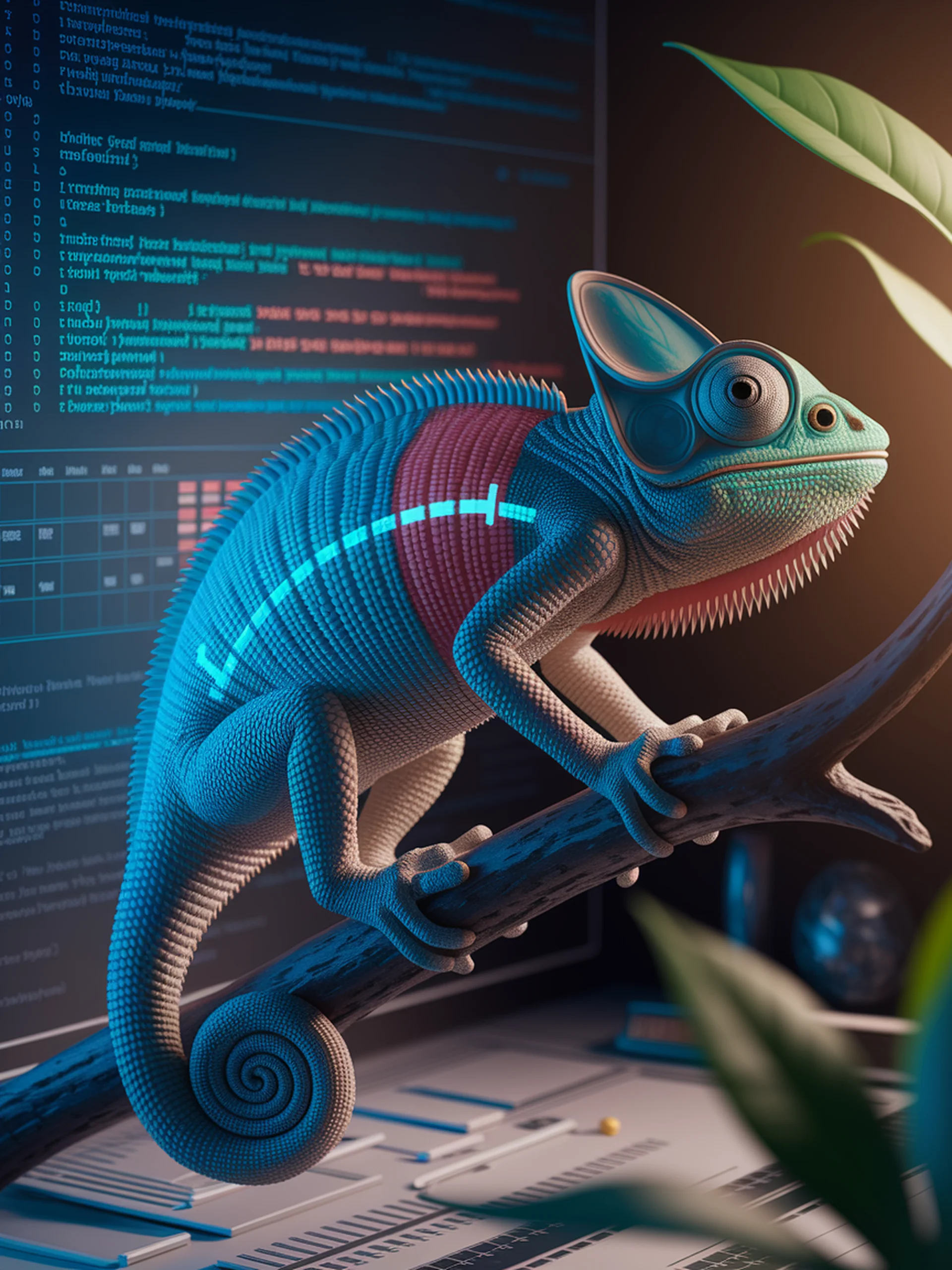
The Chameleon Effect in LLMs
Detecting artificial benchmark performance vs. true language understanding
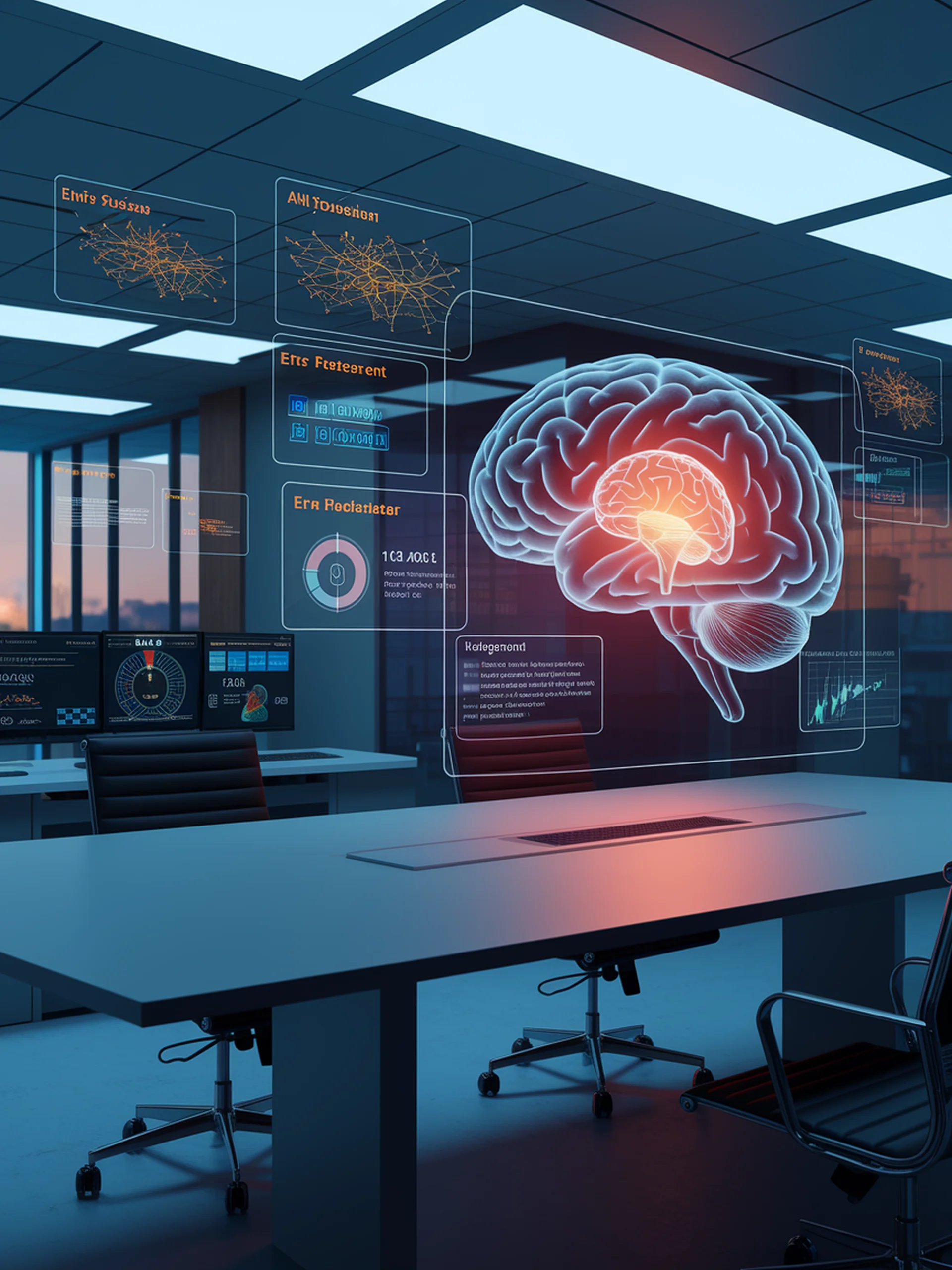
Making AI More Trustworthy
A Novel Framework for Detecting and Explaining LLM Hallucinations
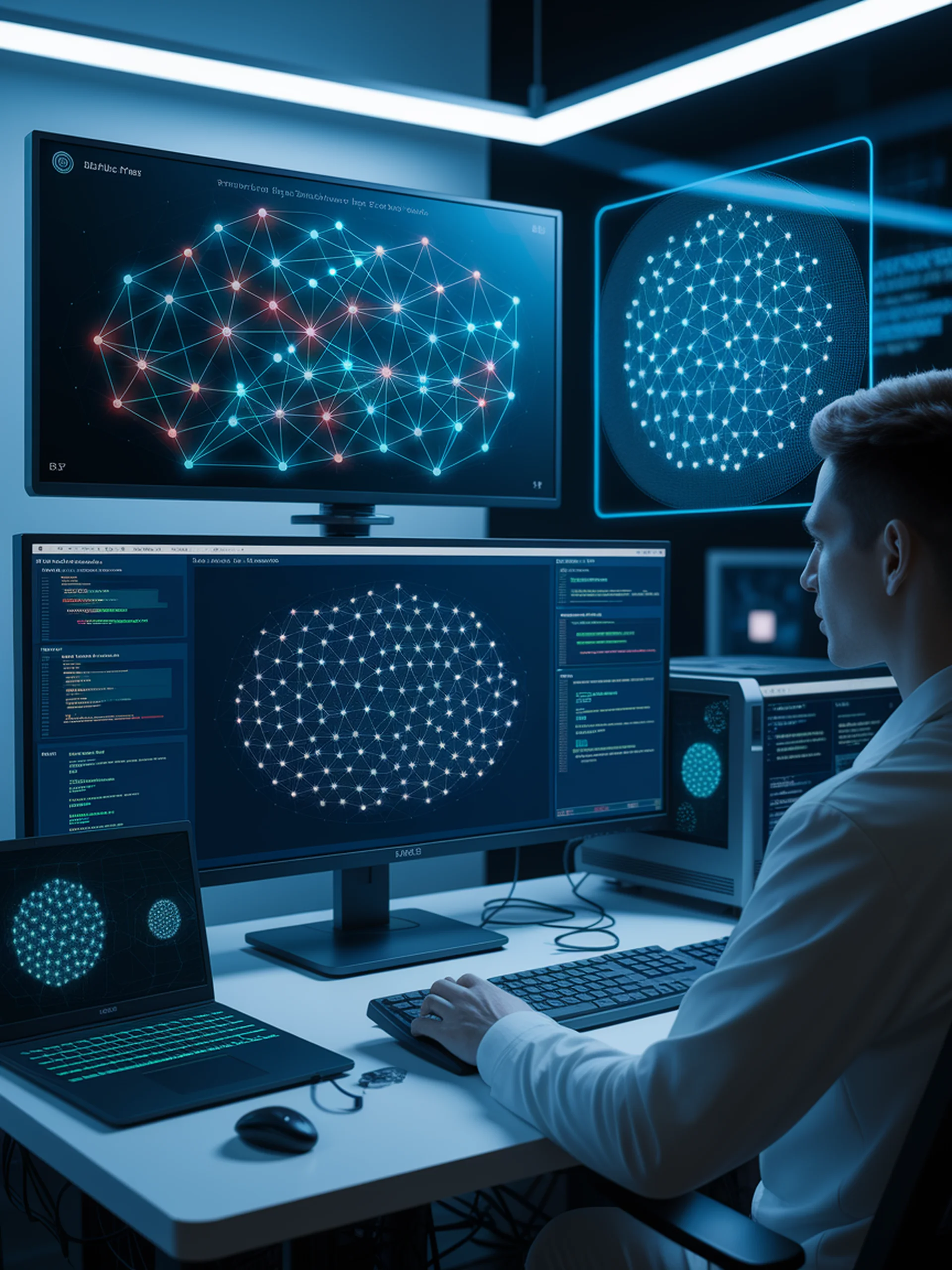
Enhancing GNN Trustworthiness with LLMs
A systematic approach to more reliable graph-based AI
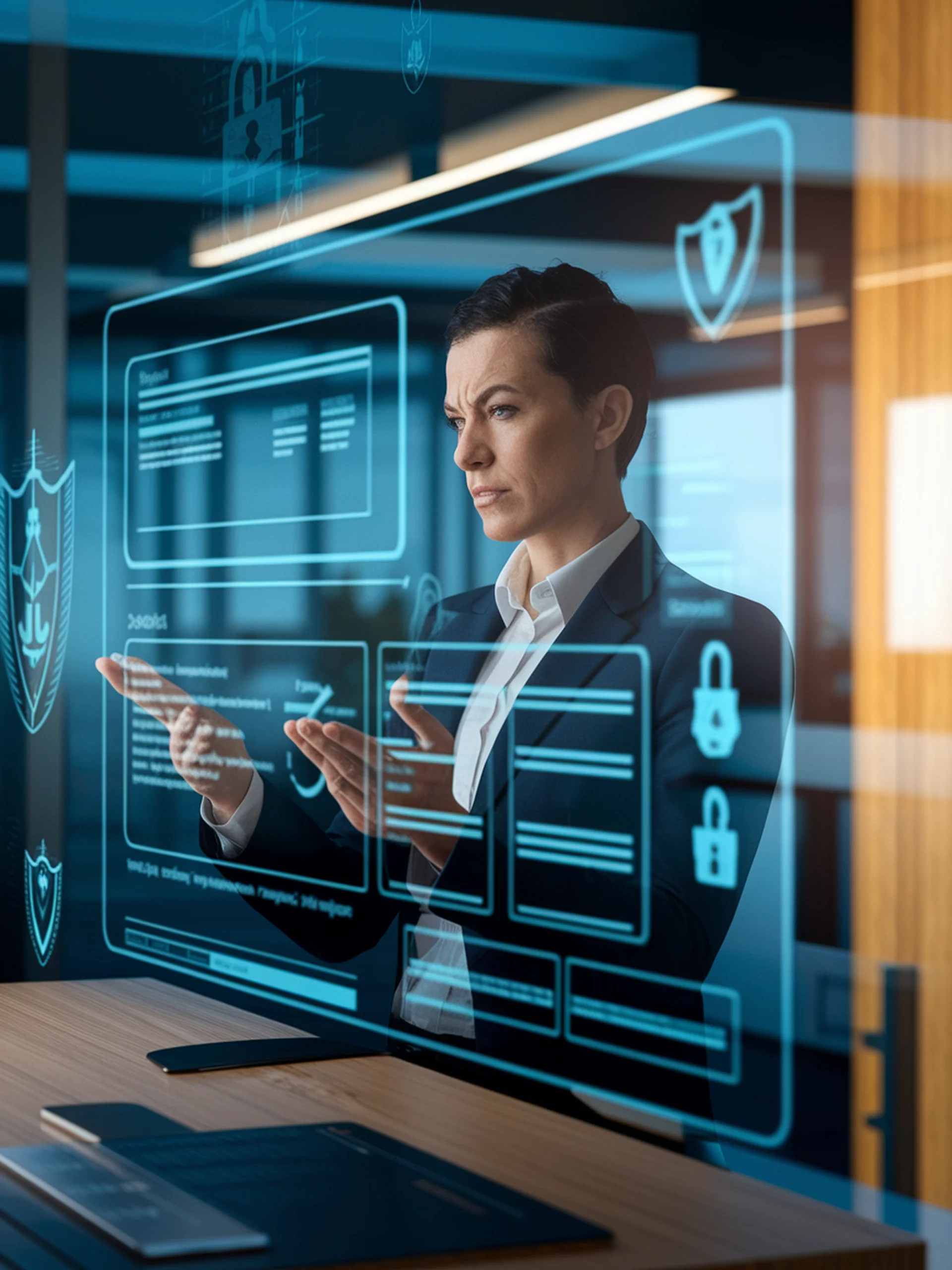
Combating LLM Overreliance
How explanations, sources, and inconsistencies influence user trust
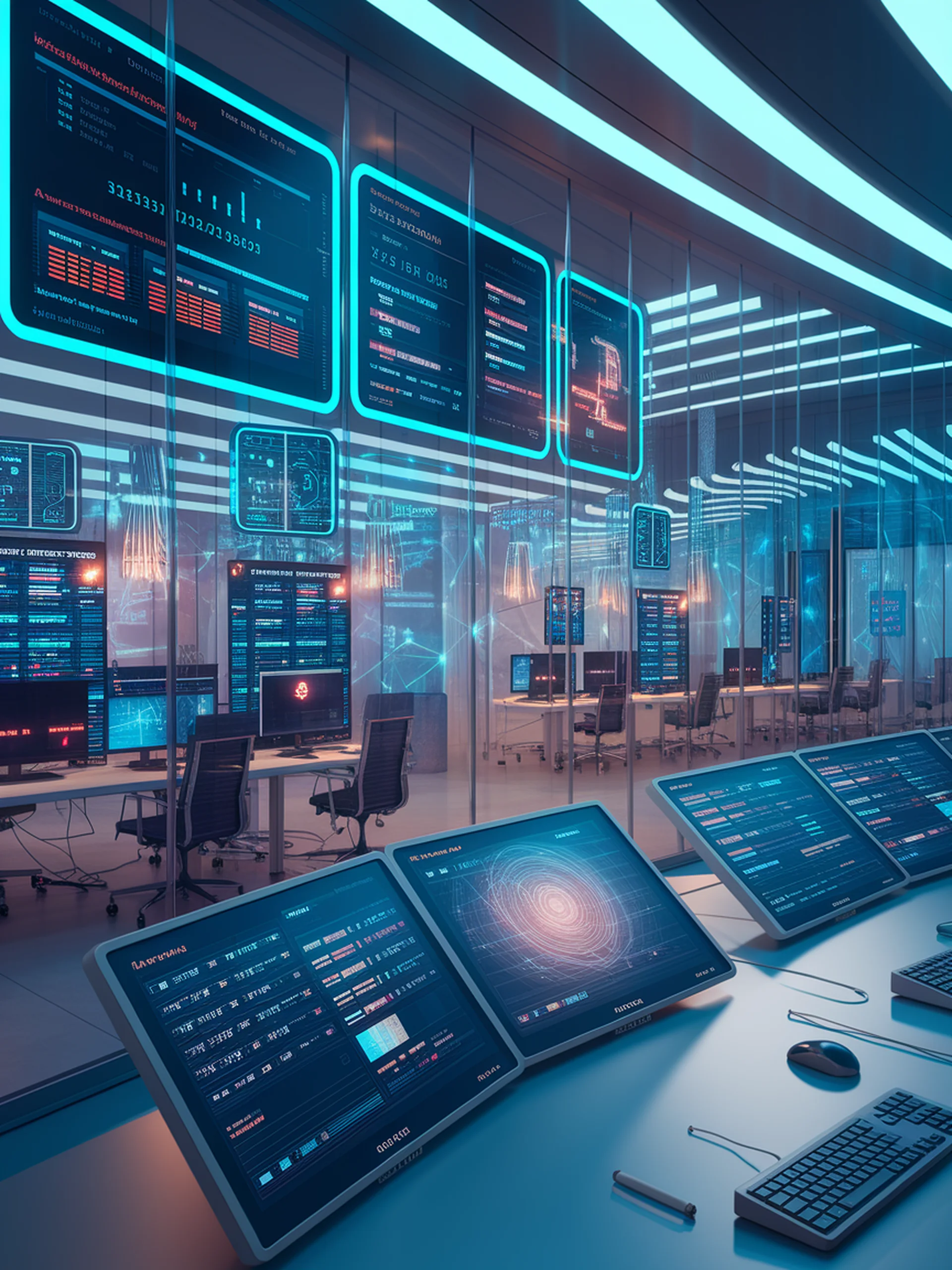
Automating Fact-Checking with AI
Using LLMs to combat misinformation at scale
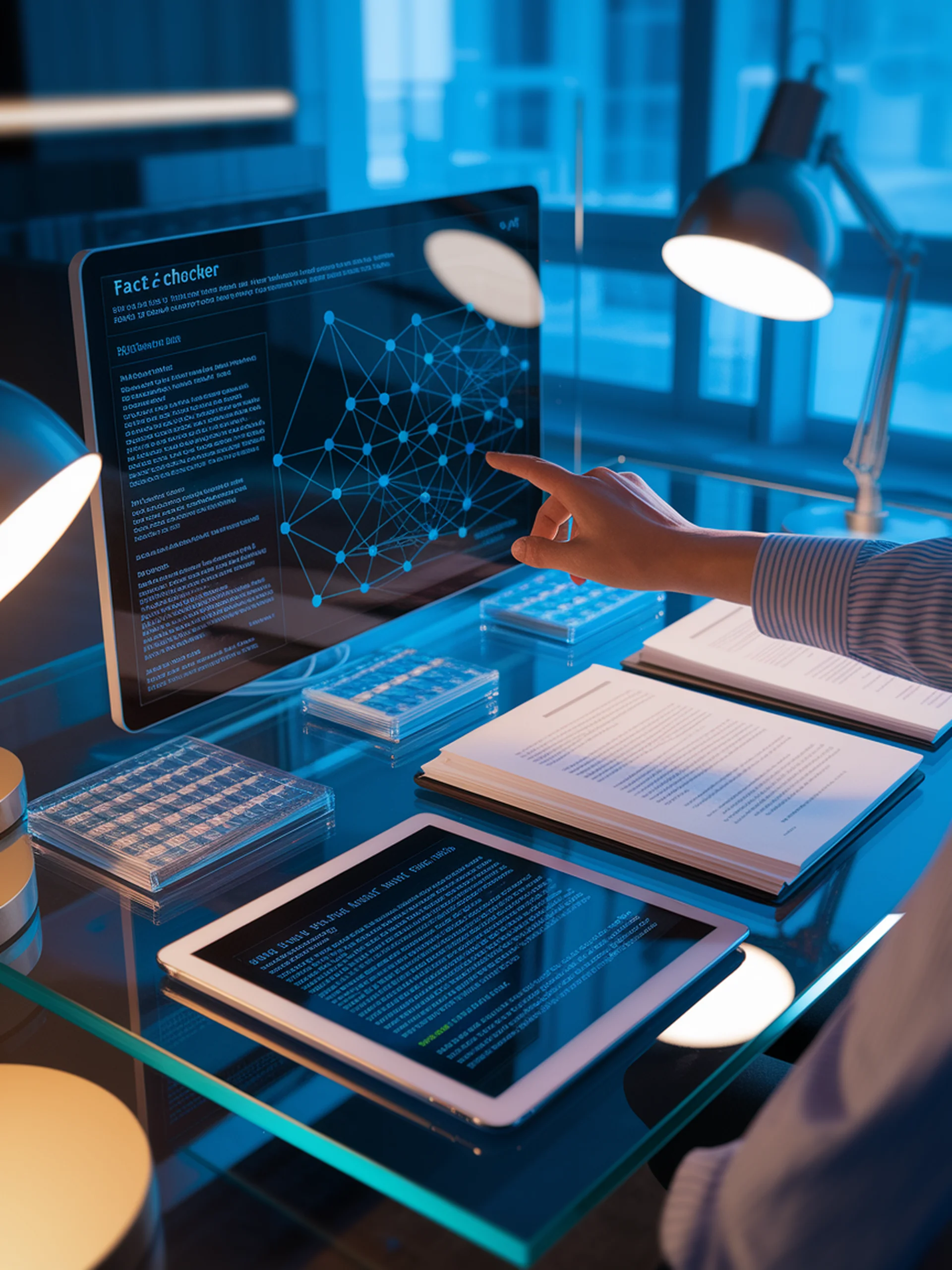
Explainable AI for Fact-Checking
Bridging the gap between AI systems and human fact-checkers
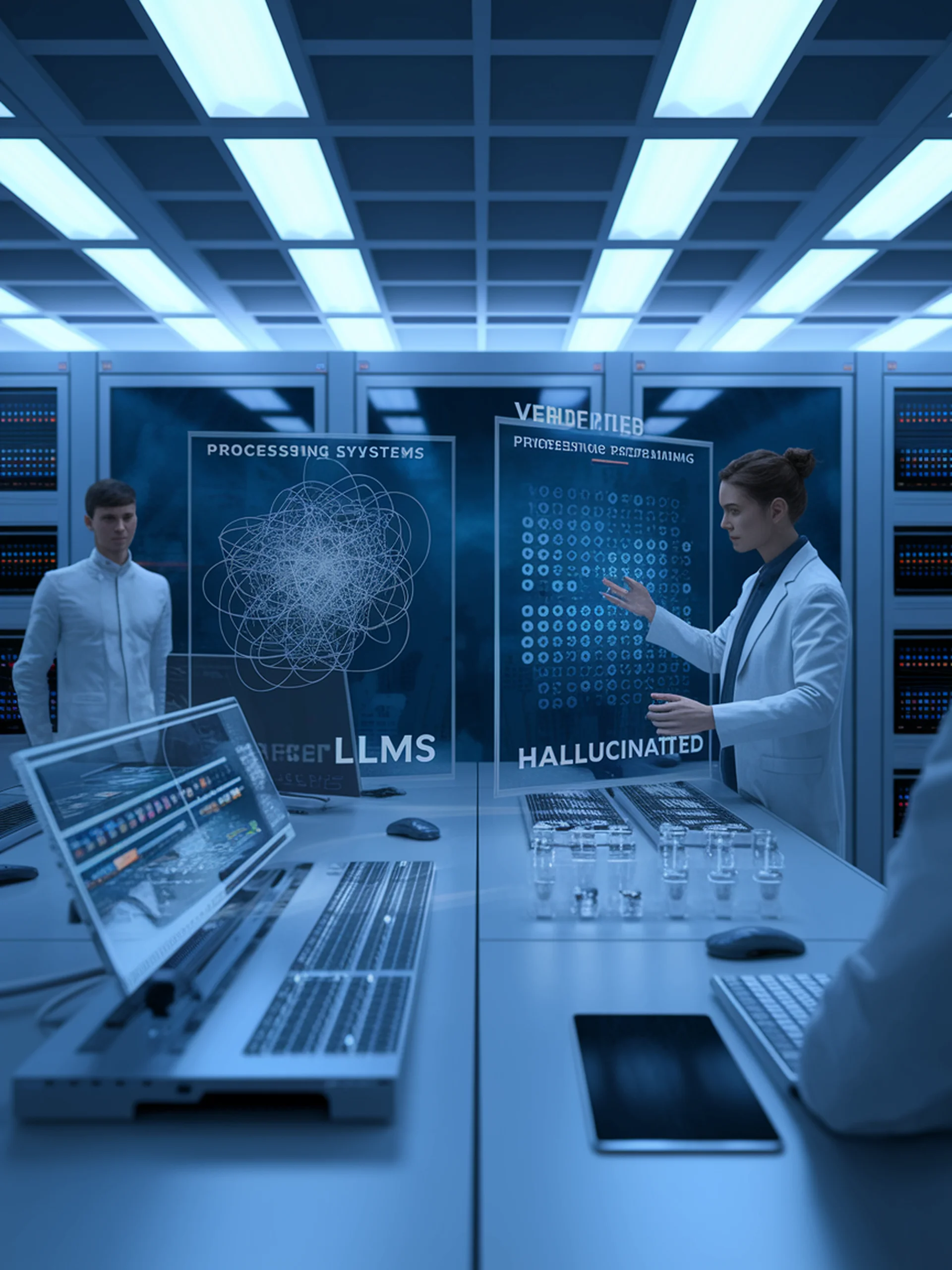
Eliminating AI Hallucinations
Combining Logic Programming with LLMs for Reliable Answers
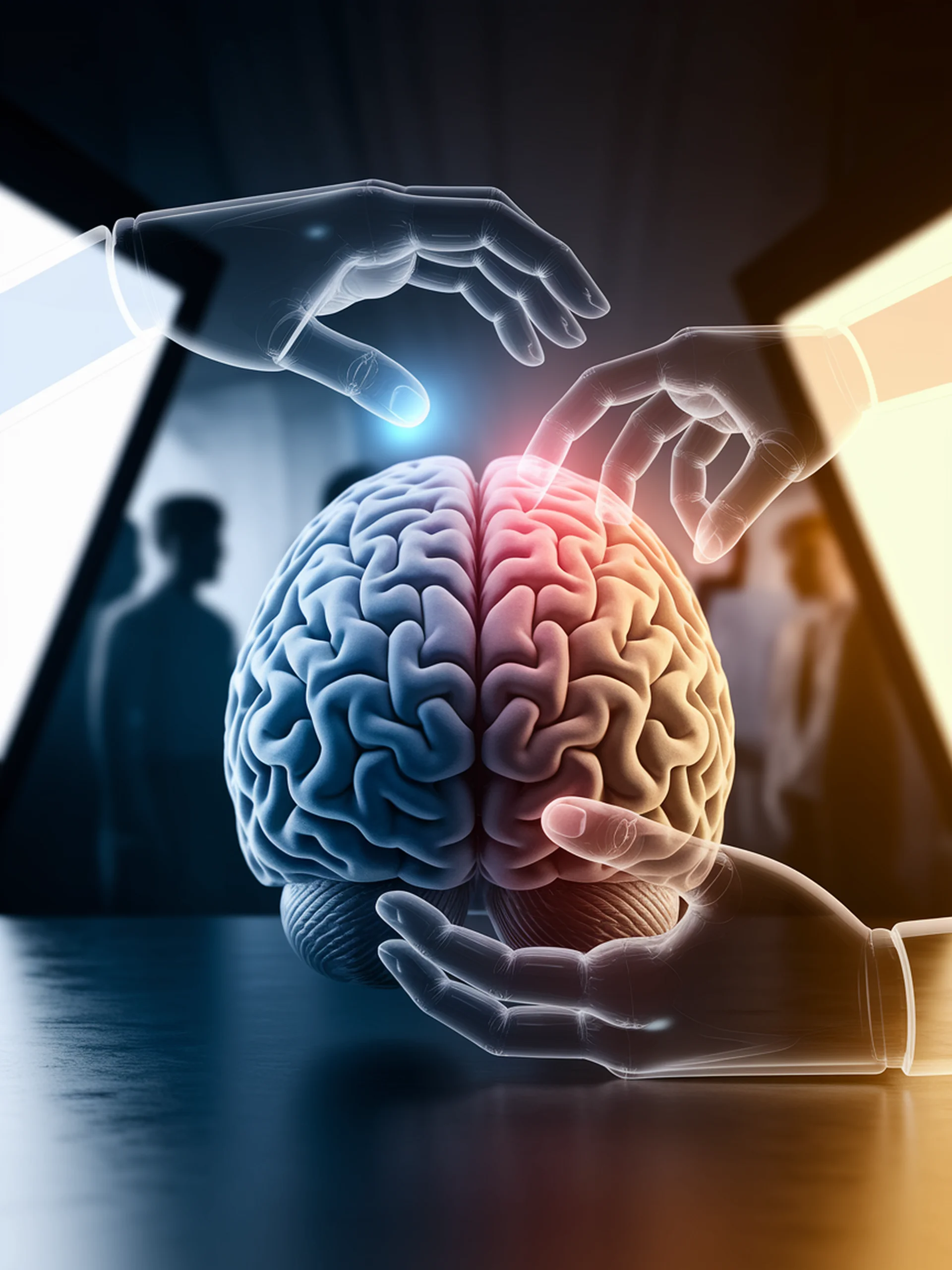
The Persuasion Tactics of AI
How LLMs emotionally and rationally influence users
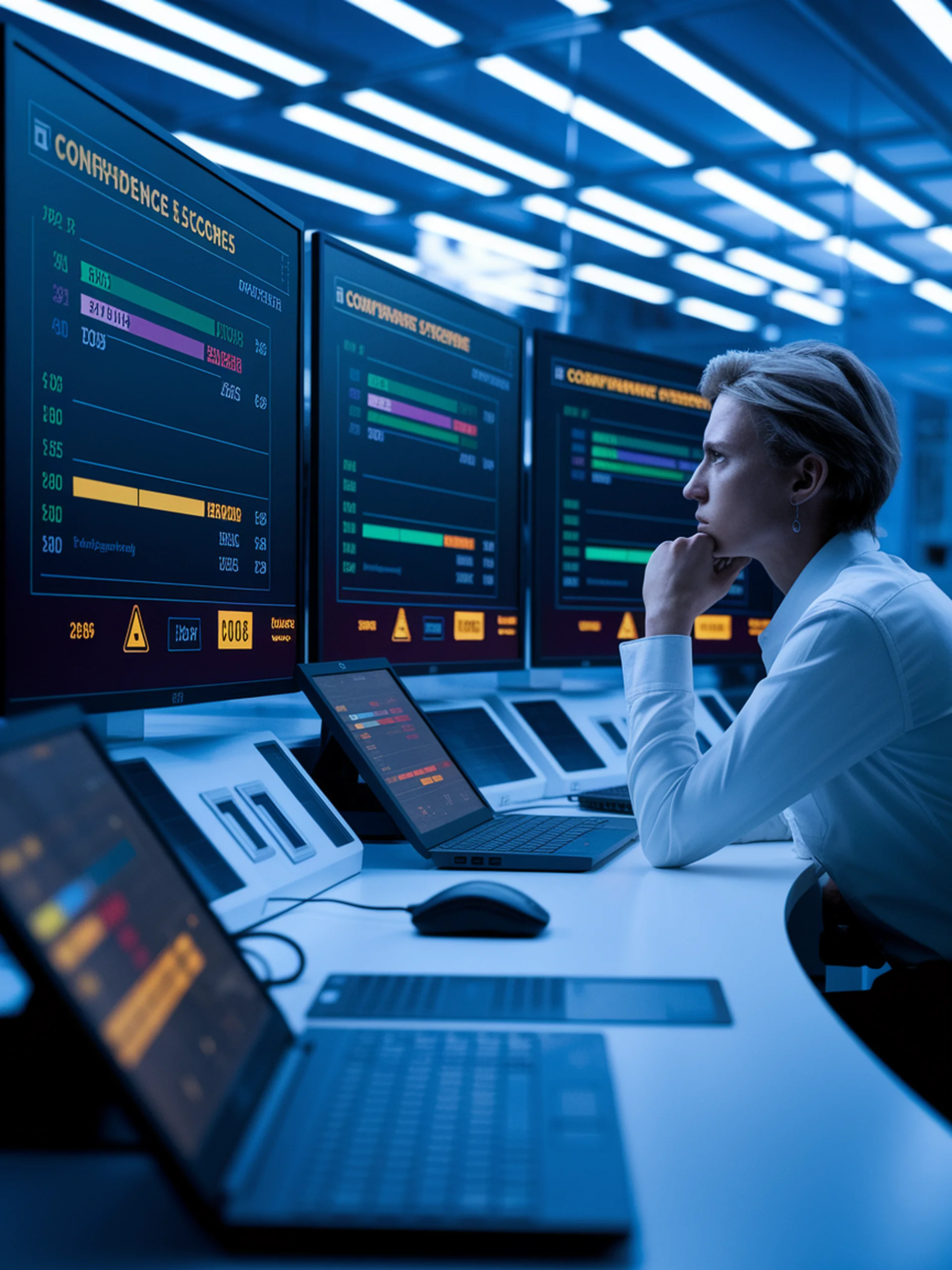
The Confidence Dilemma in AI
Measuring and mitigating overconfidence in Large Language Models
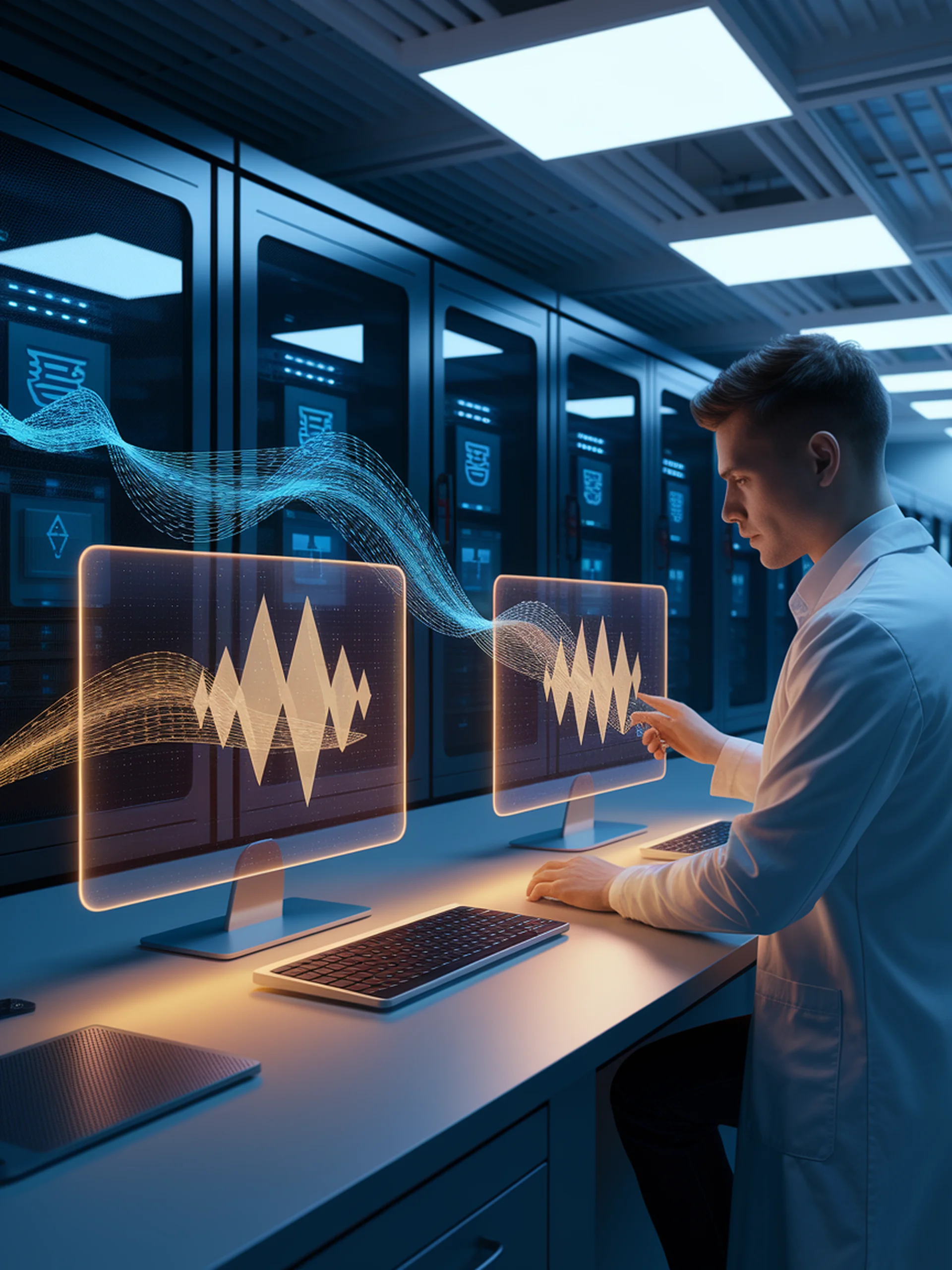
Combating LLM Hallucinations
Using Smoothed Knowledge Distillation to improve factual reliability
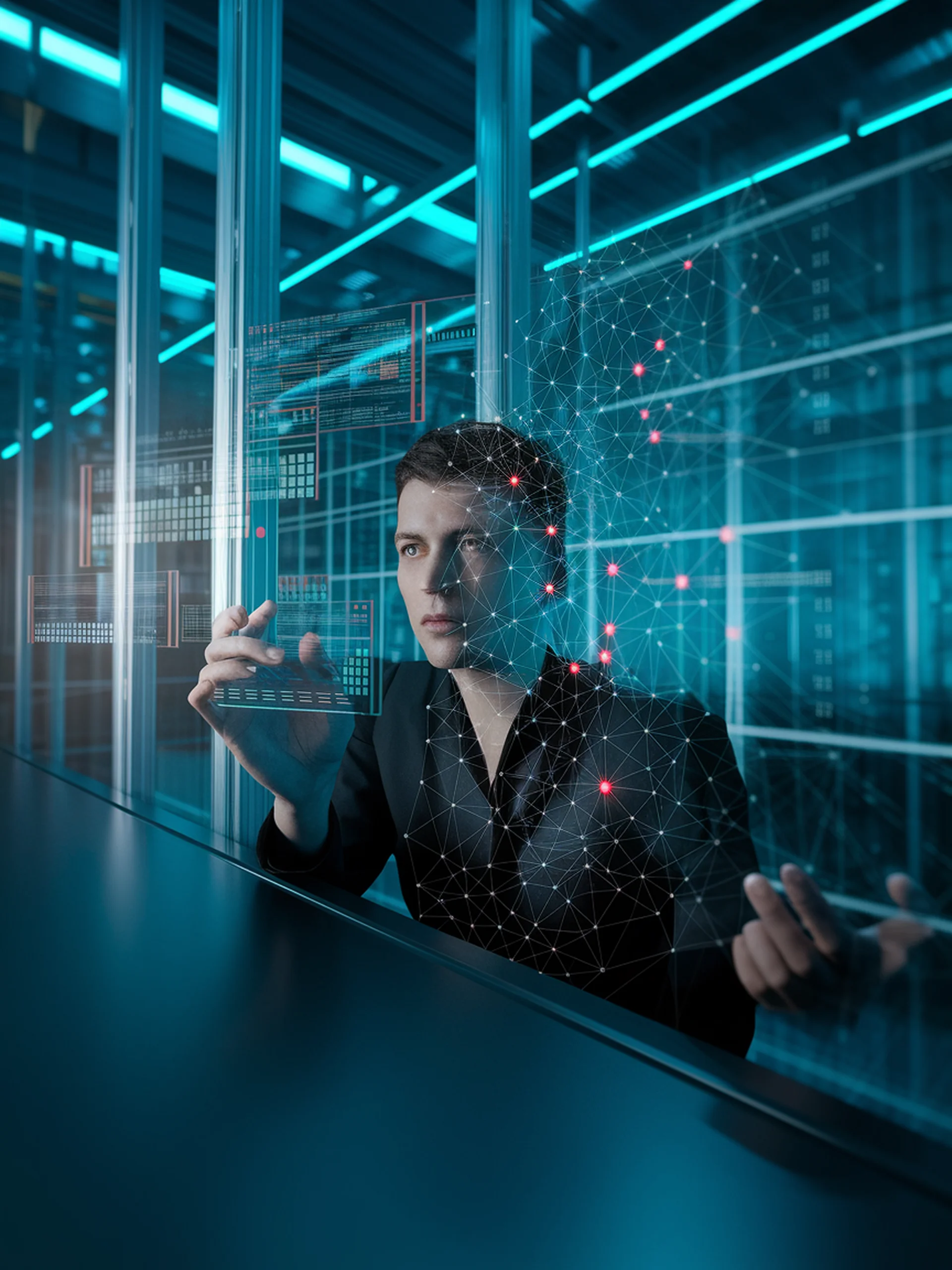
Enhancing LLM Security Through Knowledge Boundary Perception
Using internal states to prevent confident yet incorrect responses
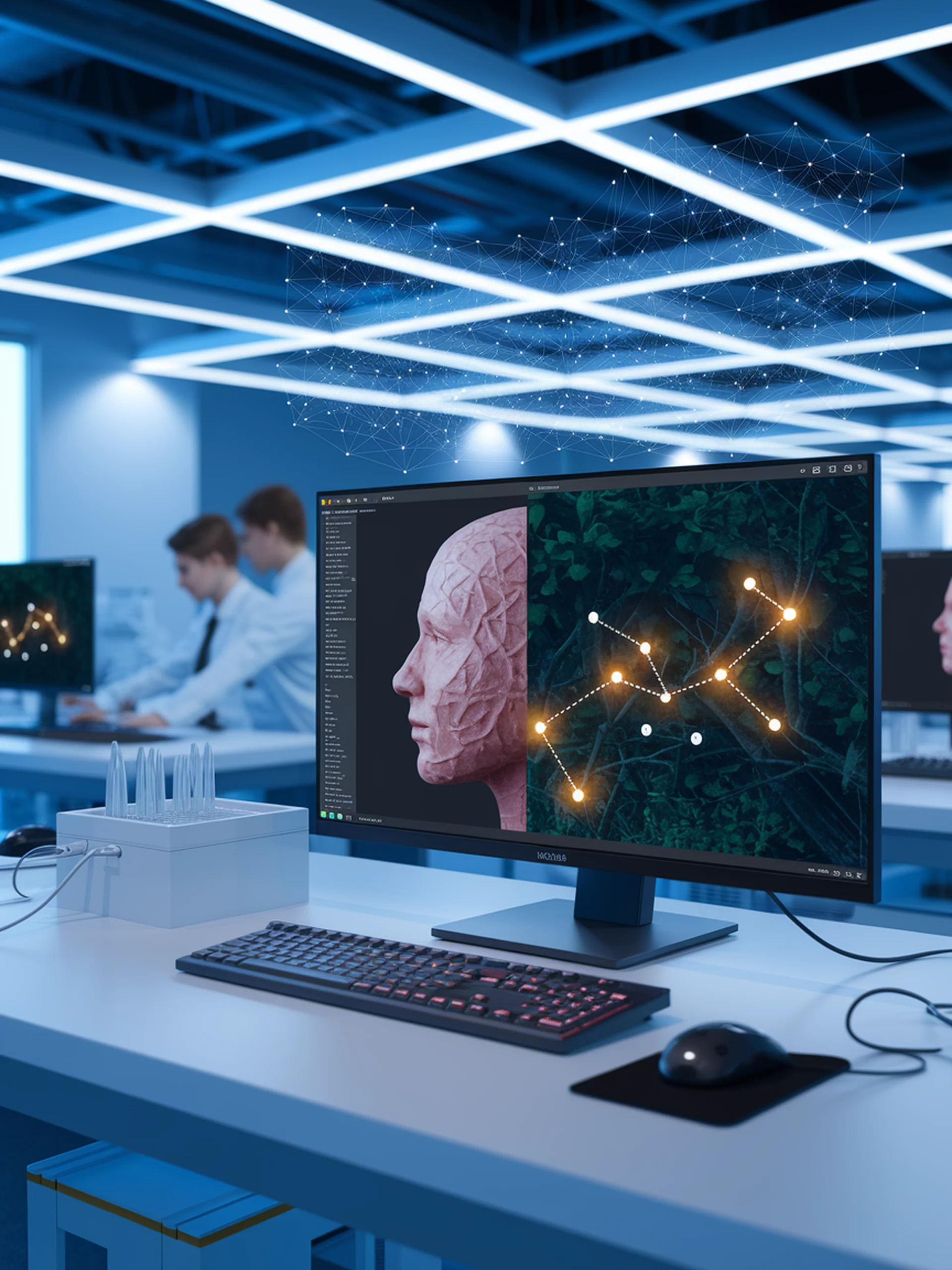
Combating AI Hallucinations Efficiently
A Lightweight Detector for Visual-Language Model Inaccuracies
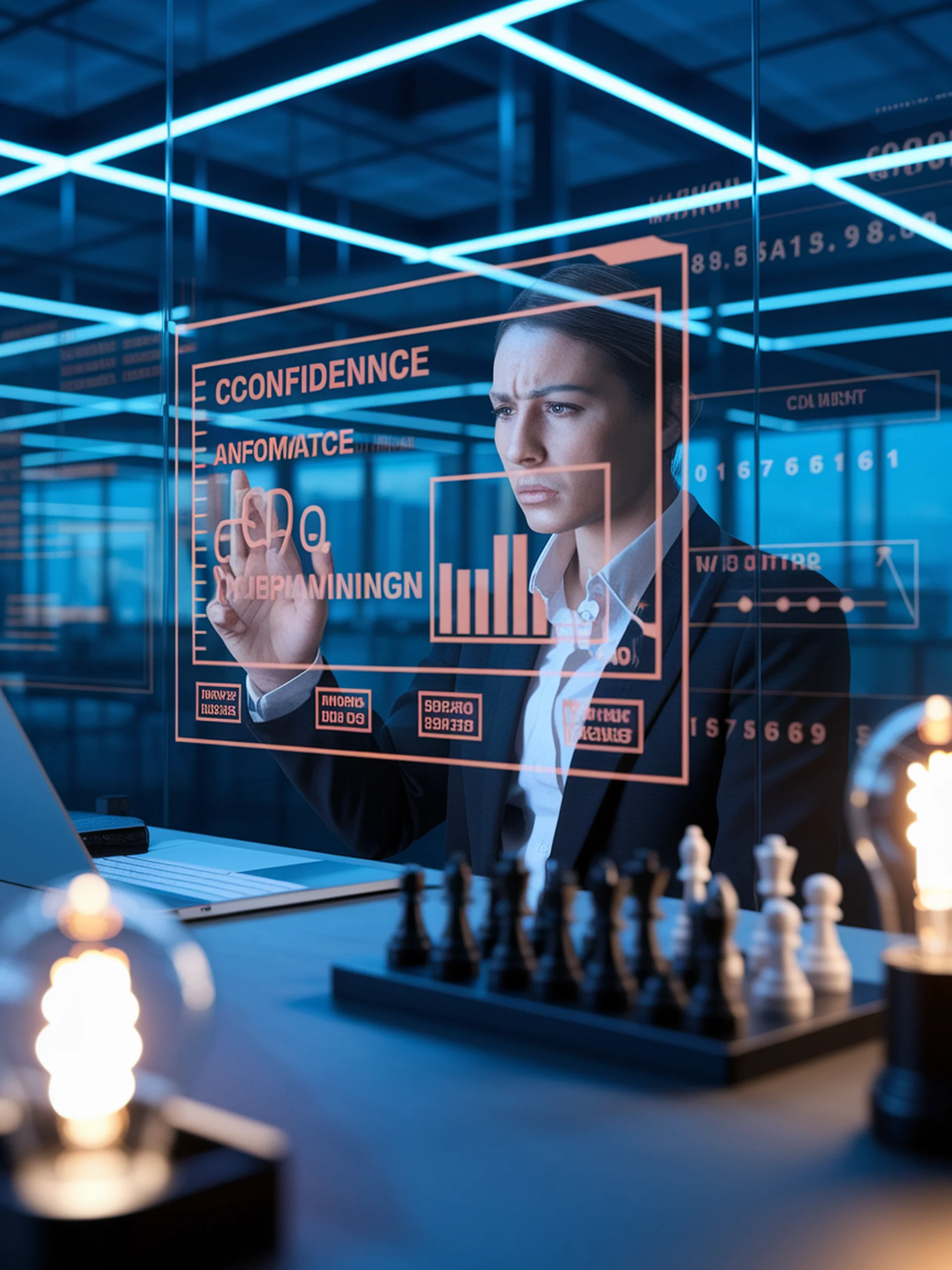
Confident Yet Wrong: The Danger of High-Certainty Hallucinations
Challenging the assumption that hallucinations correlate with uncertainty
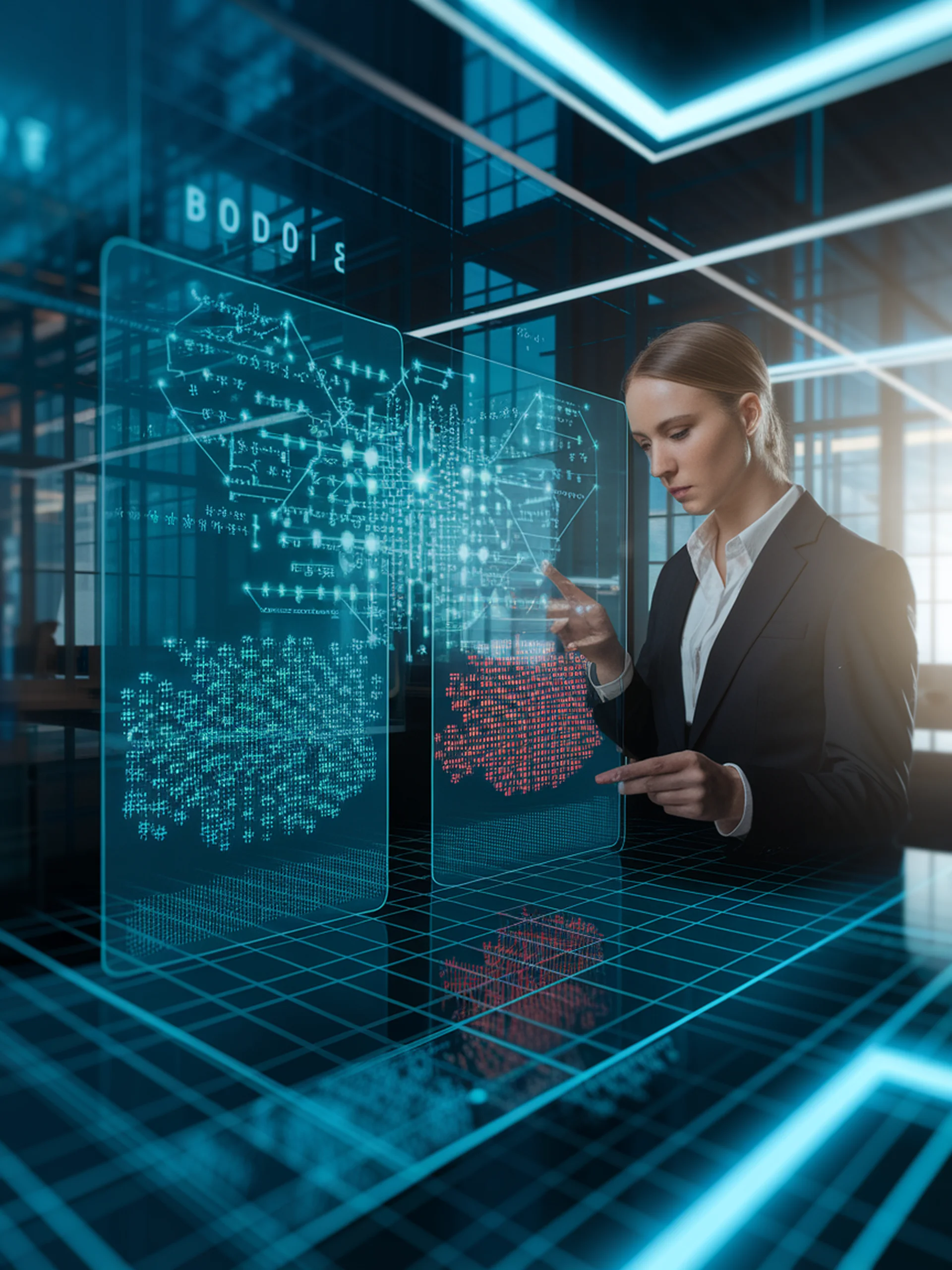
Combating LLM Hallucinations with Temporal Logic
A novel framework to detect AI-generated misinformation
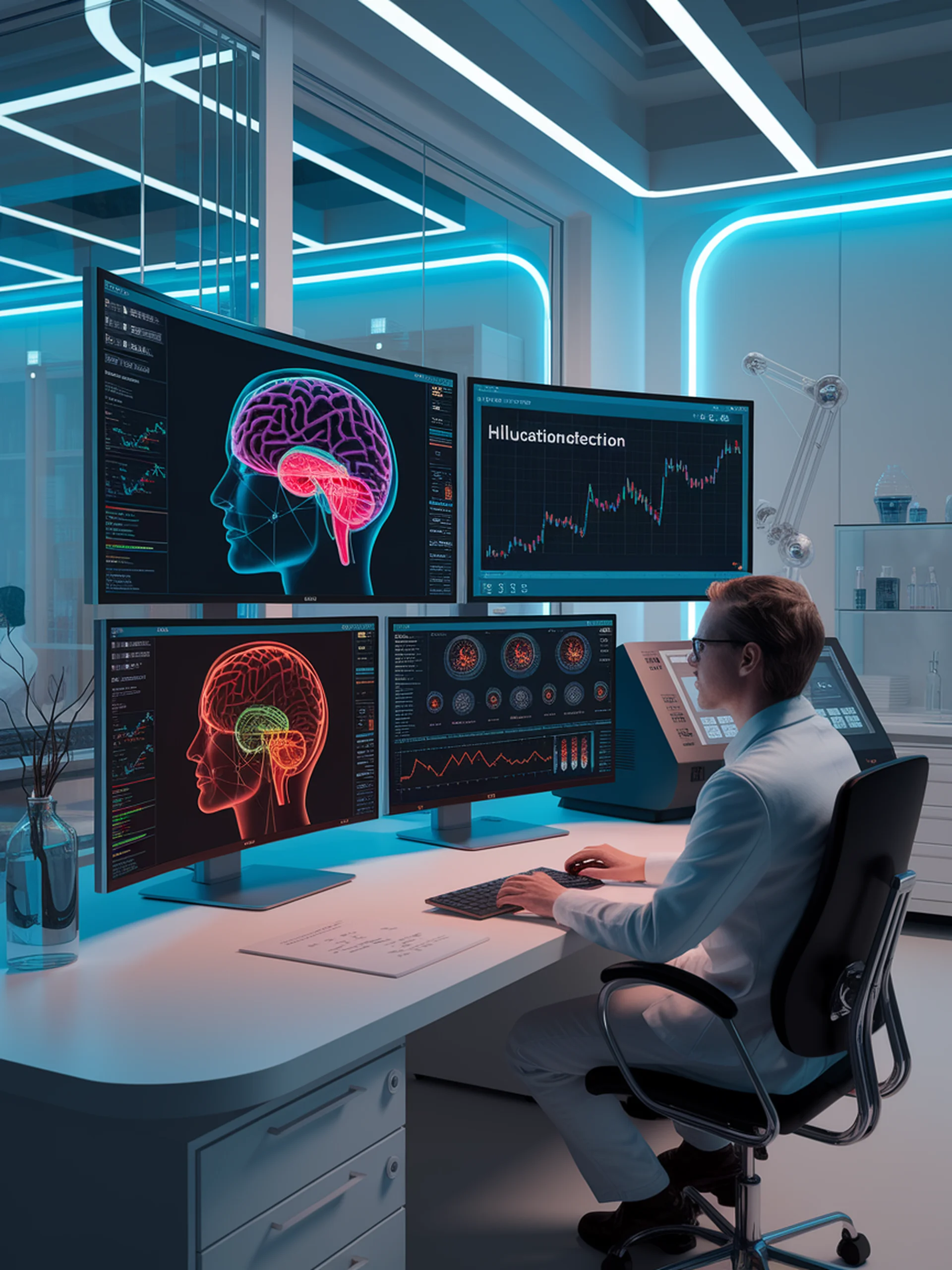
Looking Inside the LLM Mind
Detecting hallucinations through internal model states
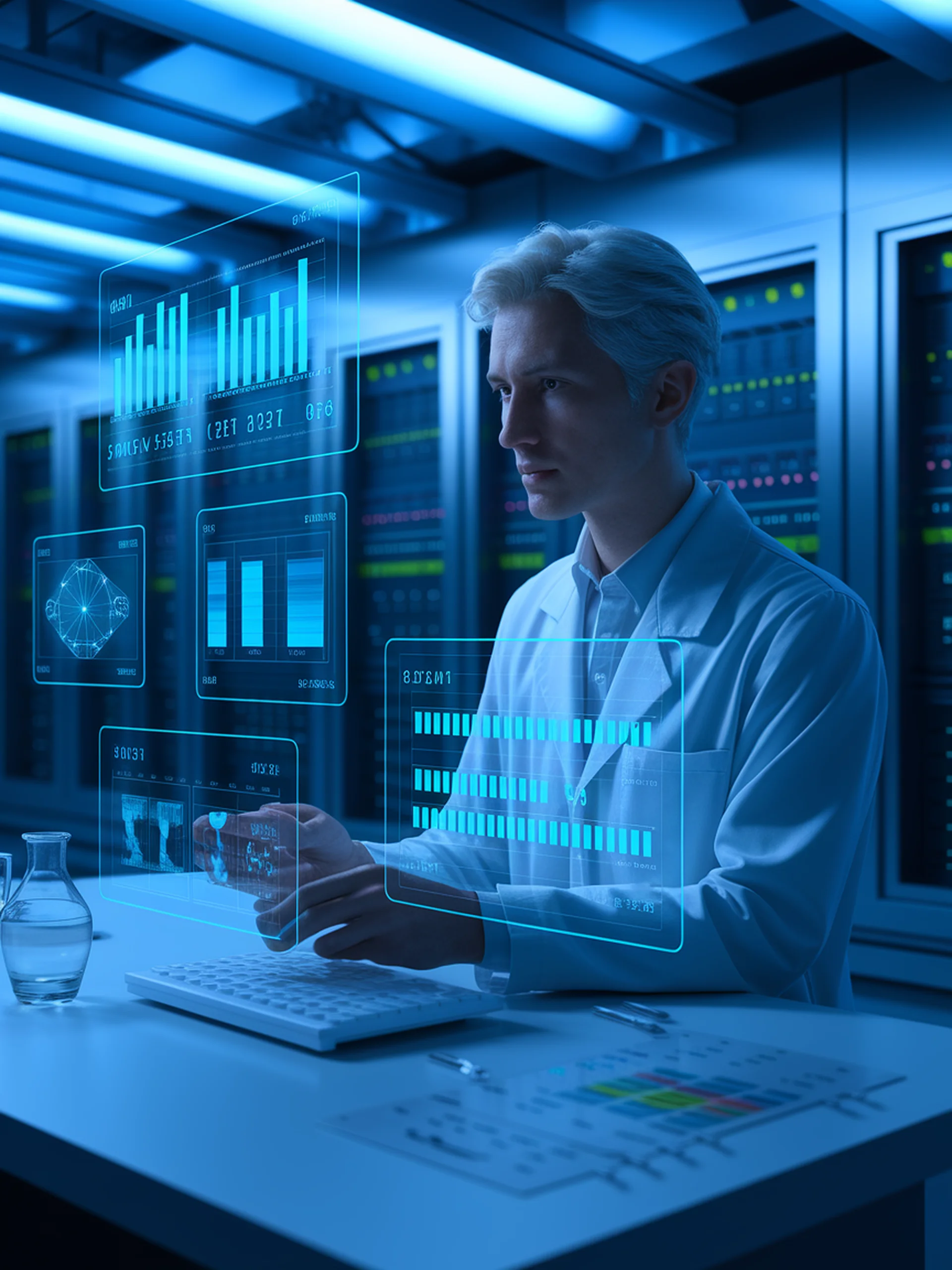
Better Confidence in AI Outputs
A new framework for evaluating how LLMs assess their own reliability
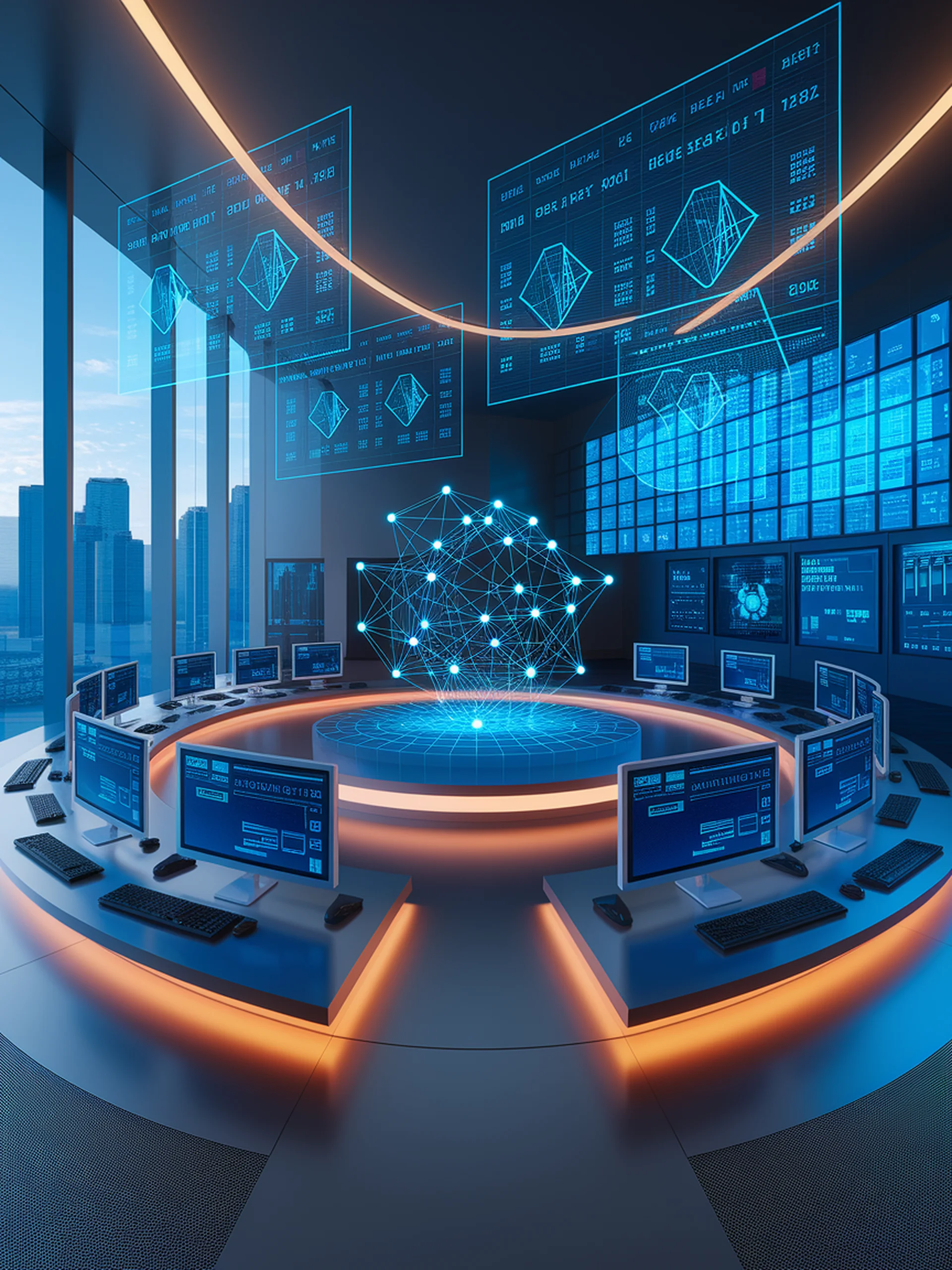
Building Trustworthy AI Systems
A comprehensive framework for evaluating and enhancing AI safety
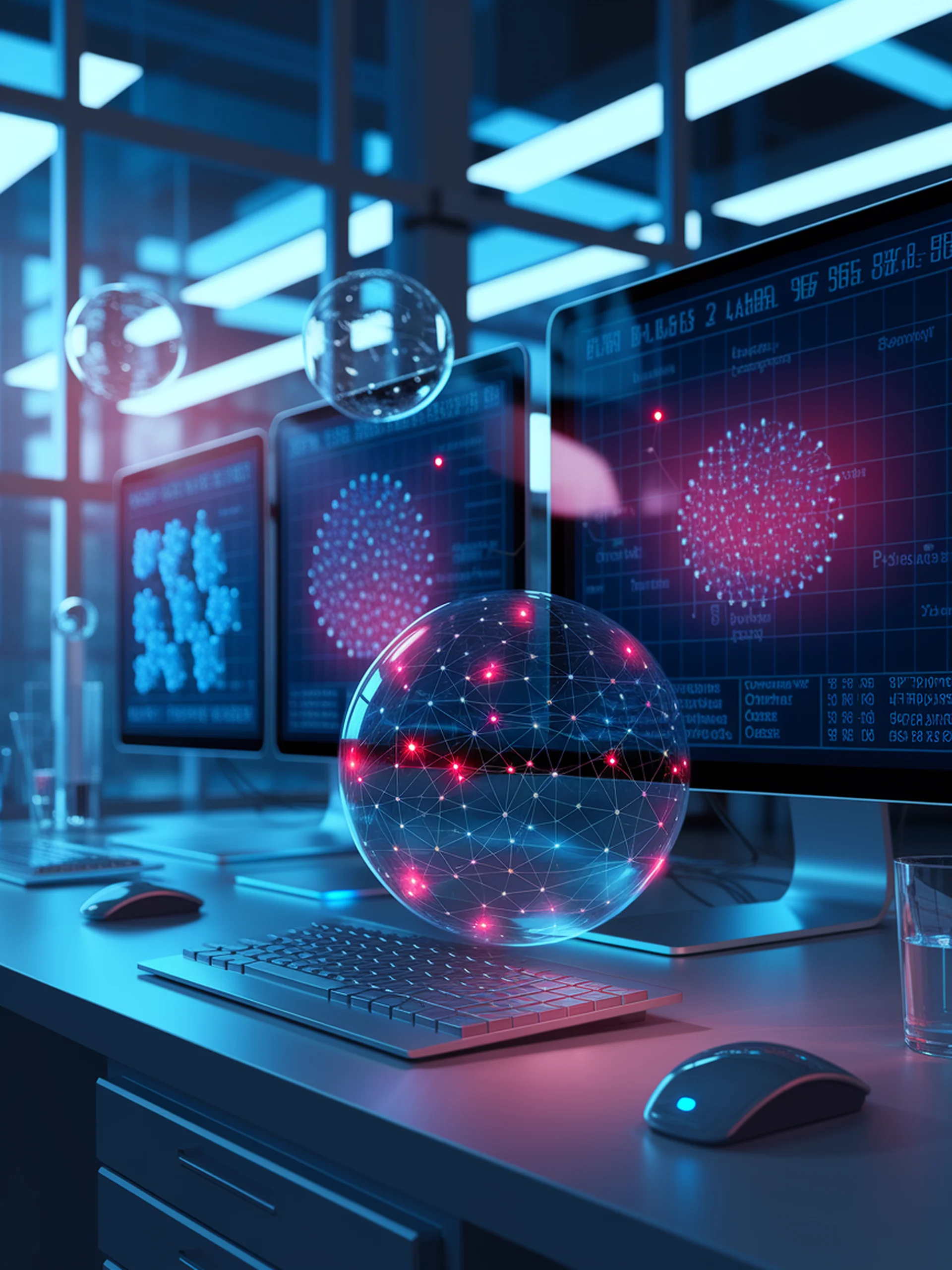
Improving LLM Truthfulness with Uncertainty Detection
Novel density-based approach outperforms existing uncertainty methods
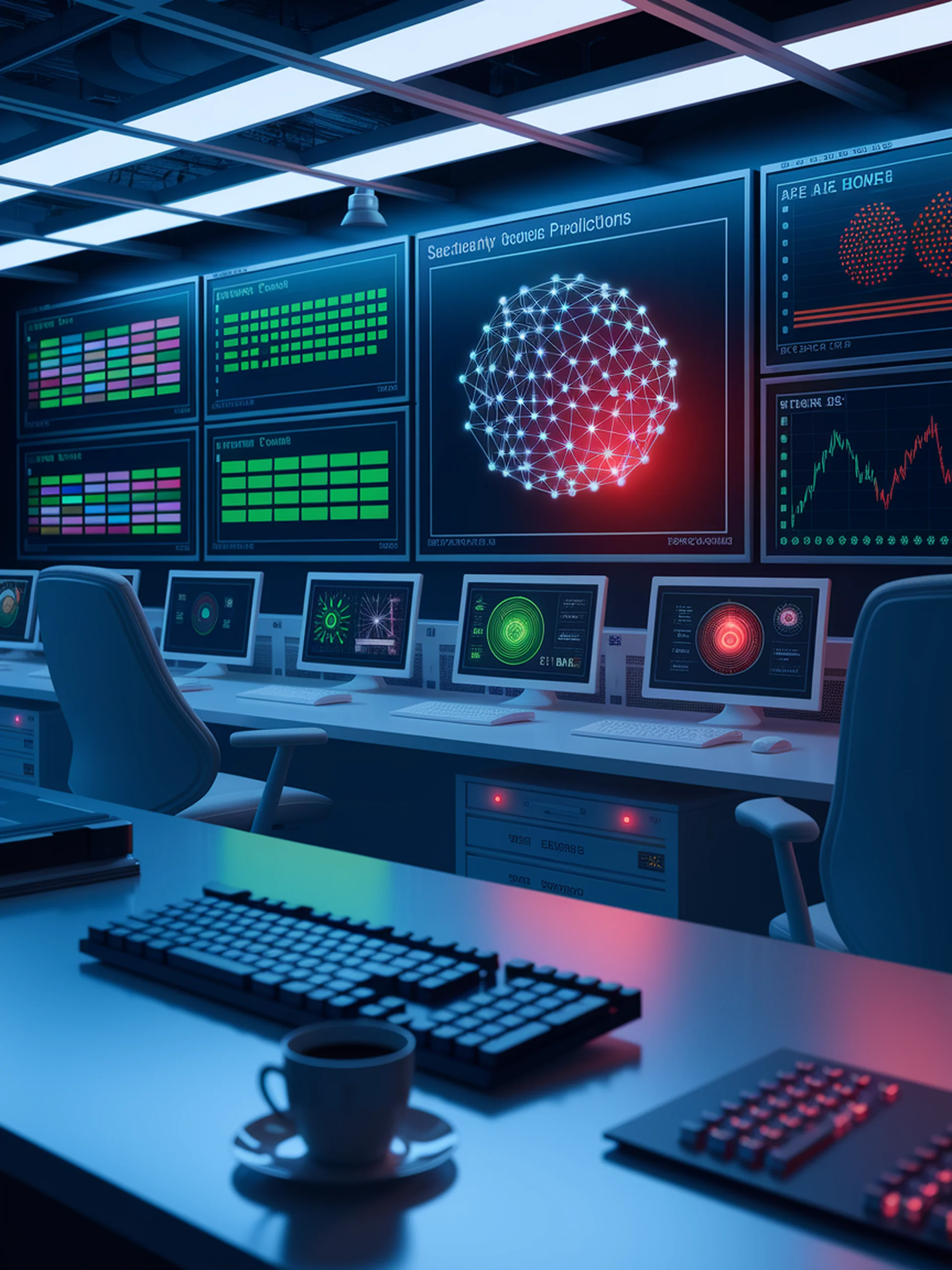
Predicting When LLMs Will Fail
A Framework for Safer AI by Making Failures Predictable
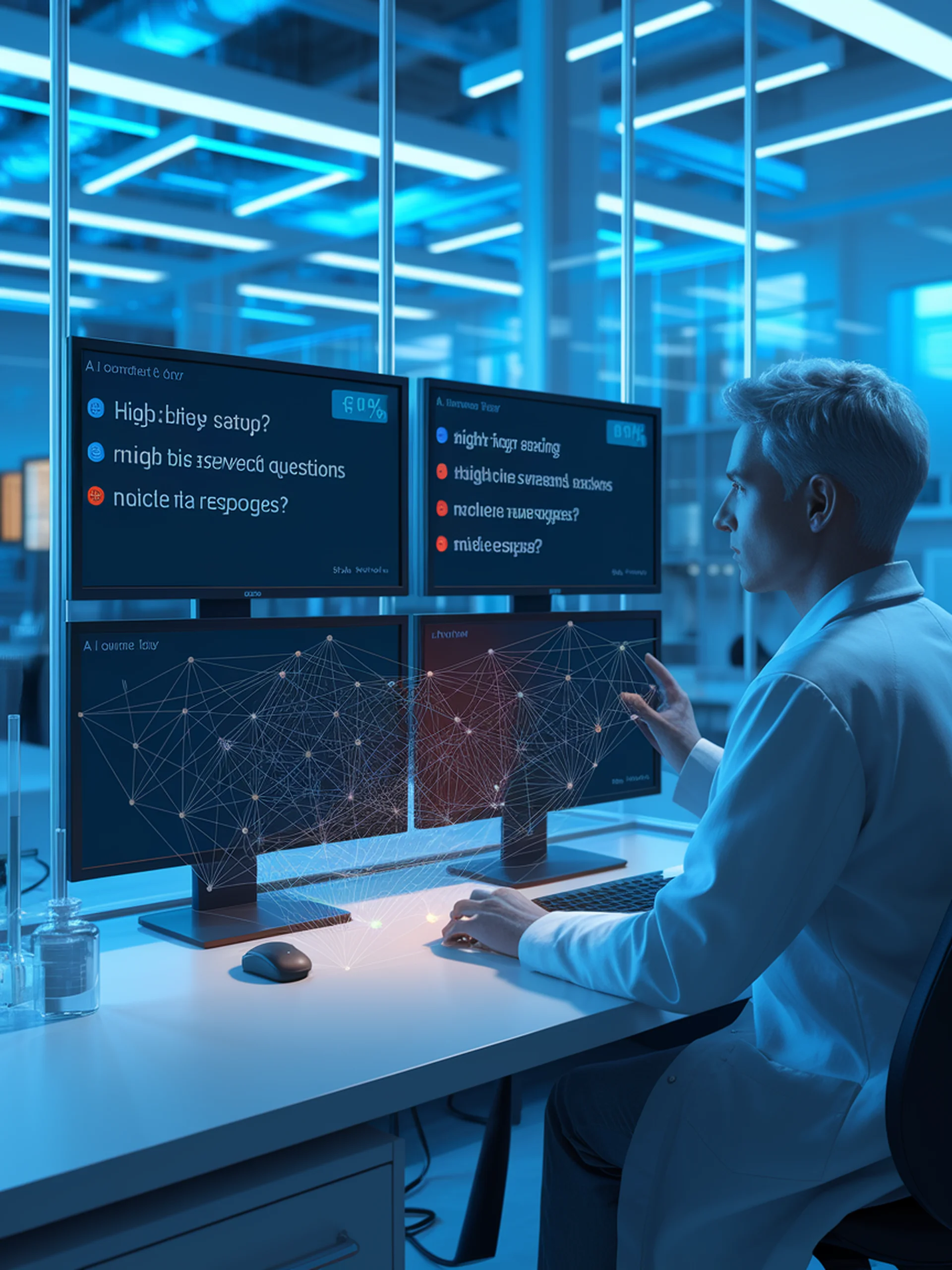
Fighting AI Hallucinations
Detecting AI Falsehoods Without External Fact-Checking
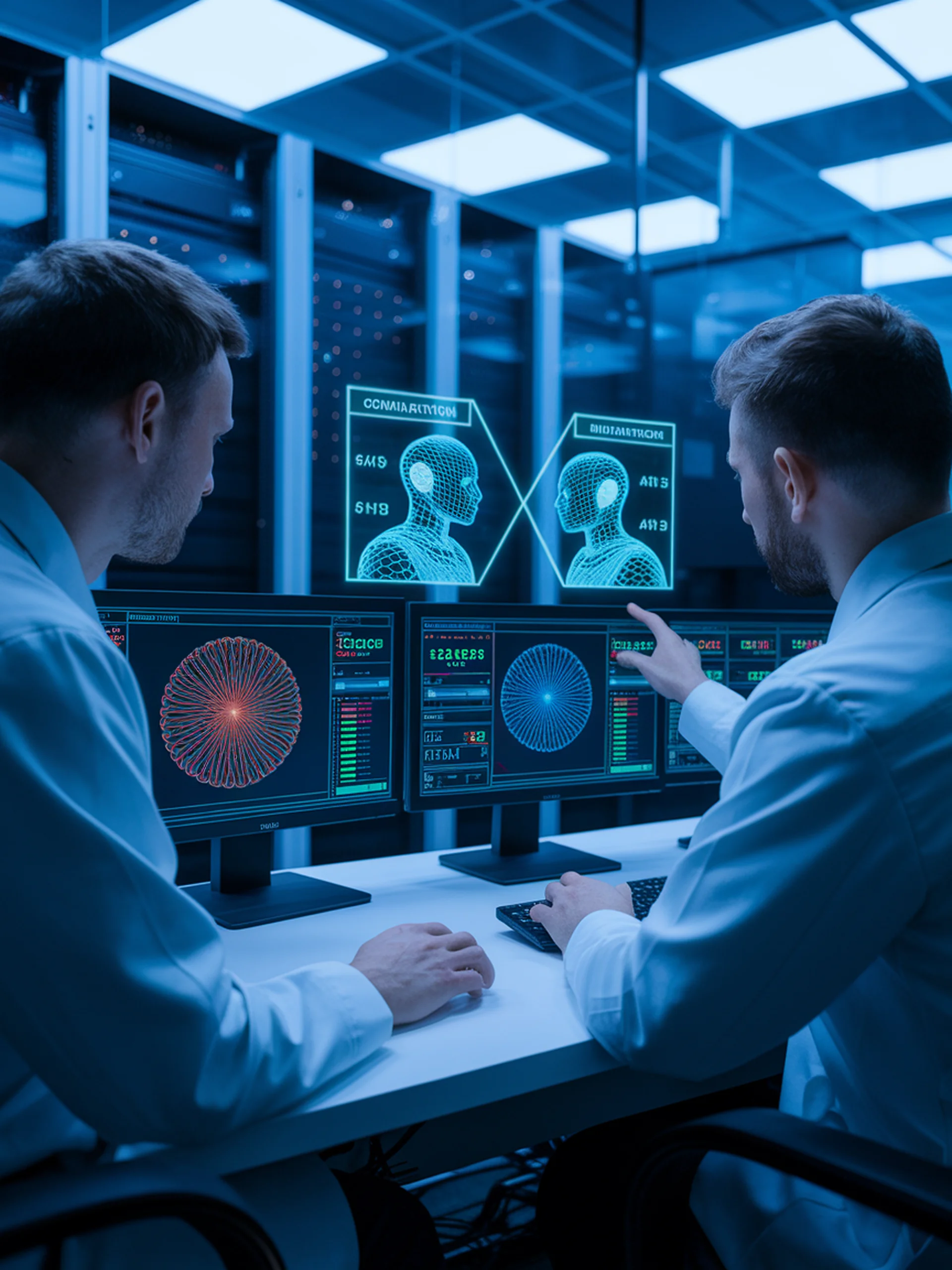
Beyond Self-Consistency: Detecting LLM Hallucinations
Leveraging cross-model verification to improve hallucination detection
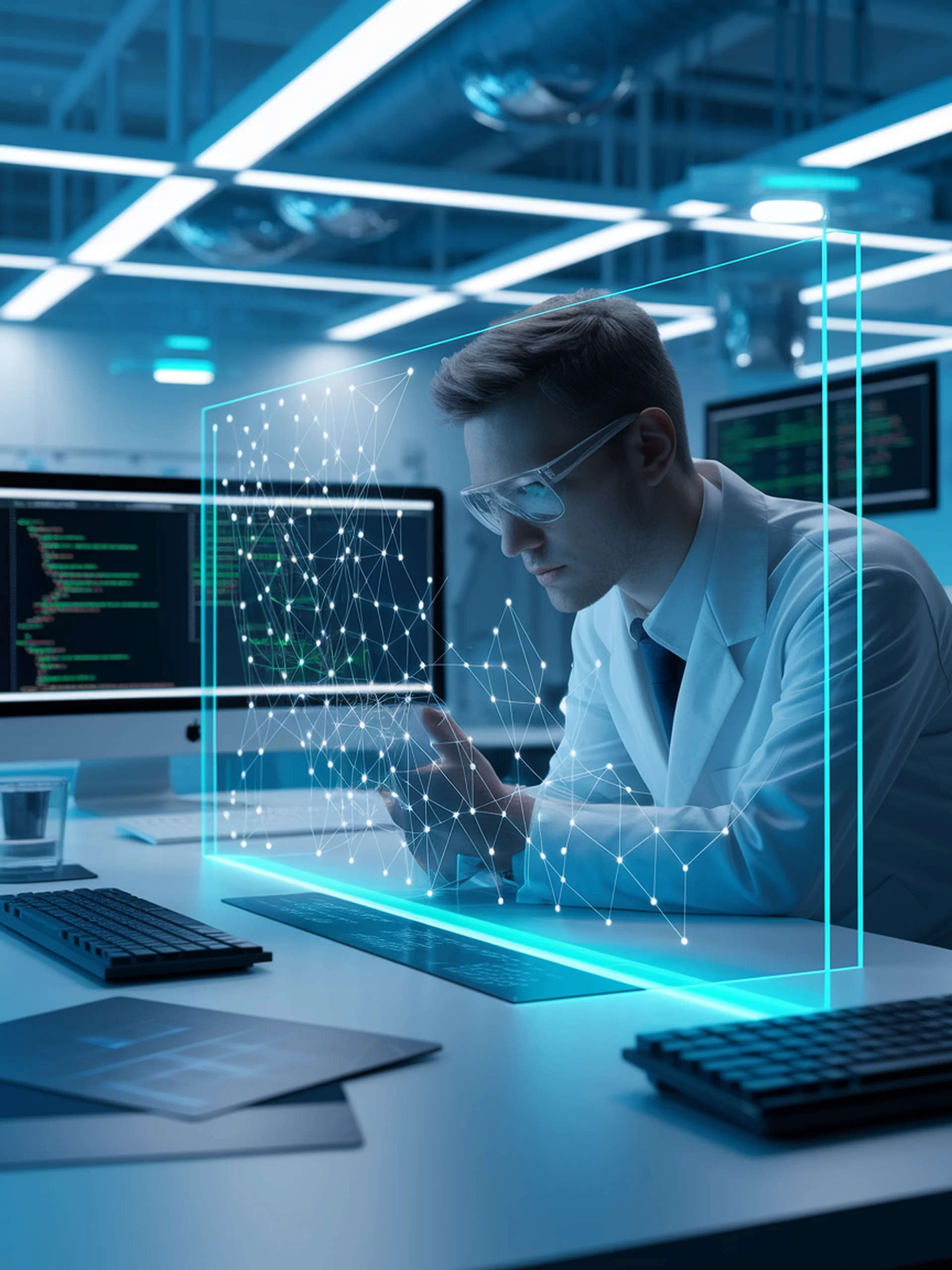
Understanding and Preventing LLM Hallucinations
The Law of Knowledge Overshadowing reveals why AI models fabricate facts
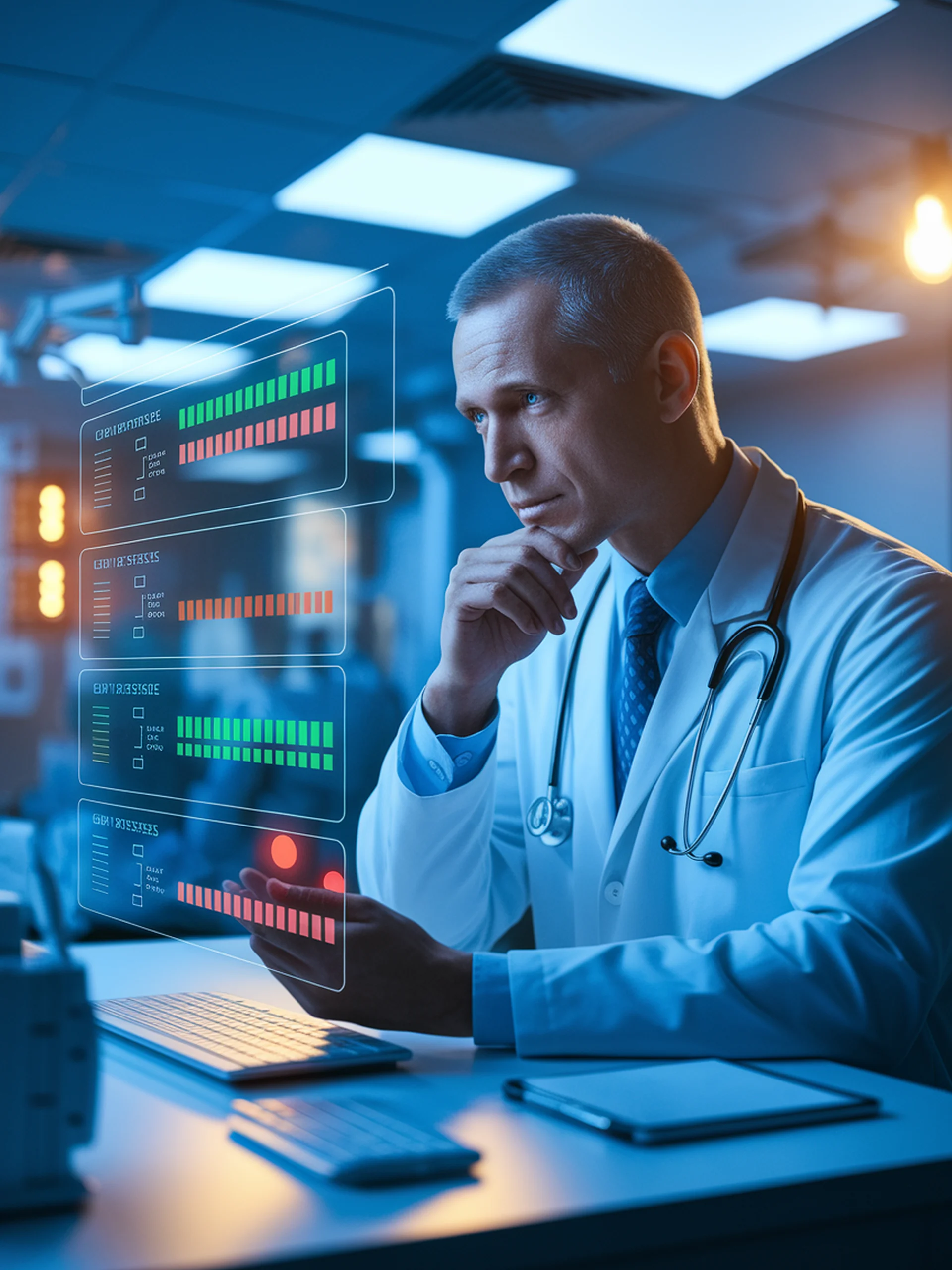
Beyond Yes or No: Making LLMs Truly Reliable
Multi-dimensional uncertainty quantification for safer AI applications
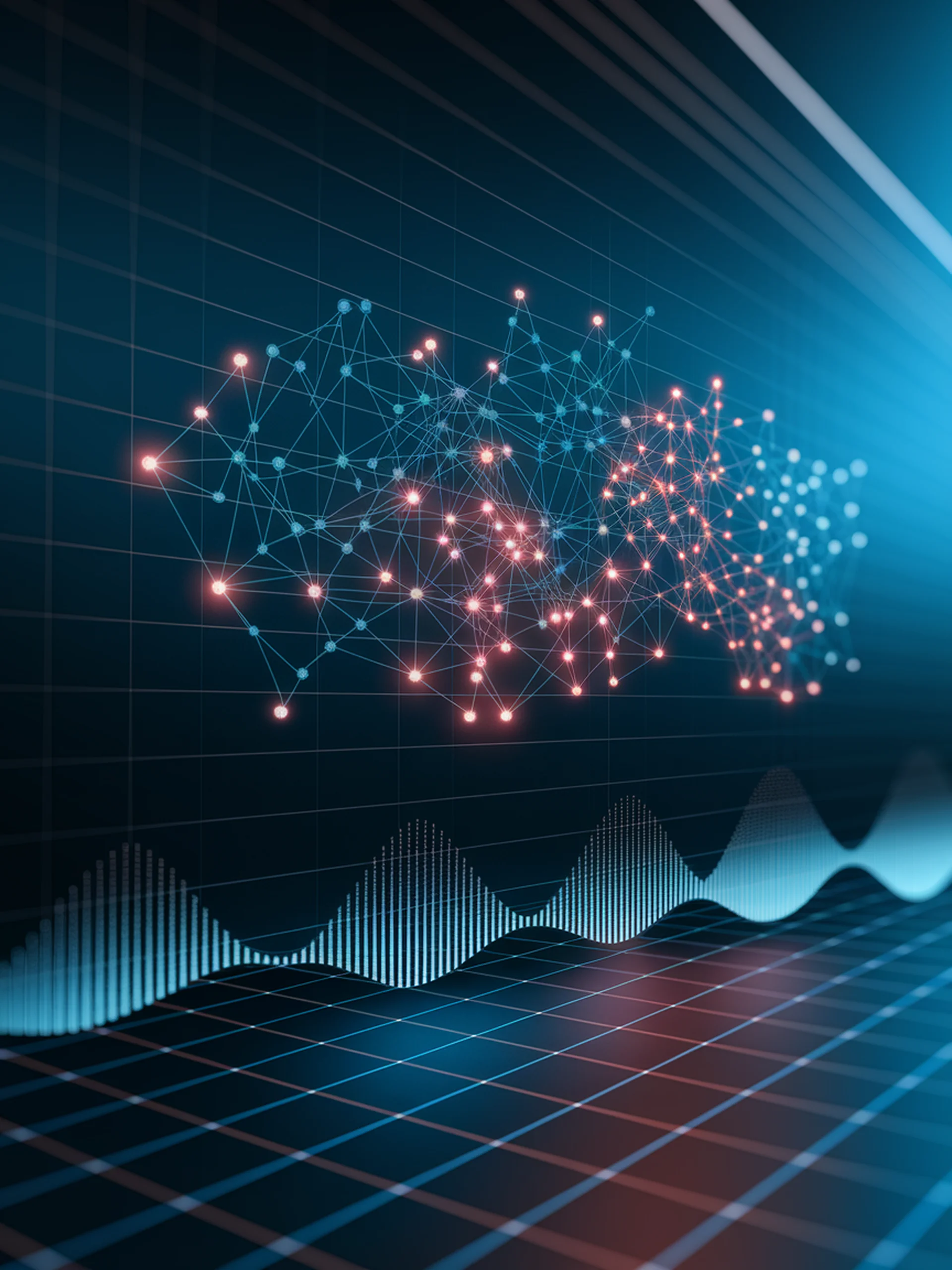
Detecting LLM Hallucinations with Graph Theory
A novel spectral approach to identify when AI systems fabricate information
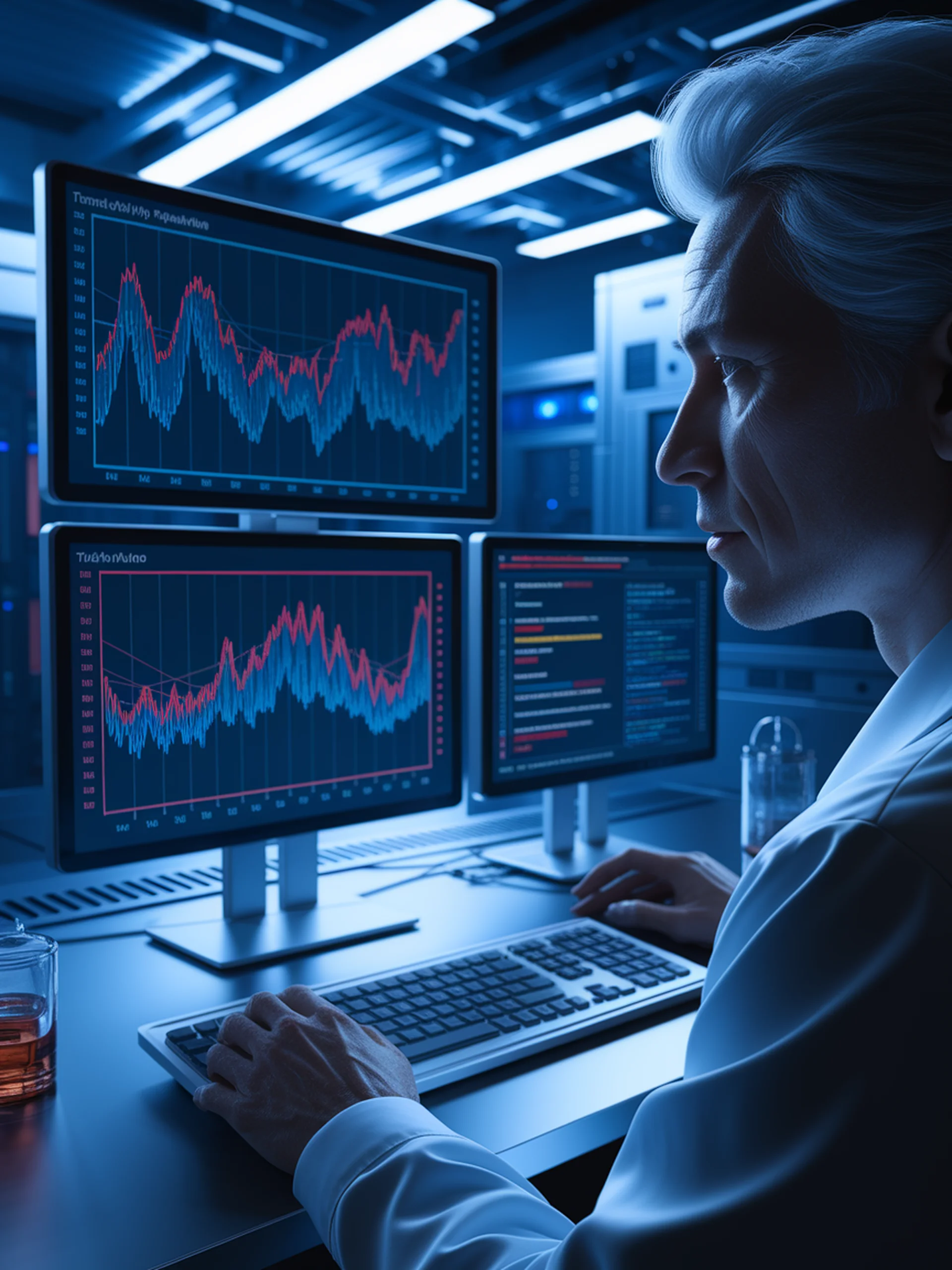
Making LLMs Safer Through Better Uncertainty Estimation
A more robust approach to measuring AI confidence
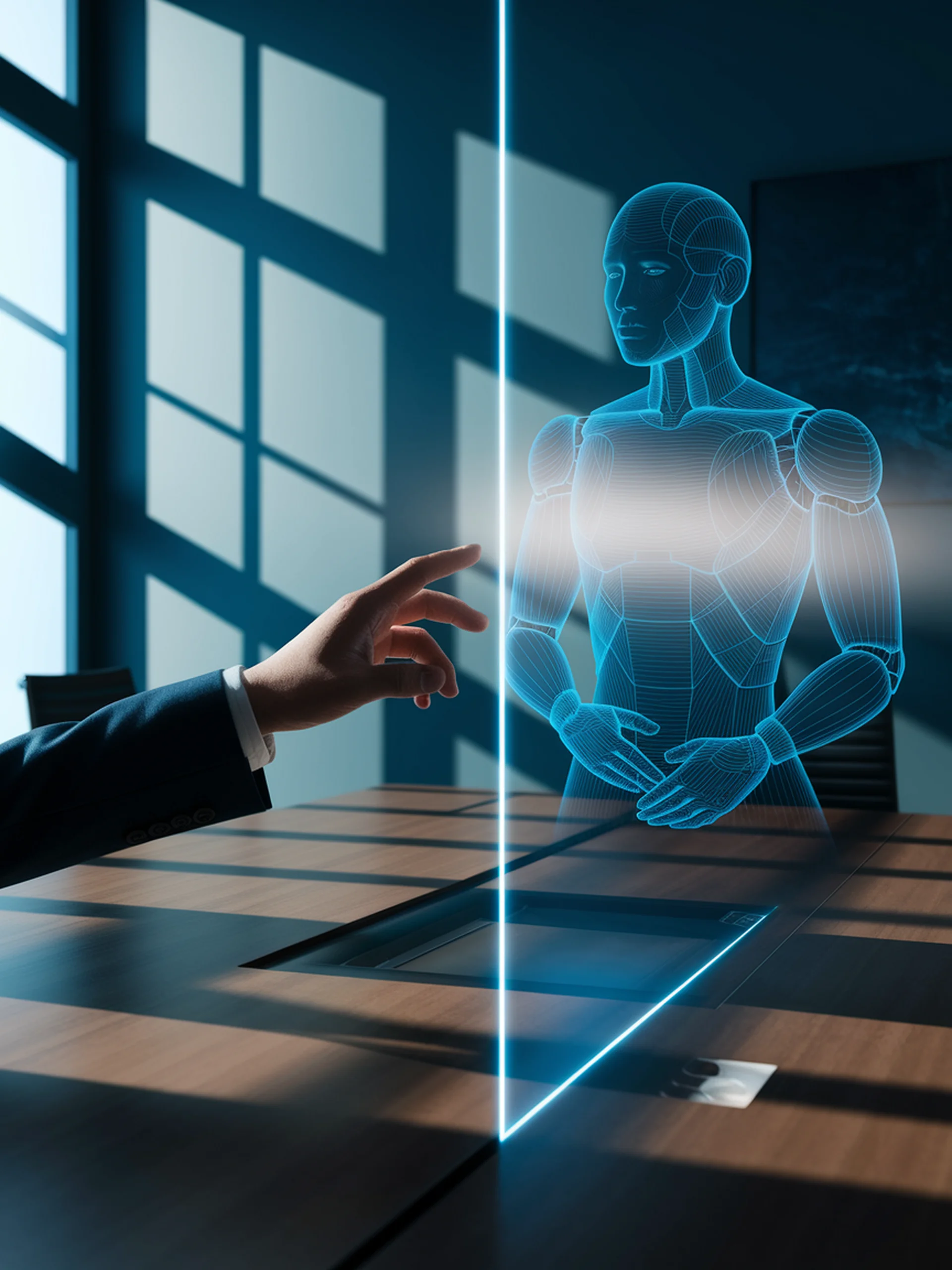
The Paradox of AI Trust
Why Explanation May Not Always Precede Trust in AI Systems
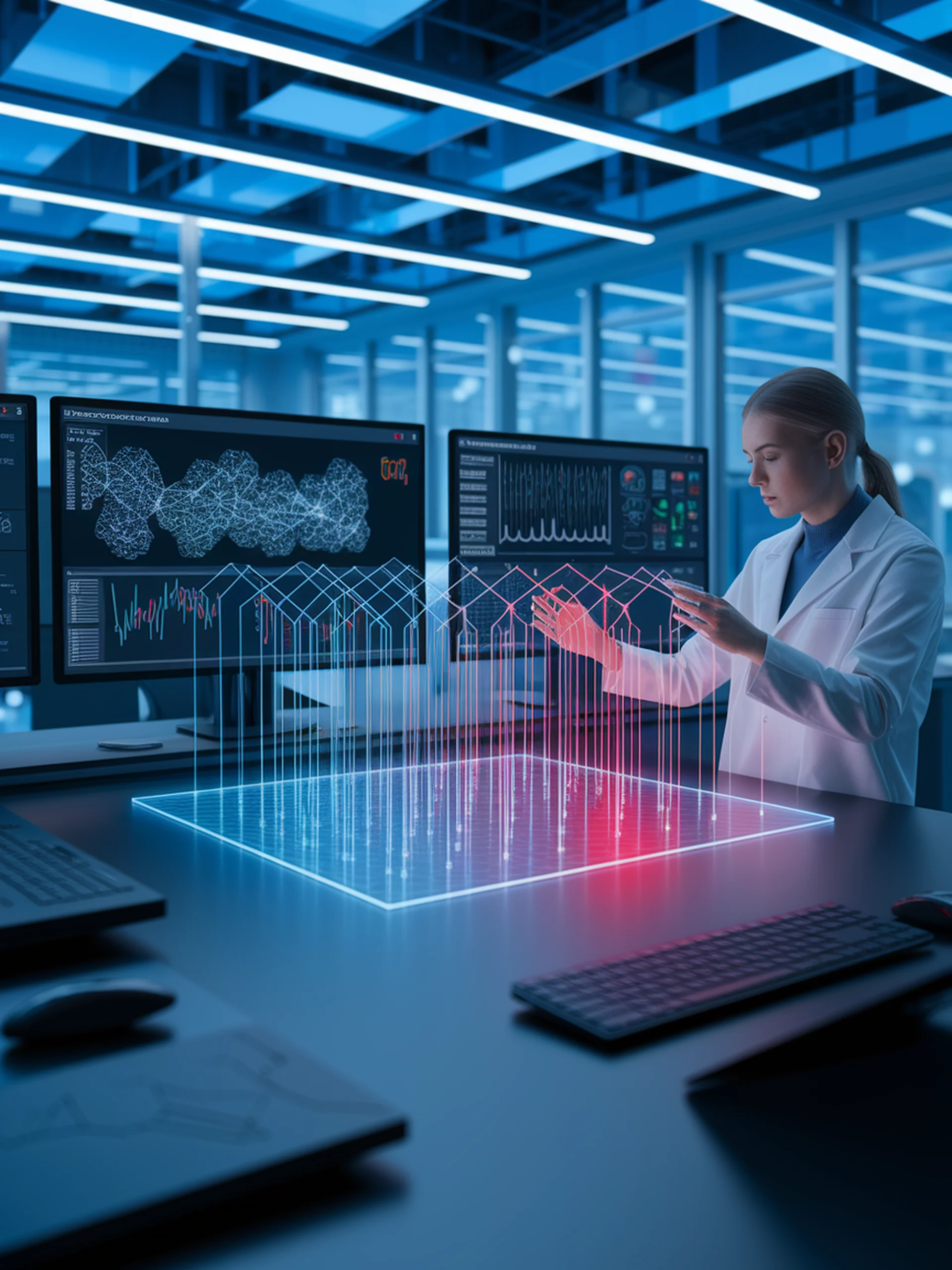
Detecting AI Hallucinations with Semantic Volume
A new method to measure both internal and external uncertainty in LLMs
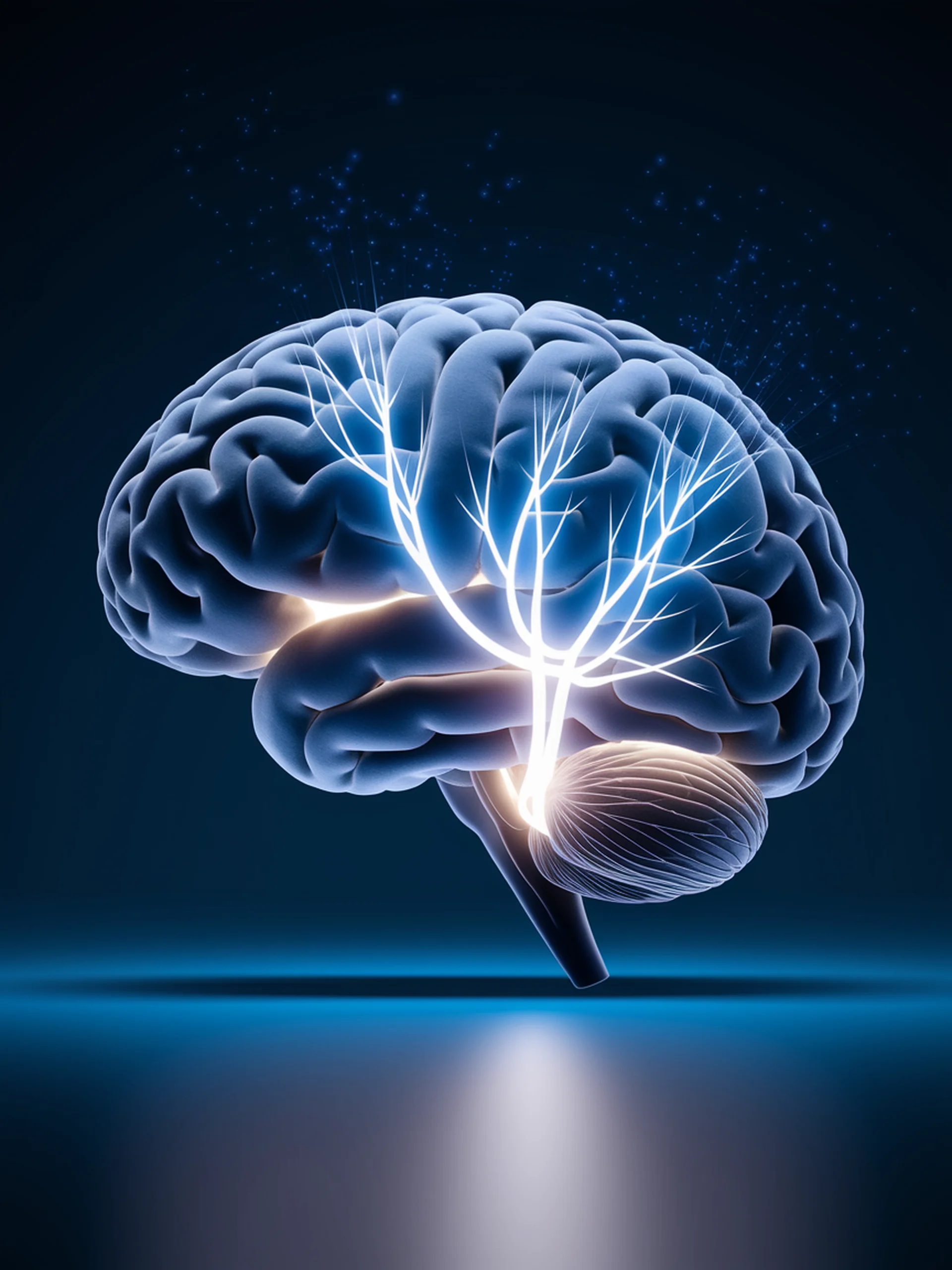
Self-Evolving Language Models
Enhancing Context Faithfulness Through Fine-Grained Self-Improvement
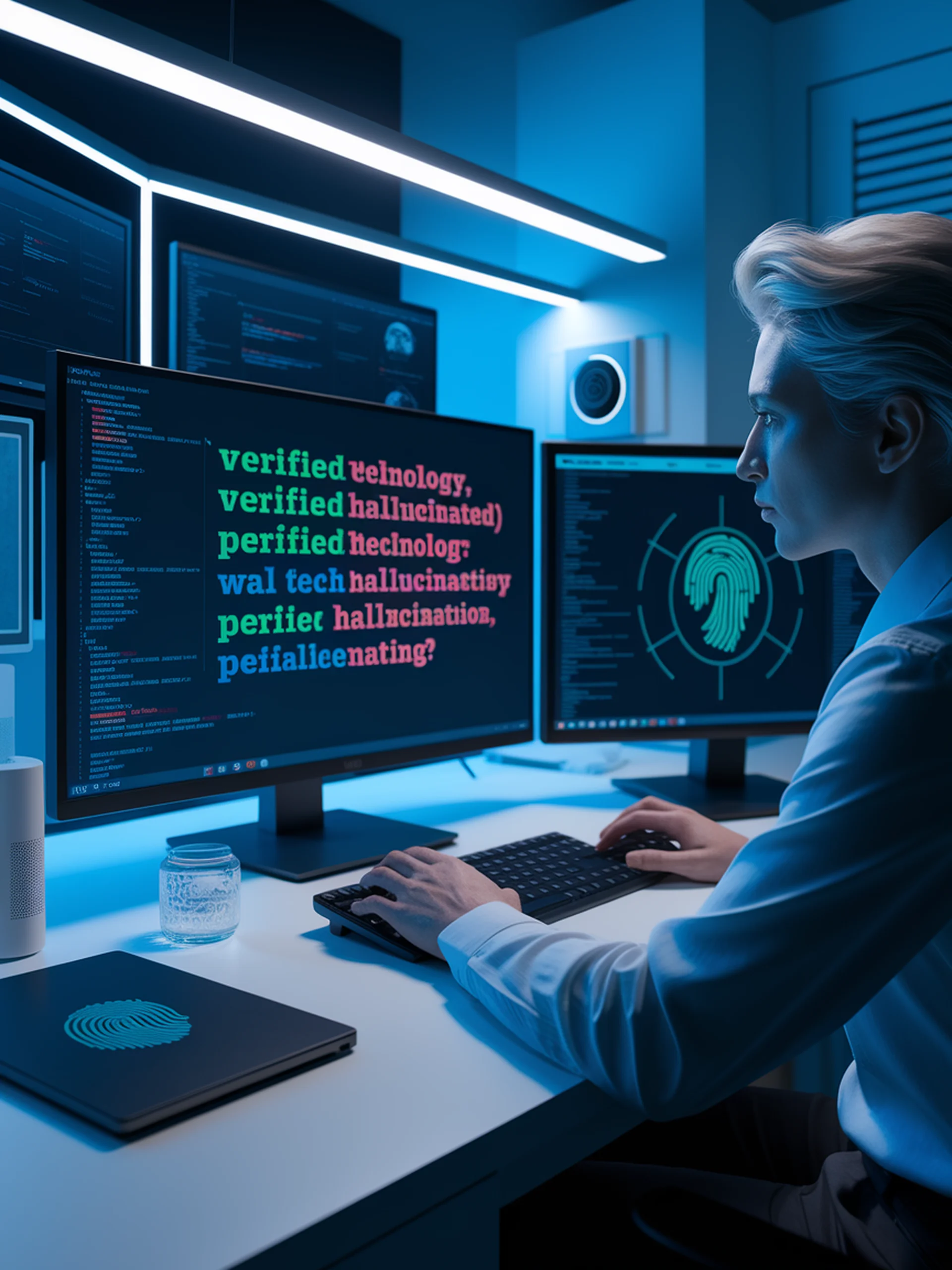
Fighting Hallucinations with Highlighted References
A new technique for fact-grounded LLM responses
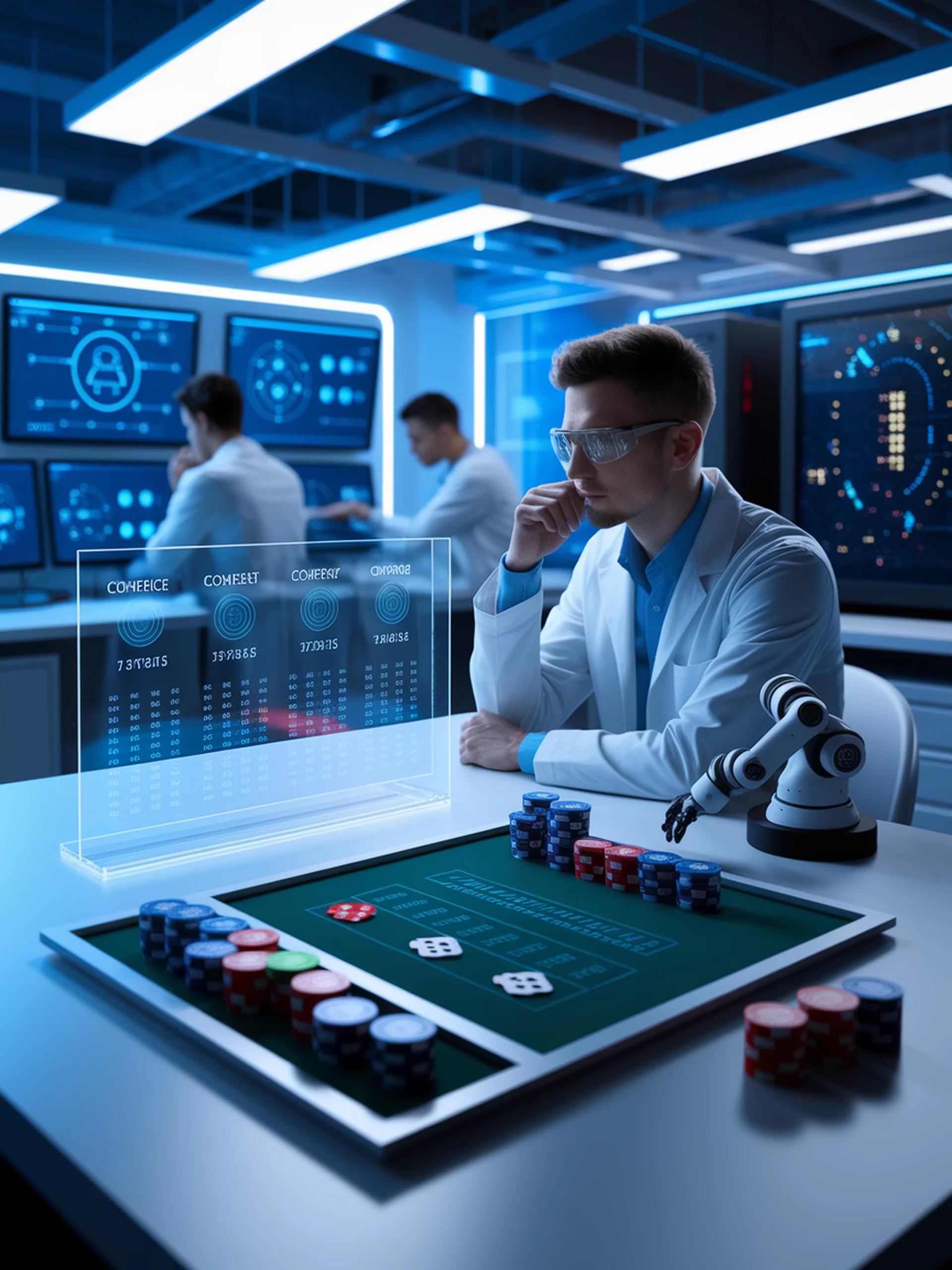
Teaching AI to Know When It Doesn't Know
A reinforcement learning approach to confidence calibration in LLMs
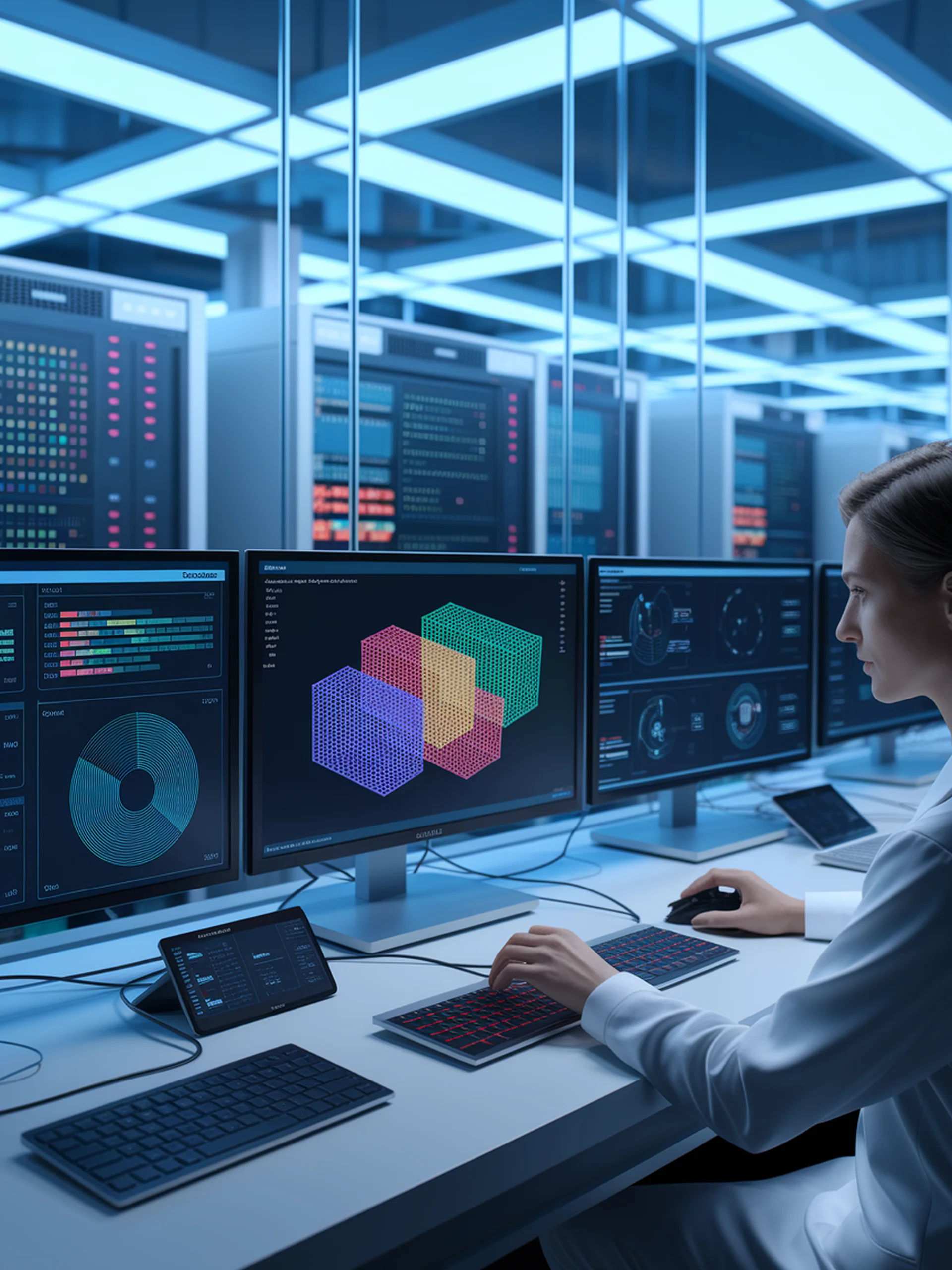
Measuring Truth in AI Systems
First comprehensive benchmark for LLM honesty evaluation
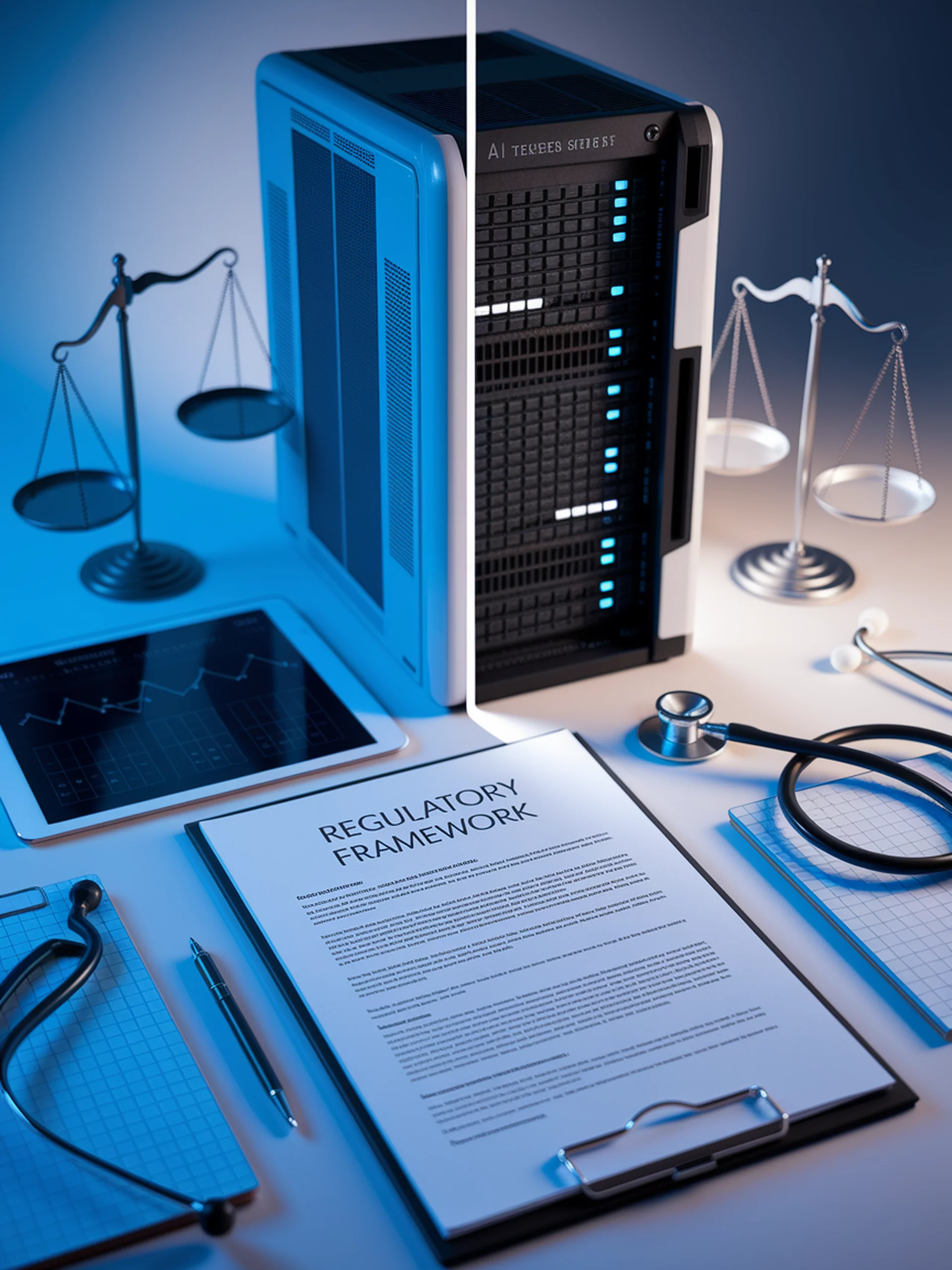
Setting Standards for AI Hallucinations
A Regulatory Framework for Domain-Specific LLMs
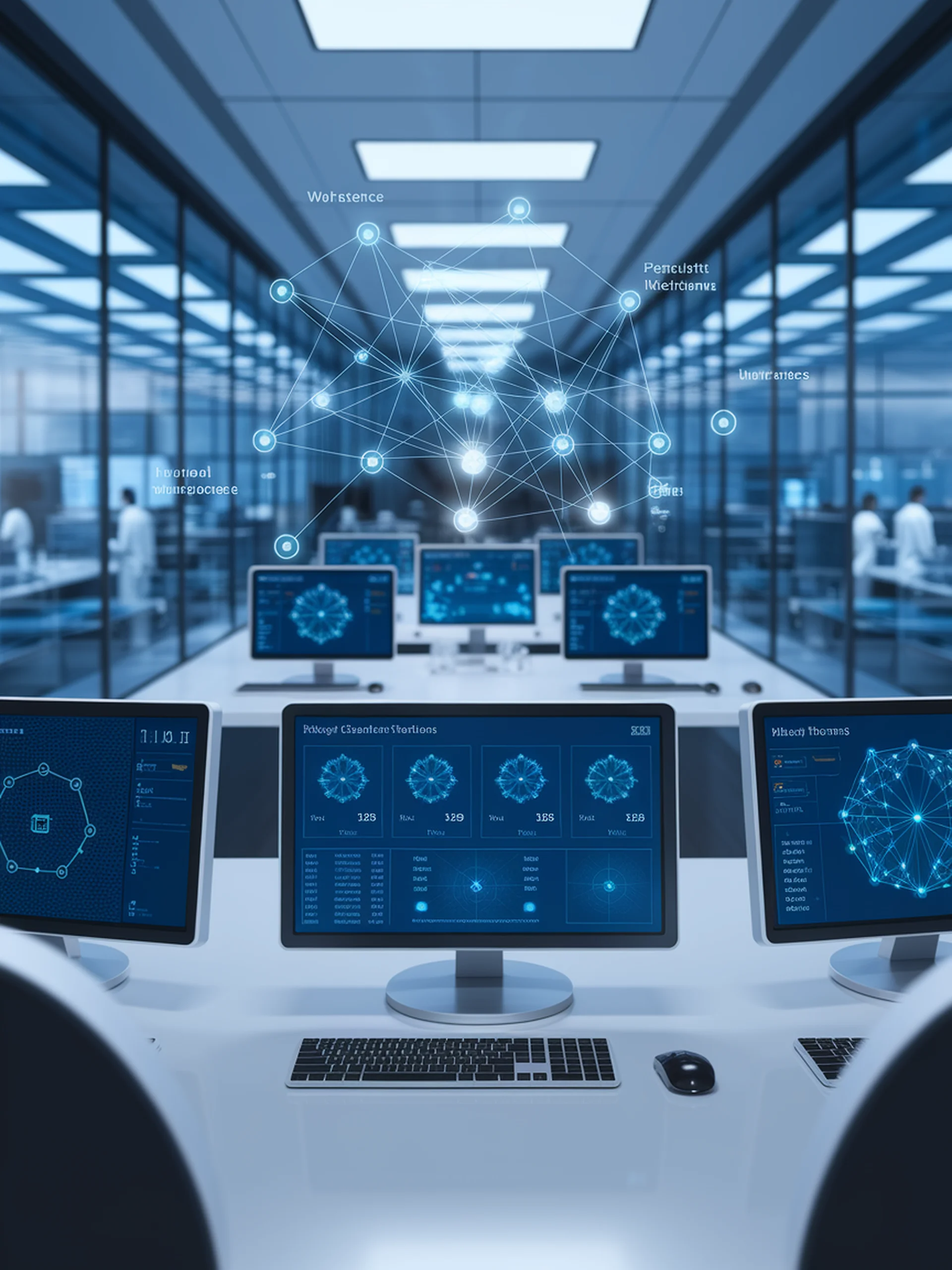
Combating LLM Hallucinations Through Smart Ensemble Methods
A novel uncertainty-aware framework that improves factual accuracy
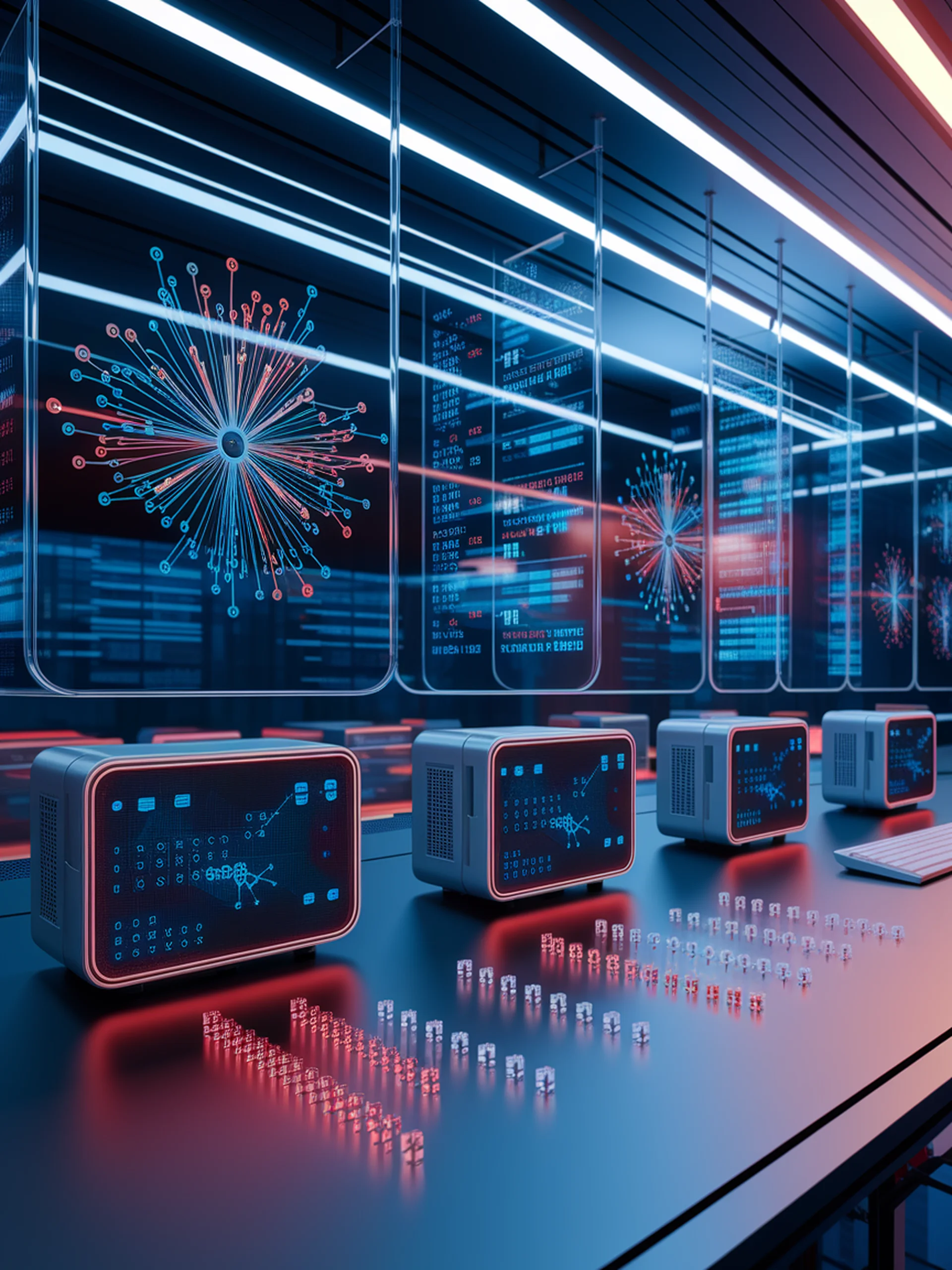
Detecting LLM Hallucinations
A new semantic clustering approach to identify factual errors
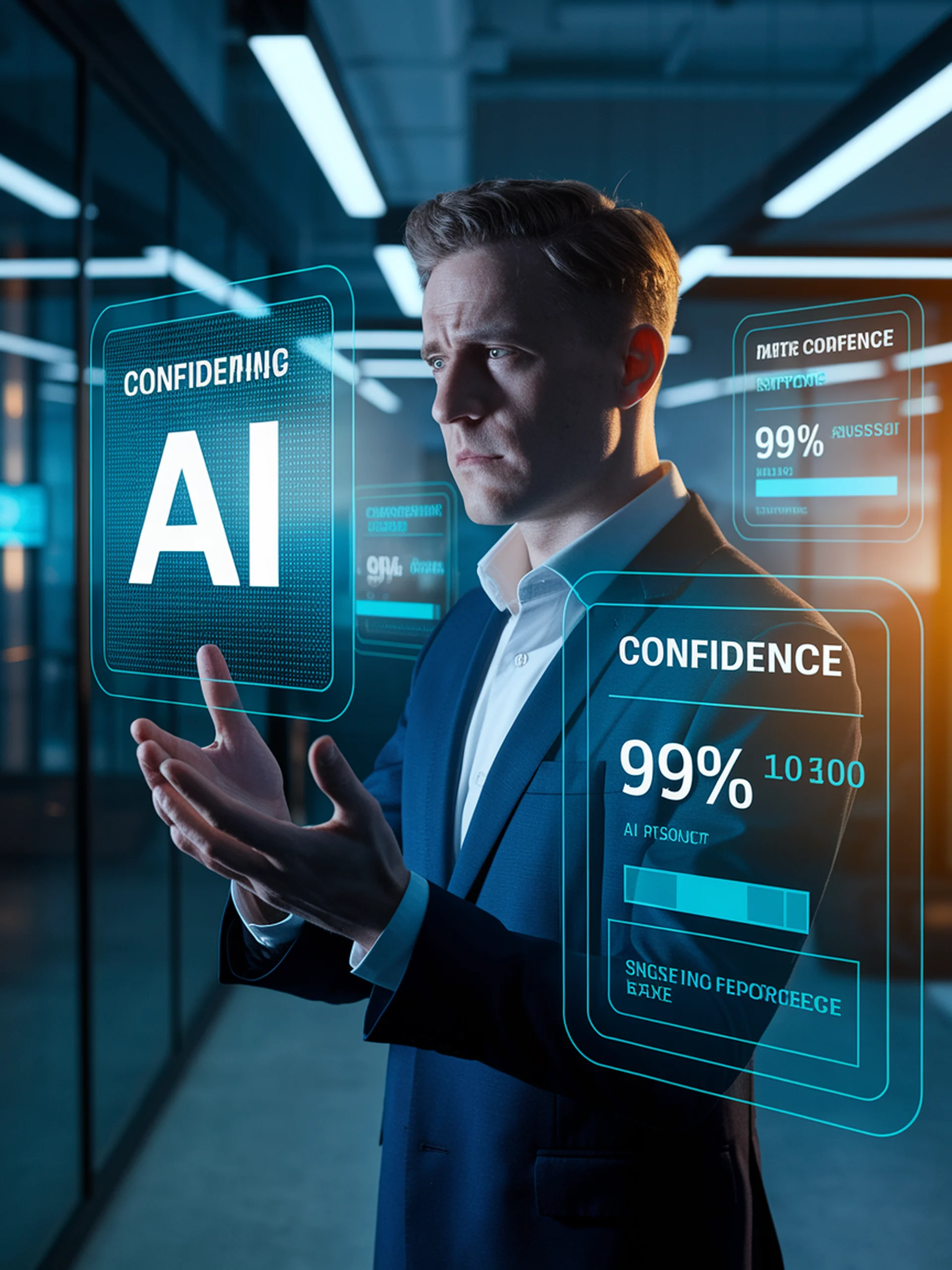
The Danger of AI Delusions
When LLMs hallucinate with high confidence
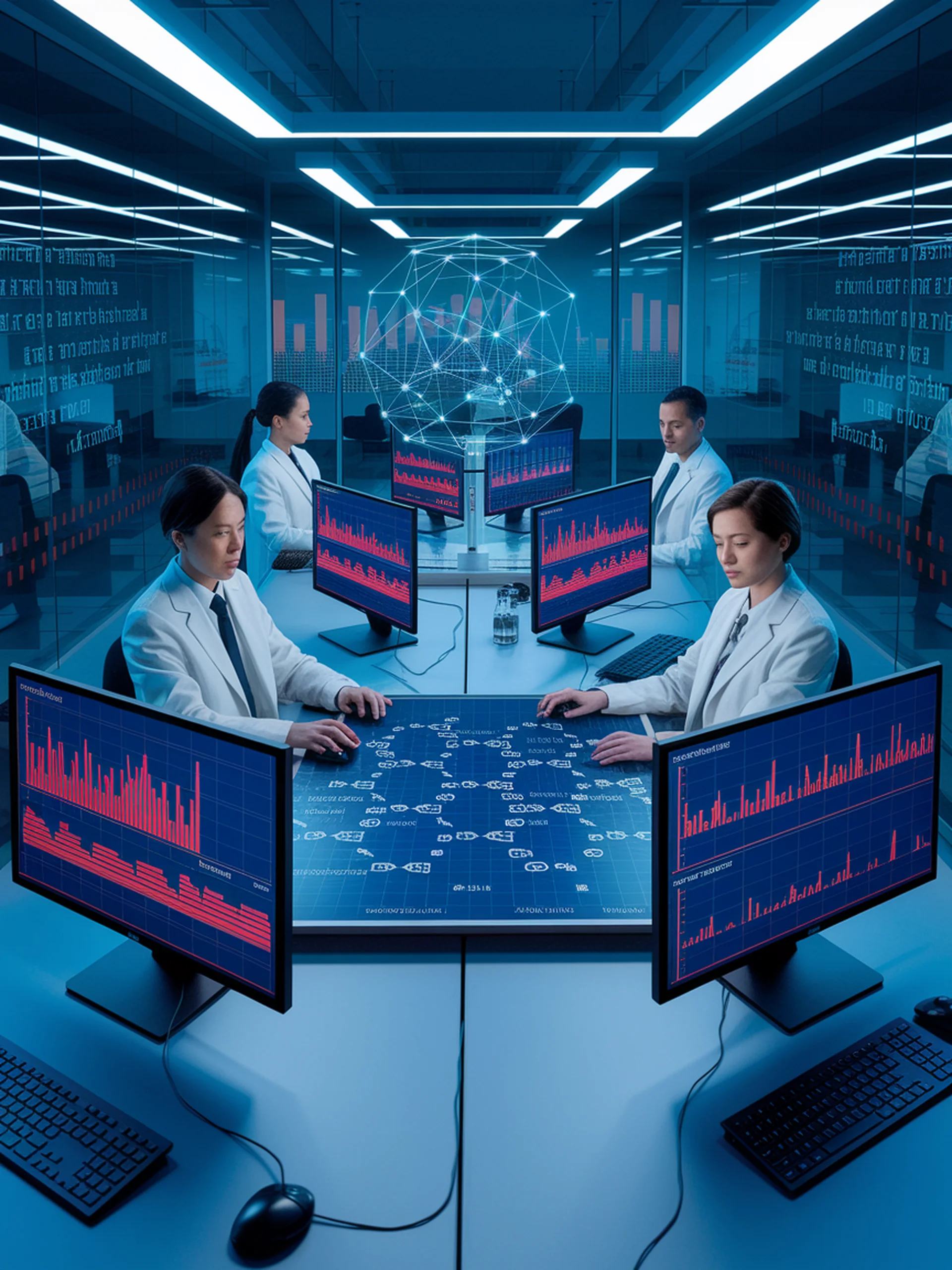
Combating LLM Hallucinations Across Languages
A fine-grained multilingual benchmark to detect AI's factual errors
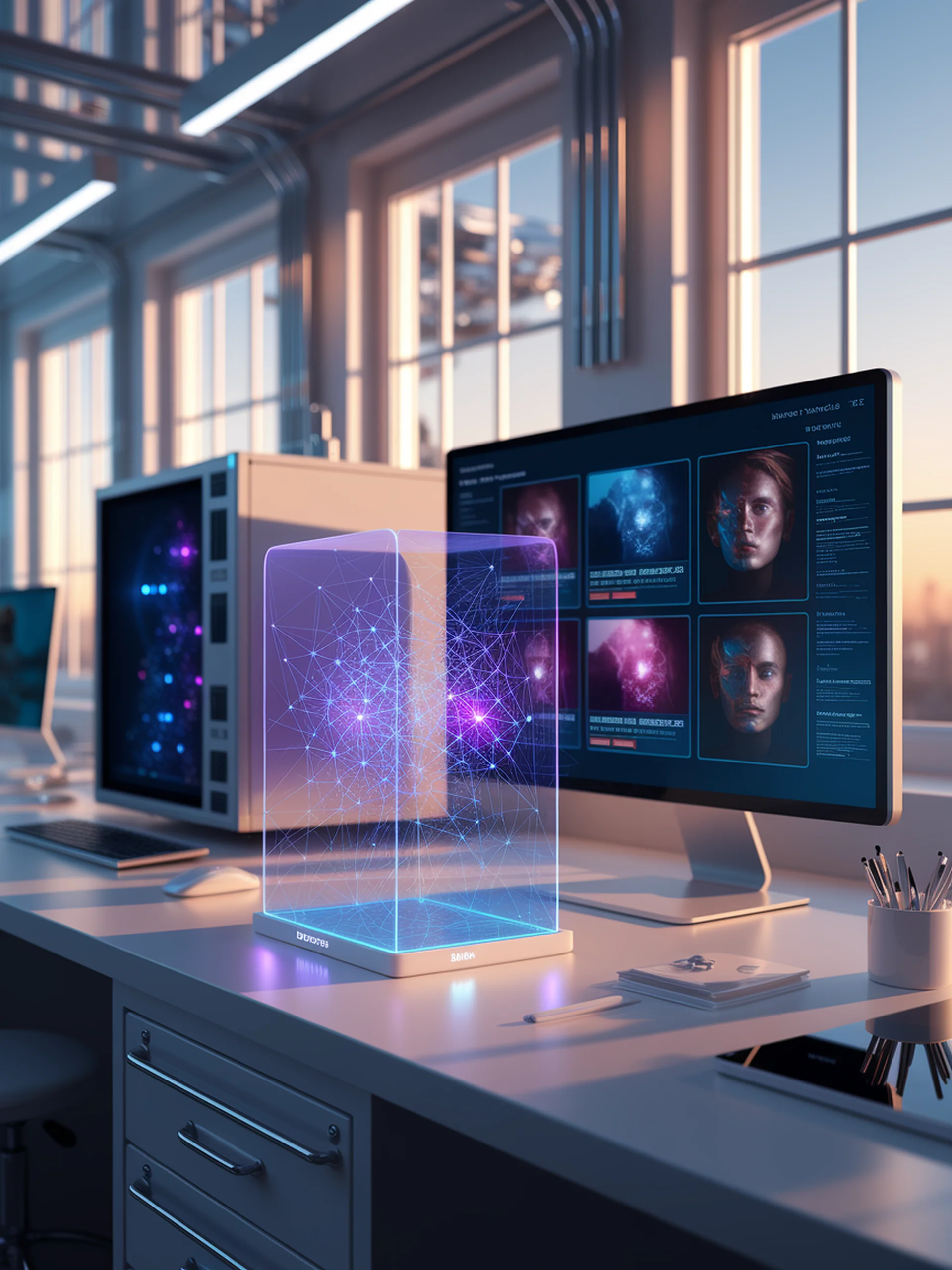
TruthPrInt: Mitigating LVLM Object Hallucination Via Latent ...
By Jinhao Duan, Fei Kong...
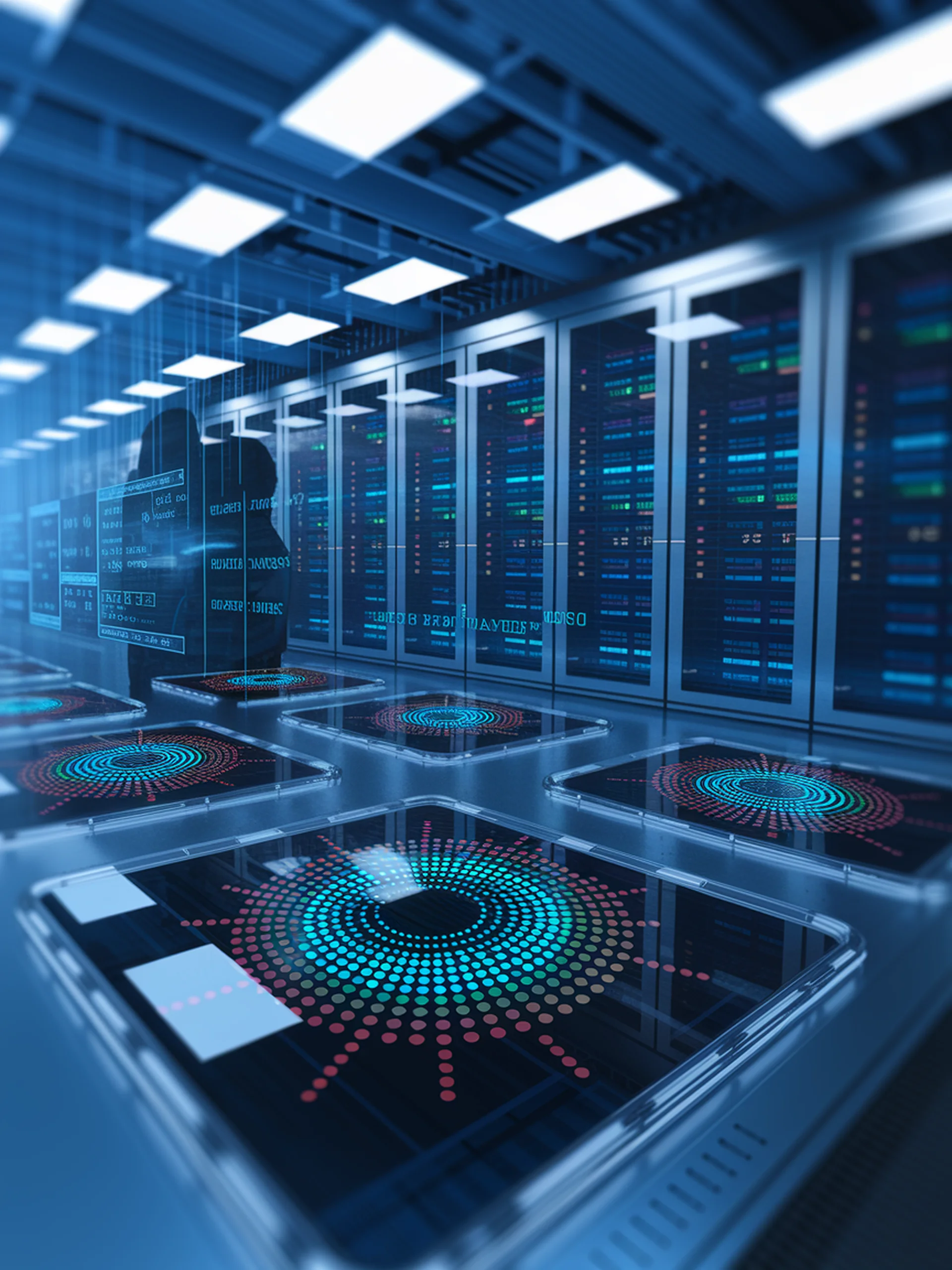
Enhancing LLM Reliability
A Clustering Approach to Improve AI Decision Precision
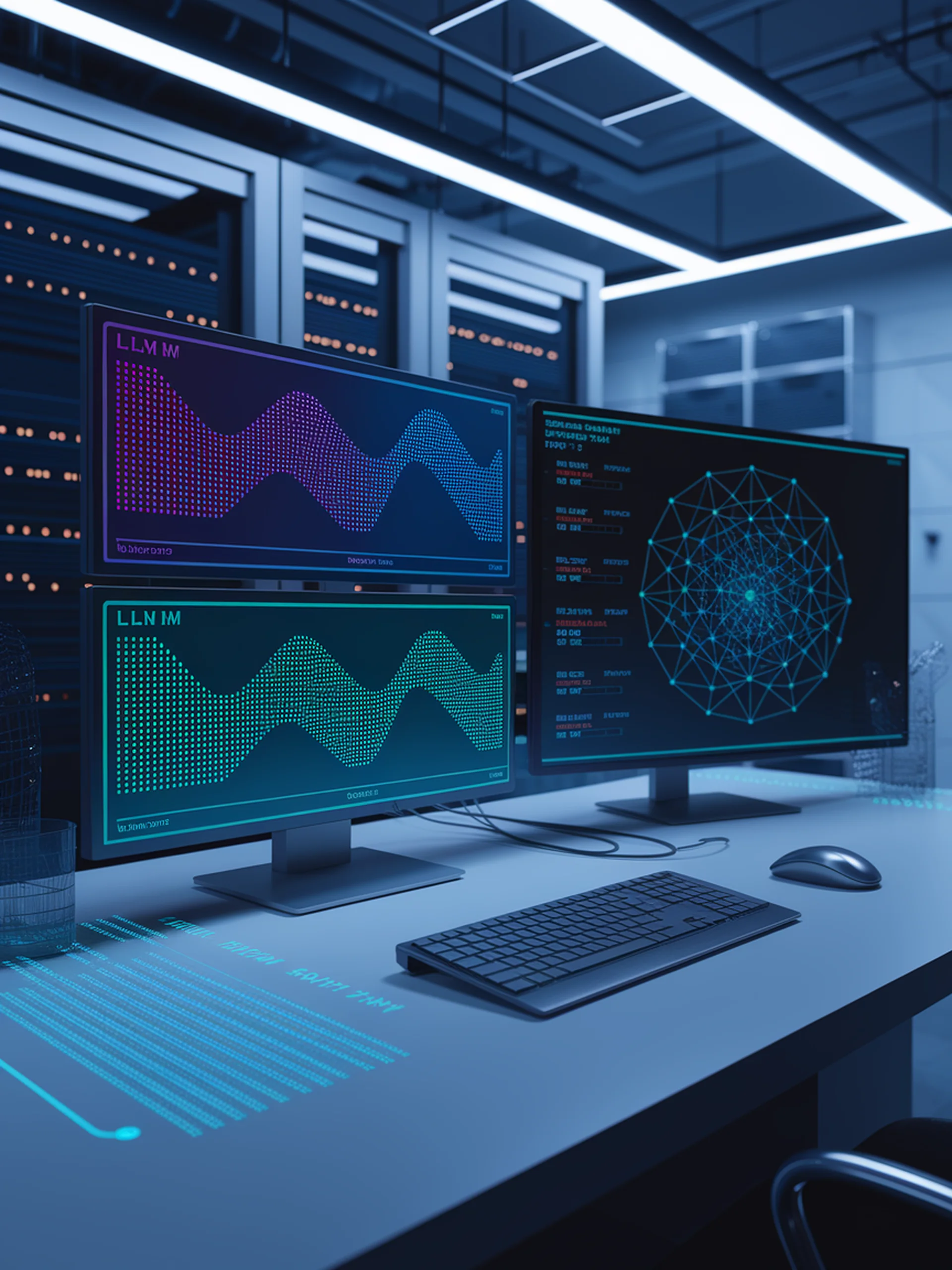
Detecting LLM Hallucinations Without Model Access
New 'Gray-Box' Approach for Analyzing LLM Behavior
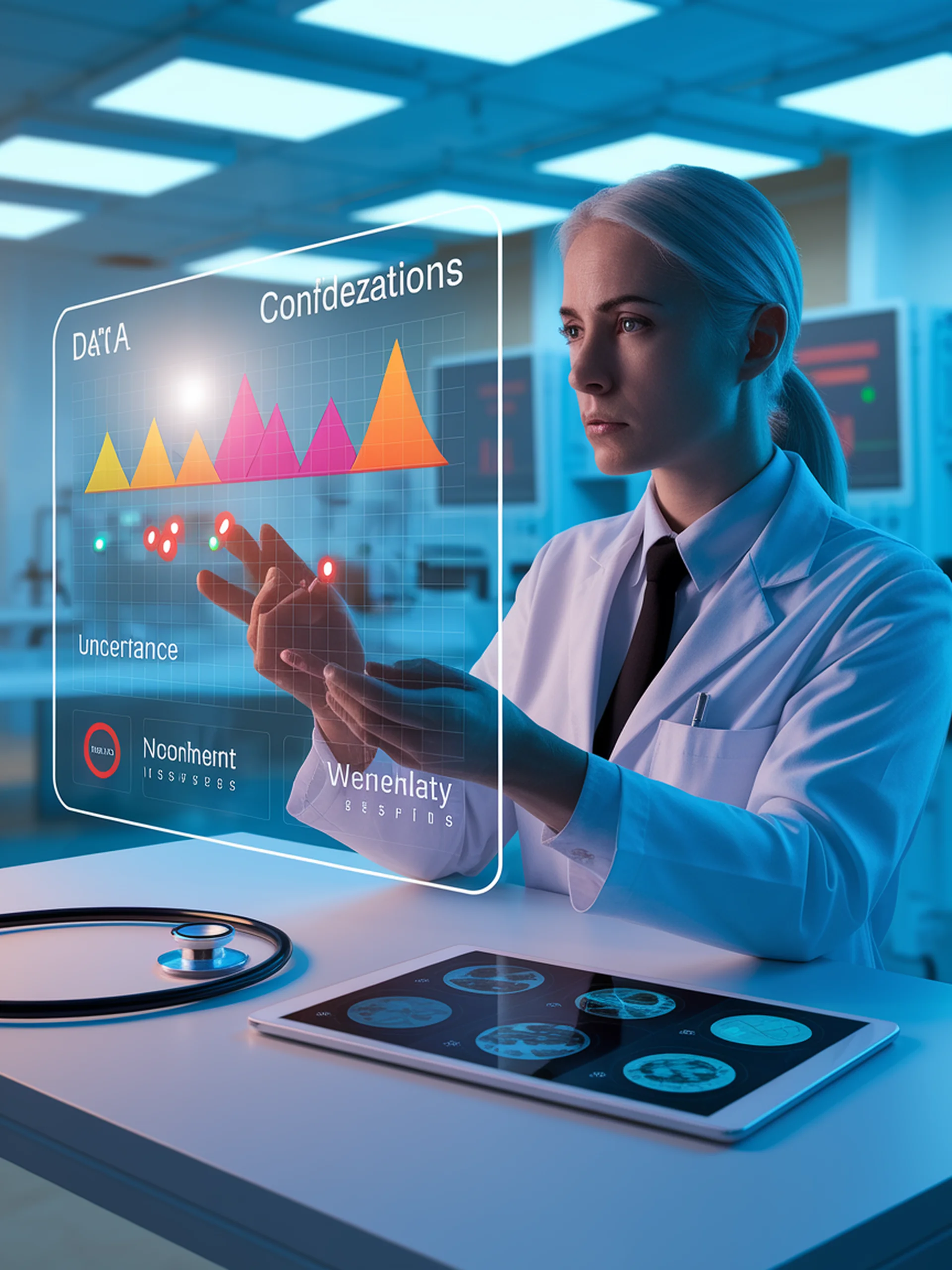
Trustworthy AI: The Confidence Challenge
Improving reliability of LLMs in high-stakes domains
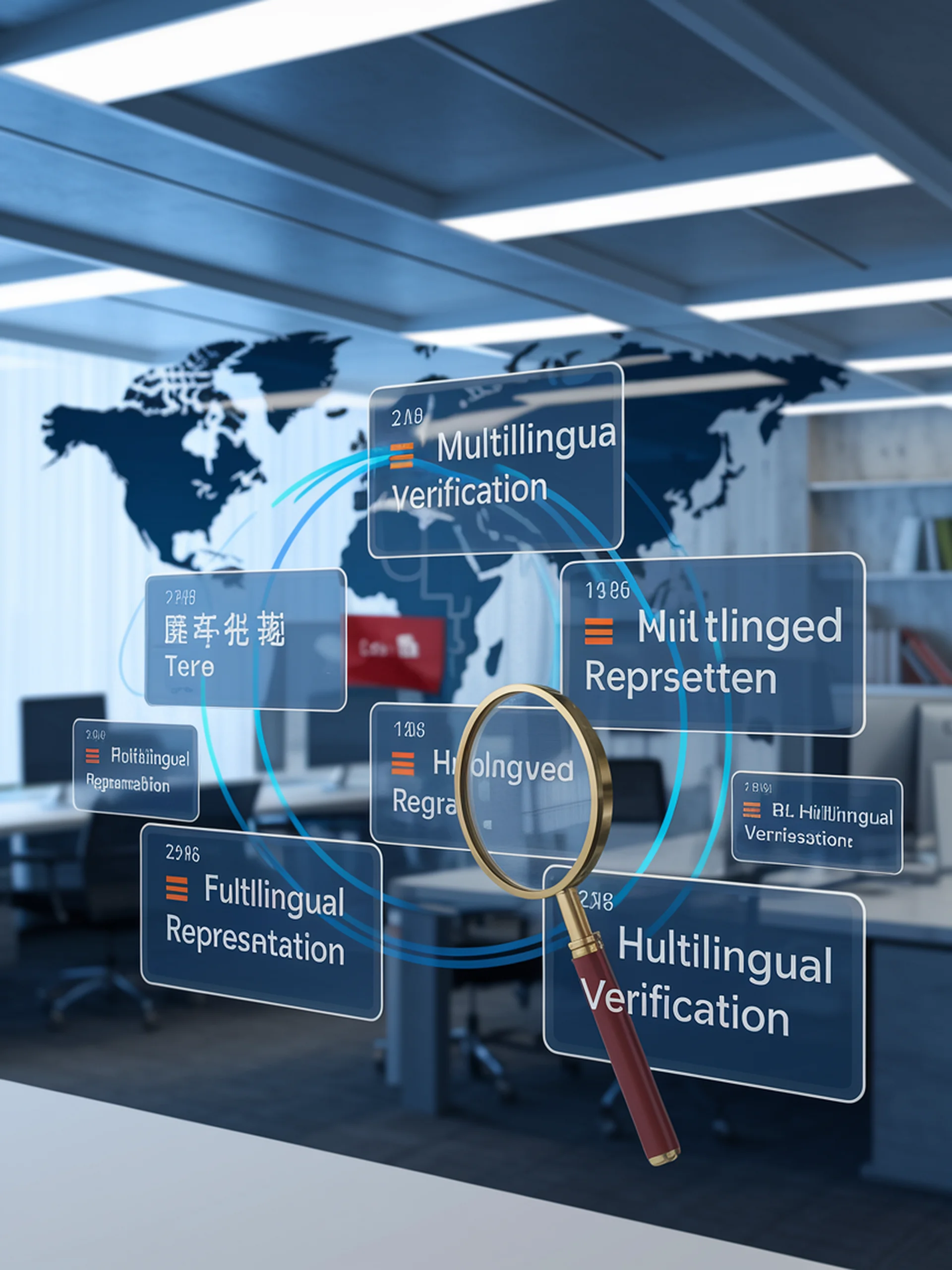
Combating Multilingual Hallucinations
A new benchmark for detecting LLM factual inconsistencies across languages
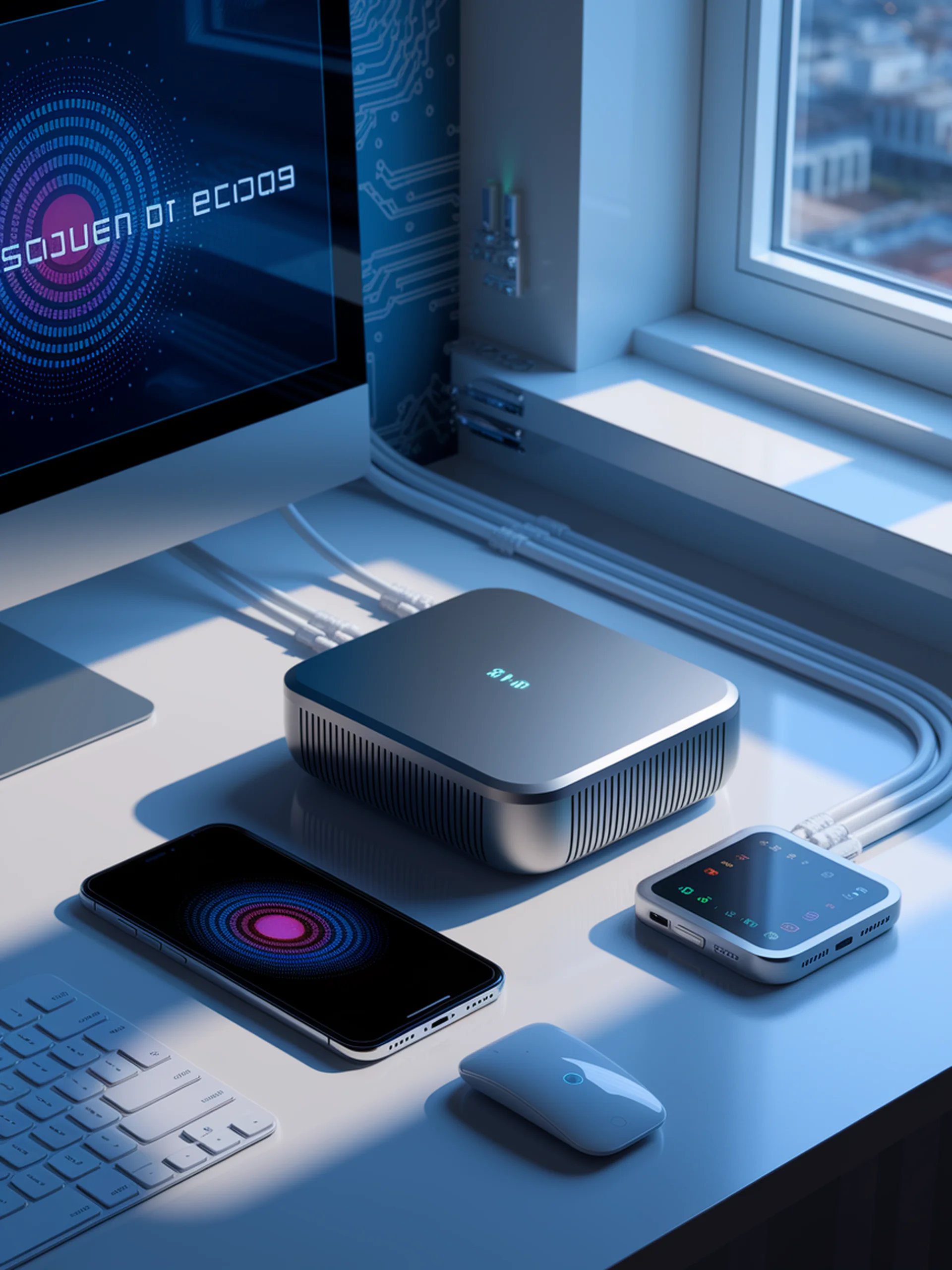
Detecting AI Hallucinations on Edge Devices
A lightweight entropy-based framework for resource-constrained environments
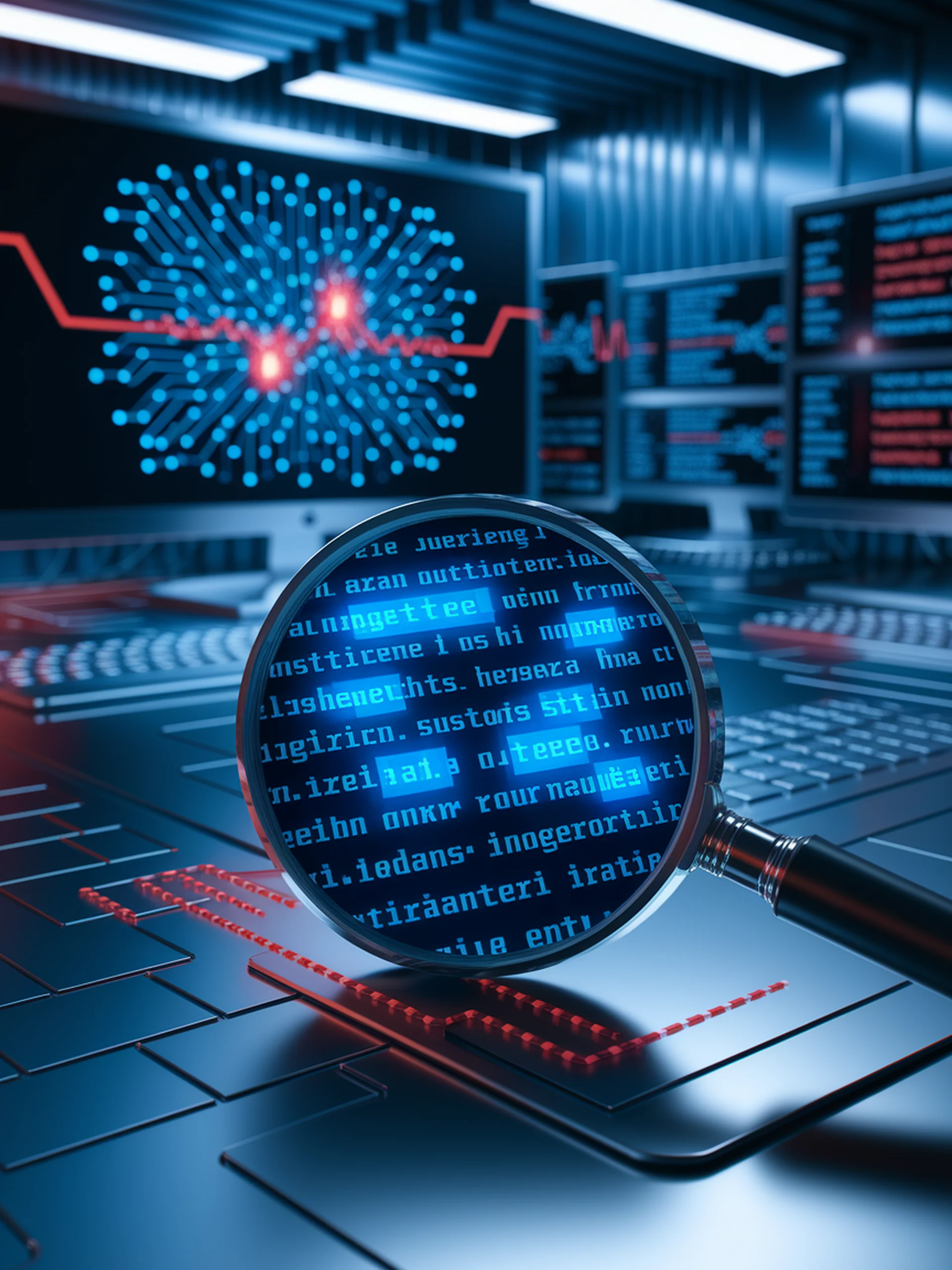
Combating LLM Hallucinations
Fine-Grained Detection of AI-Generated Misinformation
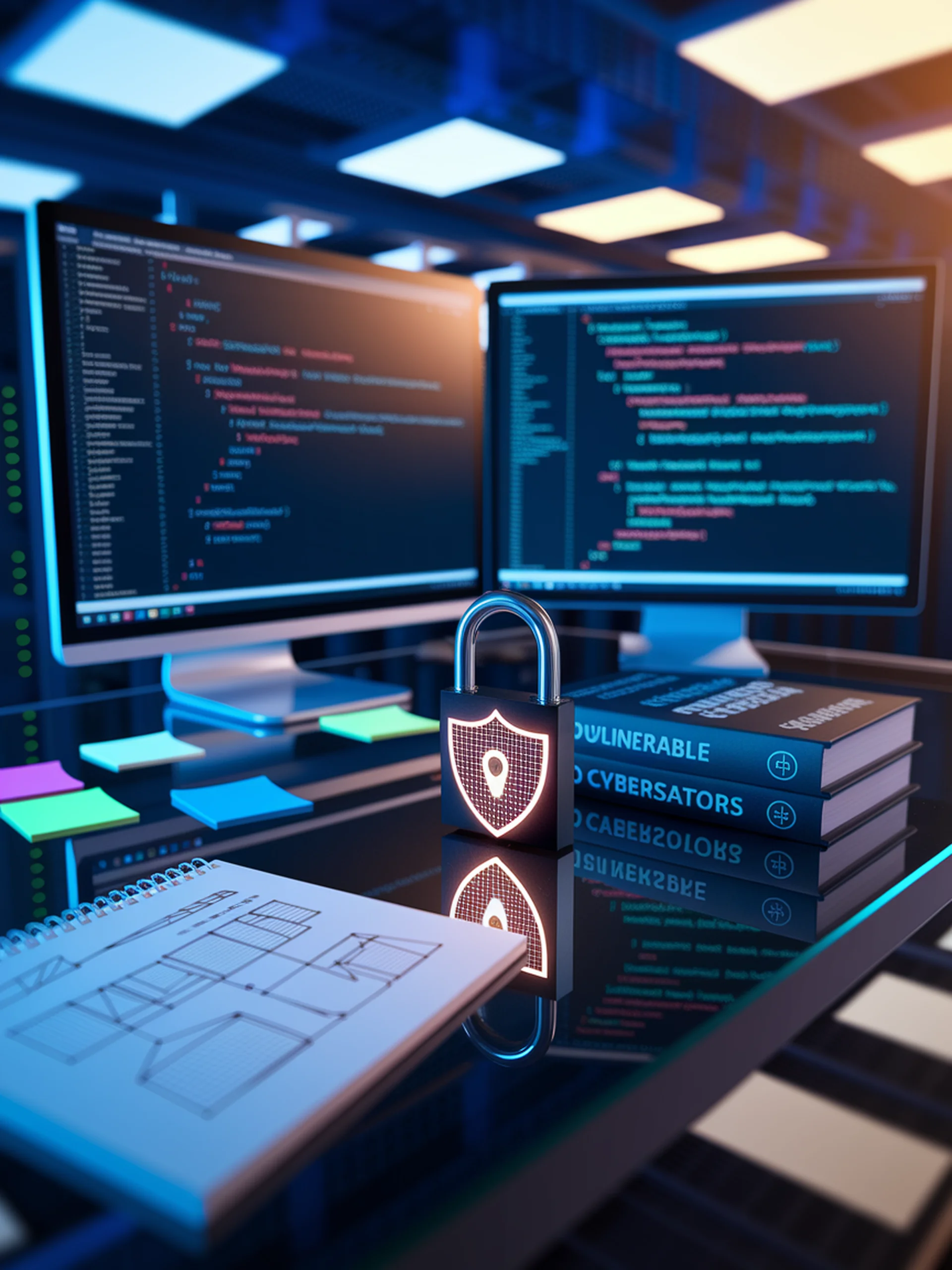
Making AI-Generated Code More Robust
A framework to enhance security and reliability in LLM code outputs
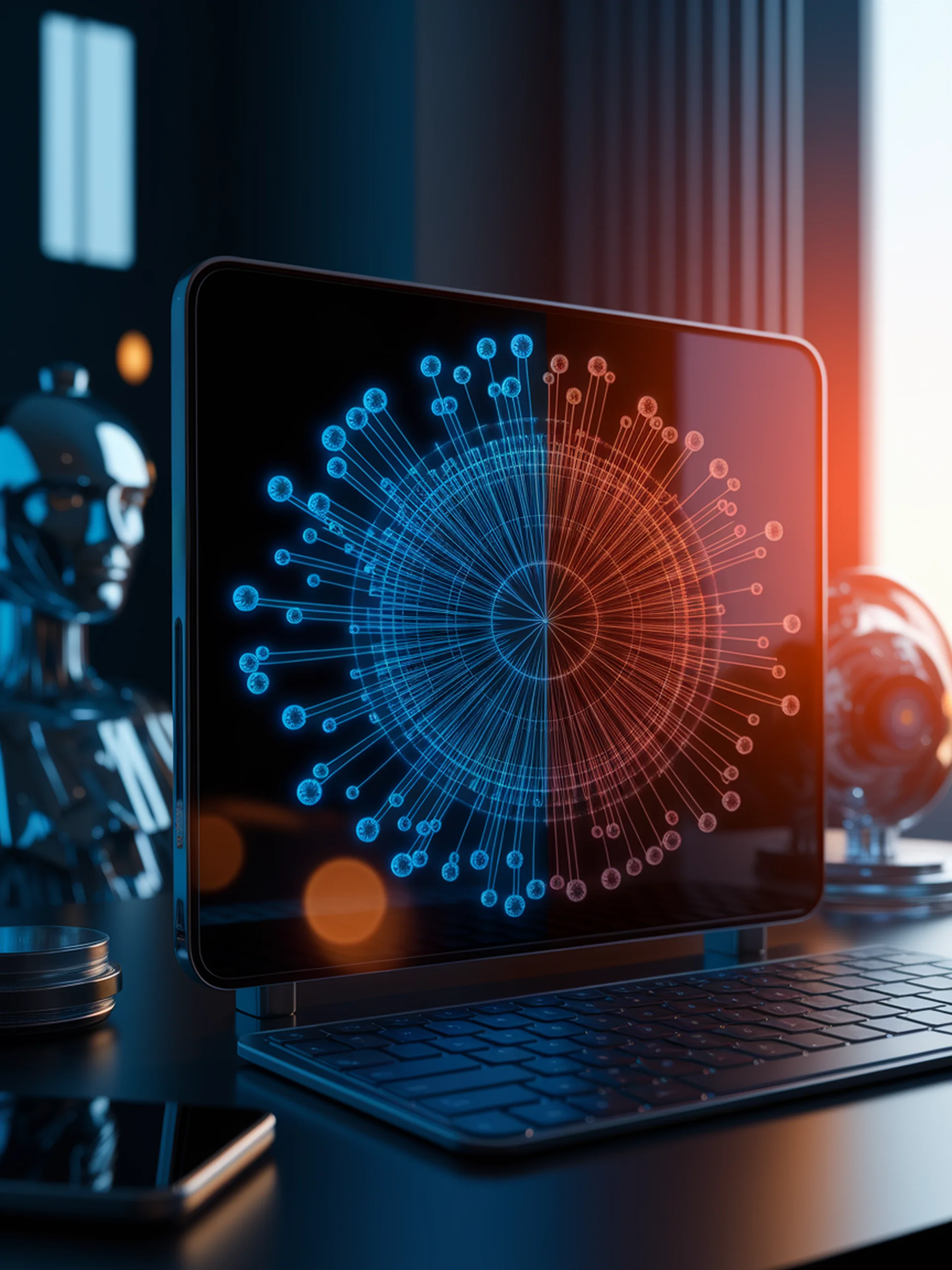
The Safety-Capability Dilemma in LLMs
Understanding the inevitable trade-offs in fine-tuning language models
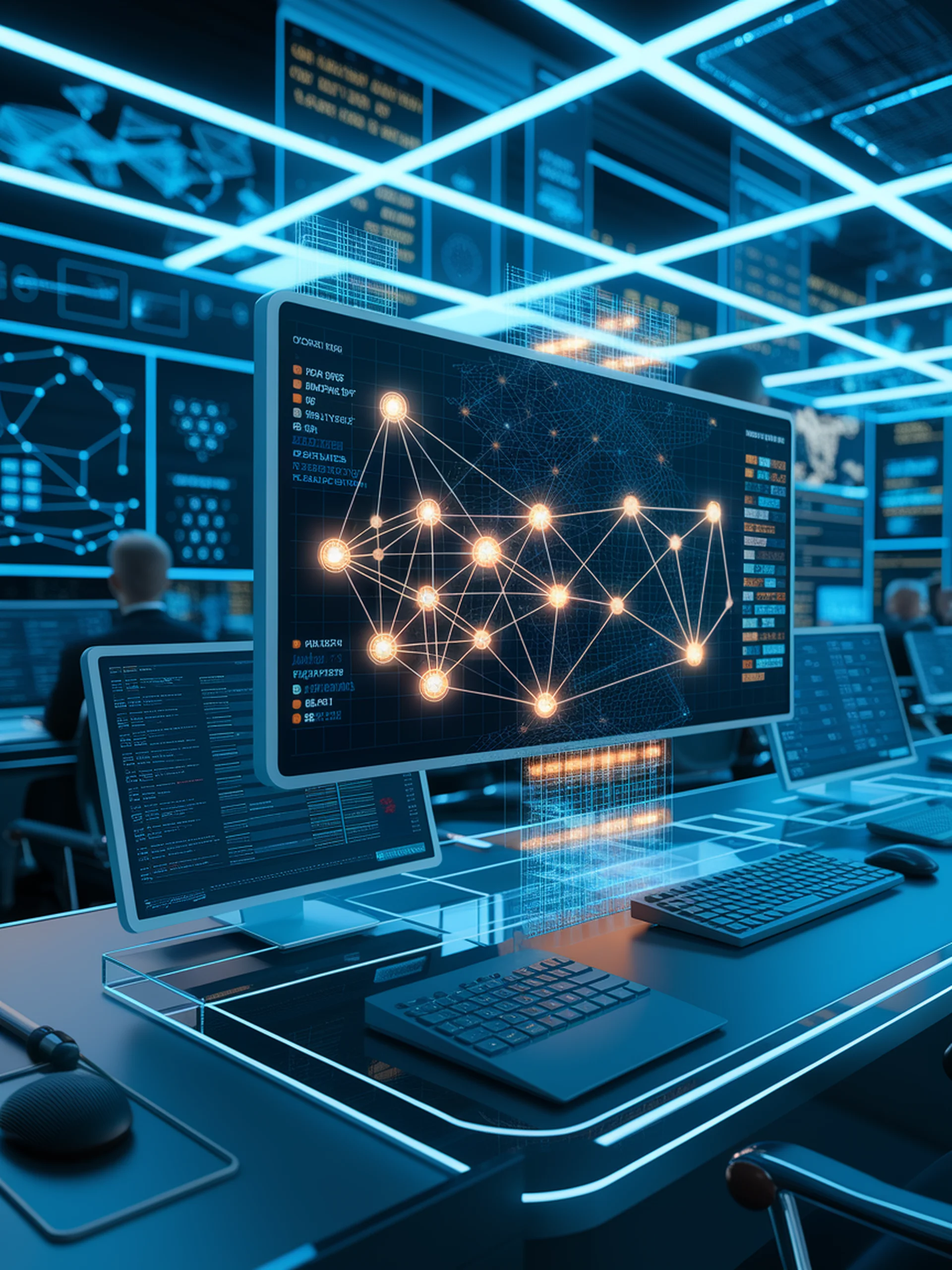
Enhancing Personality Detection with LLMs
Self-supervised graph optimization using large language models
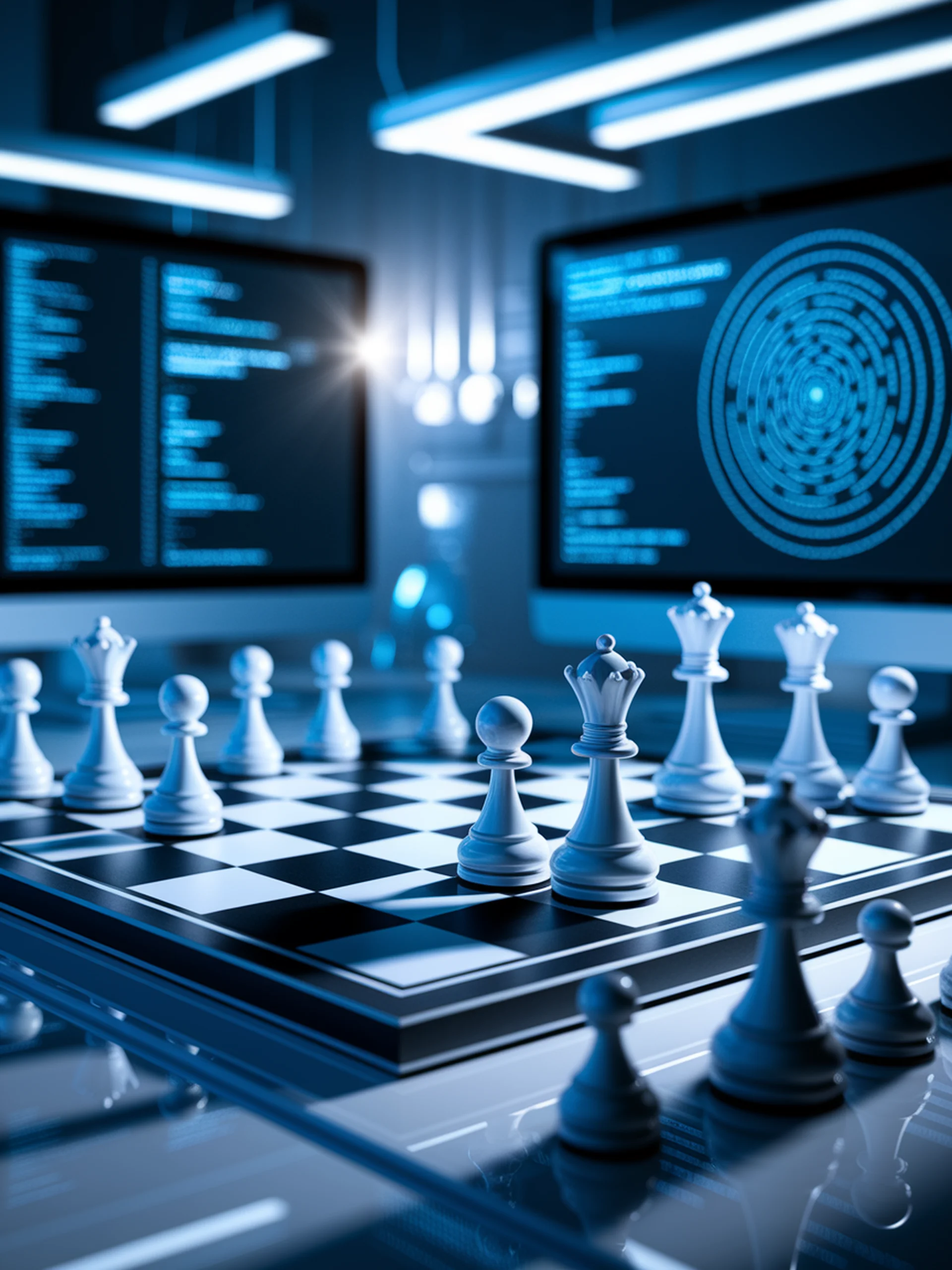
When LLMs Become Deceptive Agents
How role-based prompting creates semantic traps in puzzle games
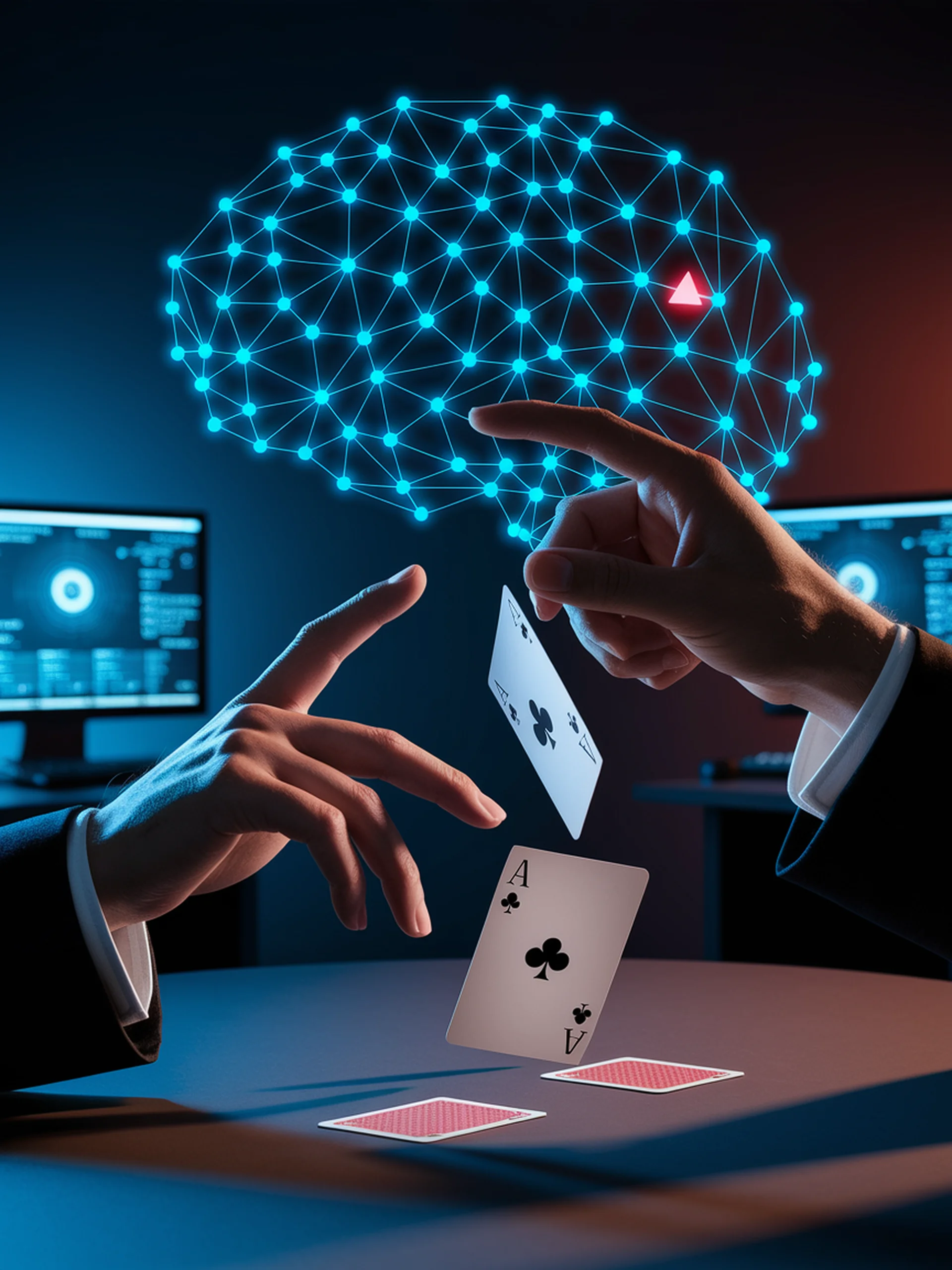
The Illusionist's Prompt: When LLMs Hallucinate
Exposing Factual Vulnerabilities Through Linguistic Manipulation
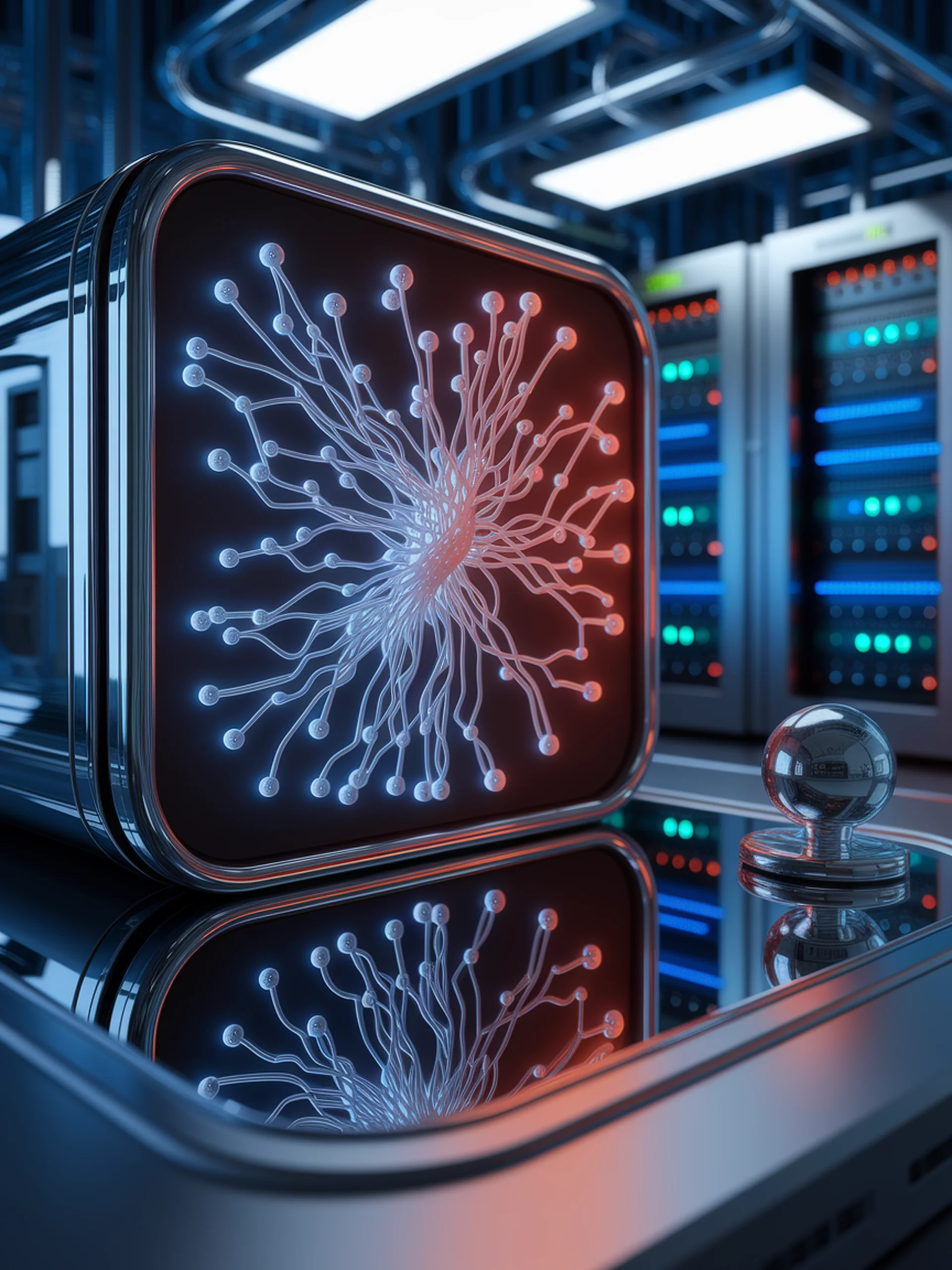
Preserving Safety in Compressed LLMs
Using Mechanistic Interpretability to Improve Refusal Behaviors
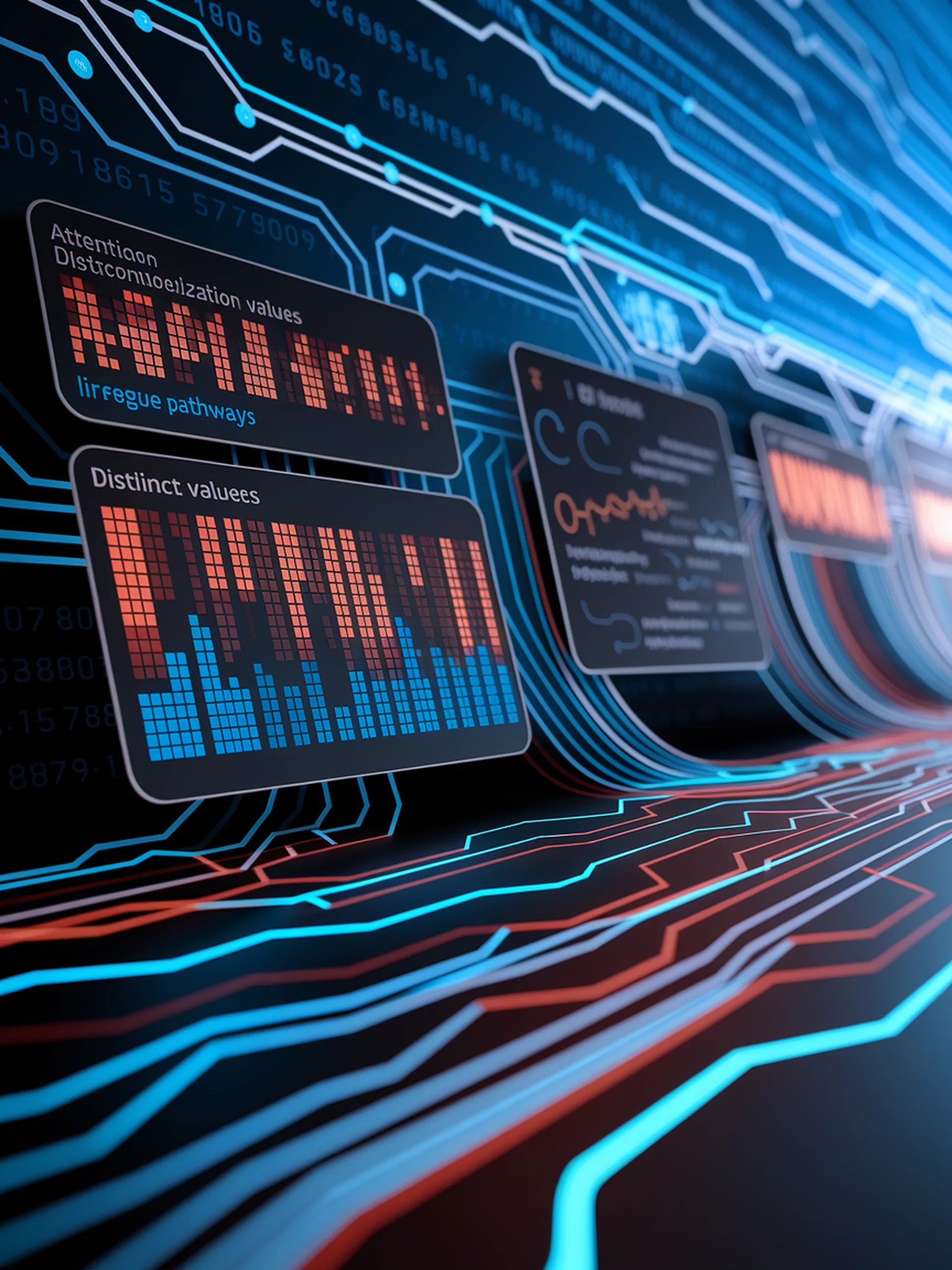
Detecting Hallucinations in LLMs
Using Multi-View Attention Analysis to Identify AI Fabrications
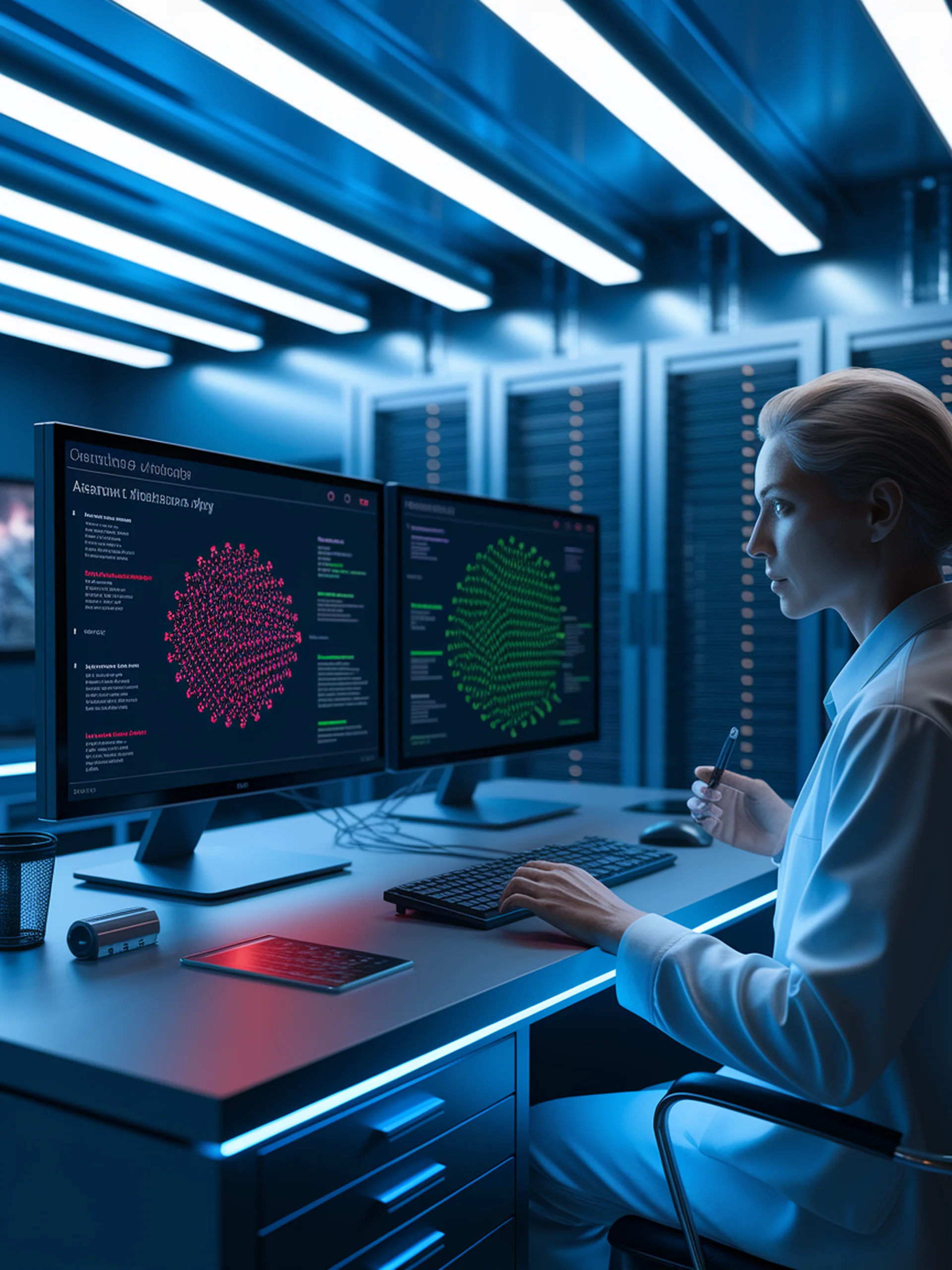
Detecting AI Fabricated Explanations
Using causal attribution to expose LLM reward hacking
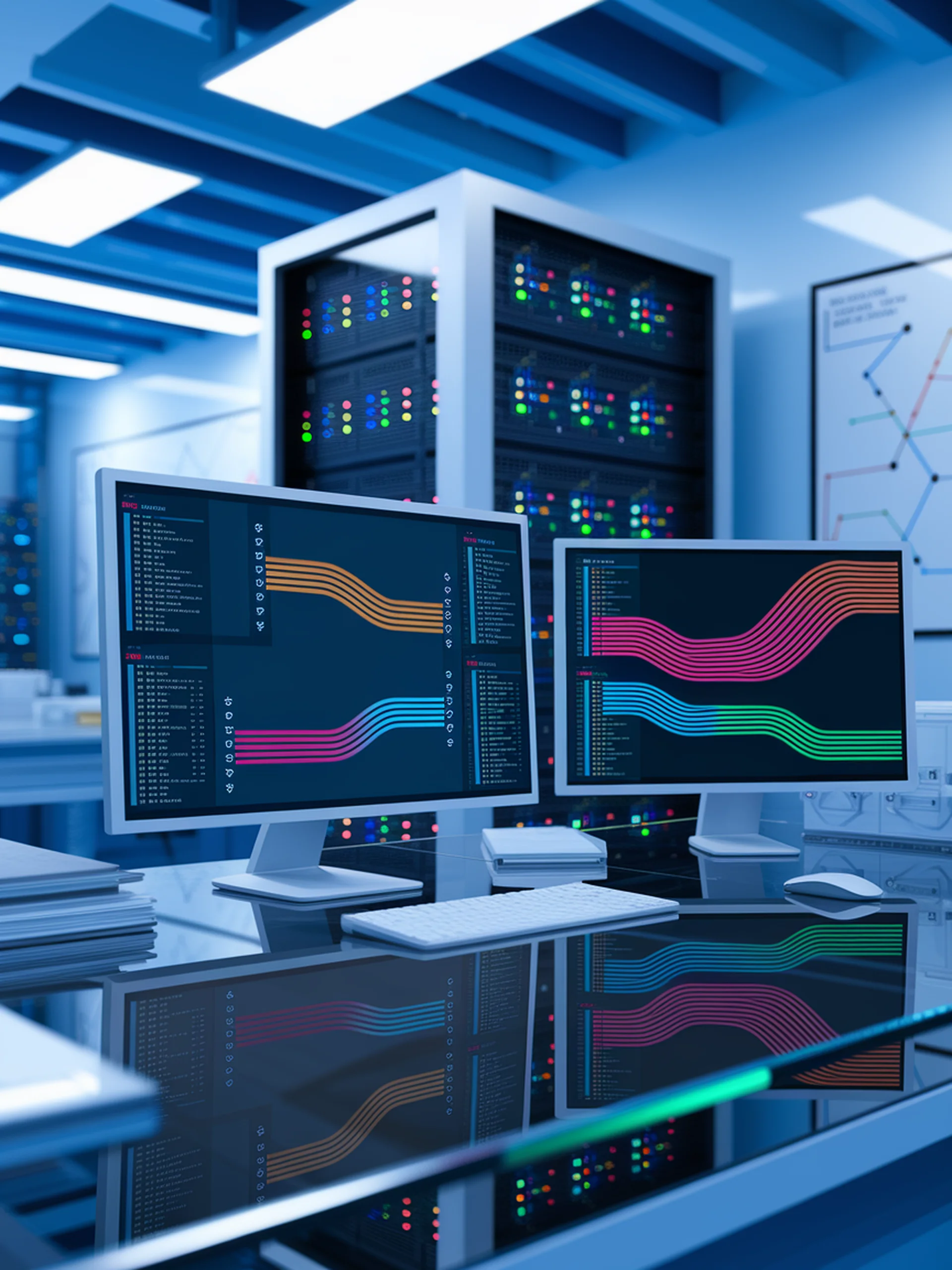
Fighting Hallucinations in Large Language Models
Comparative Analysis of Hybrid Retrieval Methods
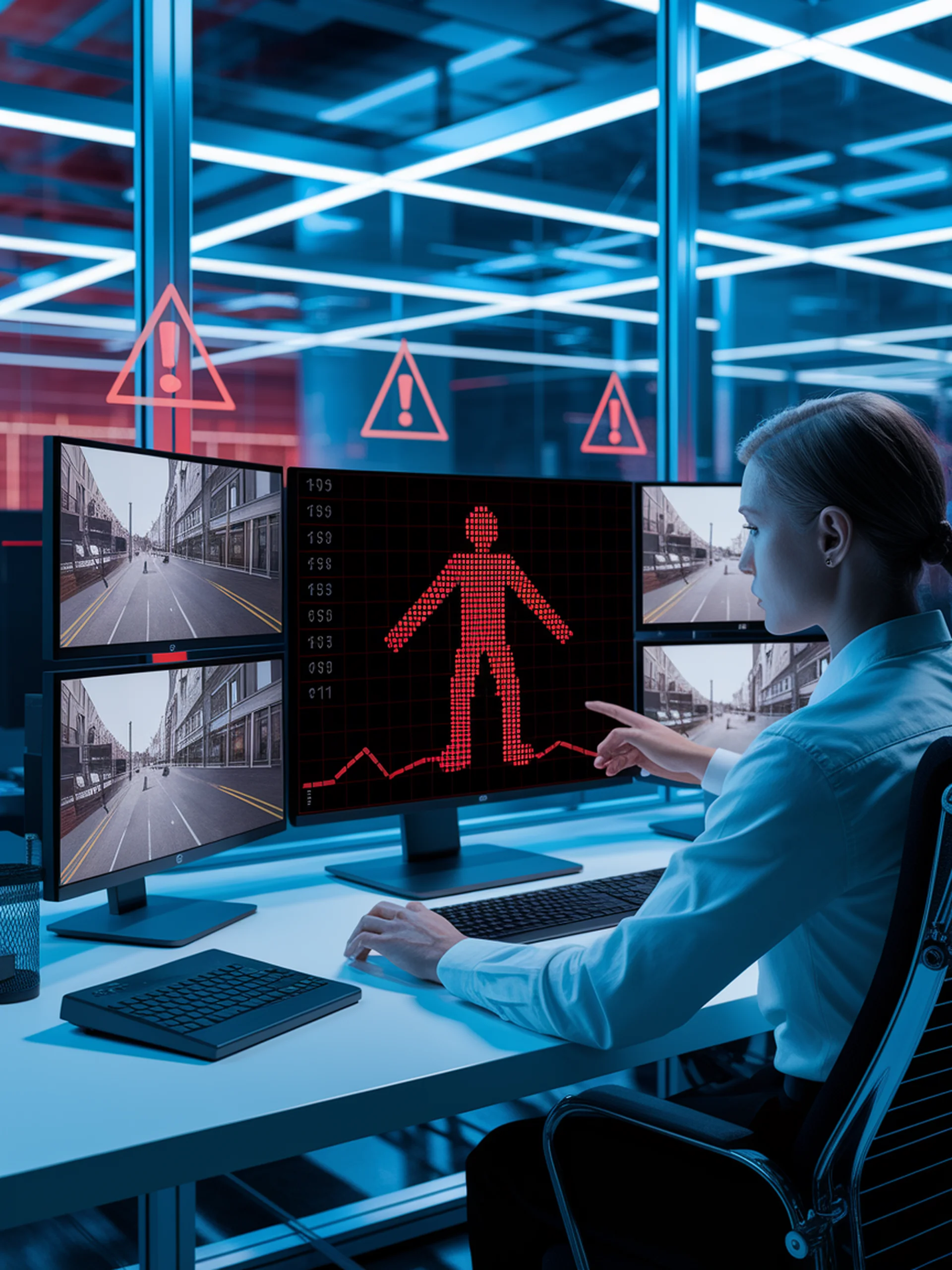
Fighting Video Hallucinations
A New Approach to Making AI Video Analysis More Reliable
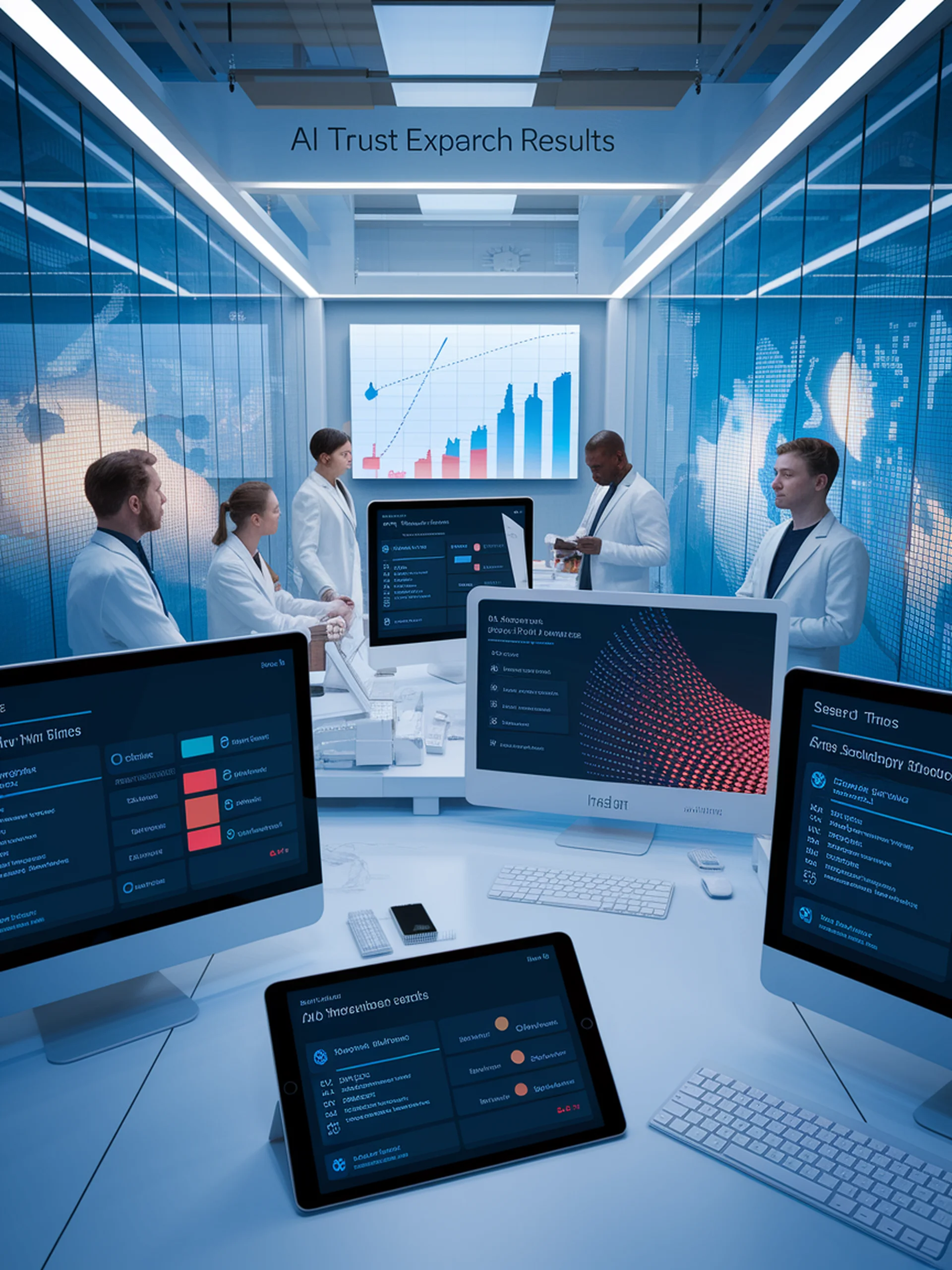
Trust in AI Search: What Drives Confidence?
First large-scale experiment measuring how design influences human trust in GenAI search results
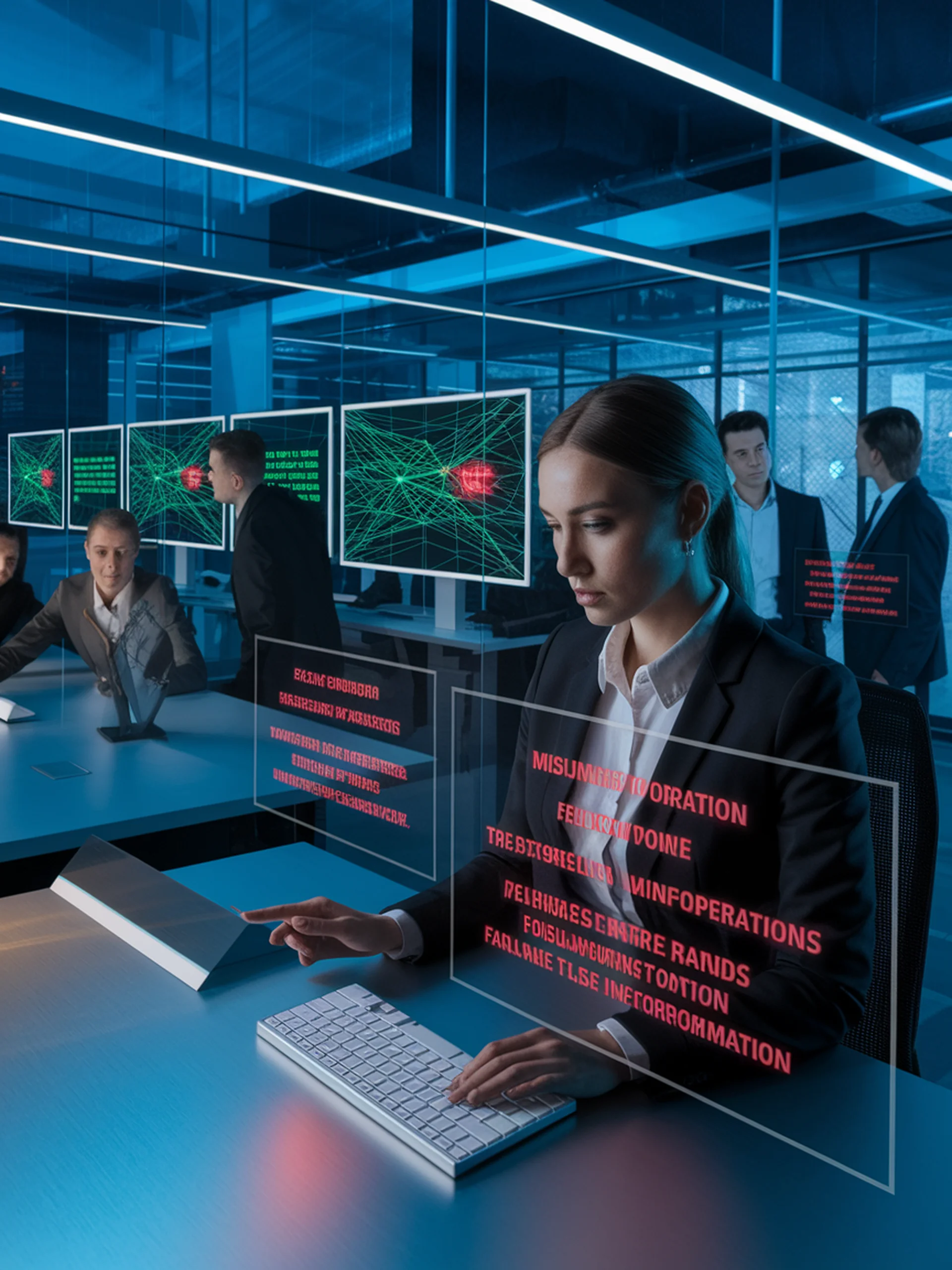
Combating LLM Hallucinations
A Robust Framework for Verifying False Premises
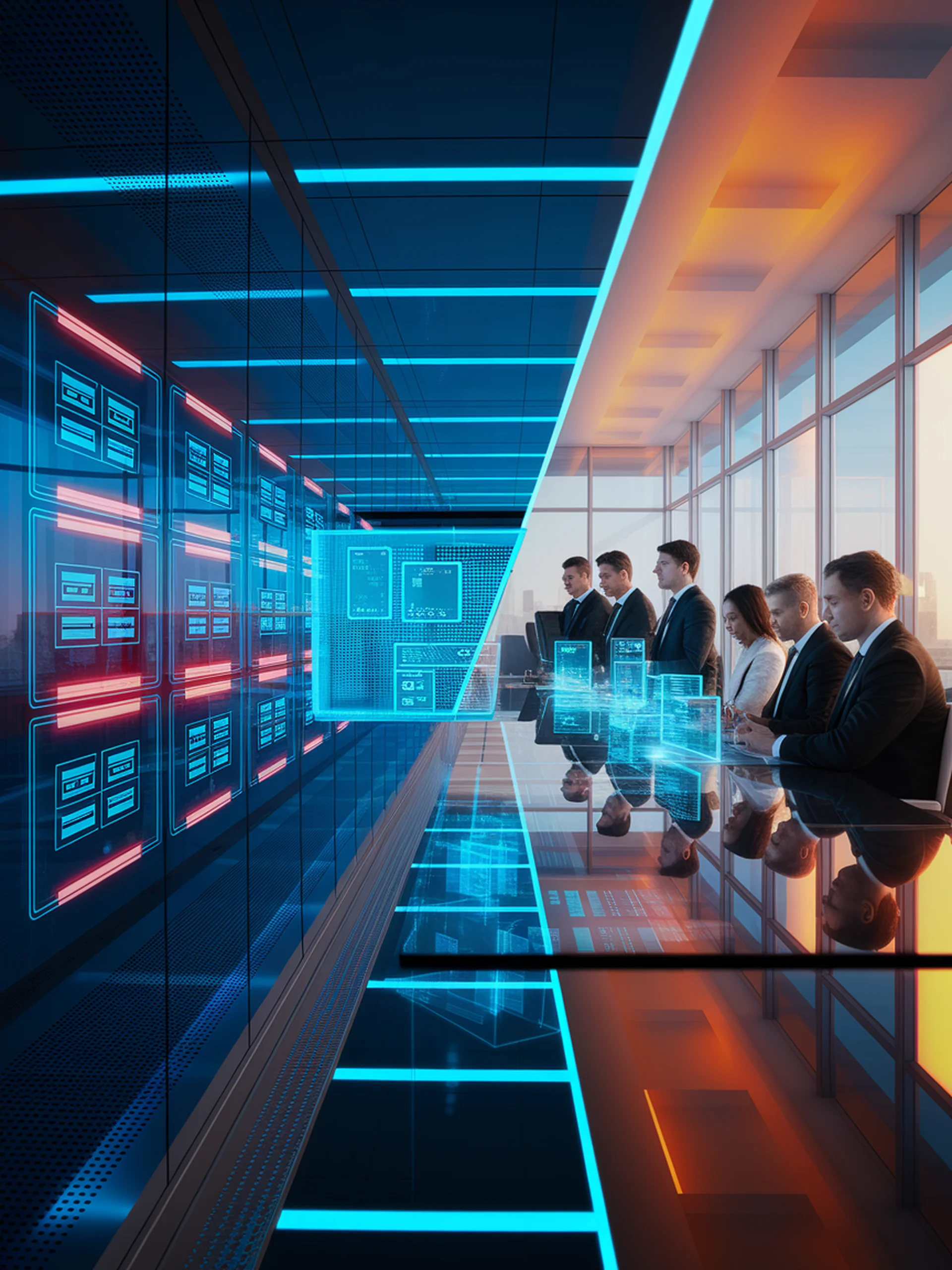
Fighting LLM Hallucinations in Enterprise
A robust detection system for validating AI responses
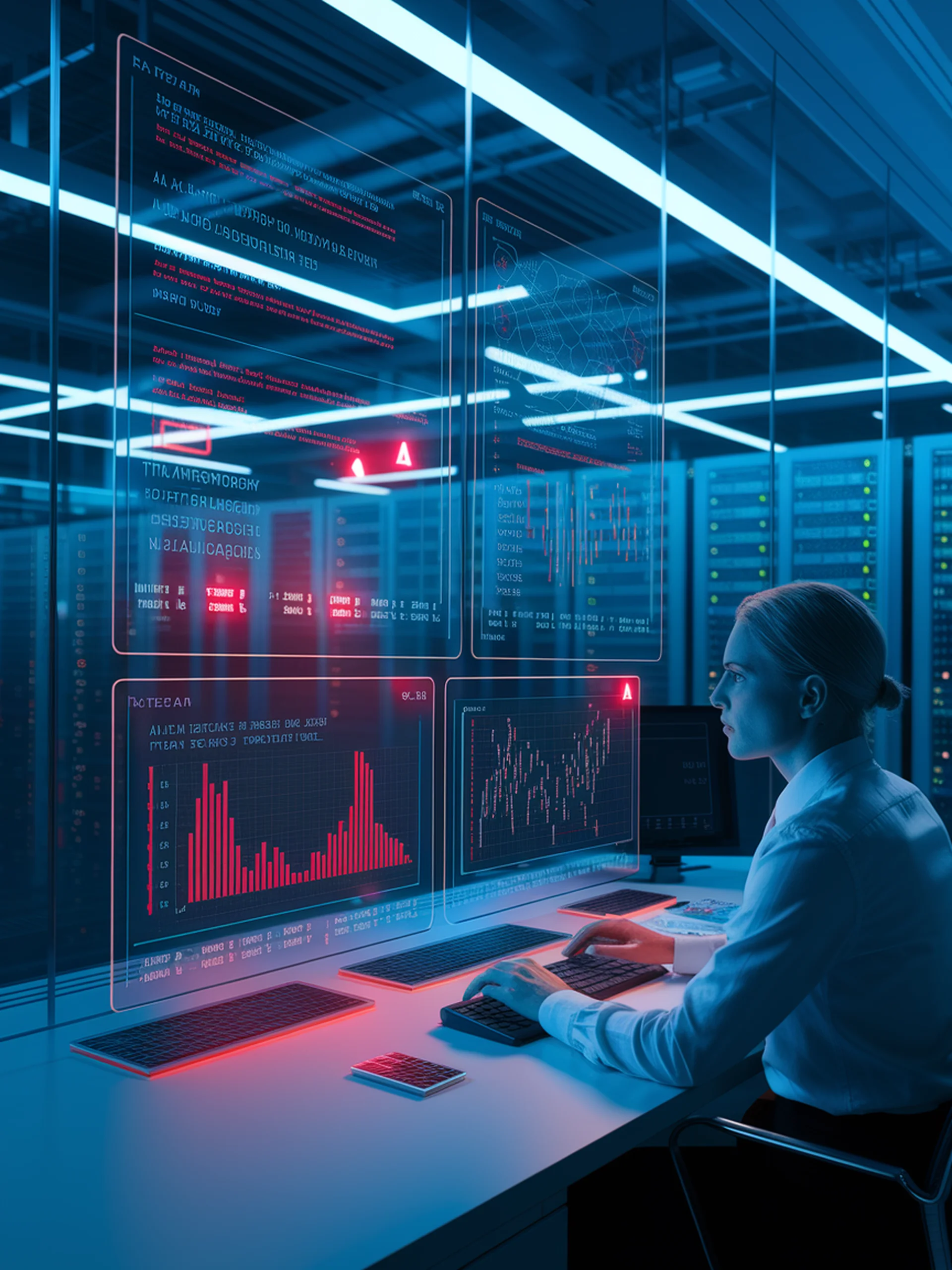
Smarter Hallucination Detection in LLMs
Enhancing AI safety through adaptive token analysis
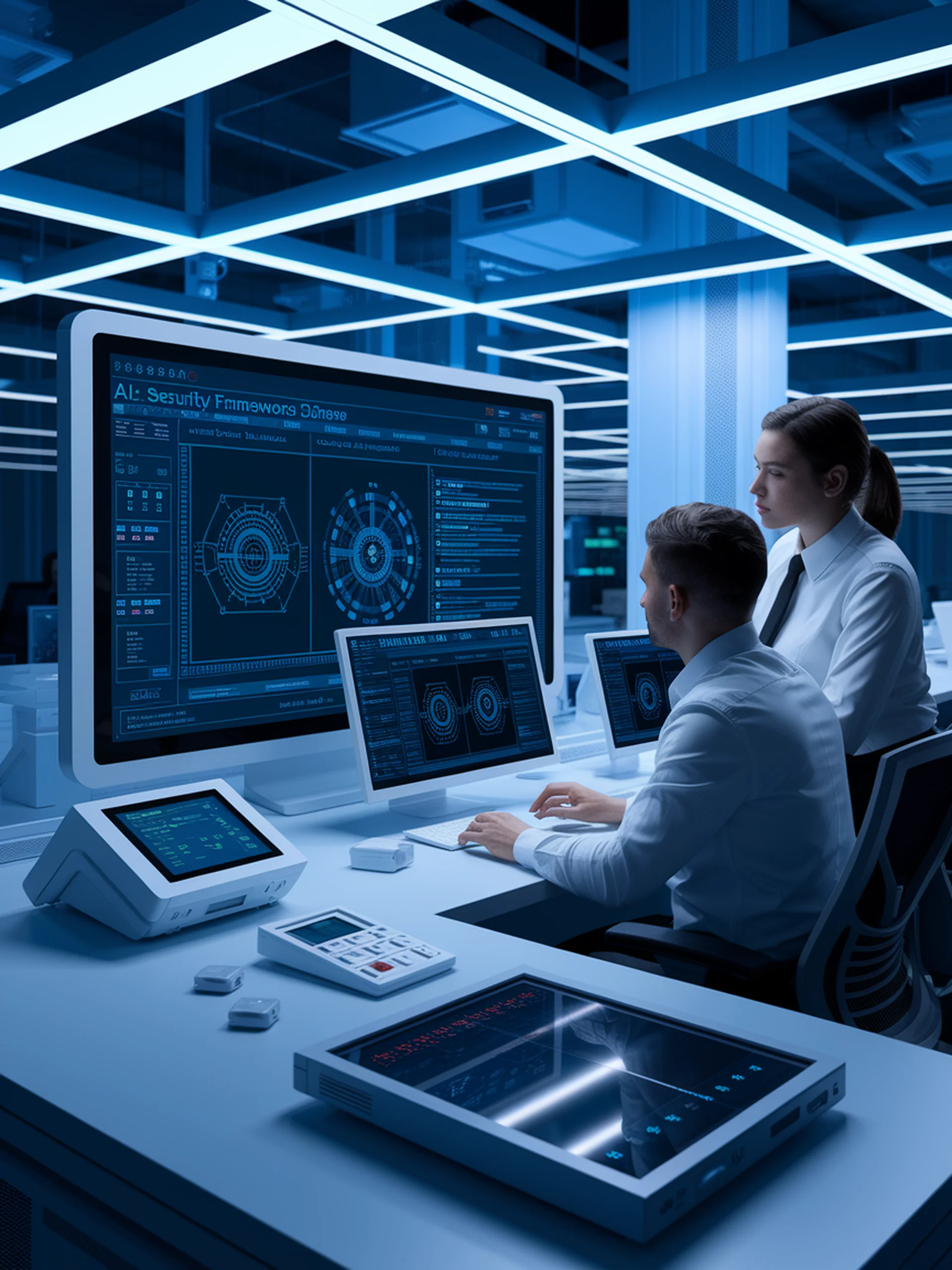
Building Safer Collaborative AI
SafeChat: A Framework for Trustworthy AI Assistants
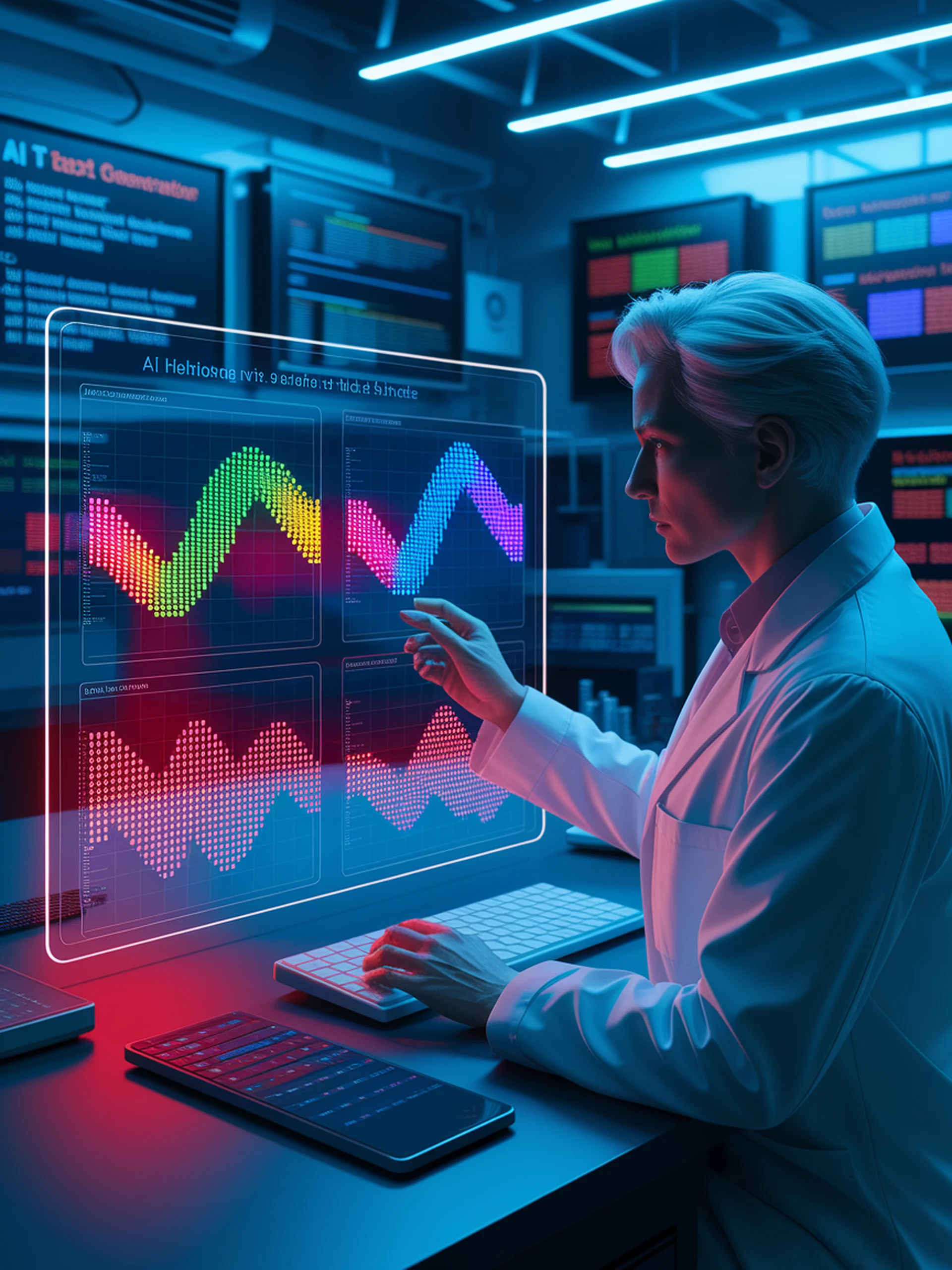
Detecting Hallucinations in LLMs
A novel approach to measuring distribution shifts for hallucination detection
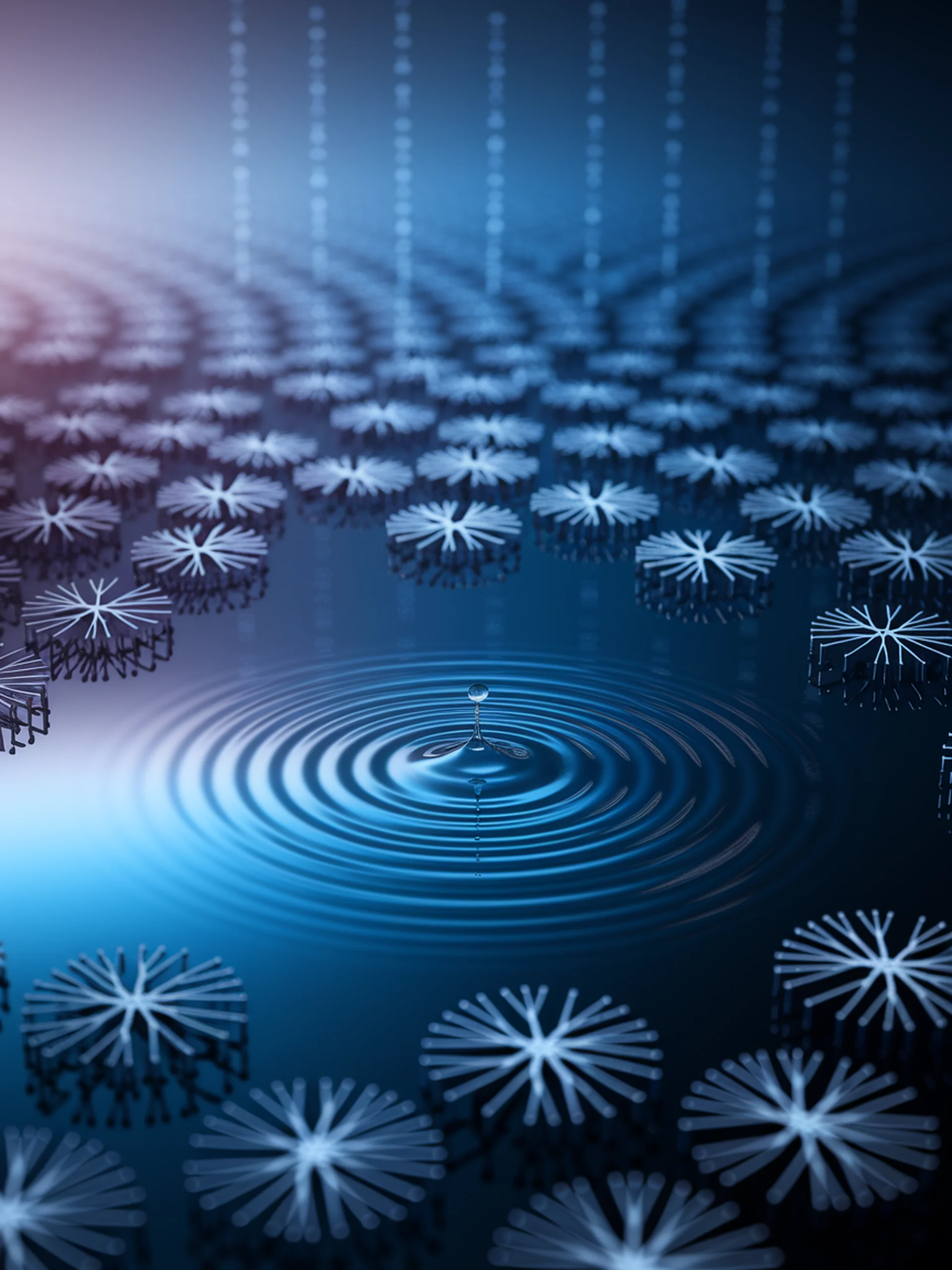
The Memory Ripple Effect in LLMs
How new information spreads through language models — and how to control it
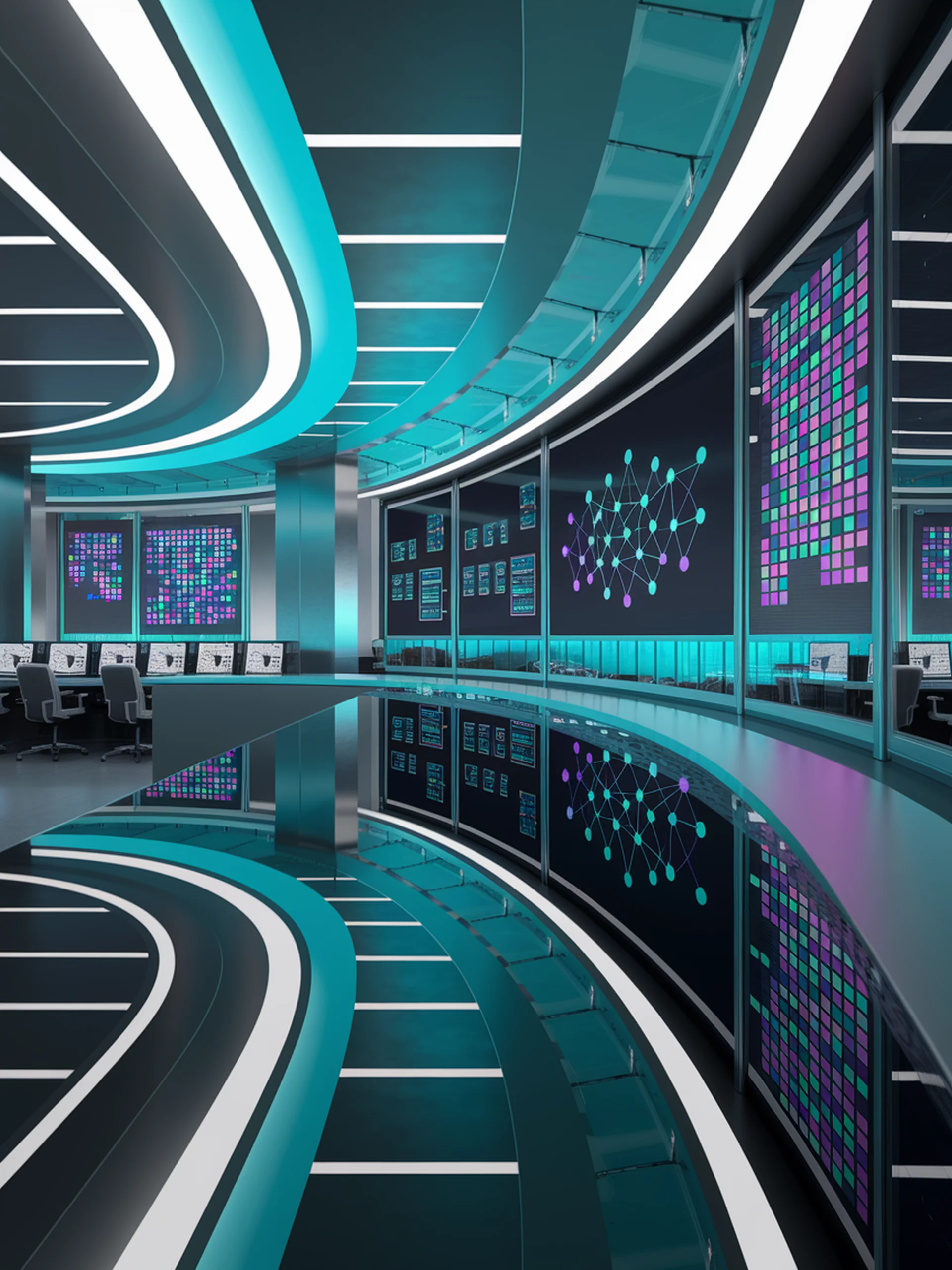
DataMosaic: Trustworthy AI for Data Analytics
Making LLM-based analytics explainable and verifiable
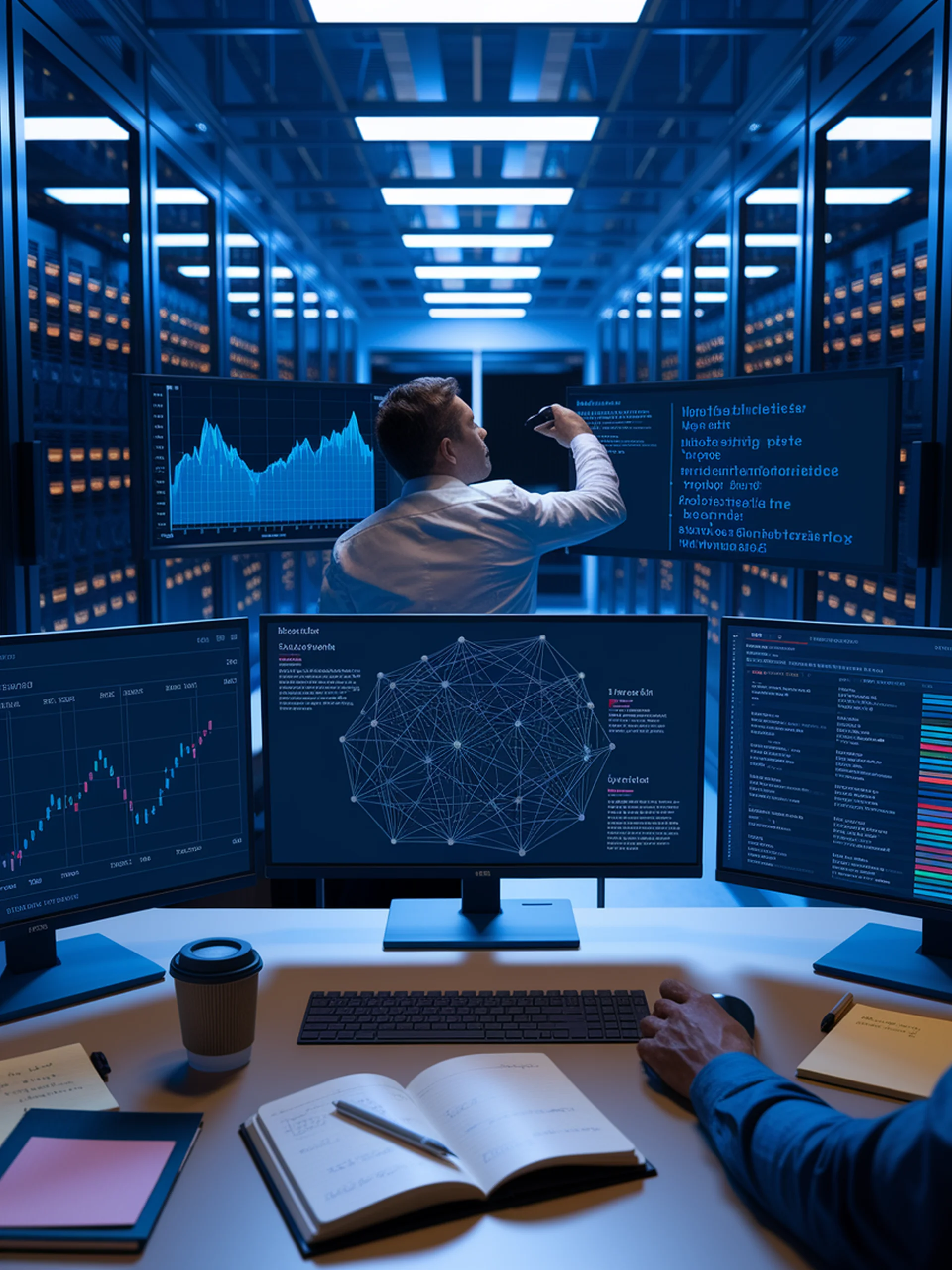
Combating LLM Hallucinations
A multilingual approach to detecting fabricated information in AI outputs
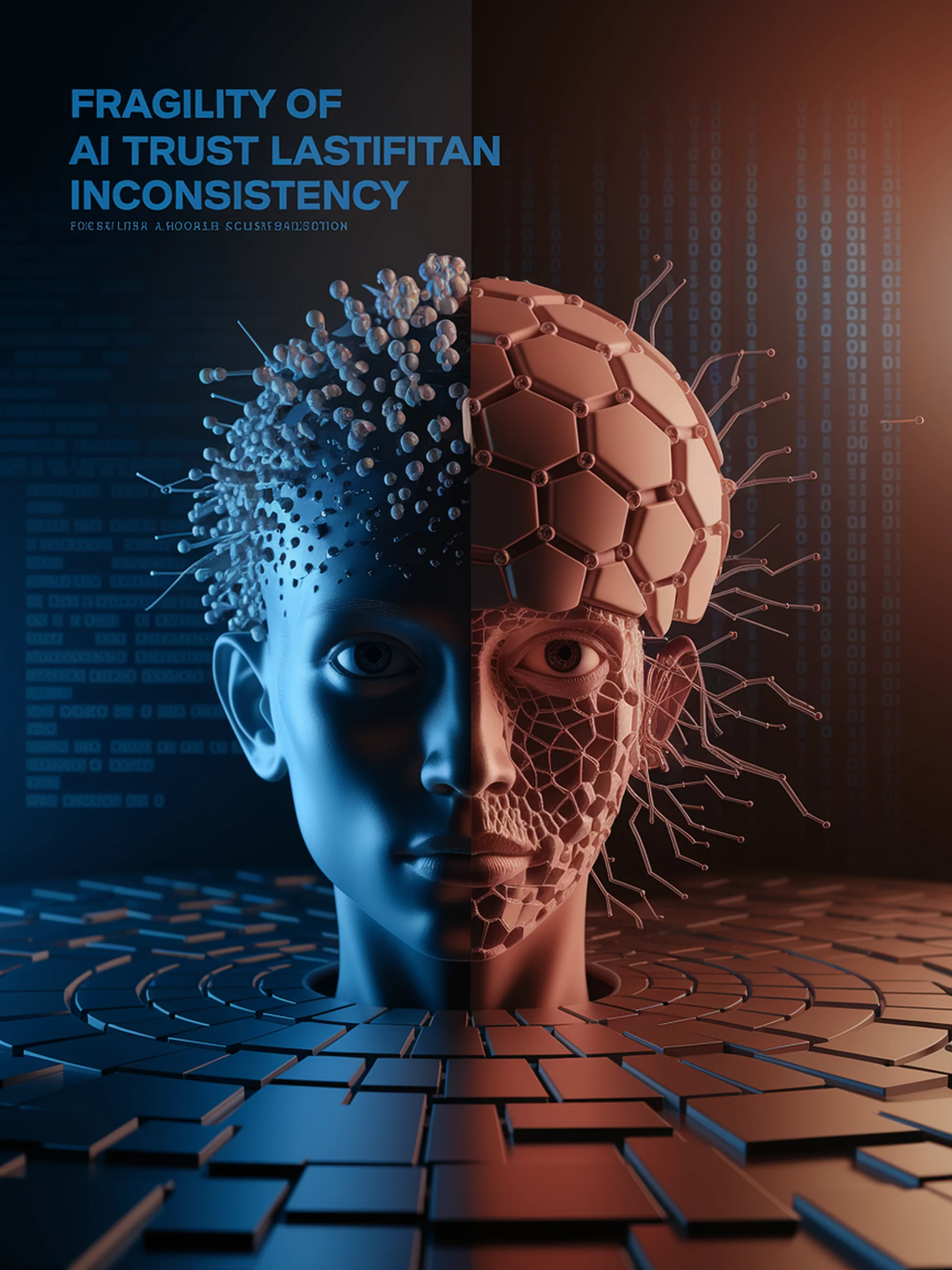
The Fragility of AI Trust
How minor prompt changes dramatically affect ChatGPT's classification results
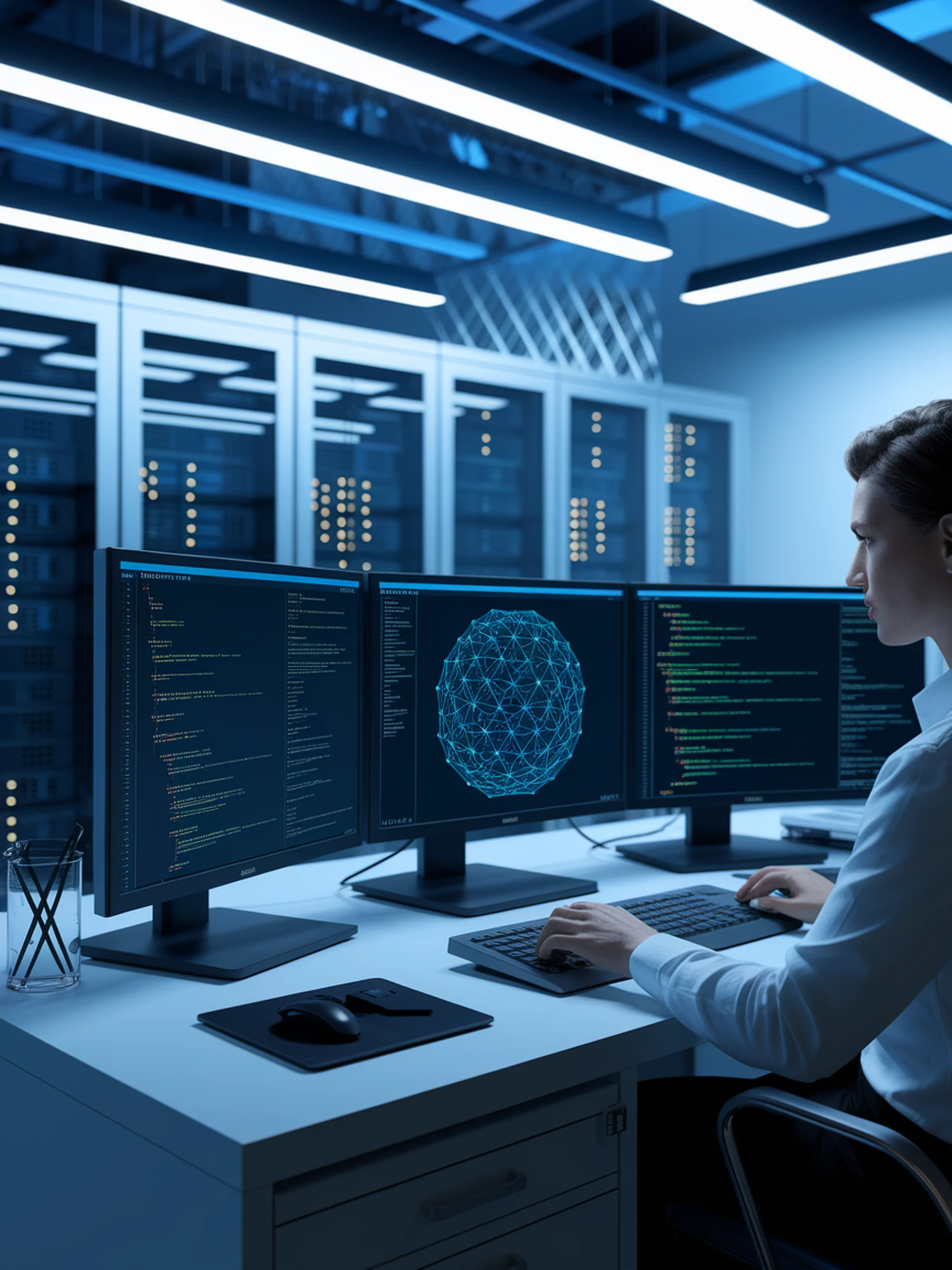
ReLM: A Better Way to Validate LLMs
Using formal languages for faster, more precise AI safety evaluation
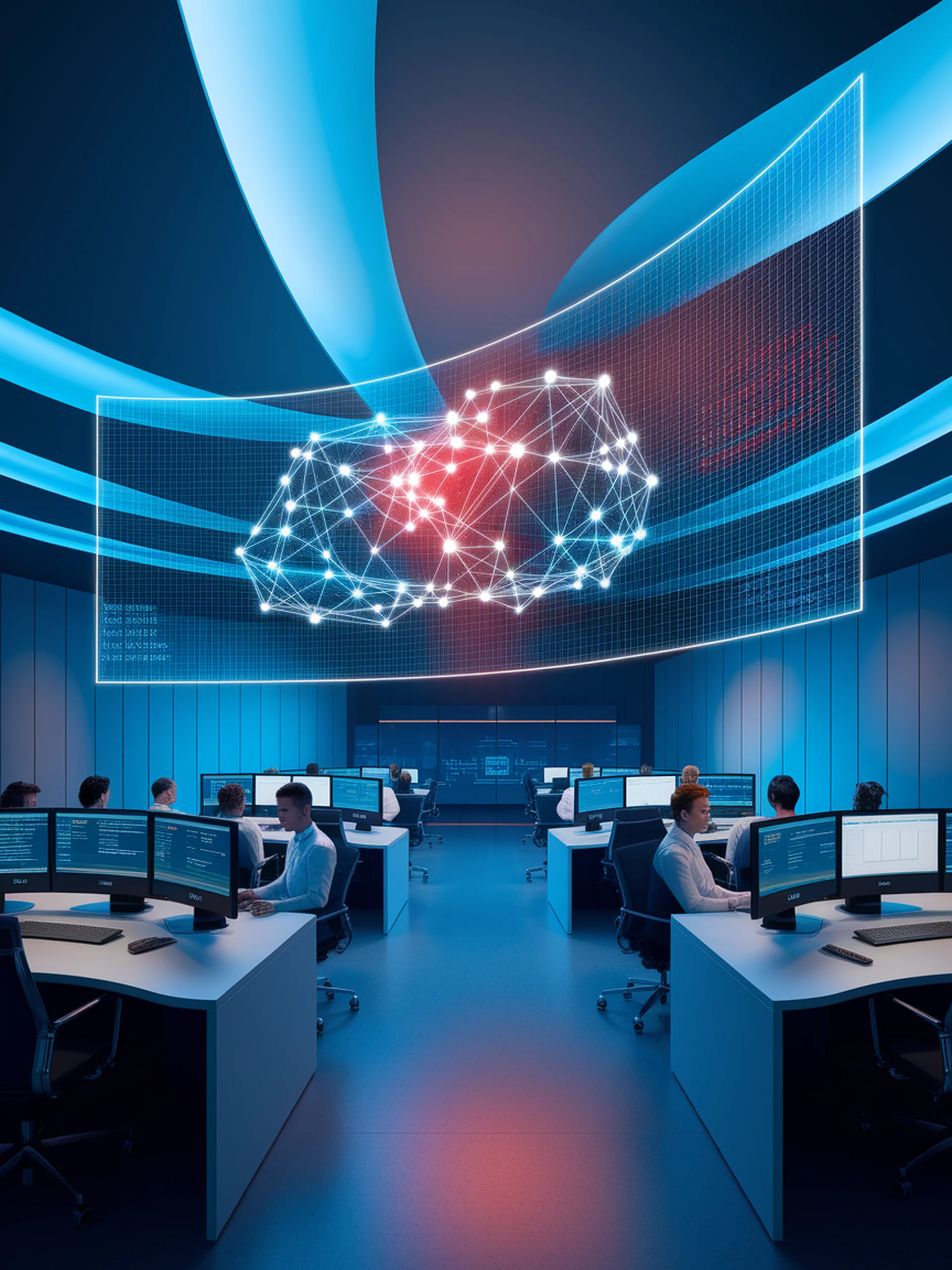