Privacy-Preserving Techniques for LLMs
Research on maintaining data privacy while utilizing LLMs through differential privacy and other methods
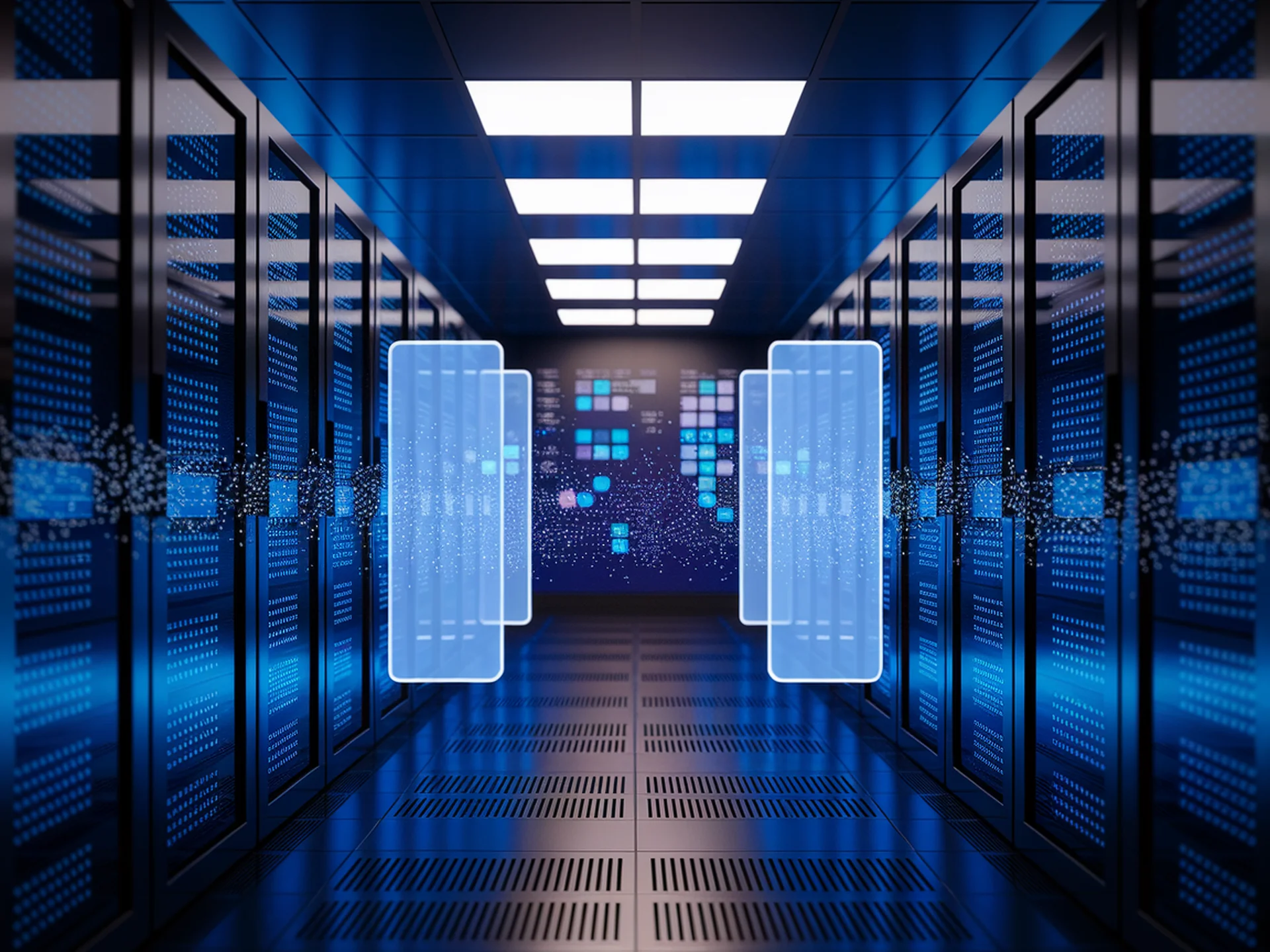
Privacy-Preserving Techniques for LLMs
Research on Large Language Models in Privacy-Preserving Techniques for LLMs
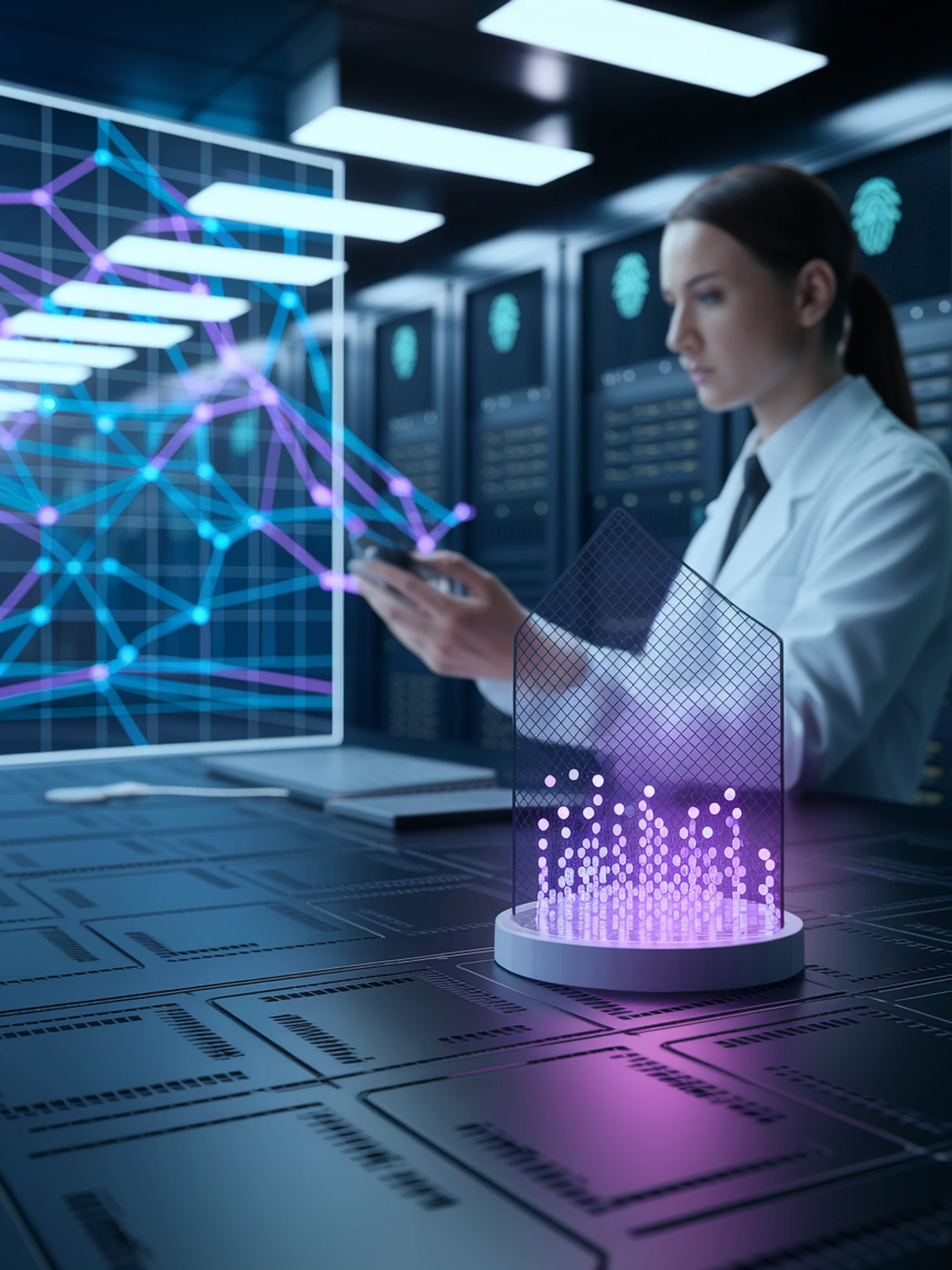
Privacy-Preserving ML for Similarity-Based Models
New DP-SGD approach for contrastive learning in LLMs
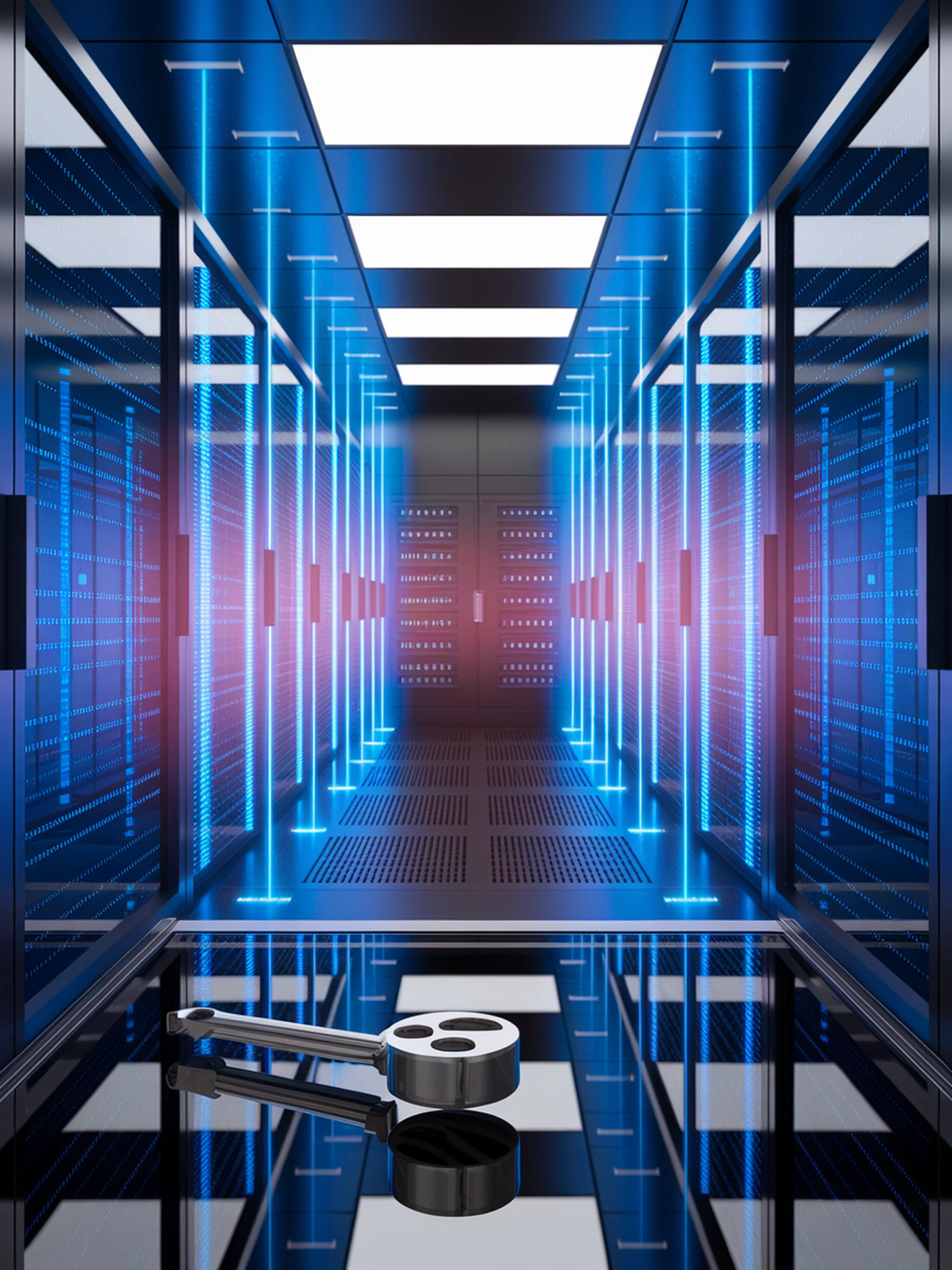
Securing LLMs: Privacy Without Compromise
A practical framework for private interactions with black-box language models
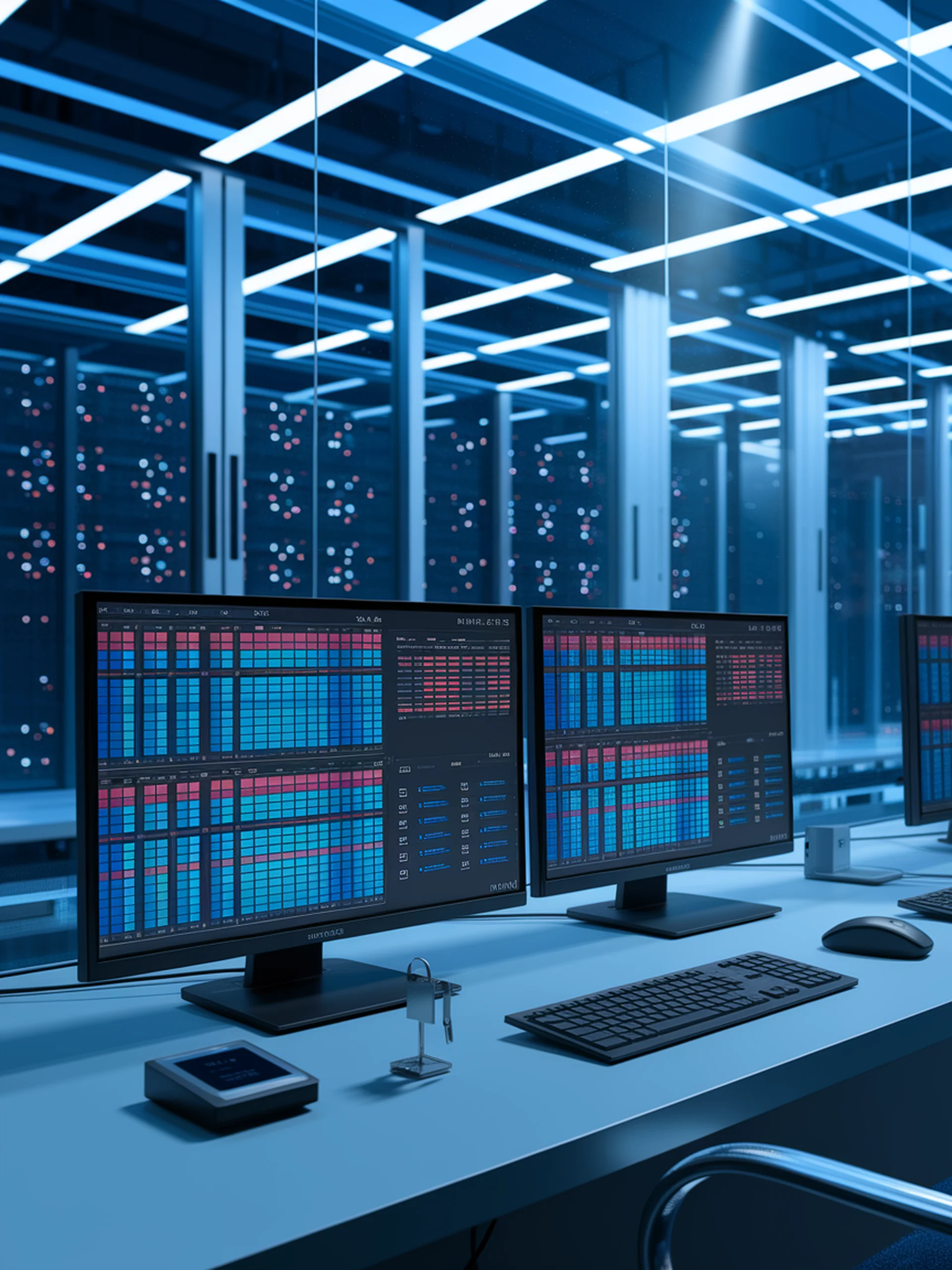
Reimagining Tabular Data Synthesis with LLMs
TabuLa: A Novel Approach to Generate Realistic Tabular Data
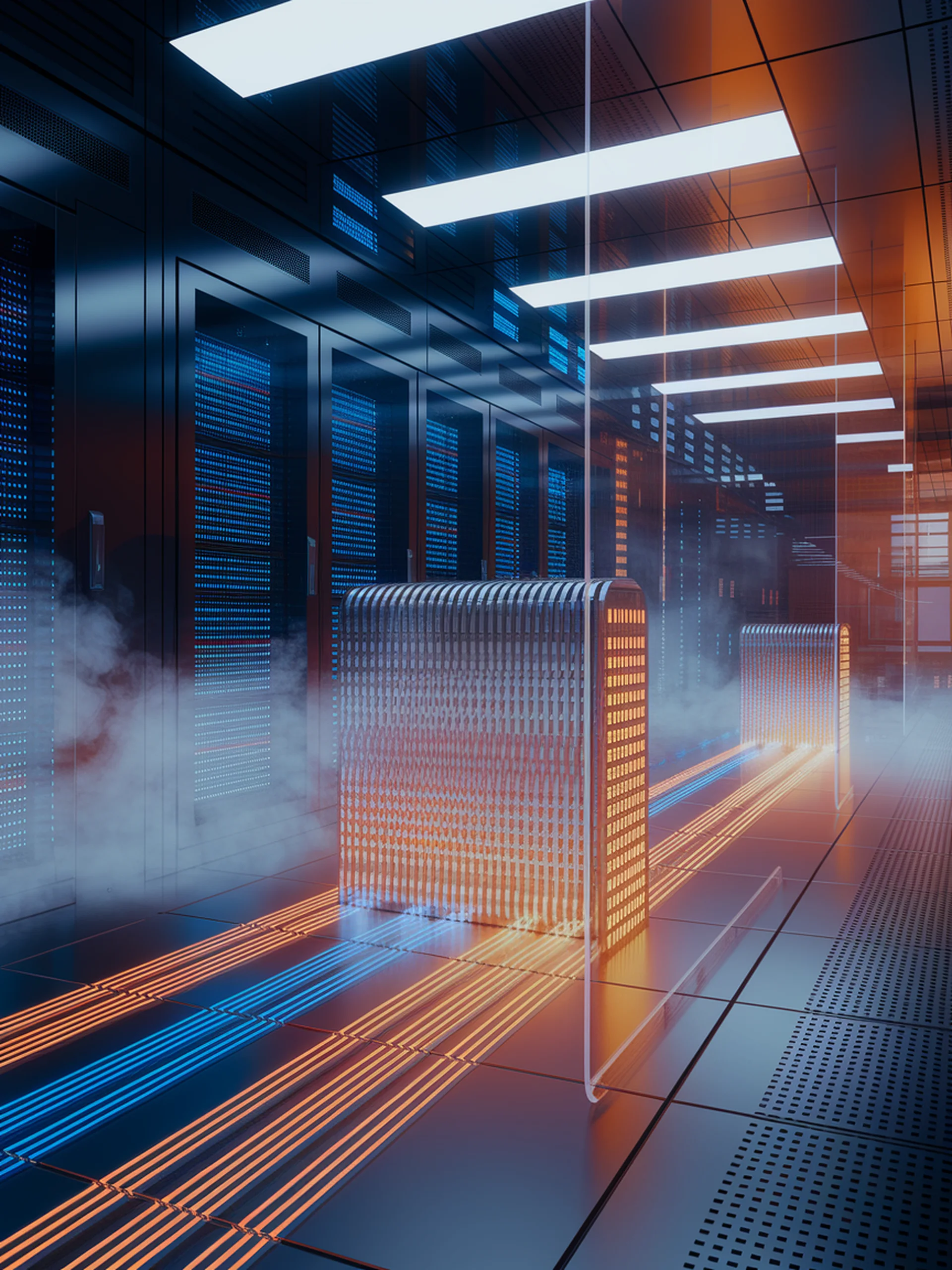
Private LLM Fine-tuning Breakthrough
Securing AI Training with Zeroth-order Optimization
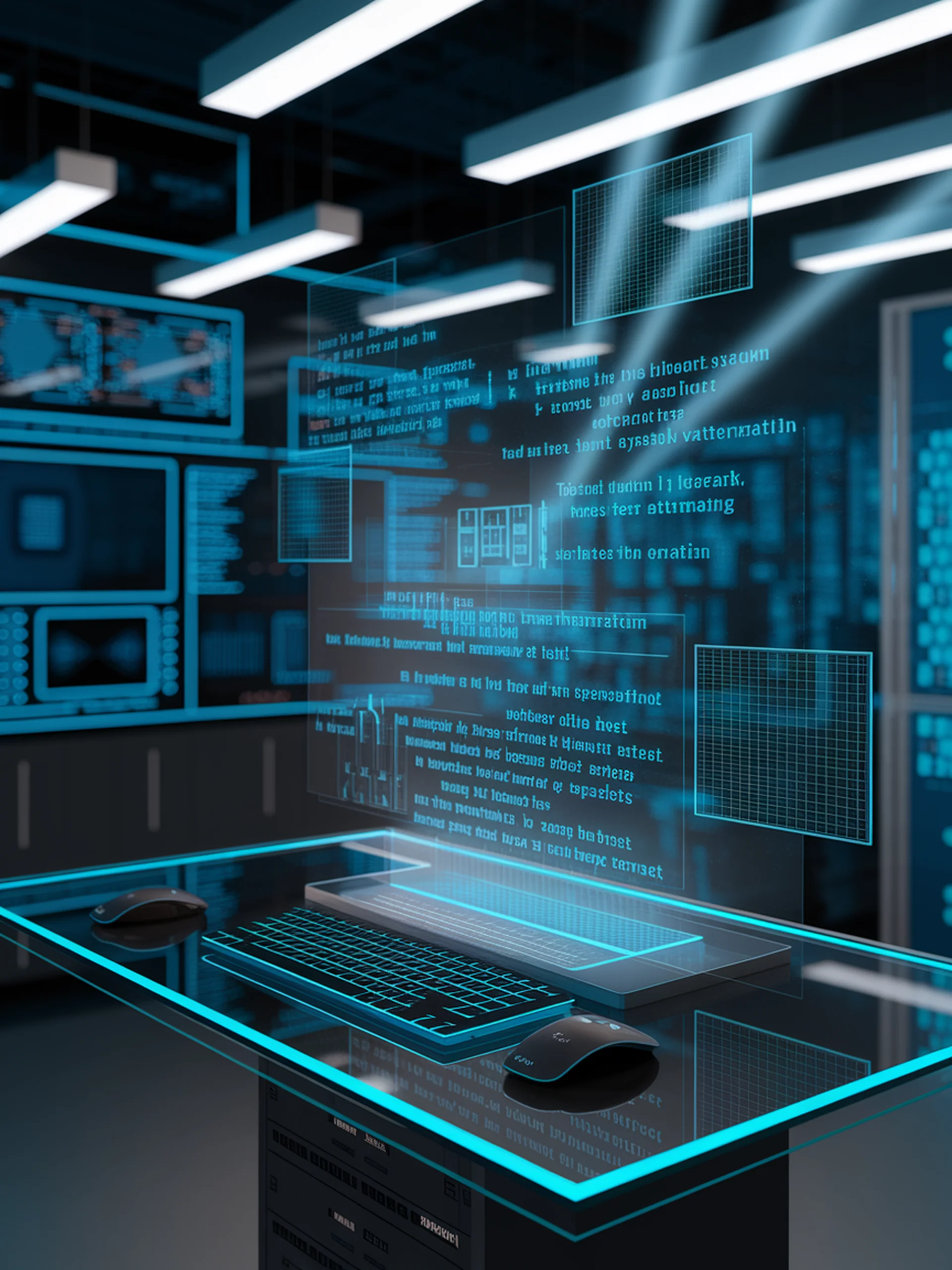
Securing AI-Generated Content
Robust Multi-bit Watermarking for LLM Text Attribution
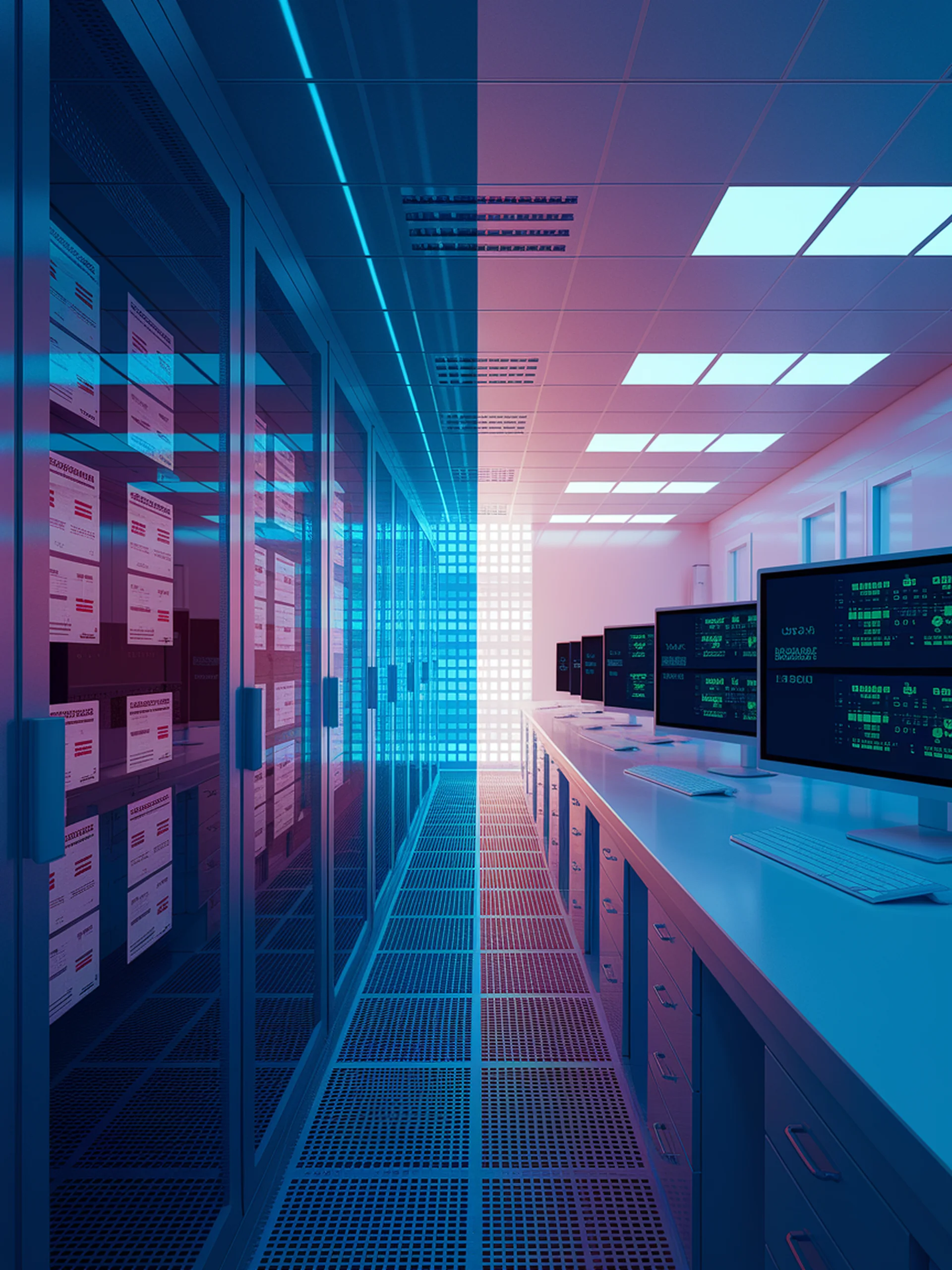
Beyond De-identification: The Promise of Synthetic Medical Data
Comparing Privacy Protection Methods for Clinical Notes
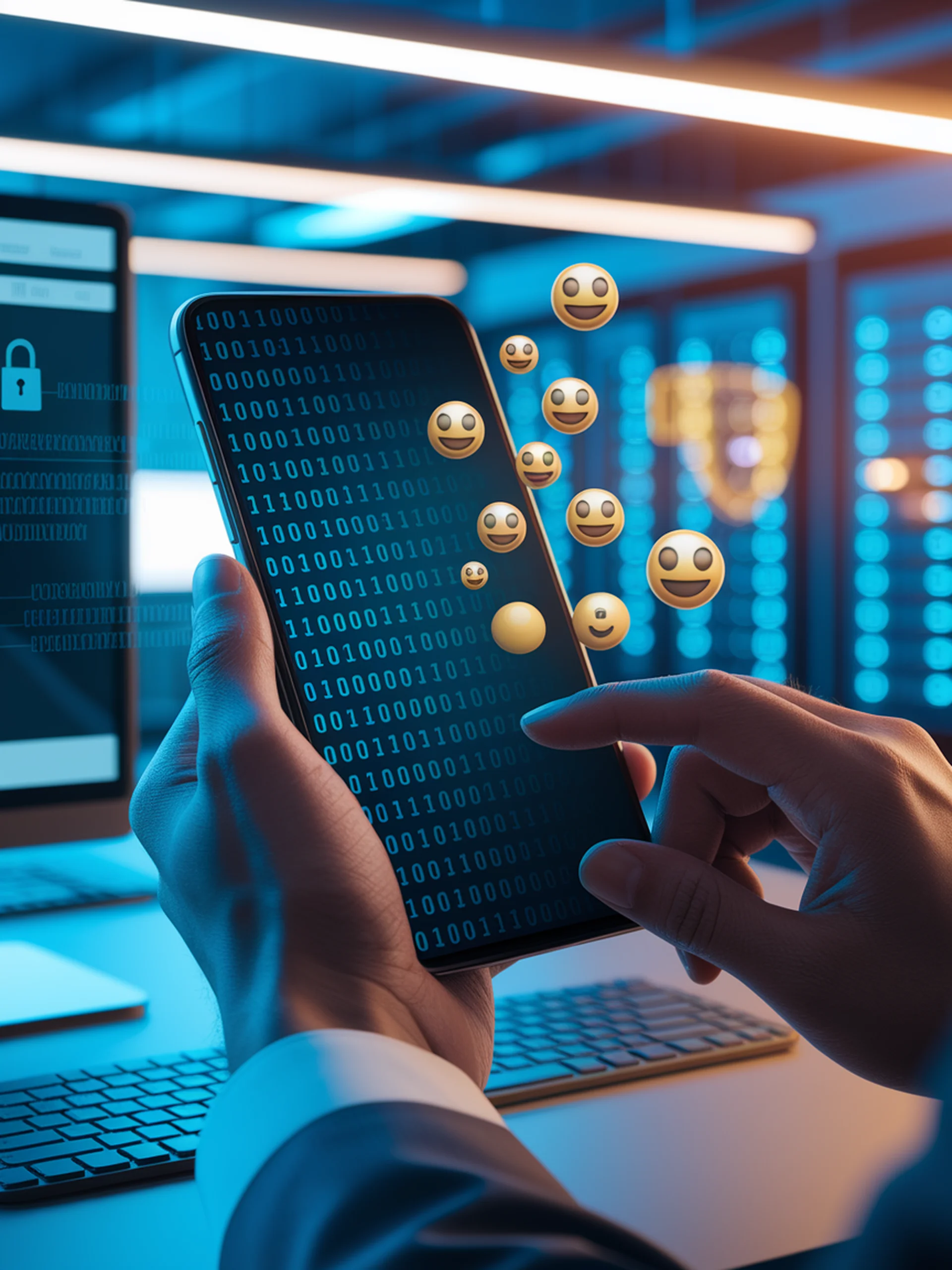
Protecting Privacy in LLM Interactions
Using Emoji-Based Obfuscation to Secure User Prompts
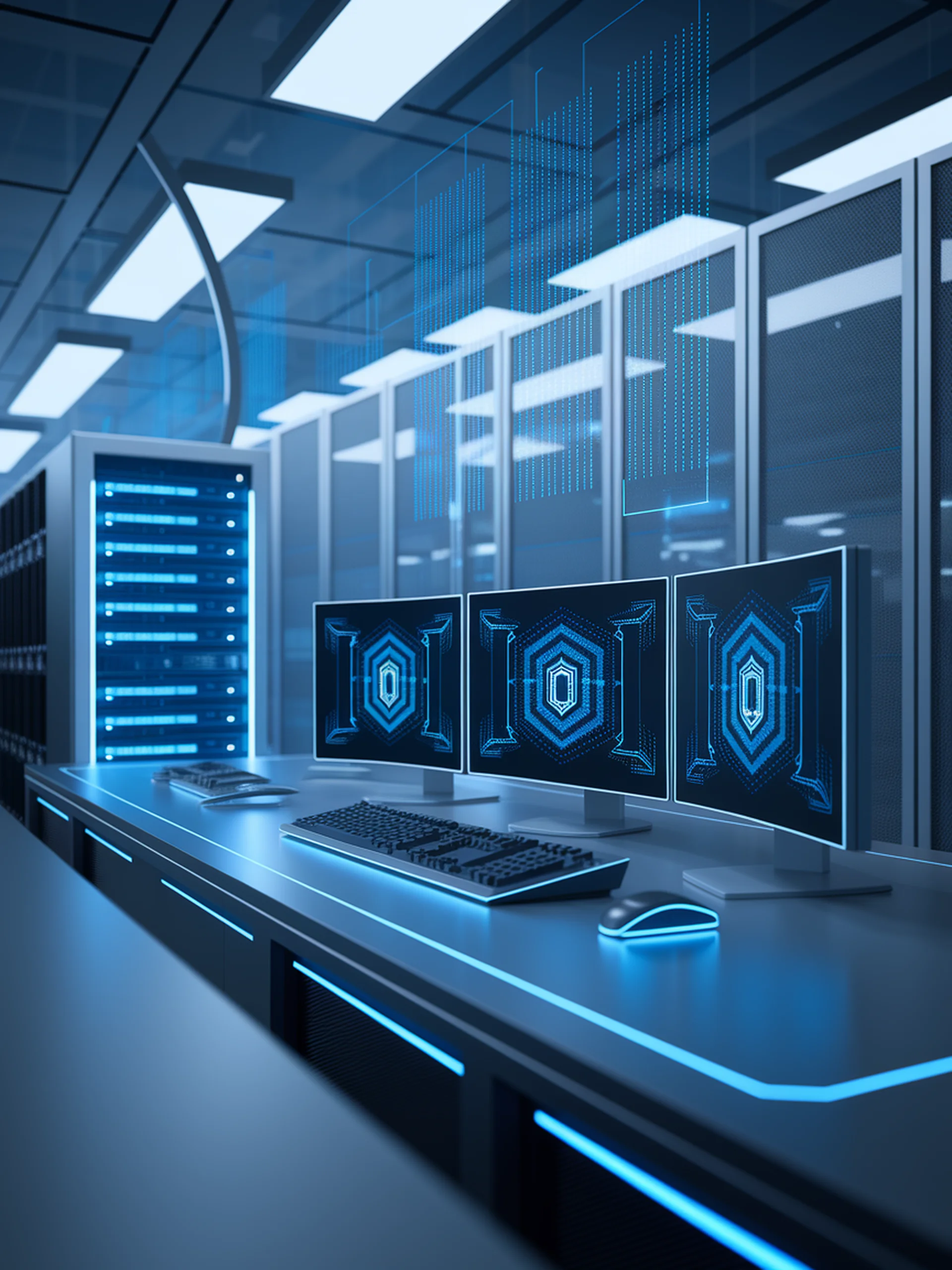
Privacy-Preserving LLM Fine-Tuning
A Zeroth-Order Approach for Balancing Privacy, Utility, and Performance
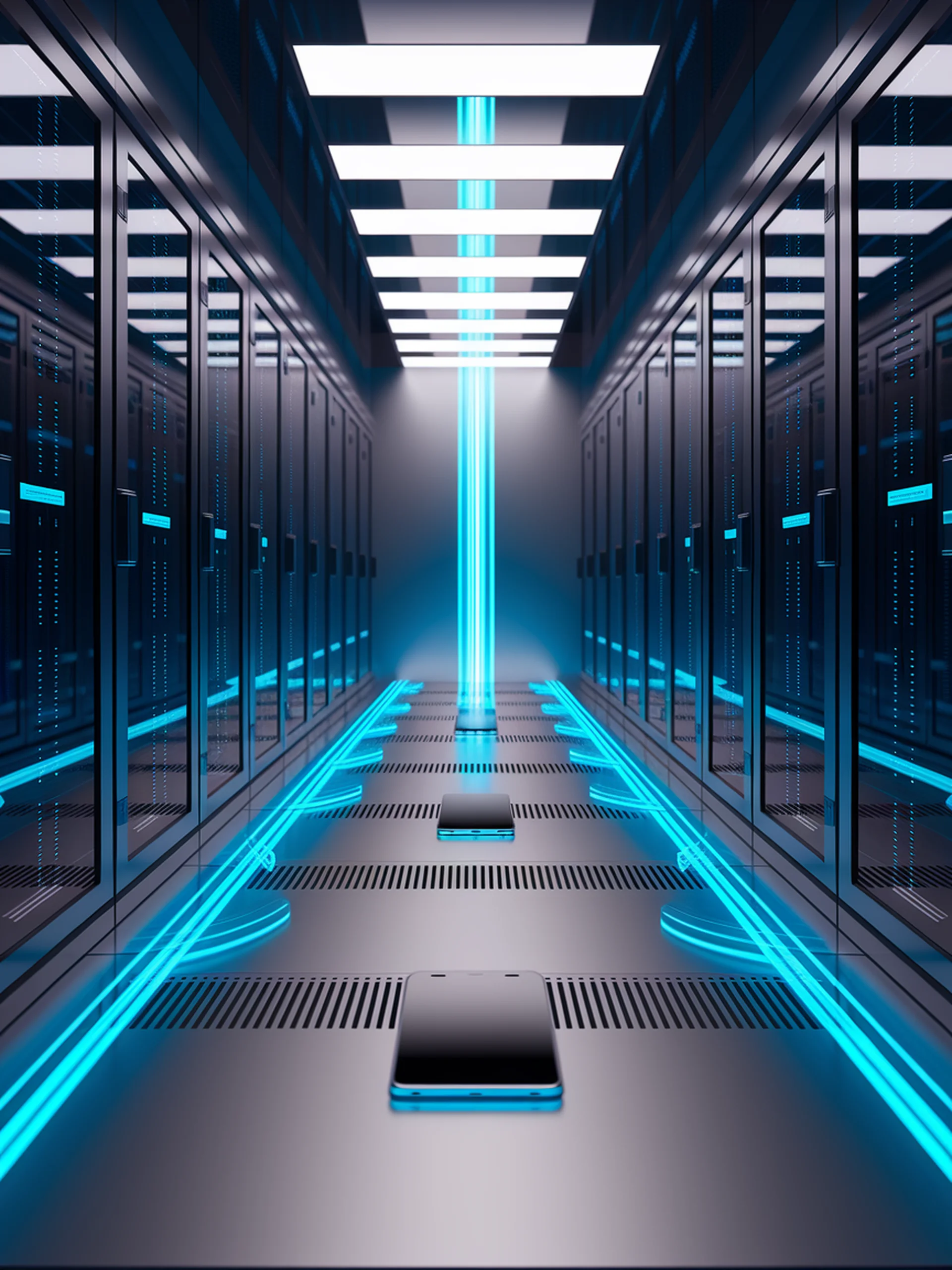
Privacy-Preserving LLM Recommendations
A federated learning approach that protects user data while enabling personalized recommendations
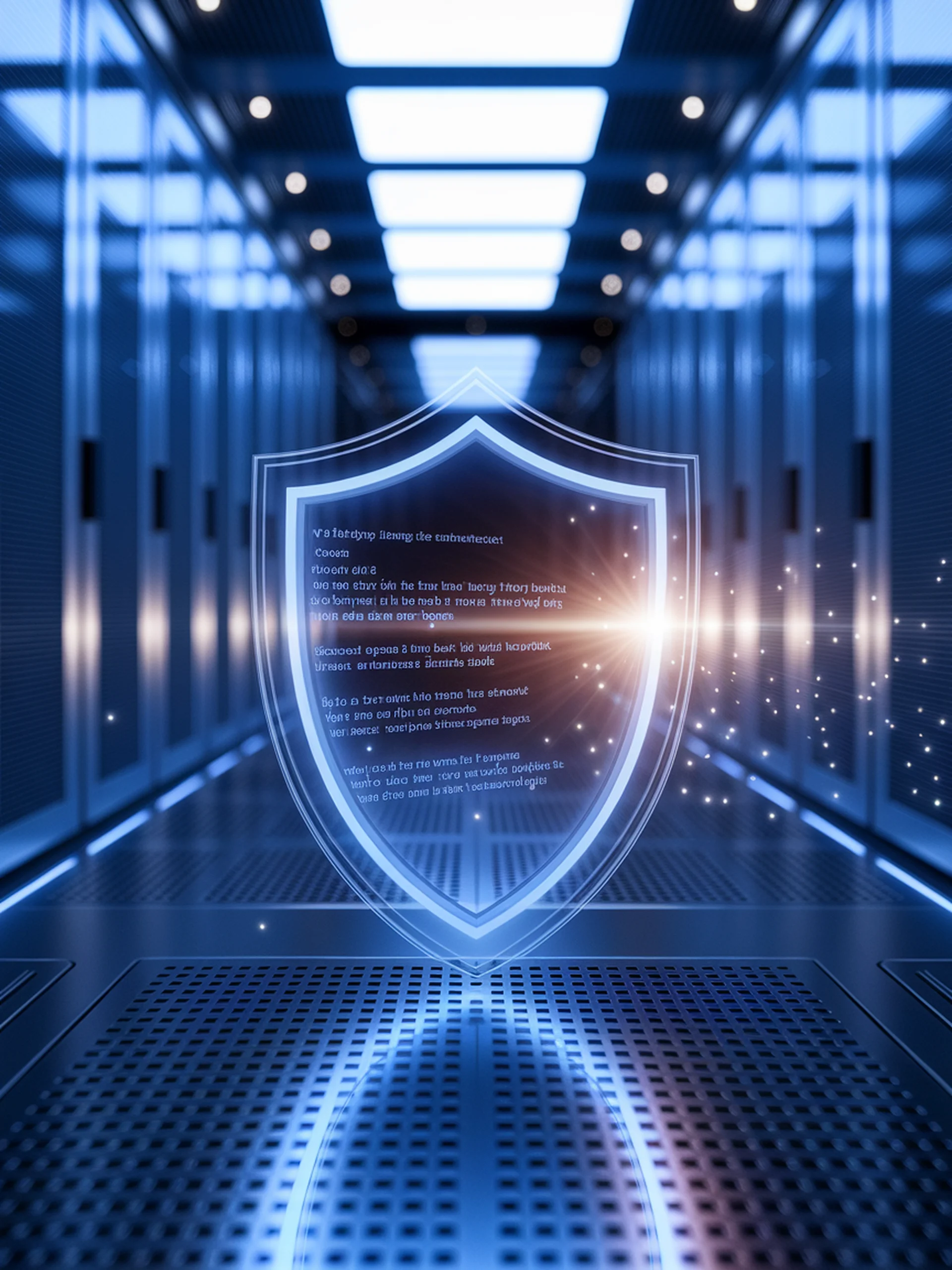
LLMs as Privacy Defenders
Leveraging language models to strengthen text anonymization
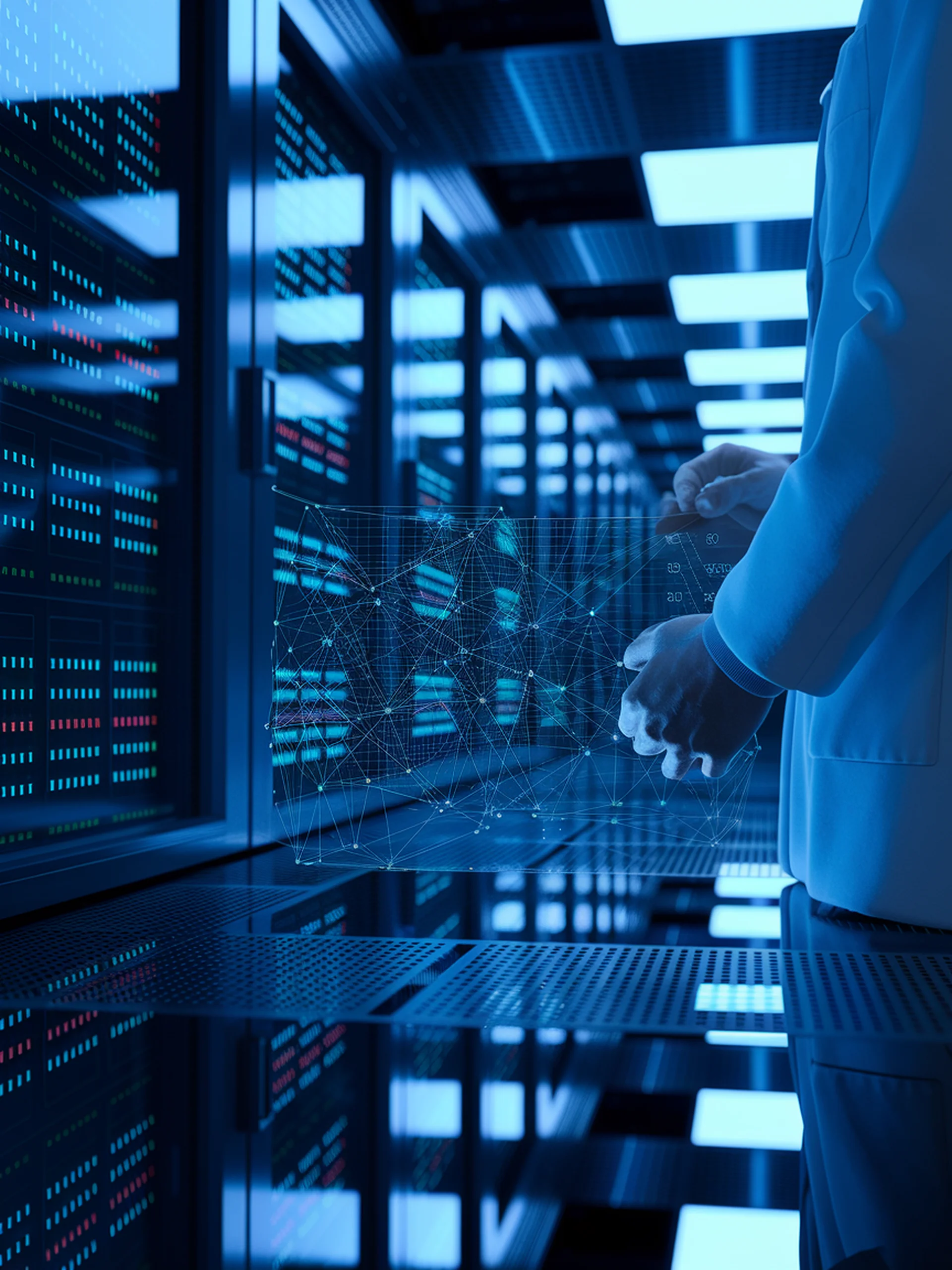
Detecting Pre-Training Data in LLMs
A new, theoretically-sound approach to identifying training data leakage
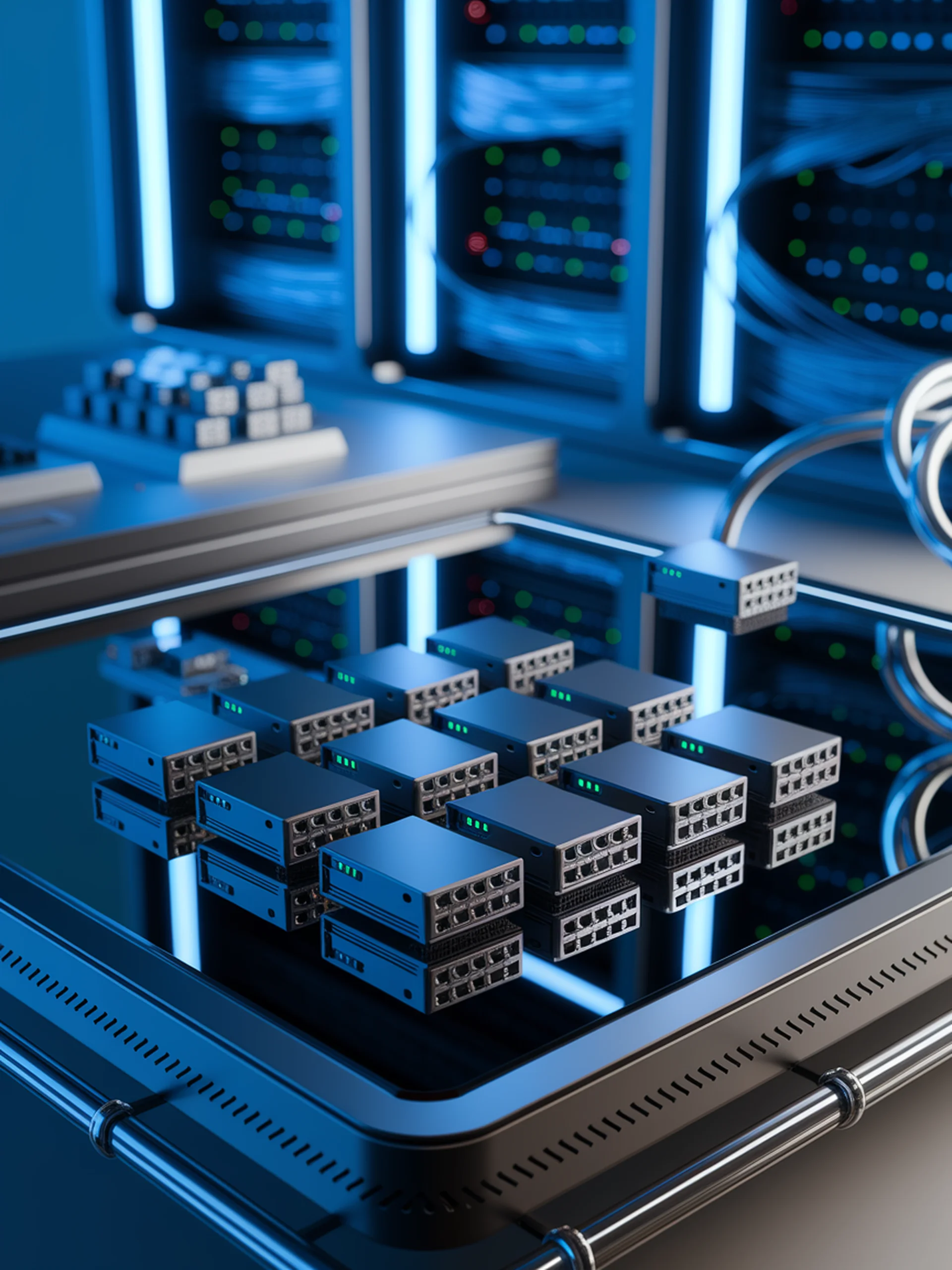
Controlled Access & Data Removal for LLMs
AdapterSwap: A framework for managing evolving data requirements in LLMs
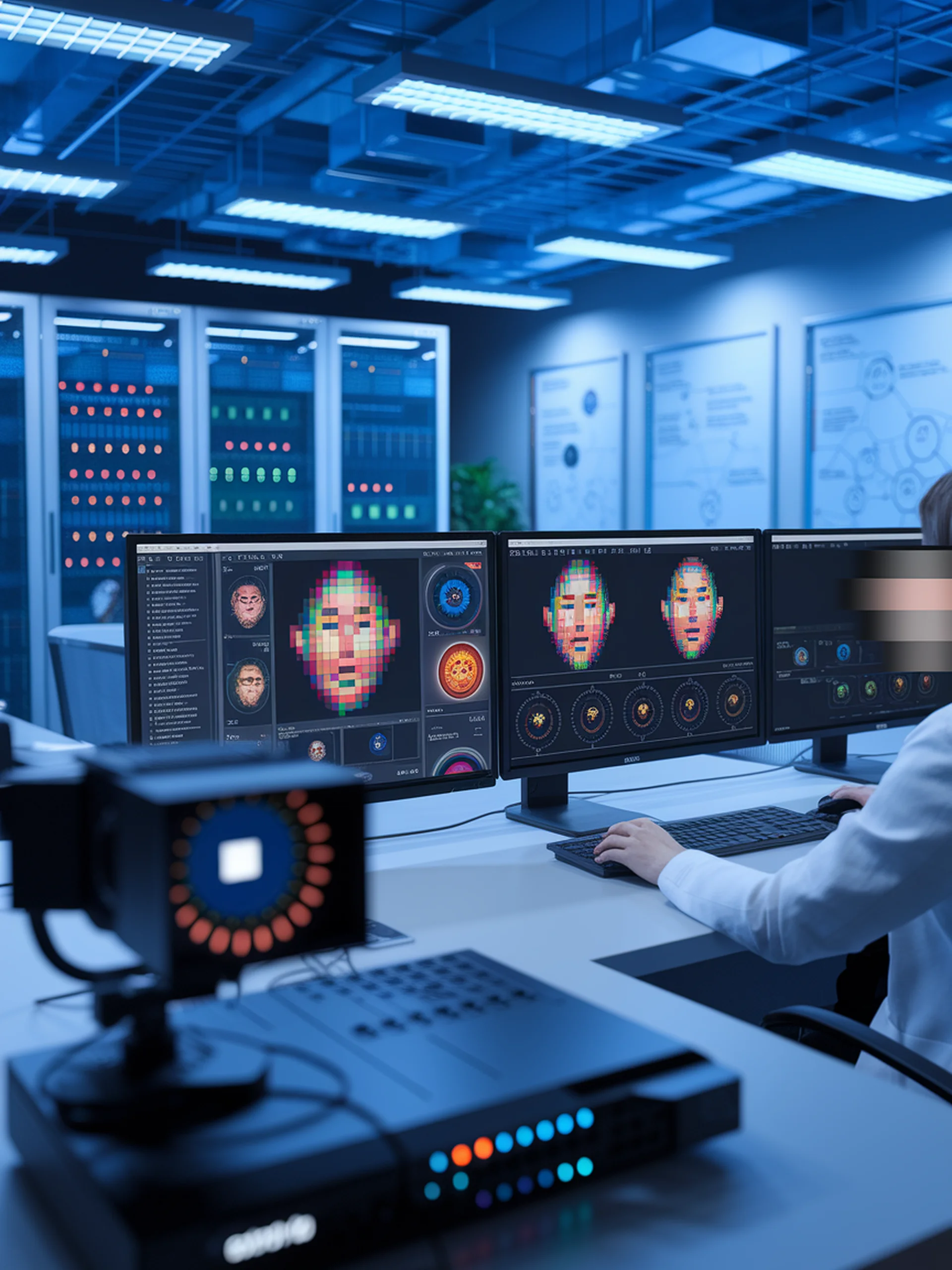
Privacy-Preserving Emotion Analysis
Advancing Emotion AI for Long Videos While Protecting Identity
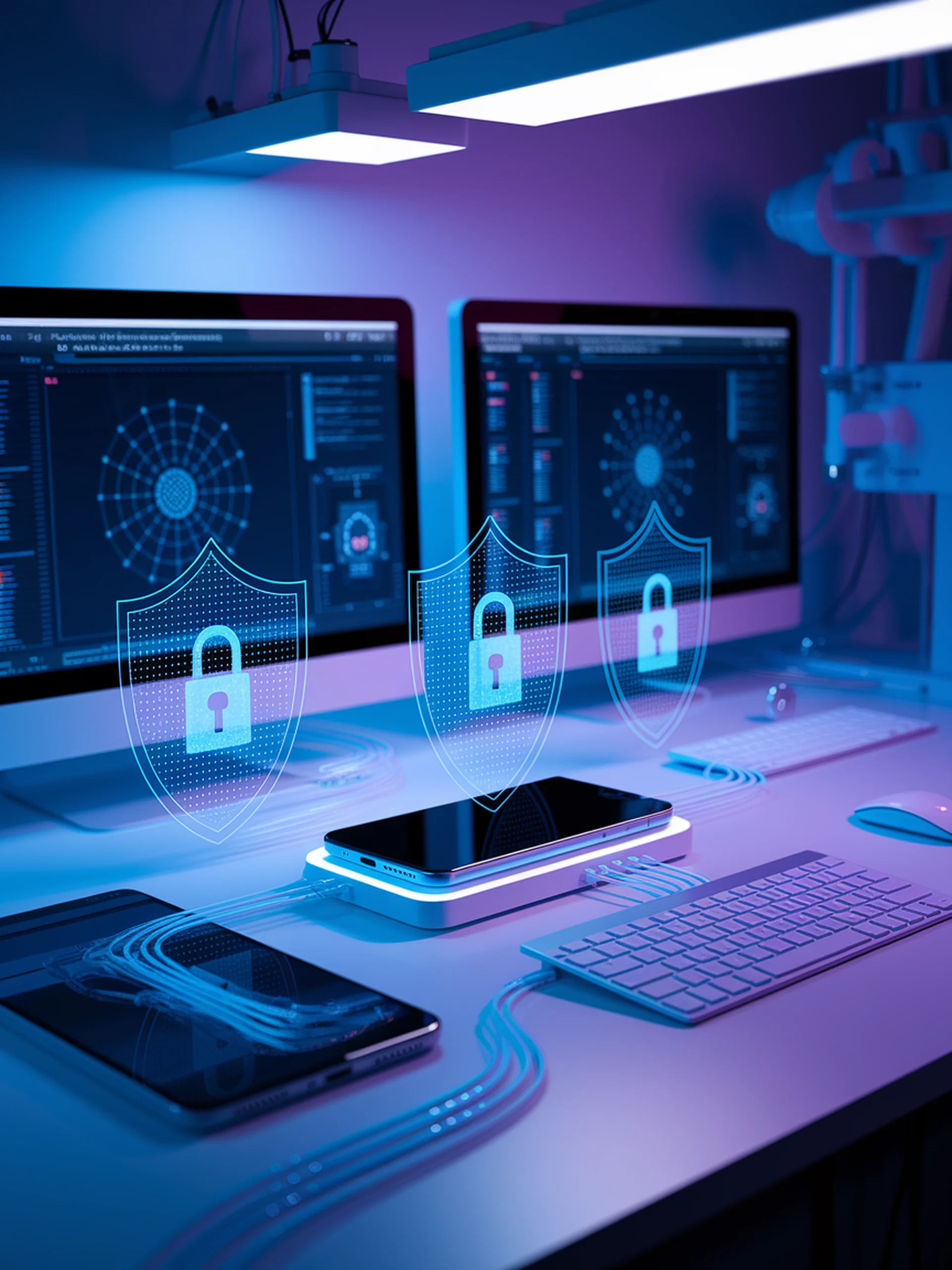
Privacy-Preserving On-Device AI
Enabling secure model fine-tuning without sacrificing performance
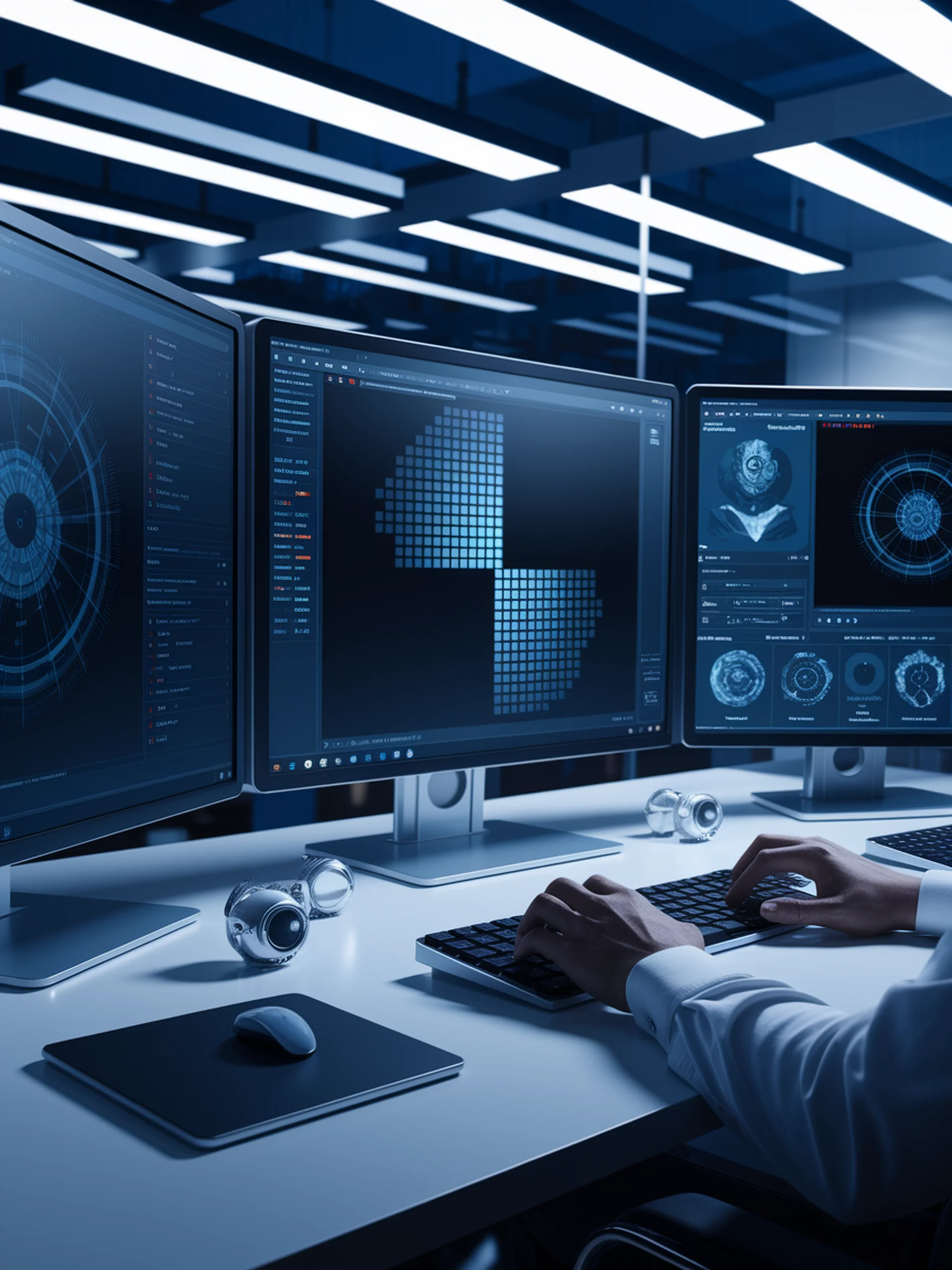
Forgetting What AI Has Seen
Enabling privacy through selective image unlearning in multimodal LLMs
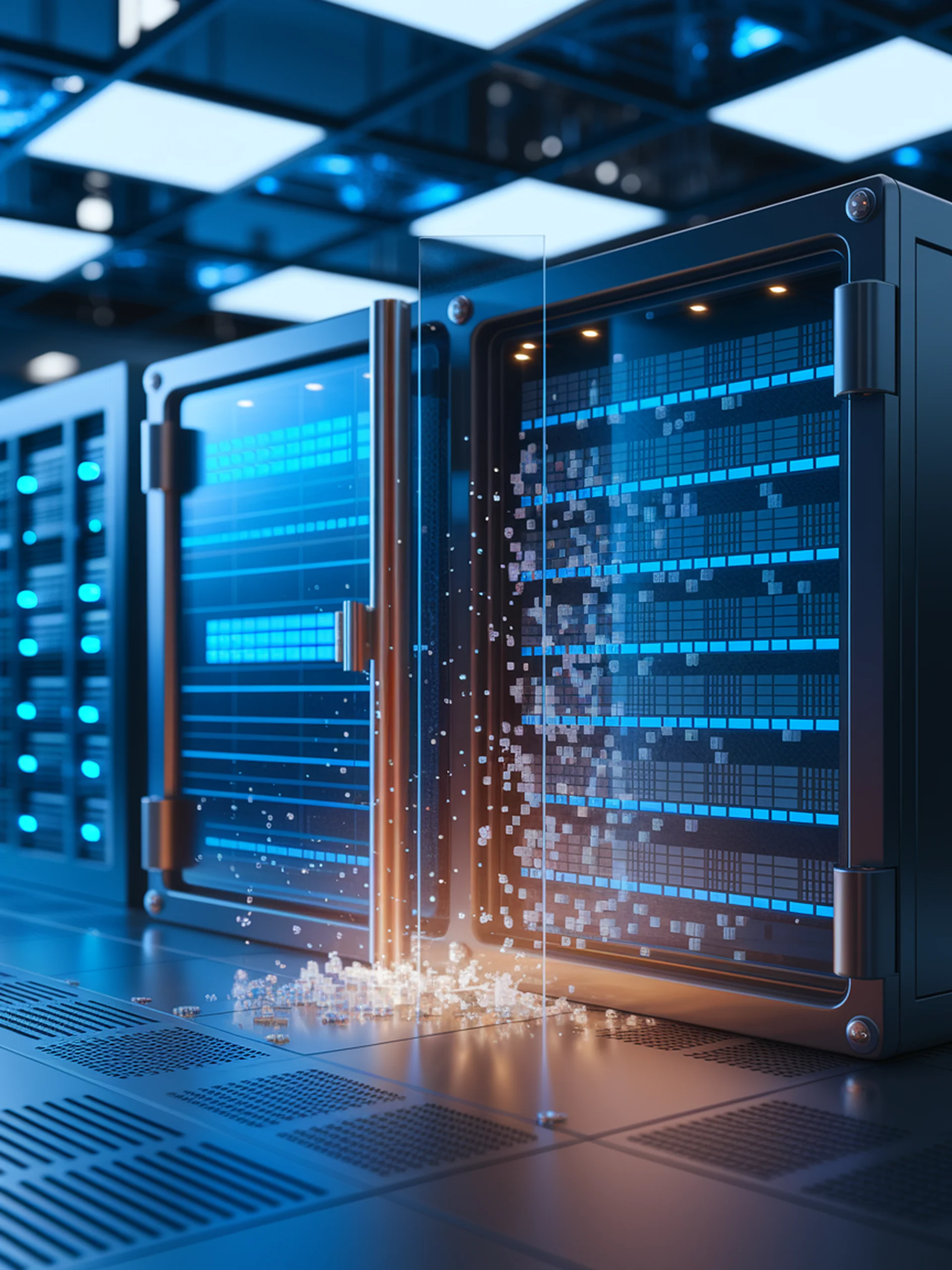
Knowledge Washing in Large Language Models
Safely removing unwanted knowledge while preserving model capabilities
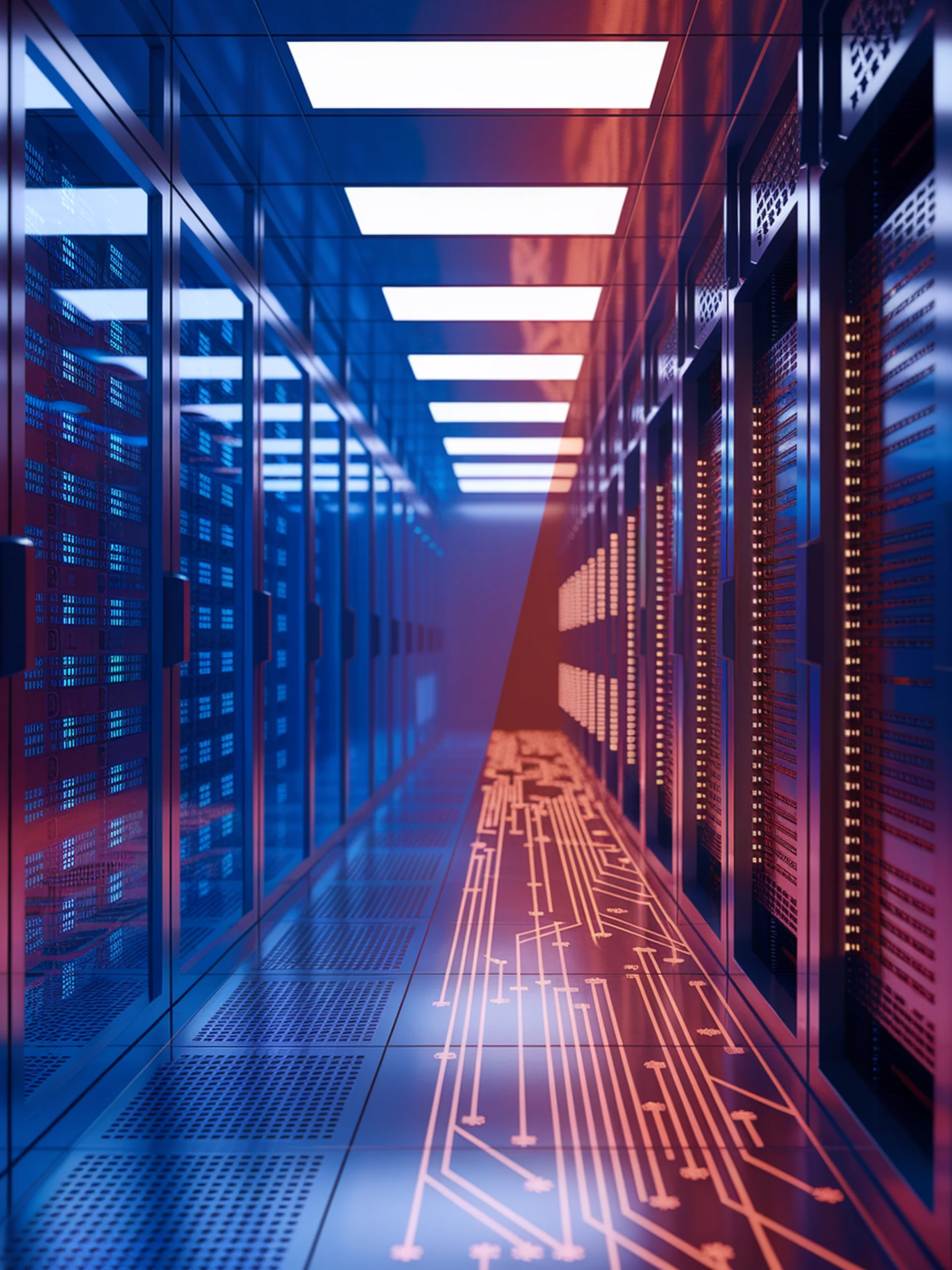
The Privacy-Performance Trade-off in LLMs
Why there's no perfect solution for private LLM inference
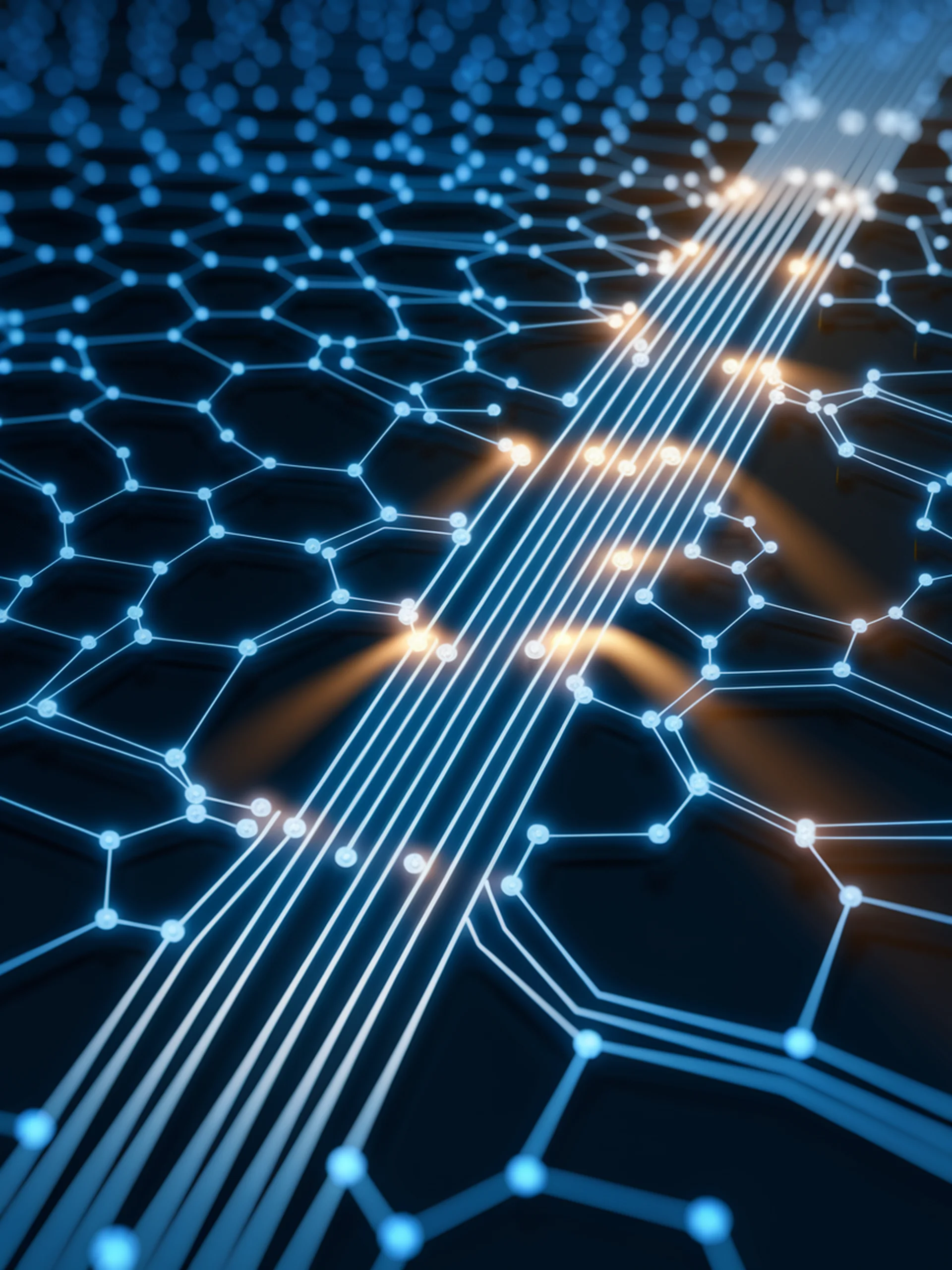
The Blind Spots in LLM Unlearning
Developing more robust evaluation frameworks for data removal
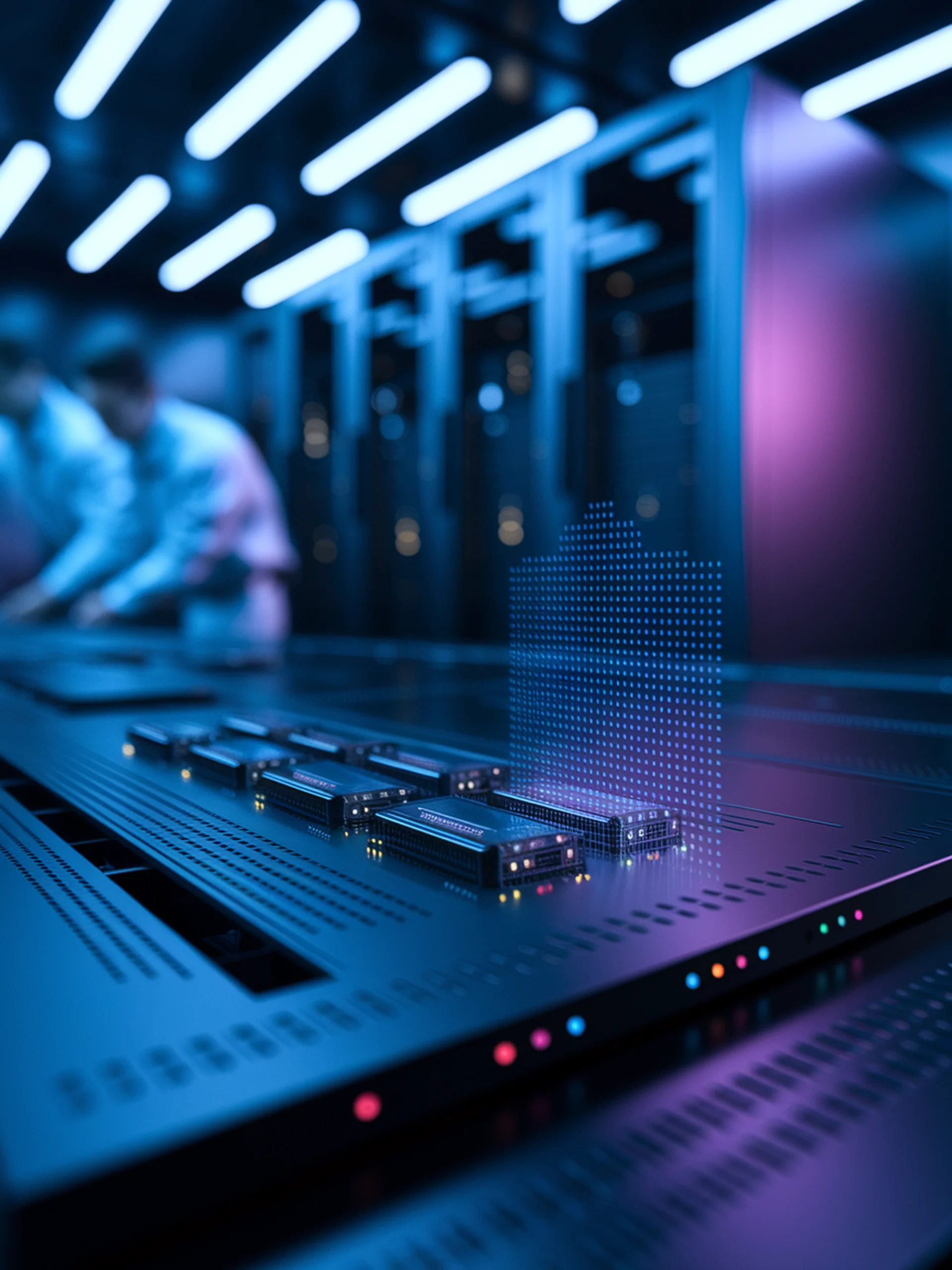
Privacy-Preserving Language Models
Memory-Efficient Transfer Learning with Privacy Guarantees
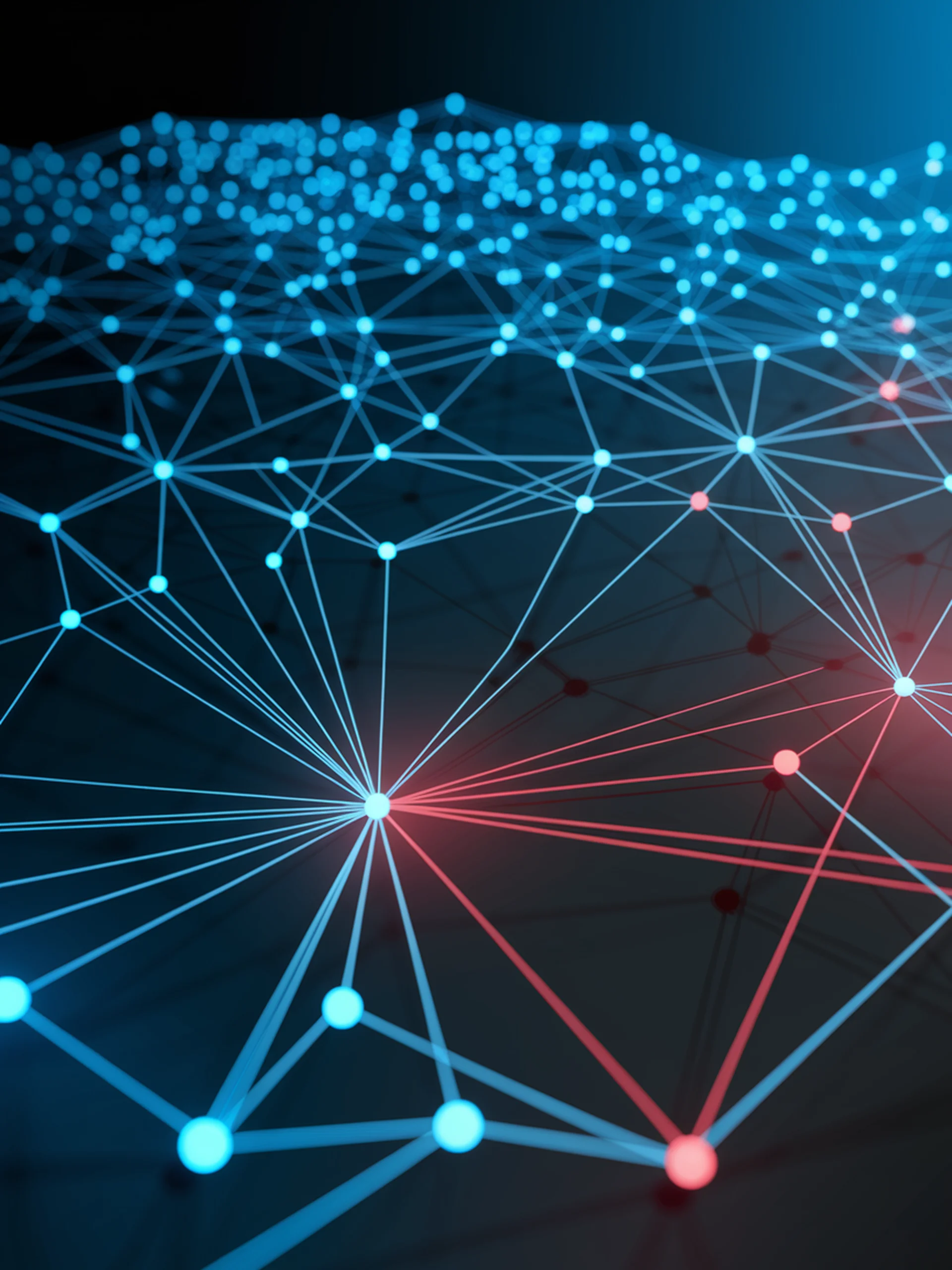
Rethinking LLM Unlearning: The Missing Data Connection
How interconnected data structures impact secure knowledge removal
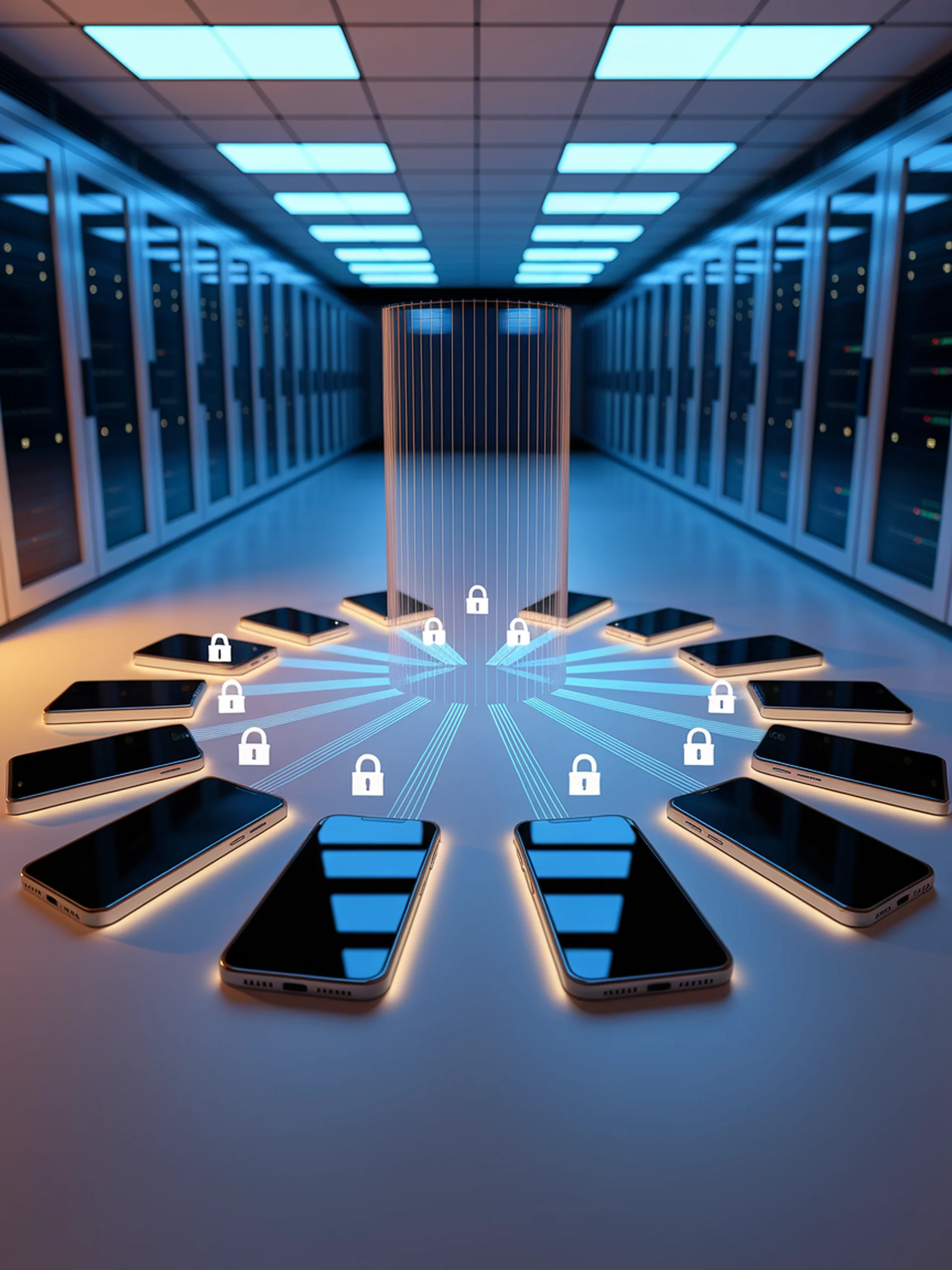
Privacy-Preserving AI Alignment
Federated Learning for RLHF Without Sharing Personal Data
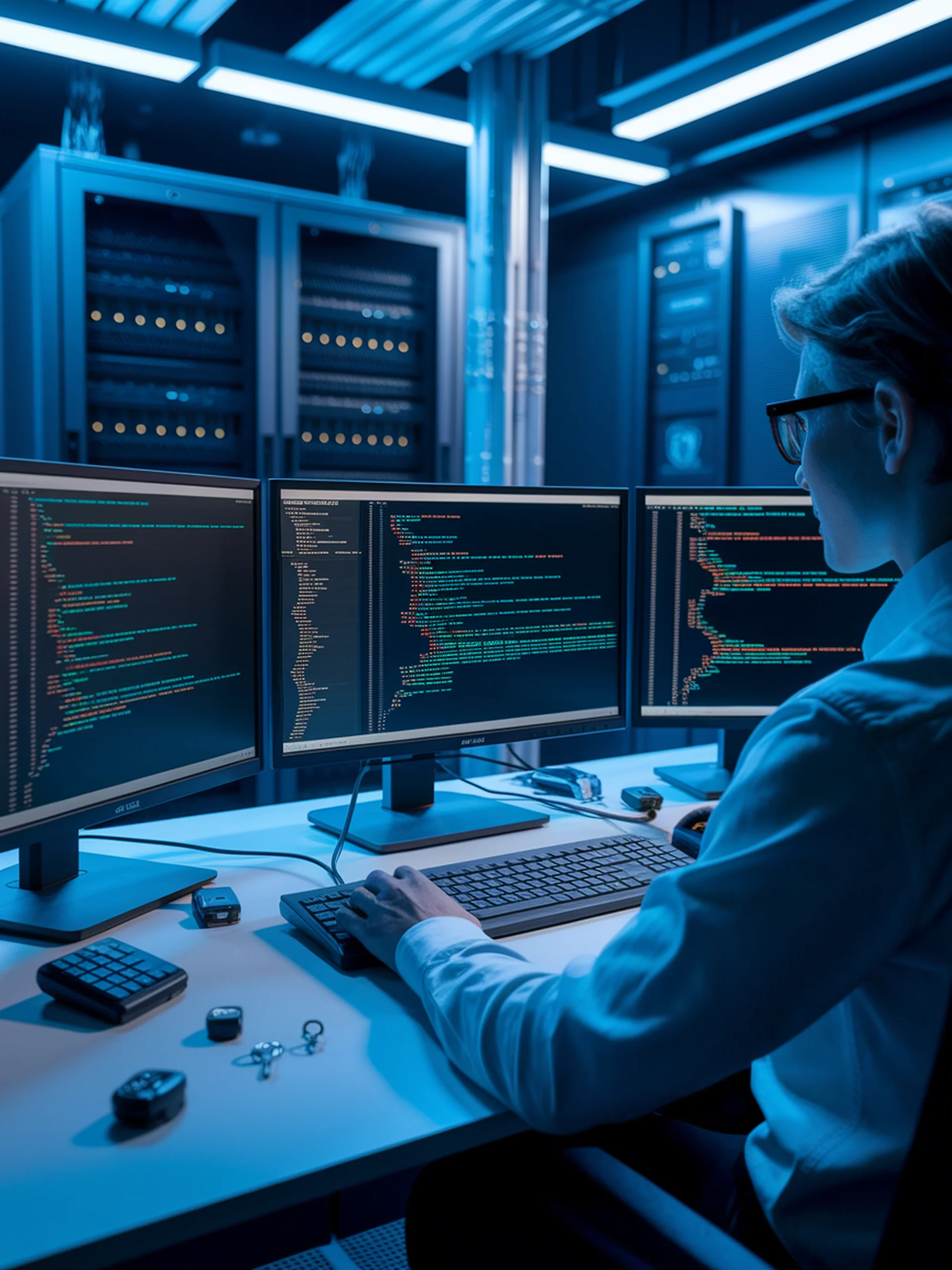
Continual Unlearning for Large Language Models
A framework for ongoing security maintenance of LLMs
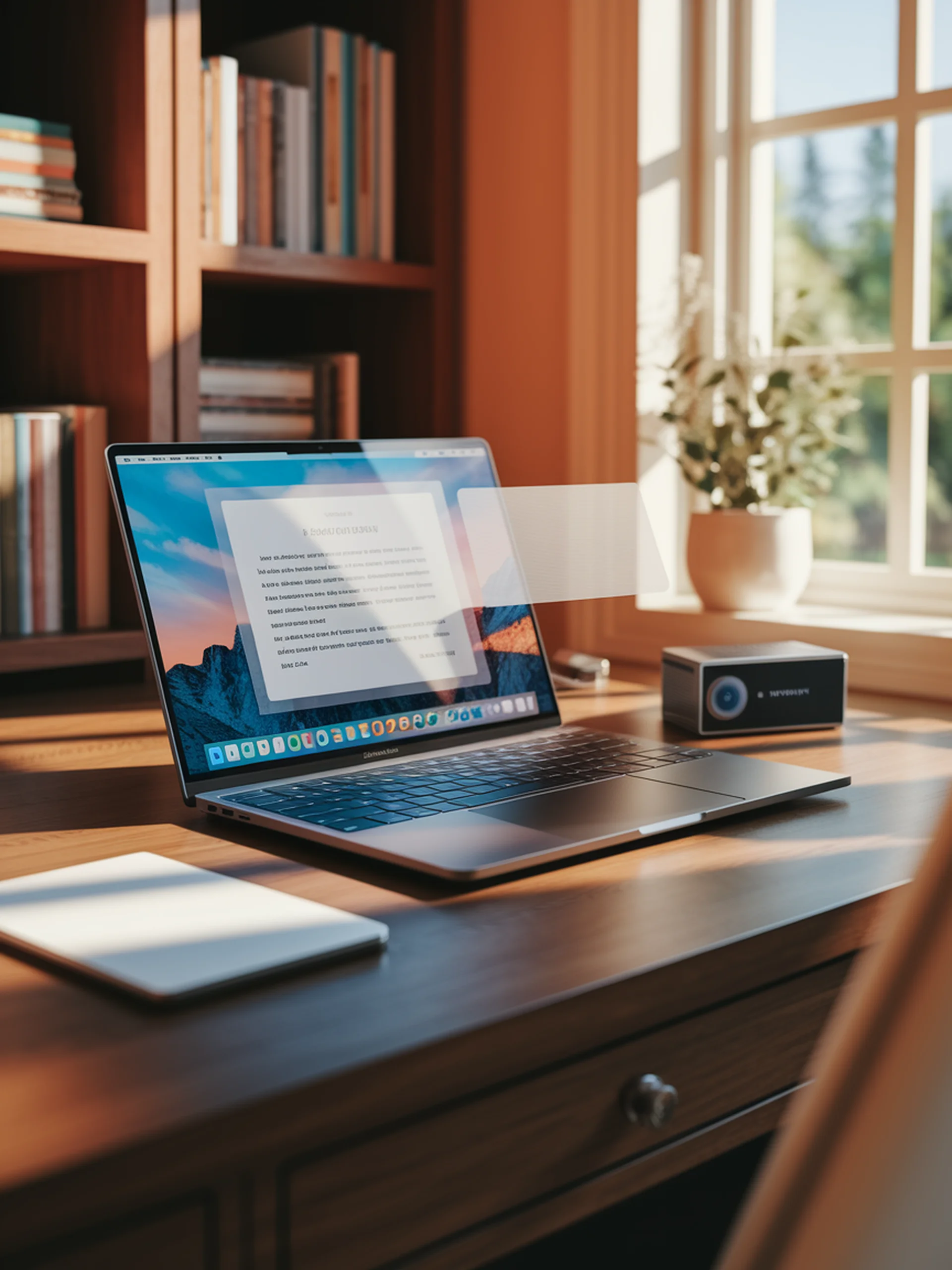
Secure Local AI for Personal Writing
Building privacy-first assistants that match your writing style
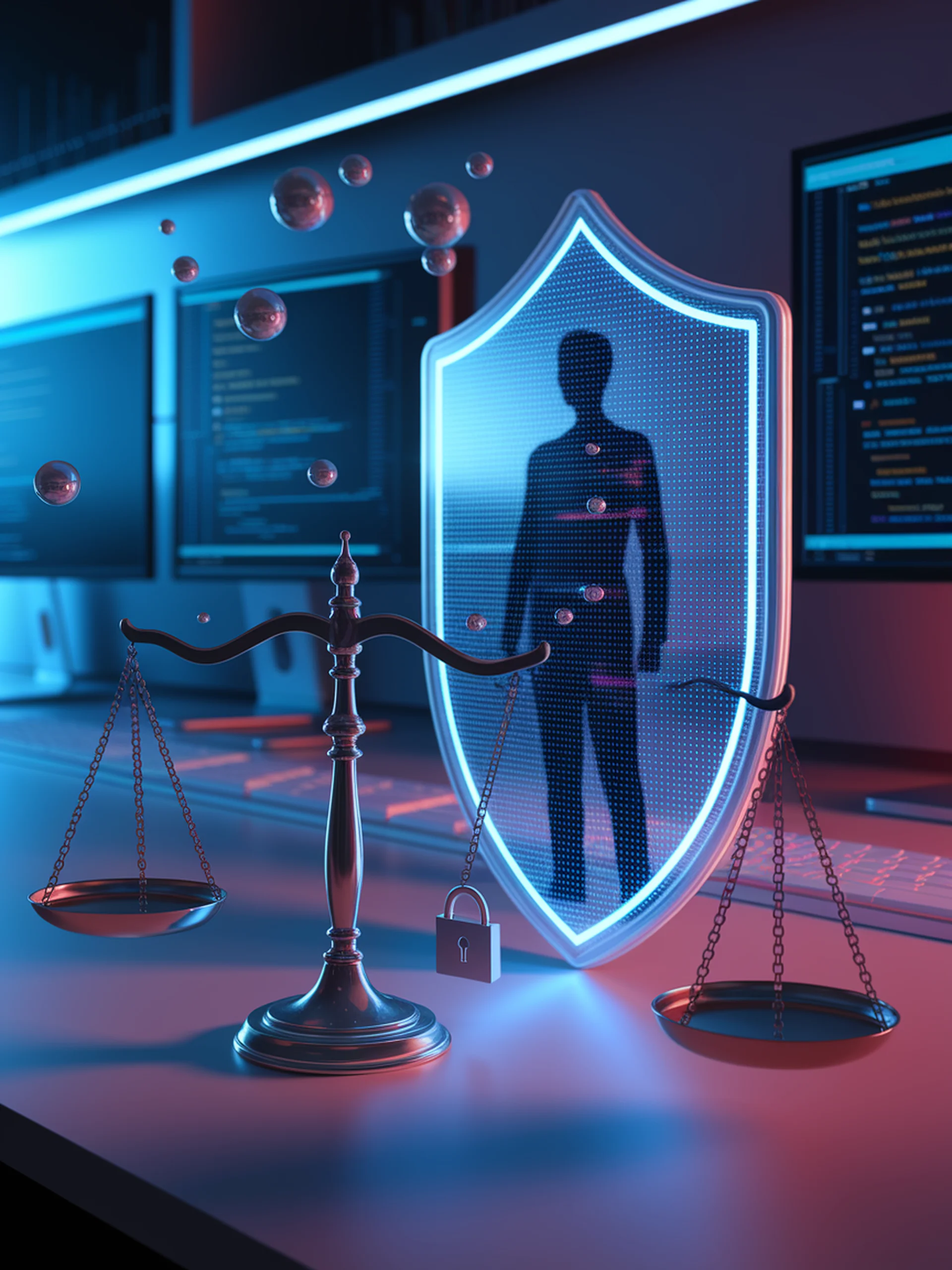
Protecting Author Identity in the AI Era
Task-oriented optimization for balancing privacy and utility
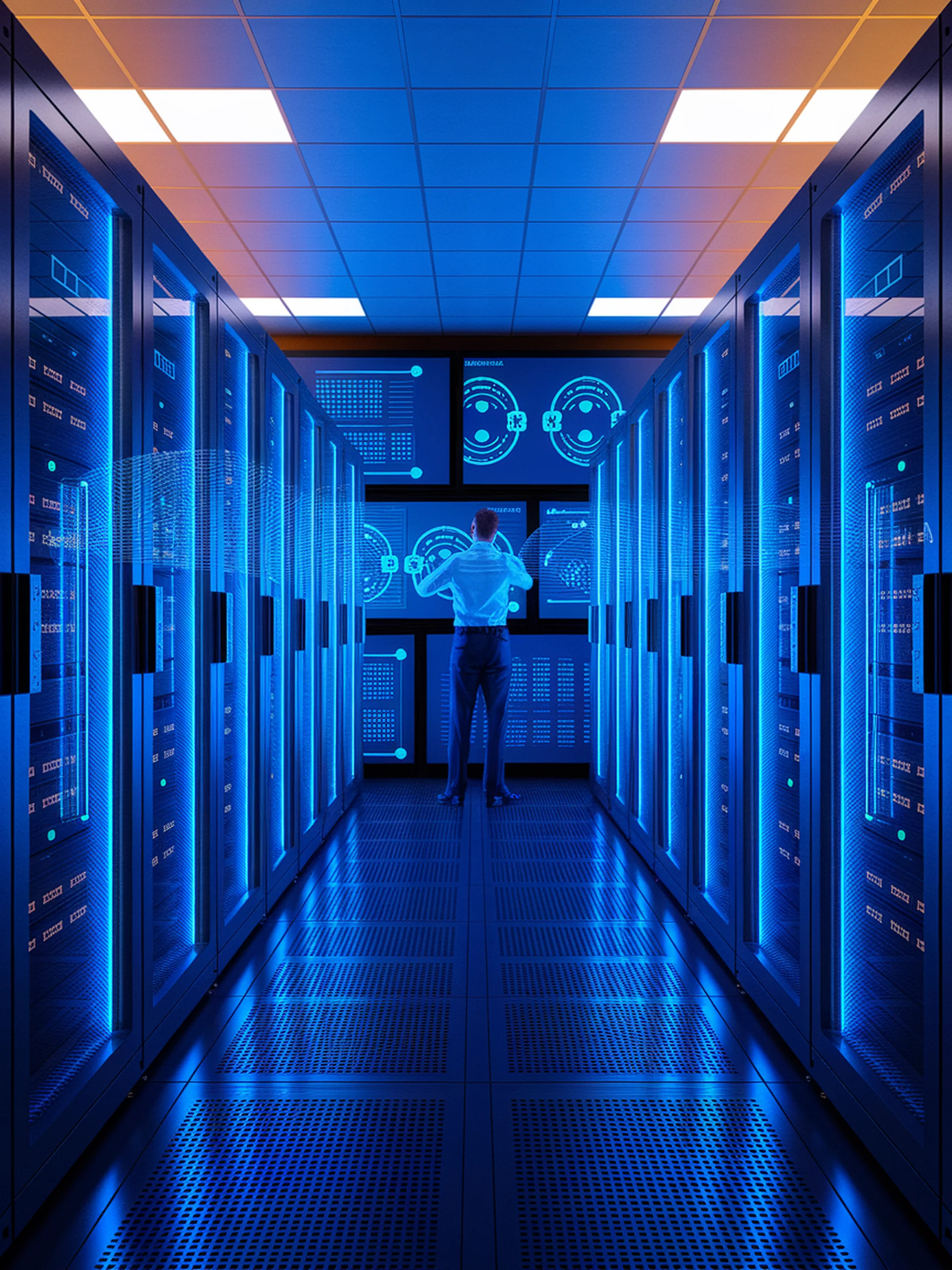
Safeguarding Privacy in the LLM Era
A comprehensive analysis of privacy threats and protection strategies
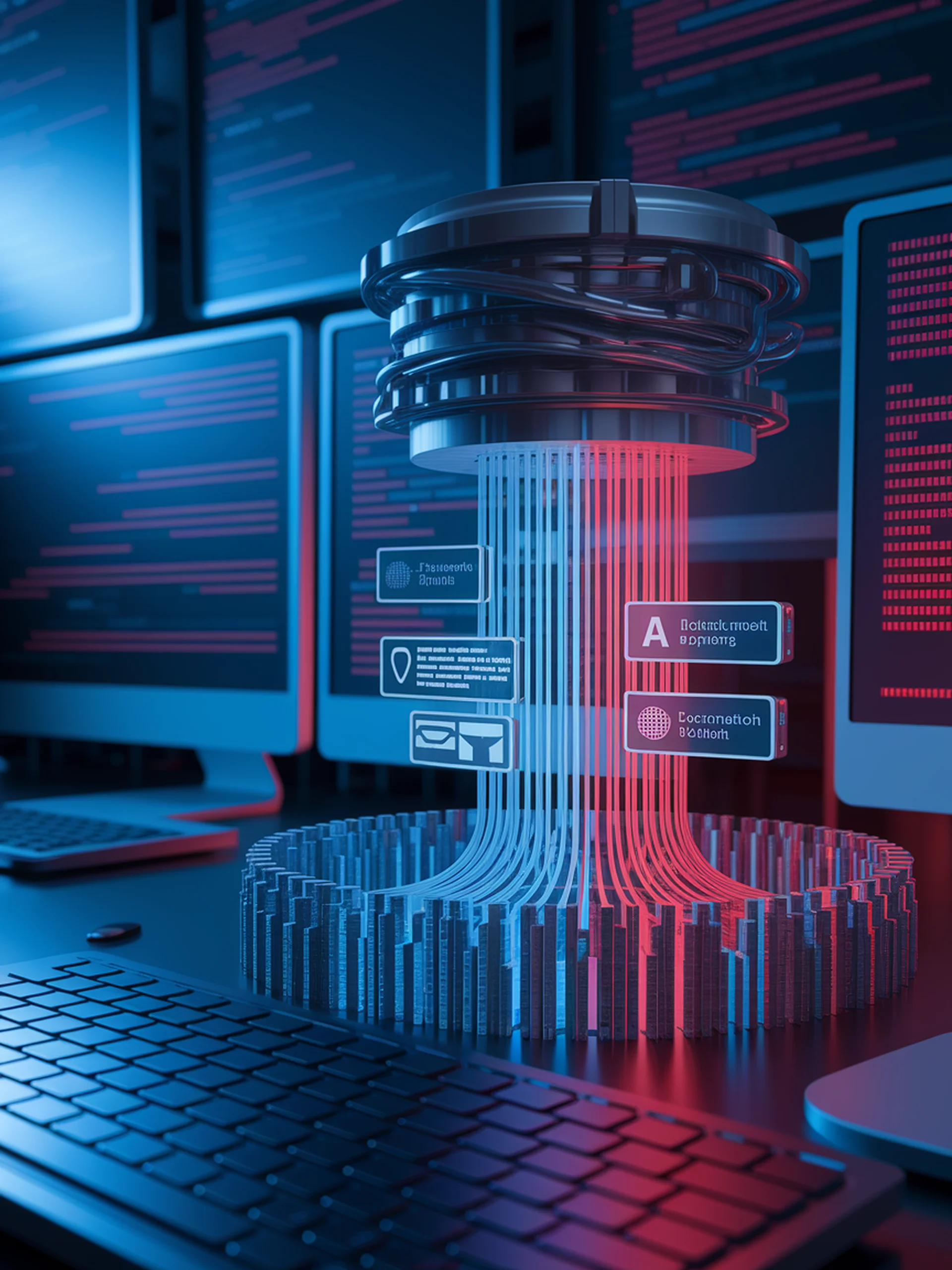
LLMs: The New Threat to Personal Data
How AI models excel at extracting personal information and what we can do about it
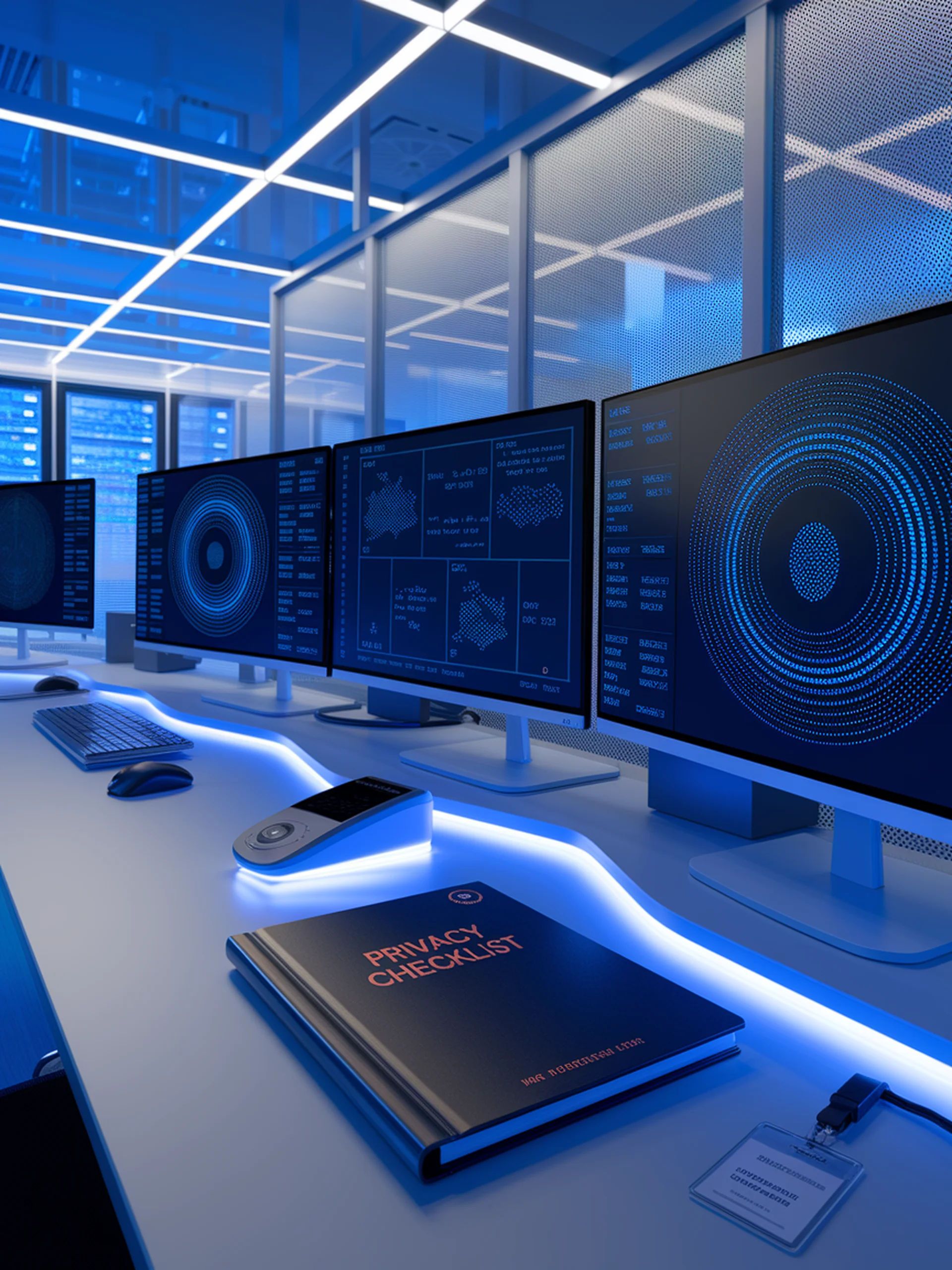
Privacy Violation Detection Framework
A Contextual Integrity Approach to Automated Privacy Monitoring
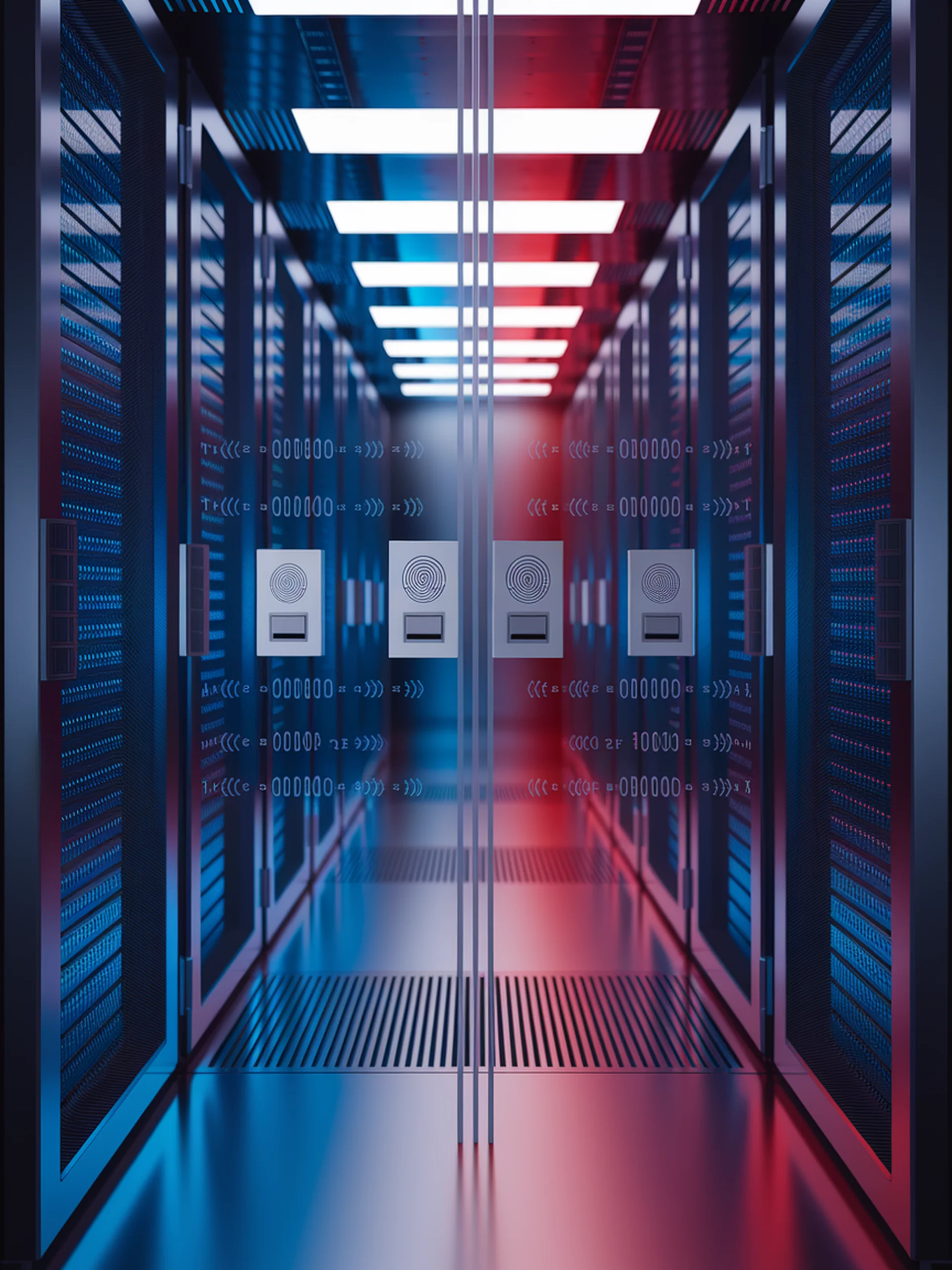
Securing LLMs with Access Control
Protecting sensitive data while maintaining model performance
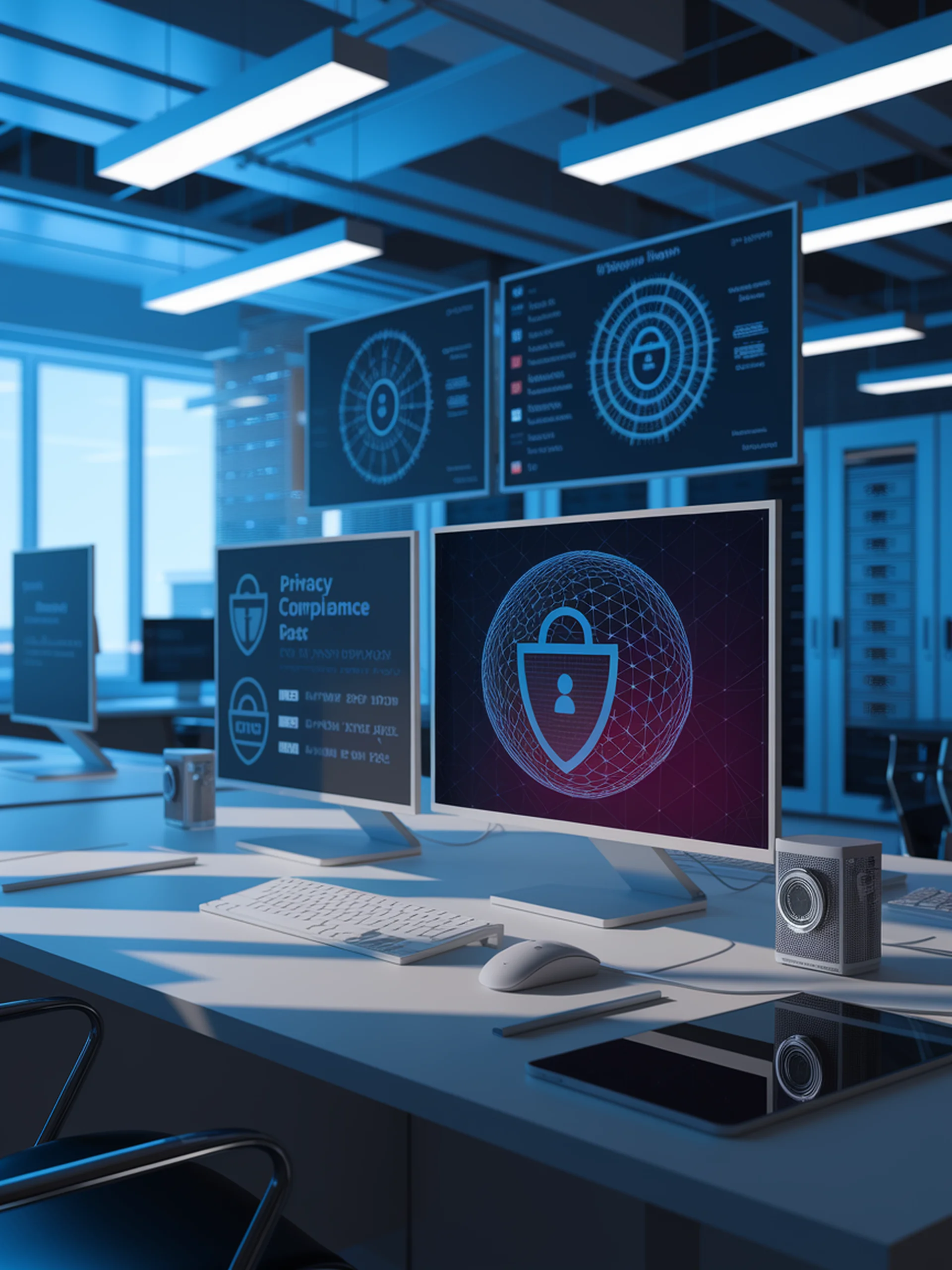
Privacy Readiness of Large Language Models
Evaluating LLMs as tools for privacy compliance and technical review
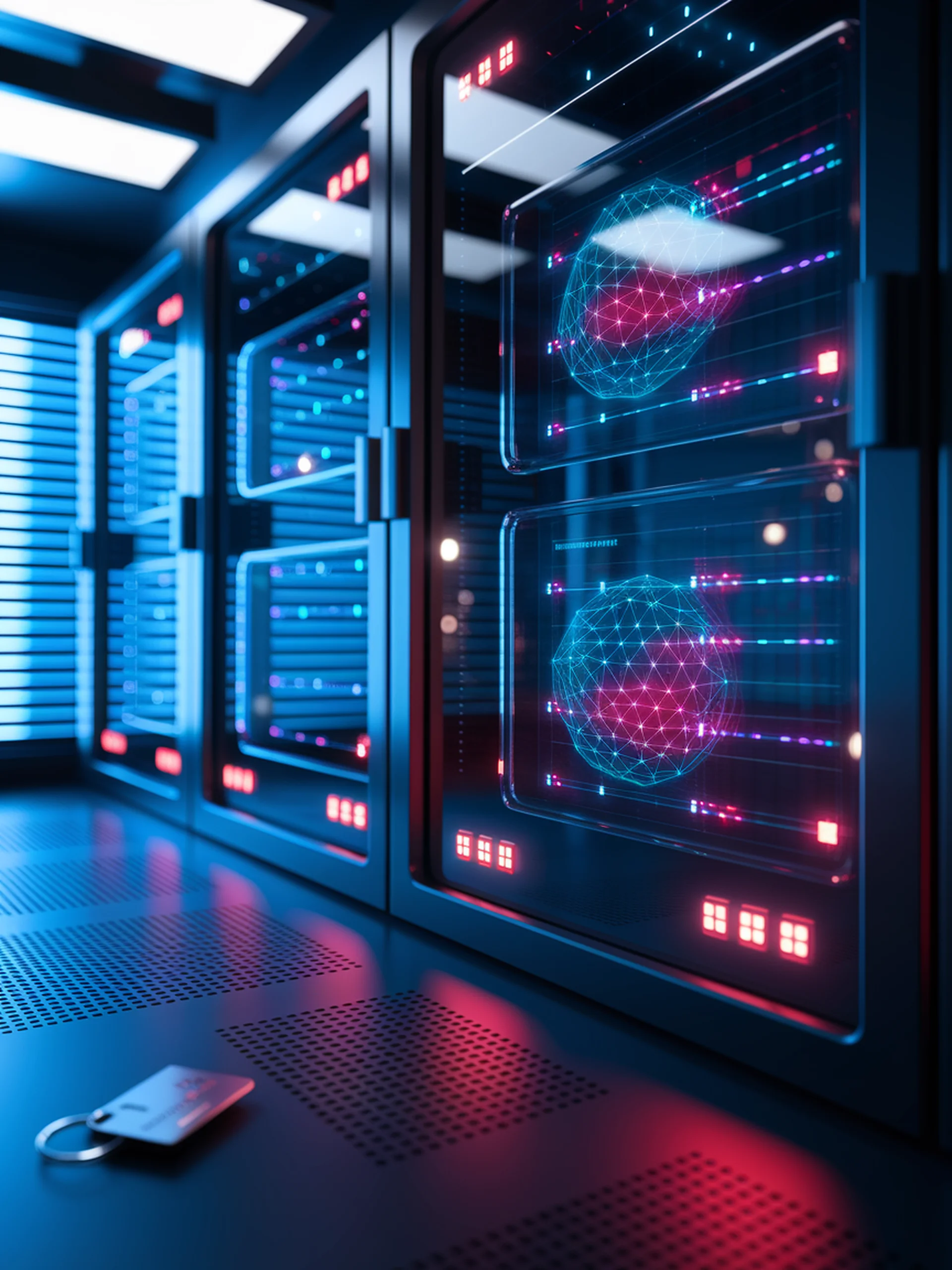
Privacy Bias in LLMs: A Hidden Threat
Examining systemic privacy issues in language model training data
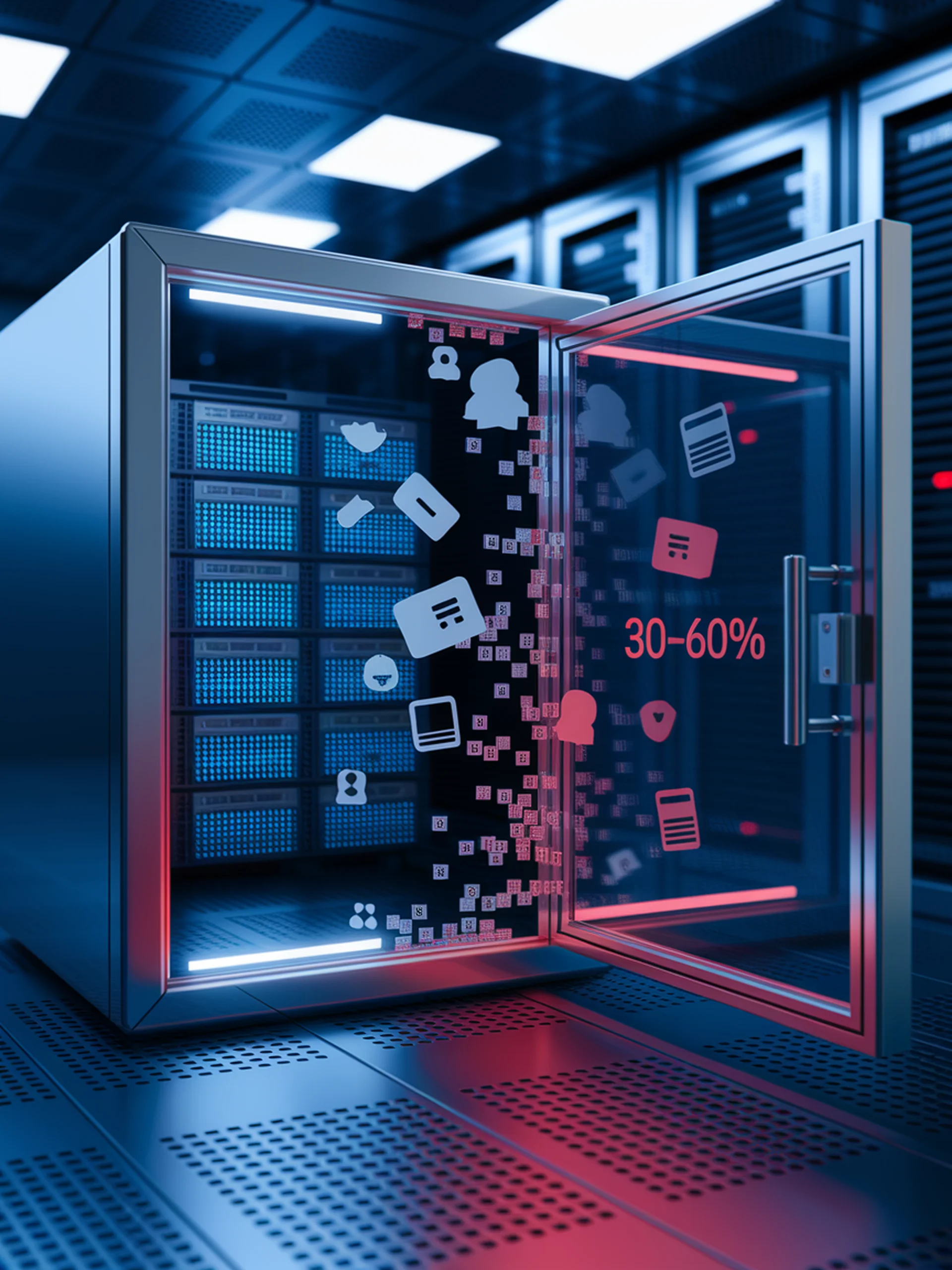
The False Privacy of Synthetic Data
Why generated data doesn't solve LLM privacy concerns
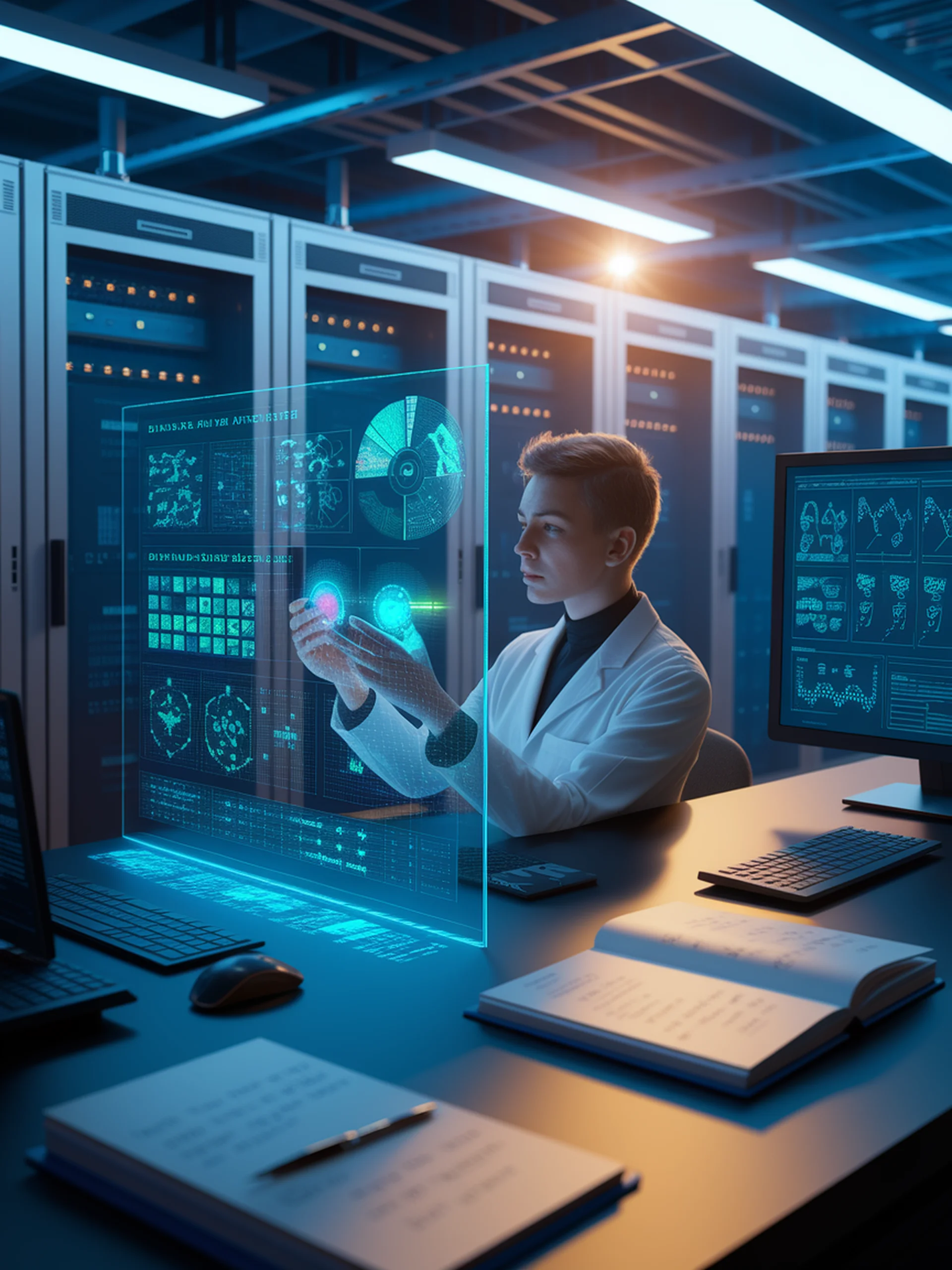
Detecting LLM Training Data
A New Method for Transparency in AI Models
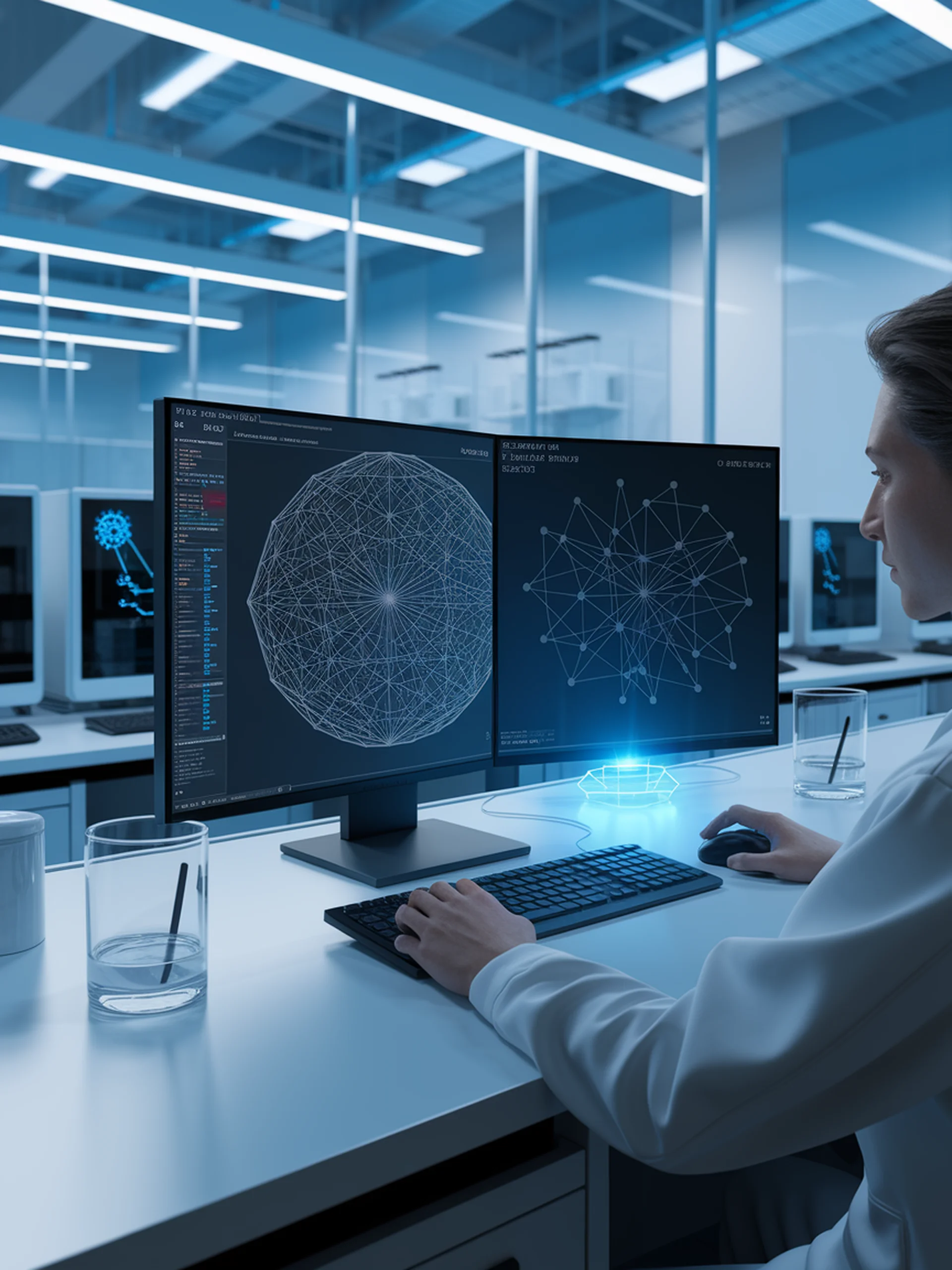
The Hidden Privacy Benefits of Low-Rank Adaptation
How LoRA and FLoRA inherently protect privacy in language models
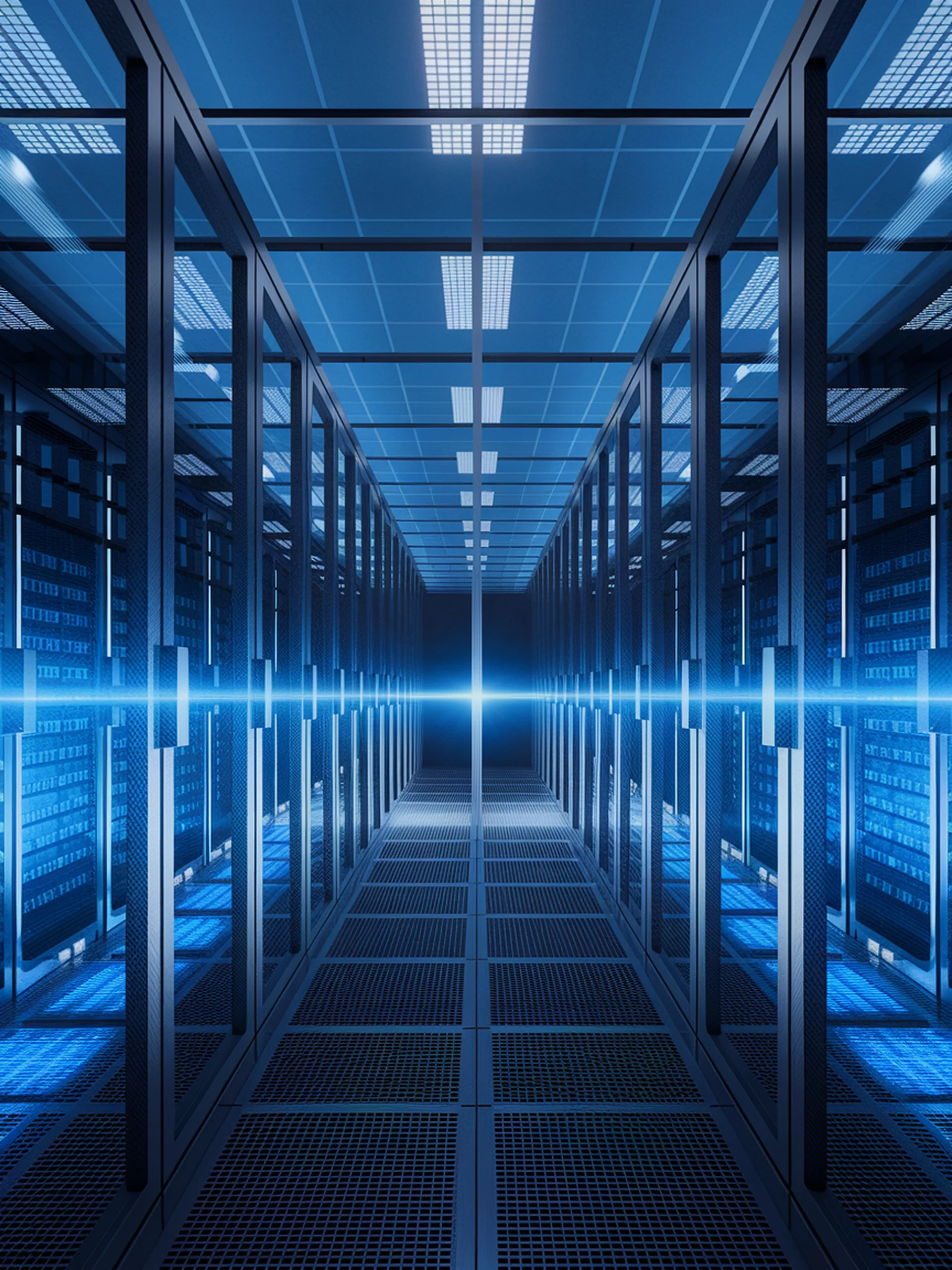
Protecting User Data in Cloud LLMs
Innovative approach to secure prompts without compromising performance
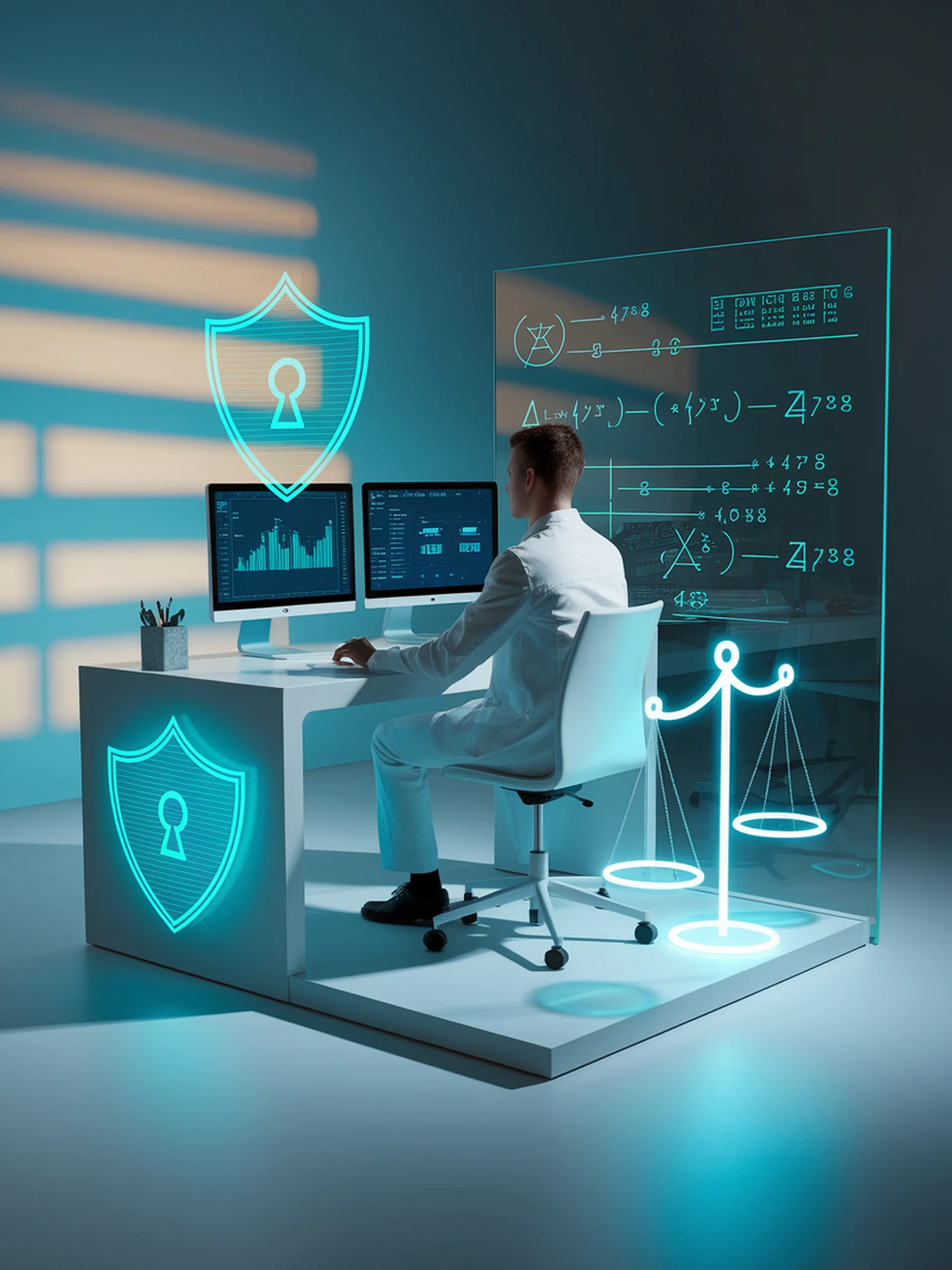
Balancing Privacy & Data Selection in Machine Learning
A novel approach to privacy-preserving active learning
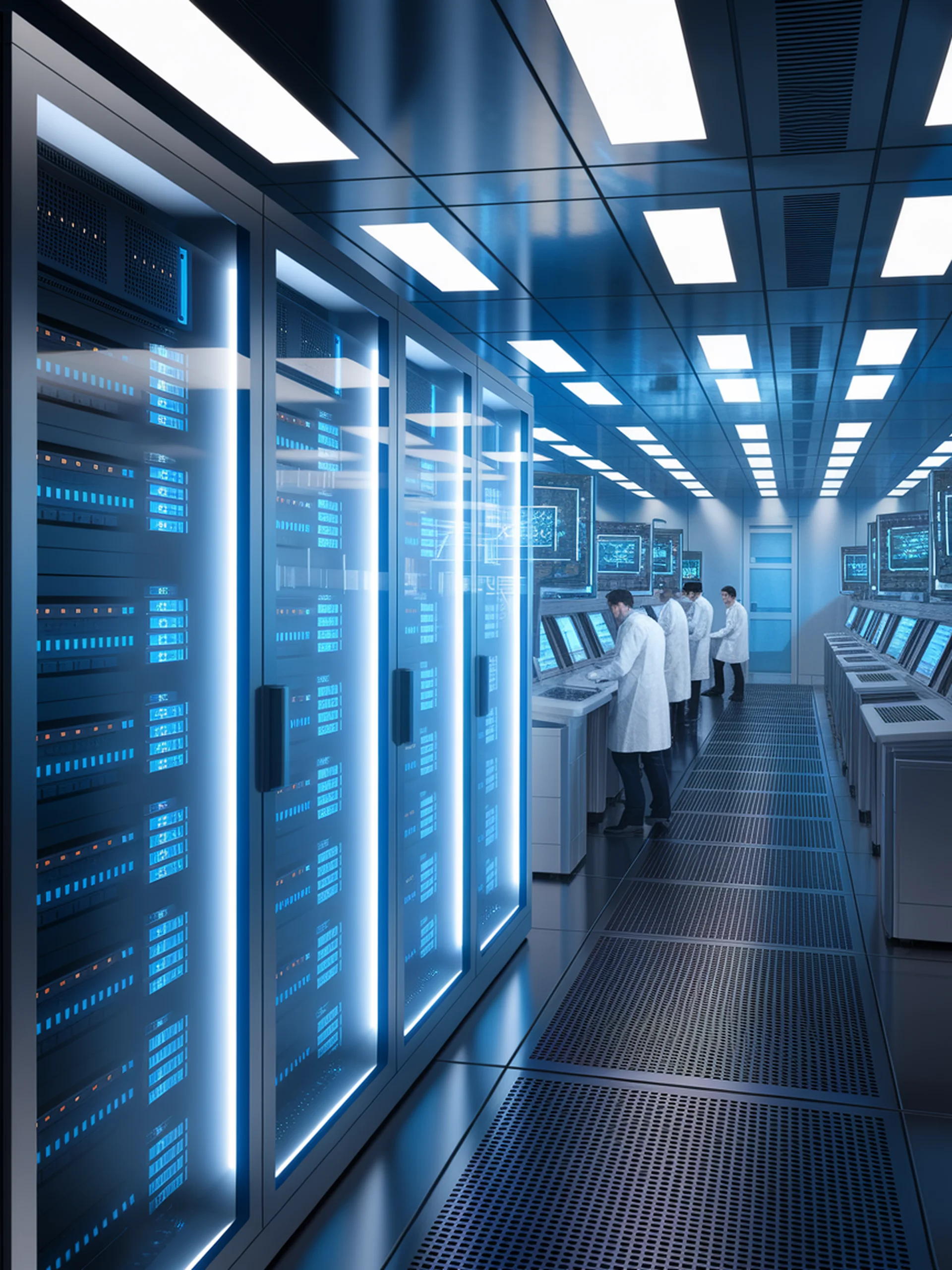
Securing LLMs with Encrypted Computation
A novel architecture for privacy-preserving language models
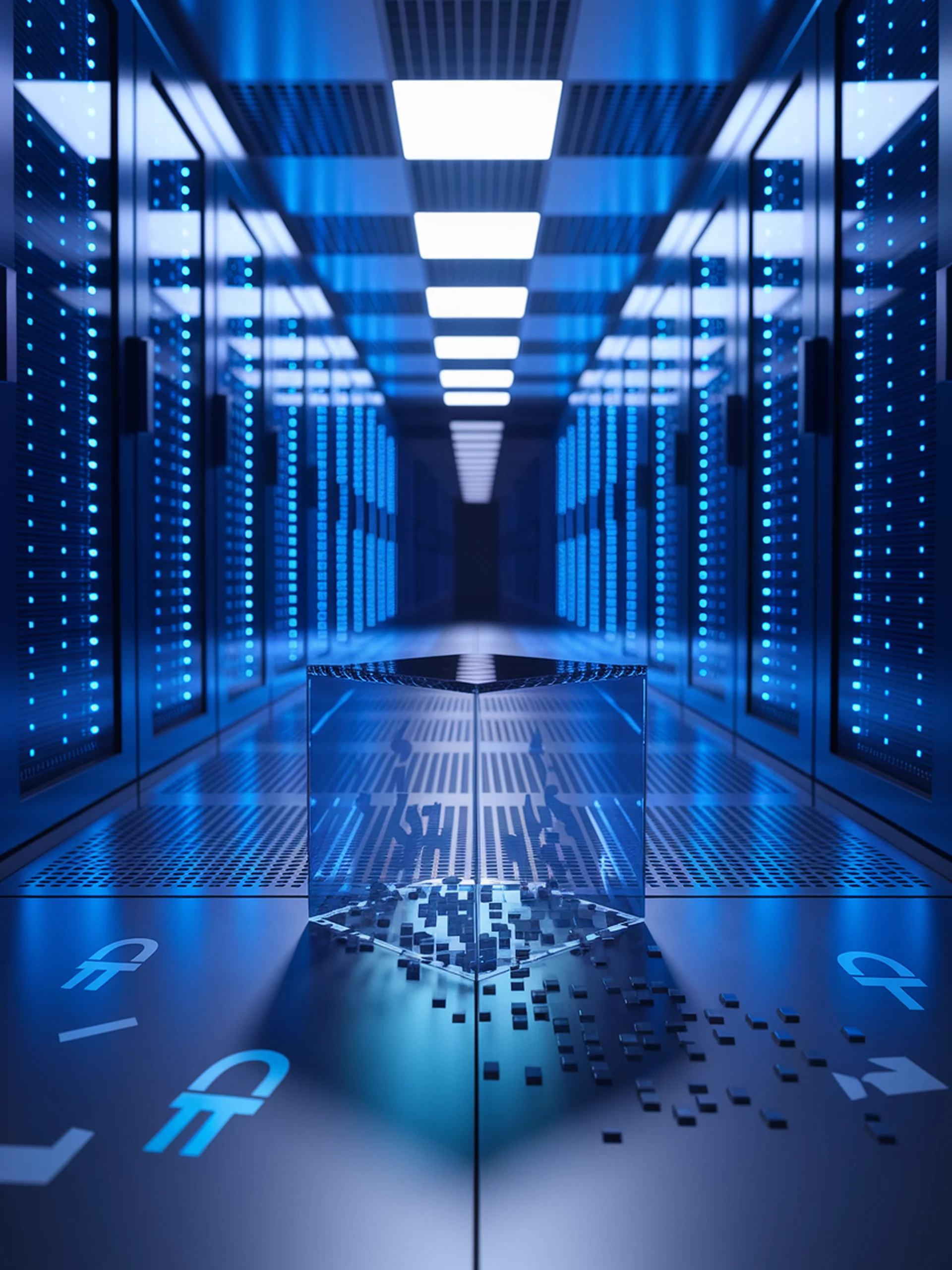
The Hidden Risks of Memorization in LLMs
Understanding privacy and security vulnerabilities in AI systems
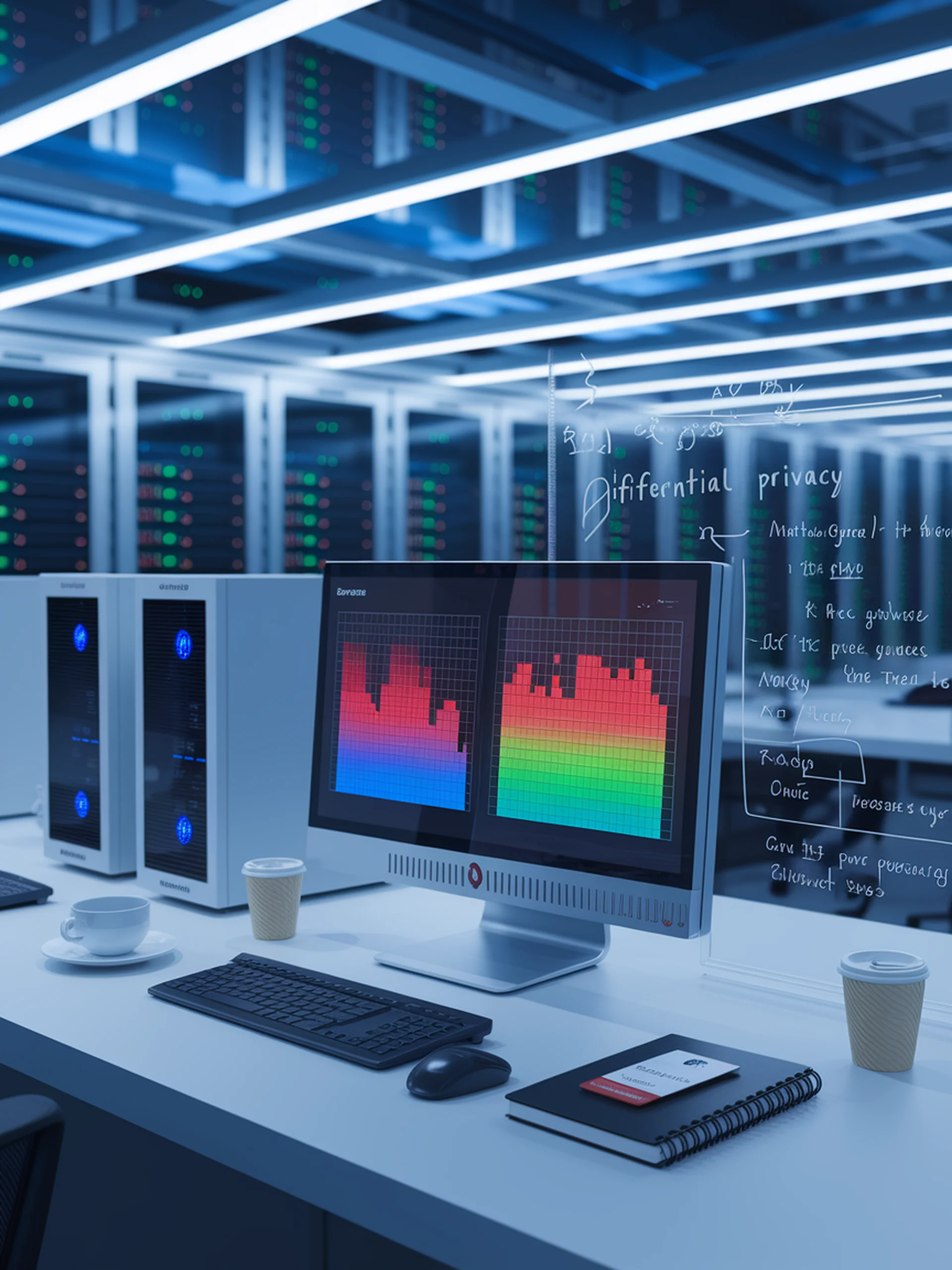
Improving Privacy in Machine Learning Models
A new heuristic analysis for DP-SGD's last iterate advantage
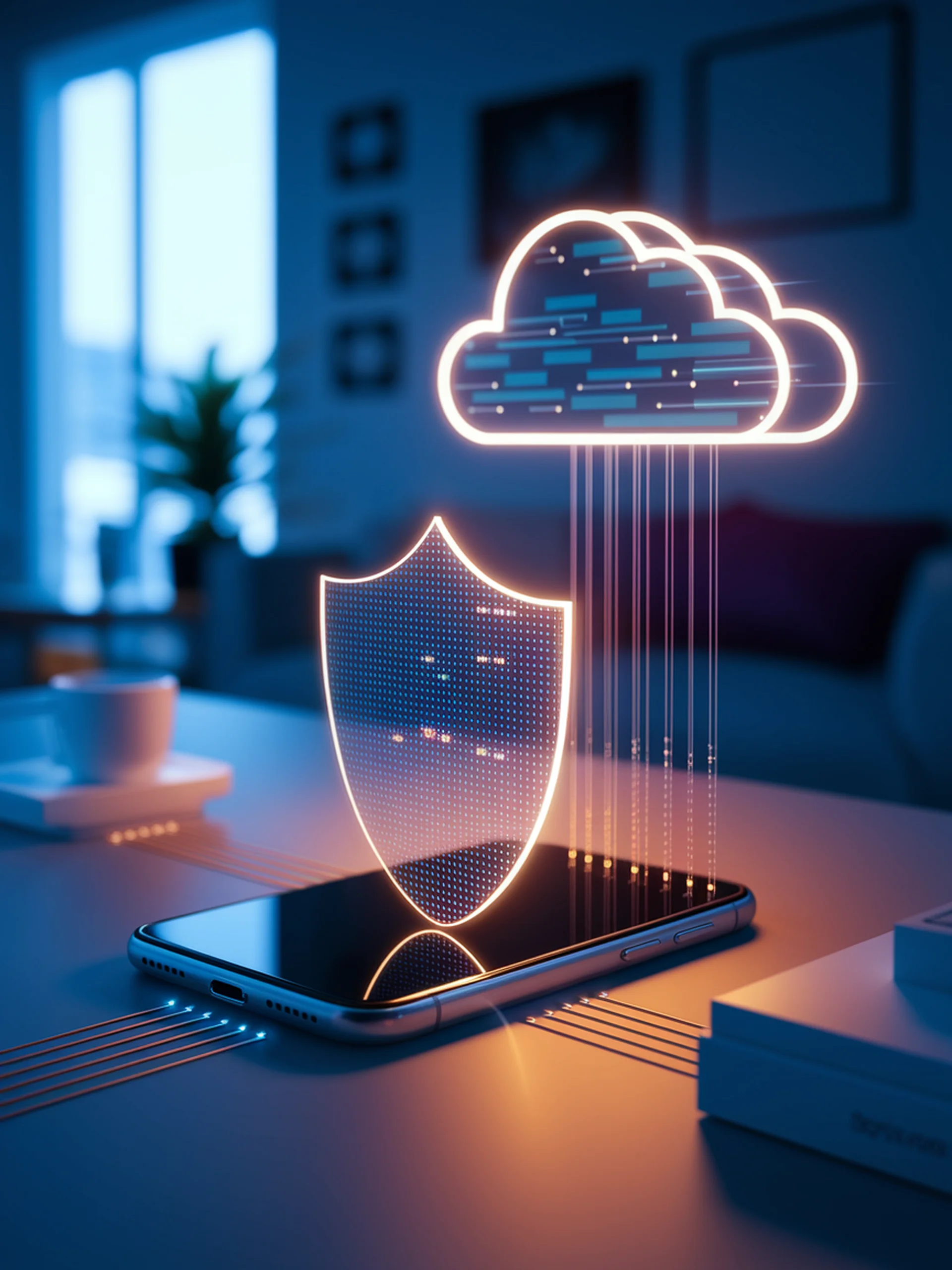
Smarter Privacy for On-Device AI
A novel framework for secure local-to-cloud AI decision making
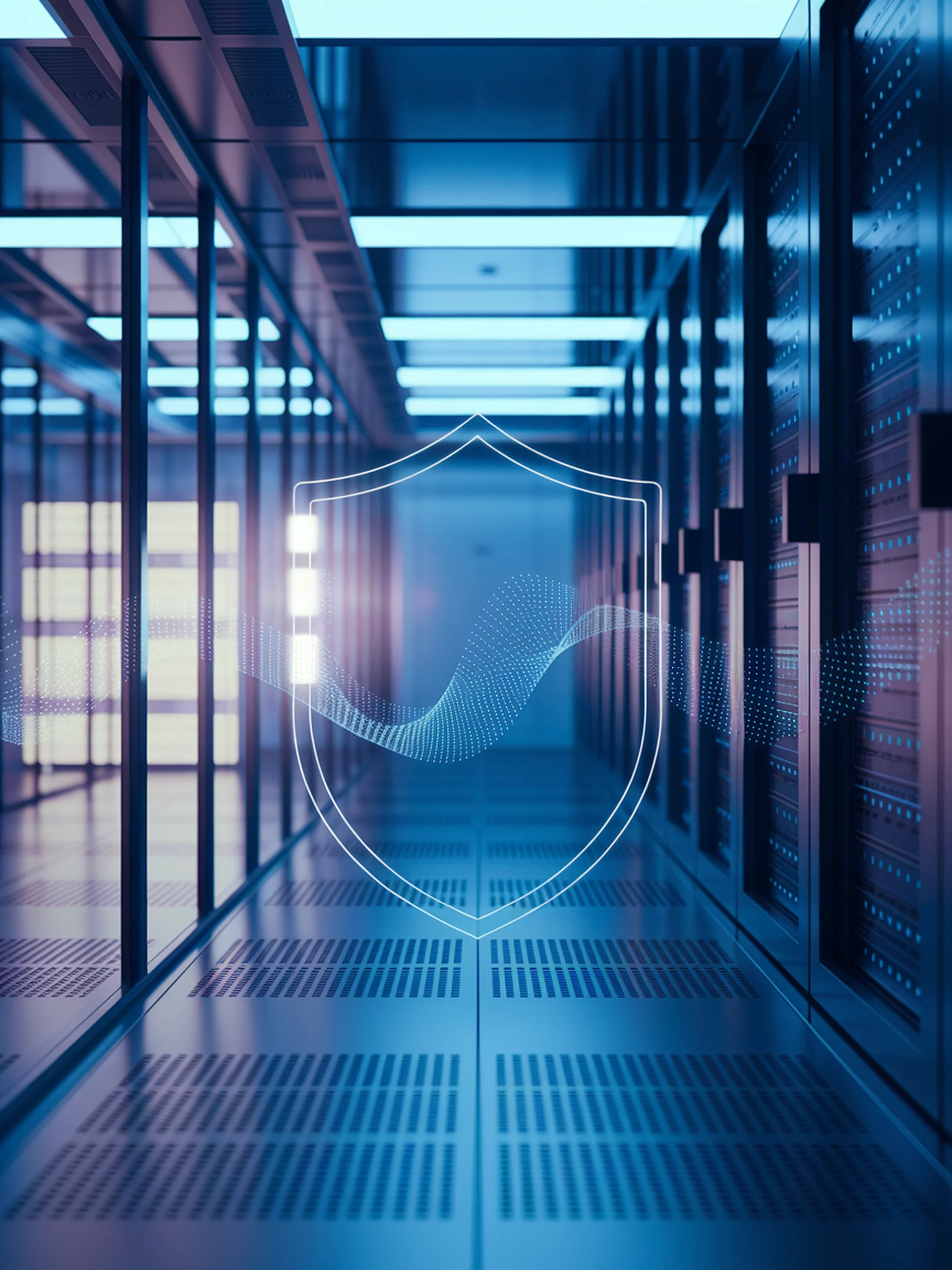
Privacy-Preserving Knowledge Transfer for LLMs
A model-based approach that balances utility and privacy
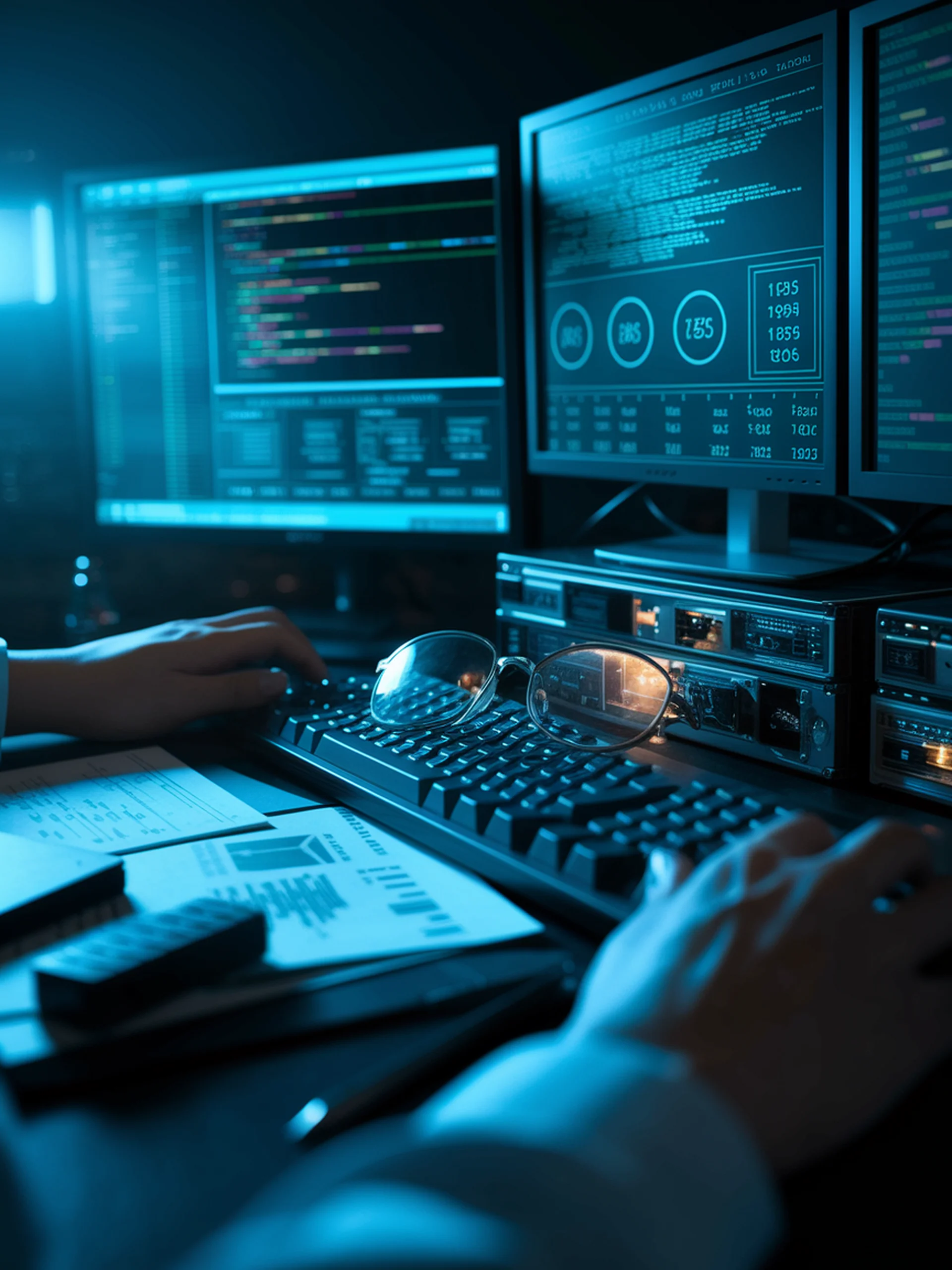
Detecting LLM Training Data
New Fine-tuning Method Improves Detection of Pretraining Data
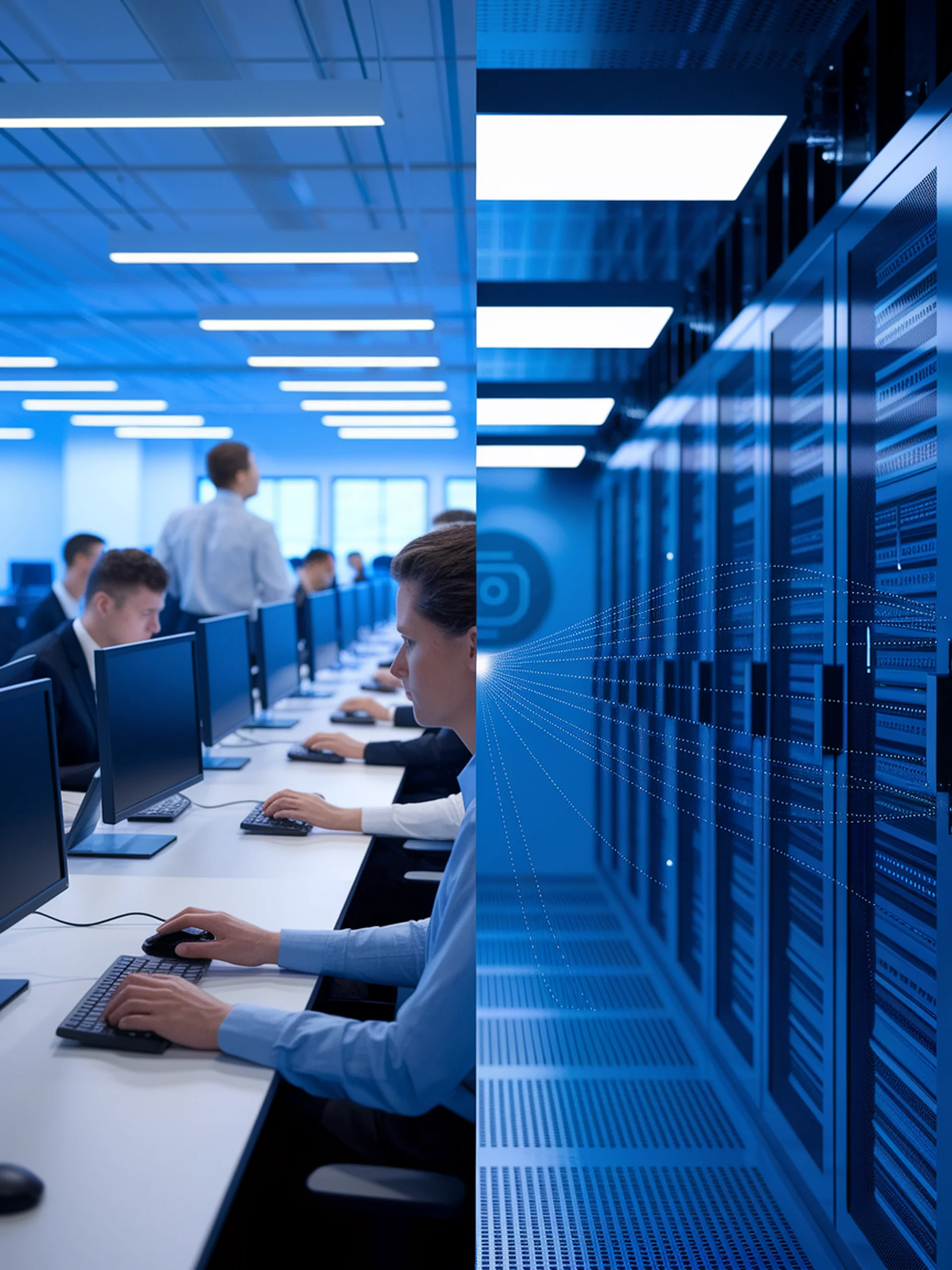
Enhancing LLM Training With Privacy-Preserving Quality Control
A federated approach to filter low-quality data without compromising privacy
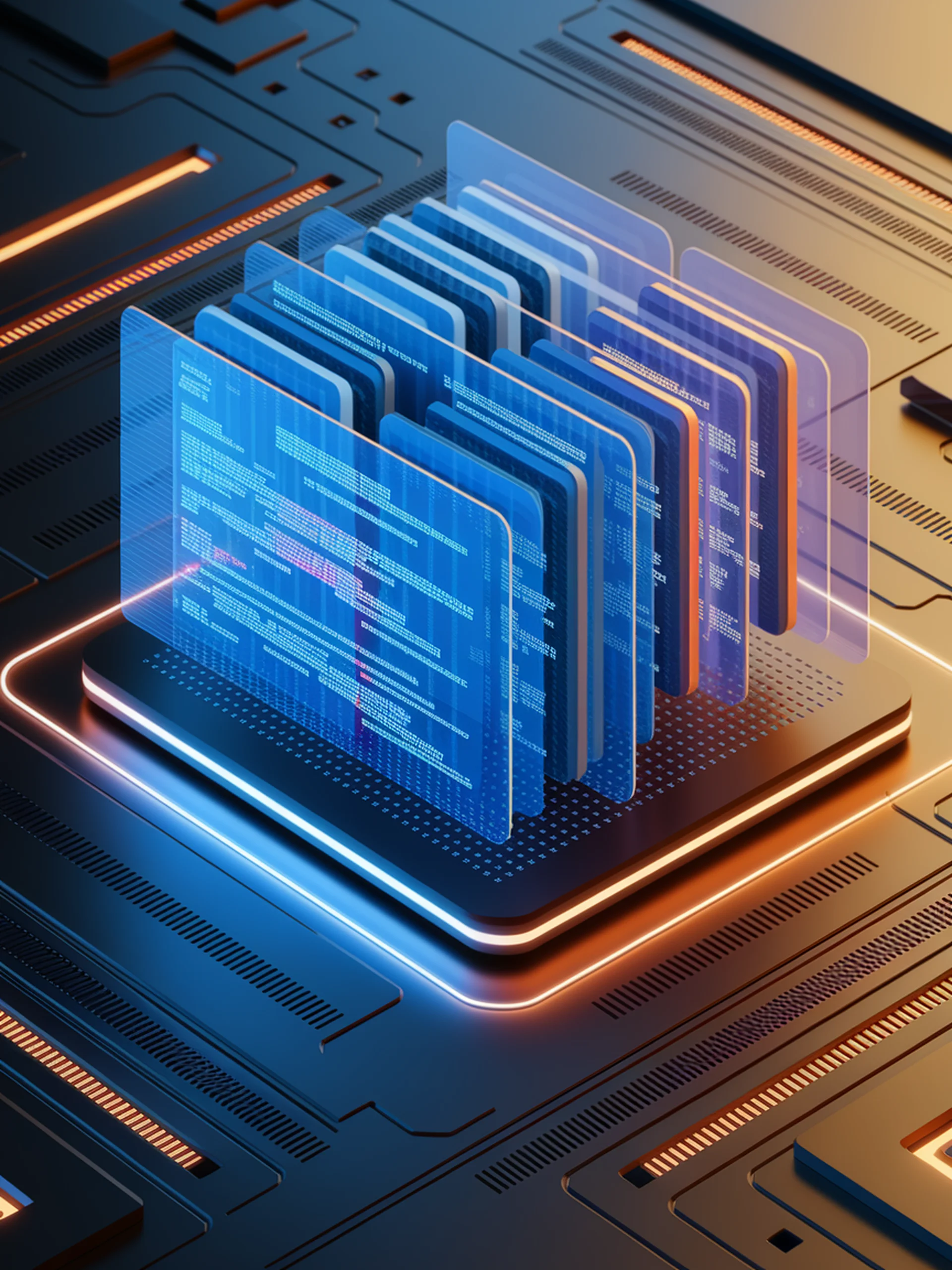
Protecting Privacy in AI Language Models
A Novel Approach to Secure In-Context Learning with Differential Privacy
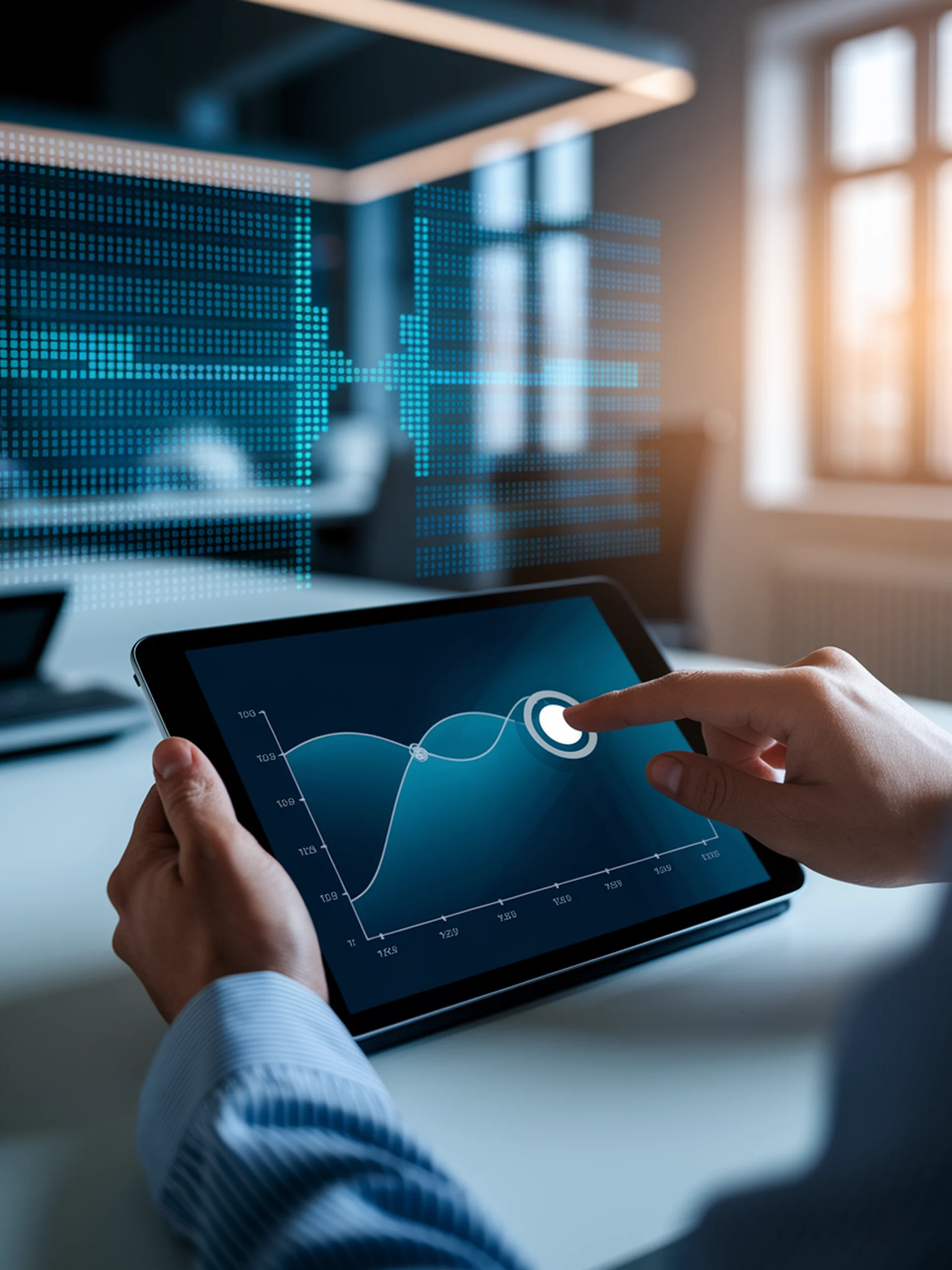
Balancing Privacy & AI Performance
User-controlled anonymization for safer LLM interactions
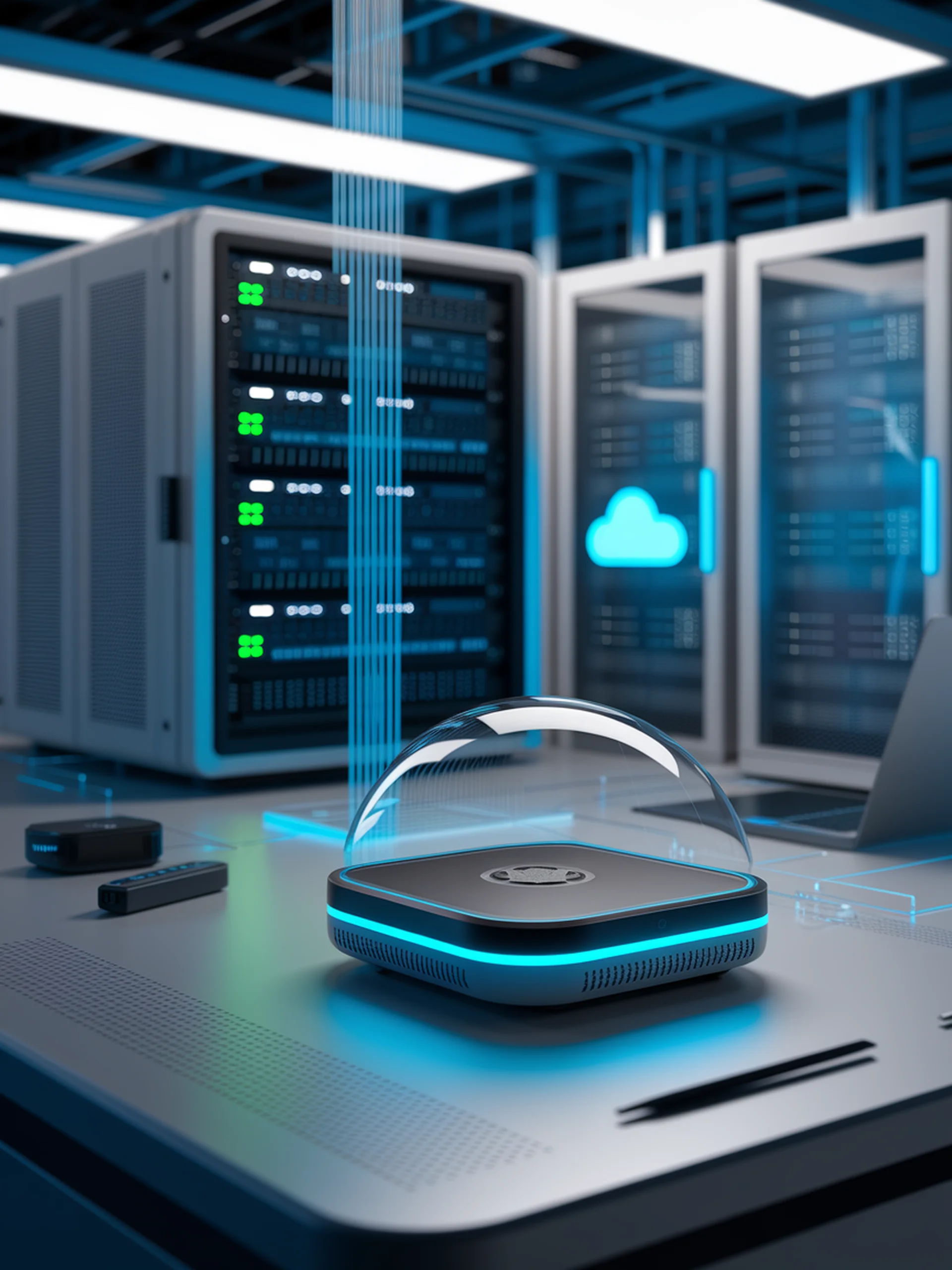
Privacy-First AI Assistants
Balancing Capability and Confidentiality through Model Delegation
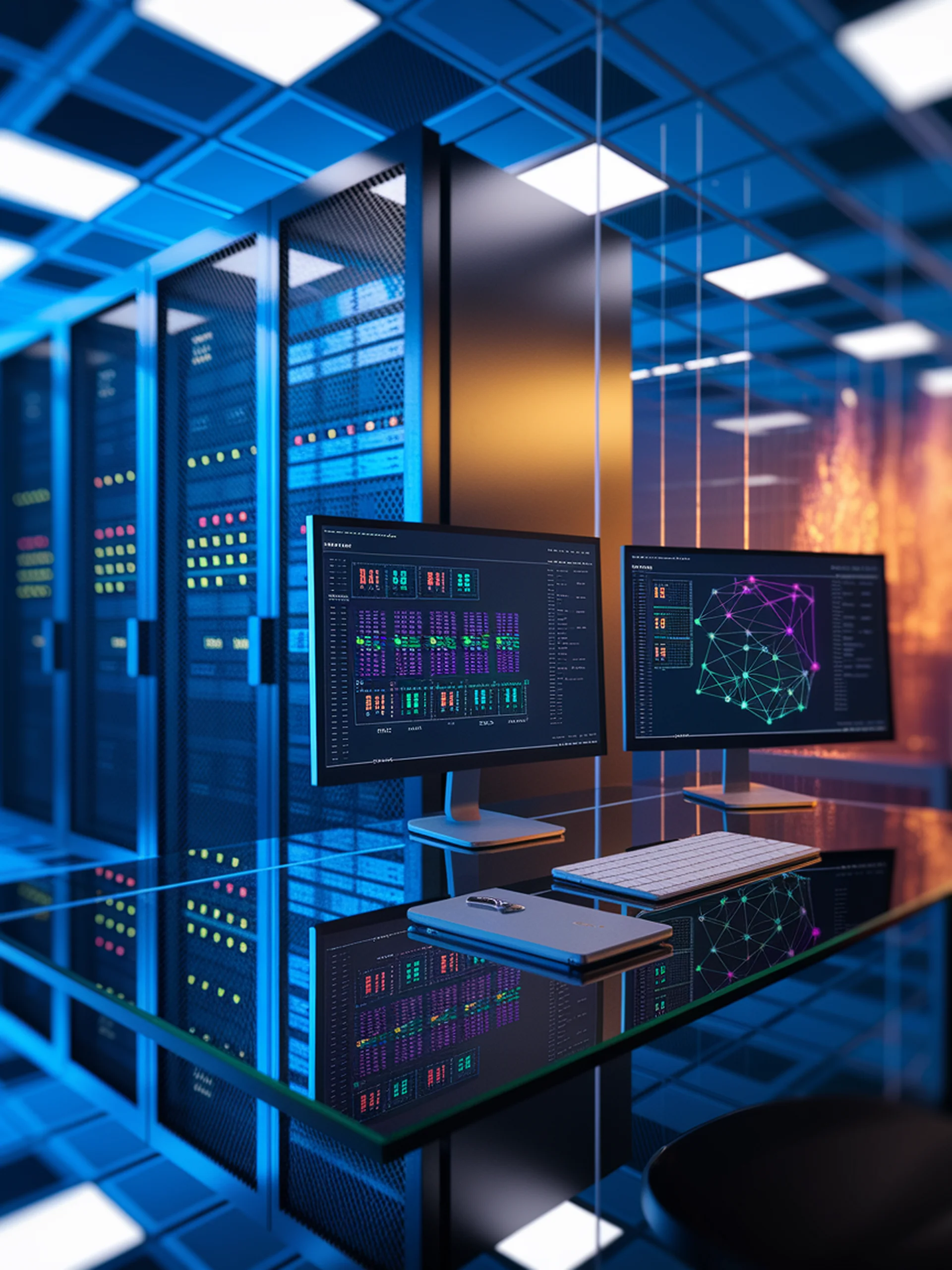
Uncovering the Hidden Memories of LLMs
A New Framework to Measure Privacy Risks in AI Models
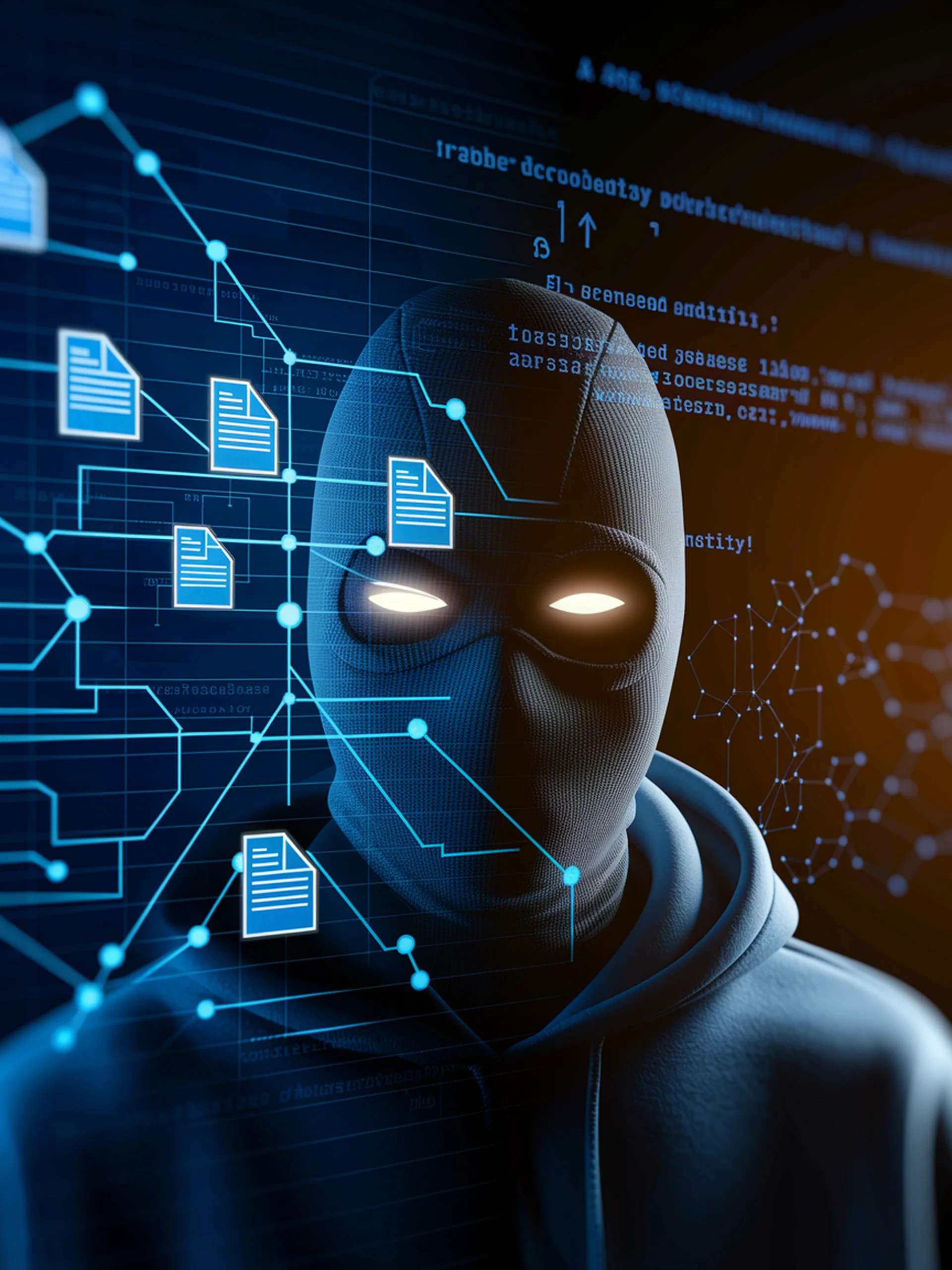
Unmasking the Vulnerabilities in RAG Systems
Novel attack methods reveal security risks in retrieval-augmented LLMs
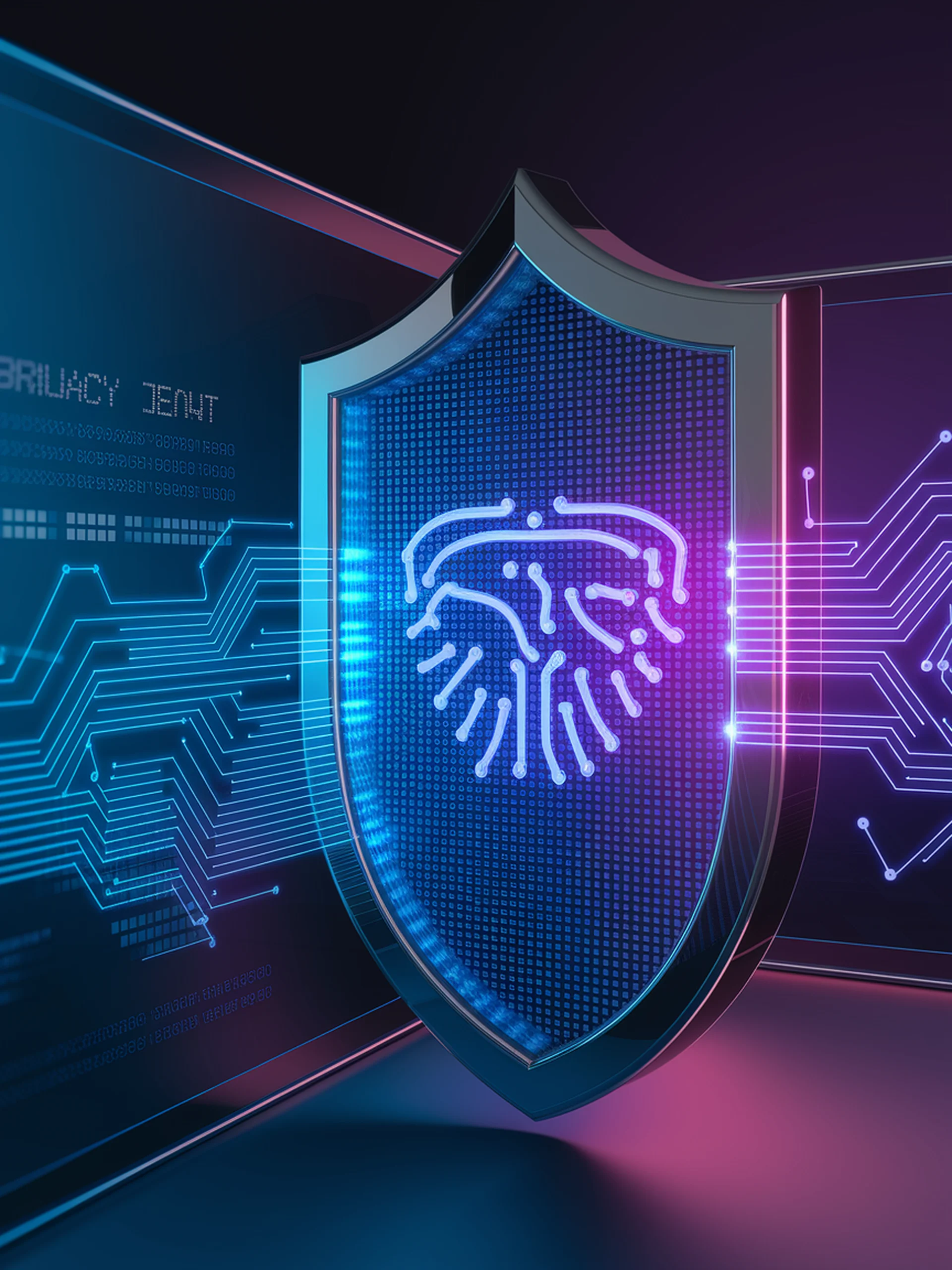
Safeguarding Privacy in Multimodal AI
Introducing MLLMU-Bench: The First Benchmark for Multimodal Model Privacy Protection
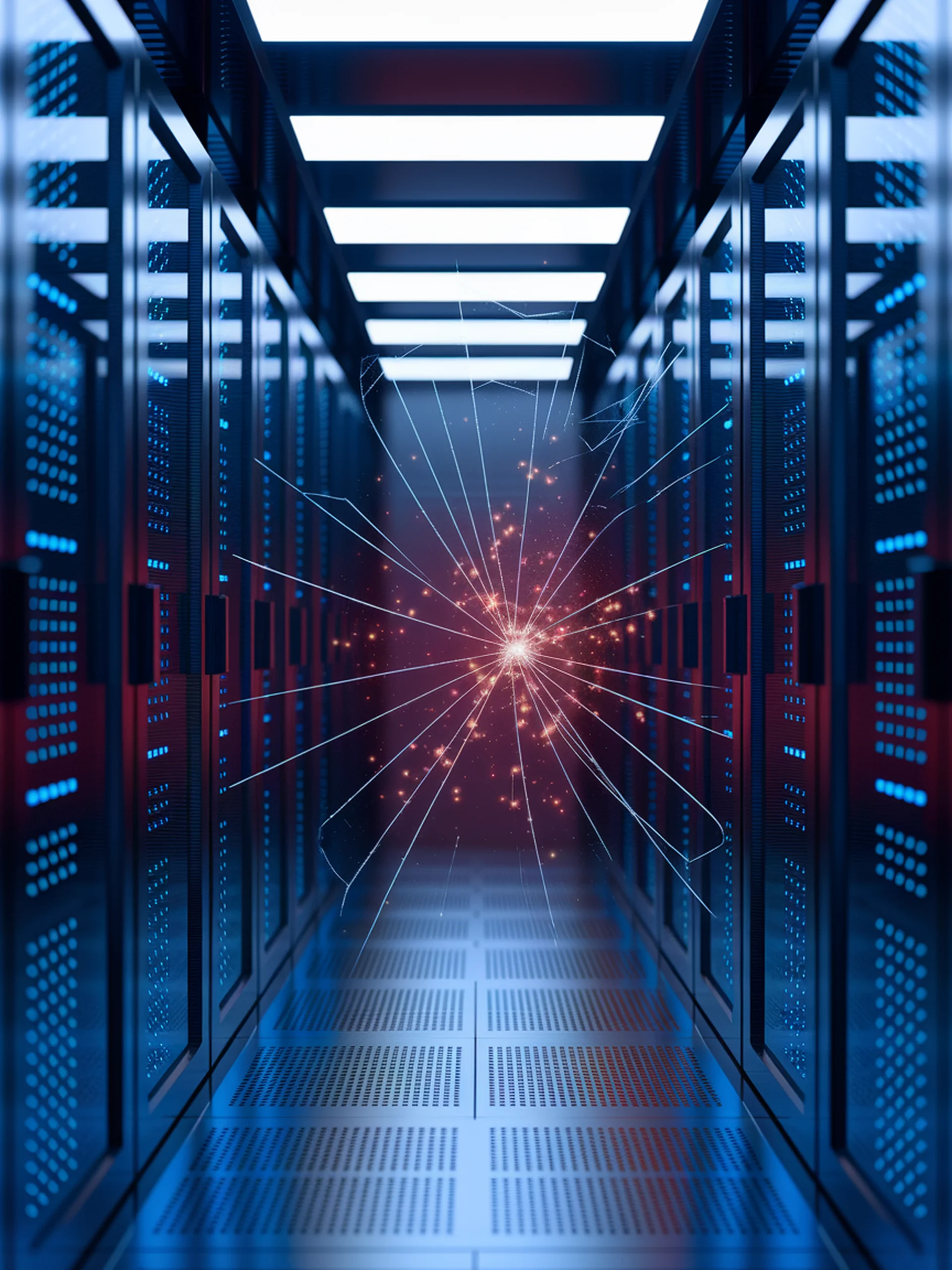
When Privacy Attacks Actually Work on LLMs
New evidence shows large language models are vulnerable to specific membership inference attacks
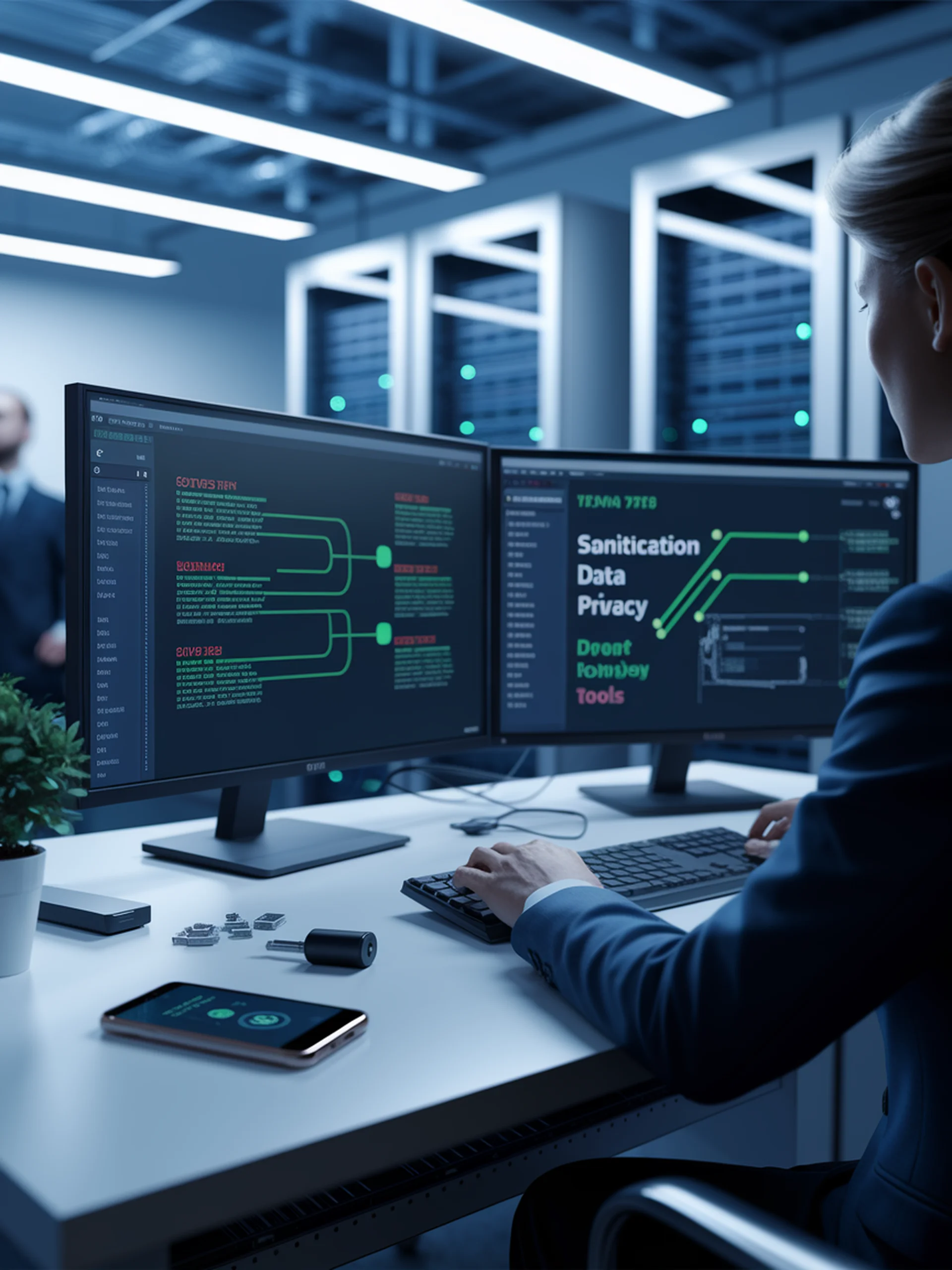
Protecting Privacy in LLM Interactions
Evaluating text sanitization effectiveness for resource-constrained environments
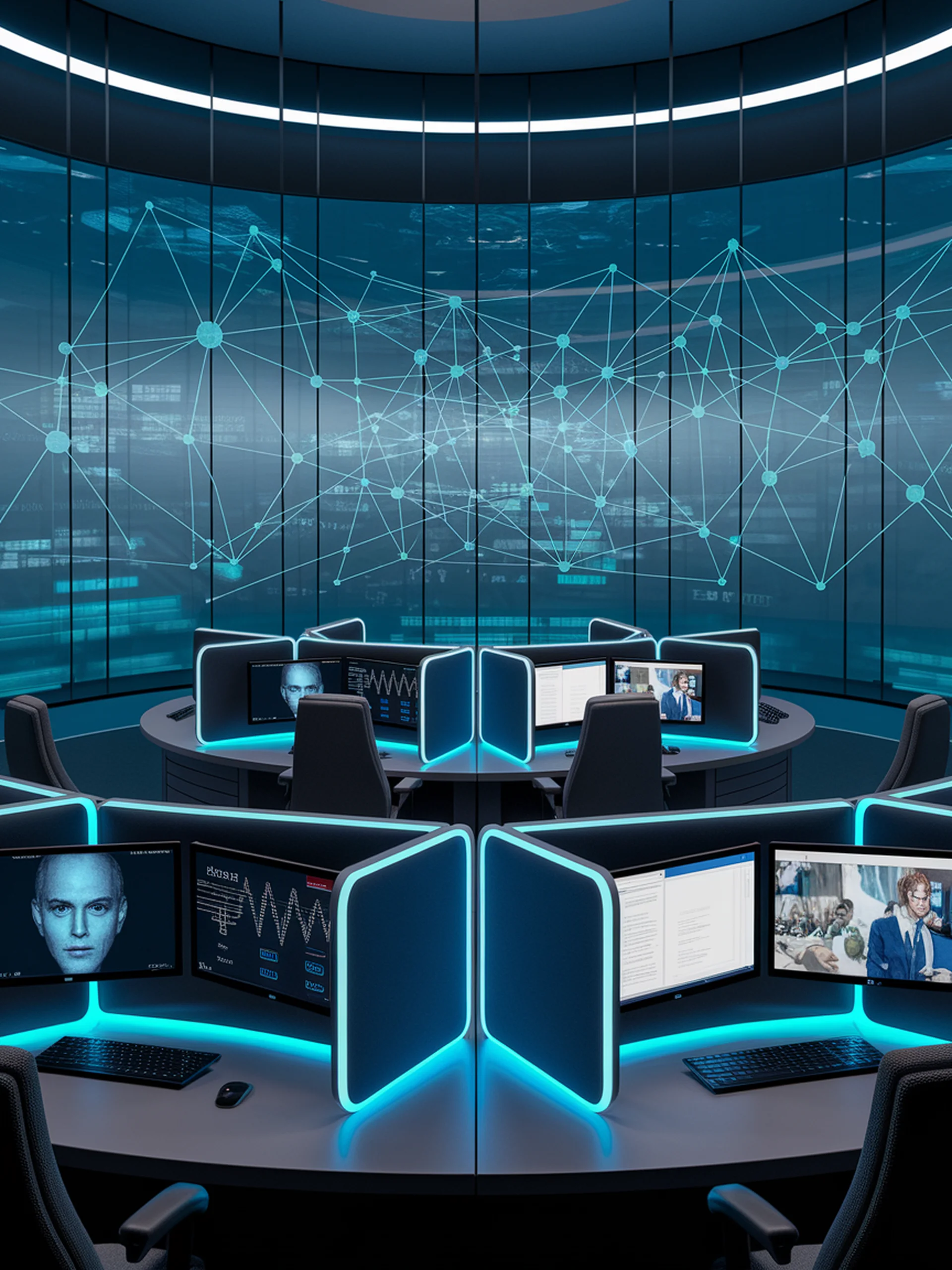
Federated Learning for Multimodal LLMs
Protecting Privacy While Training on Diverse Data Types
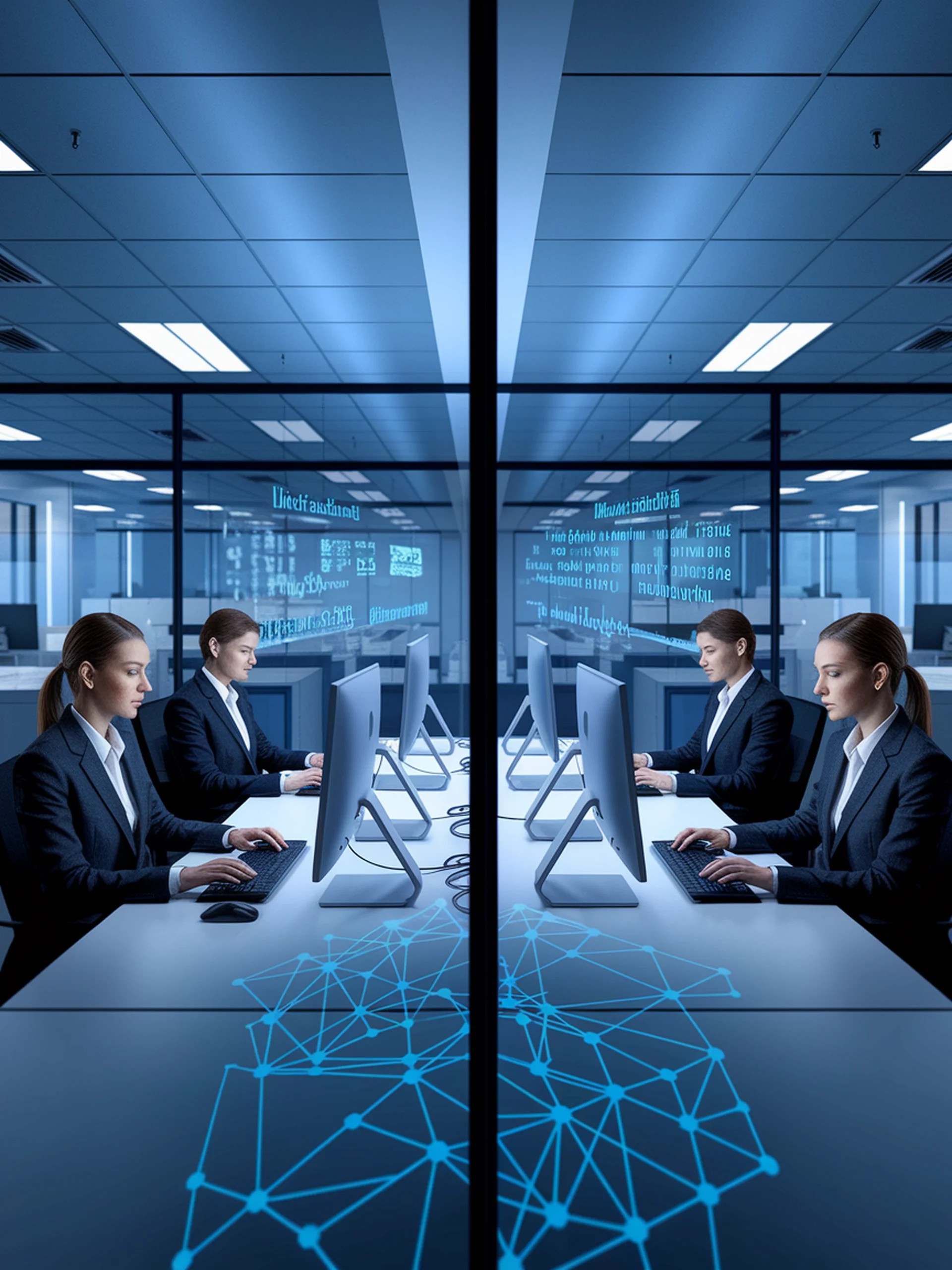
Secure LLM Fine-Tuning Without Data Sharing
Personalized federated learning for heterogeneous data environments
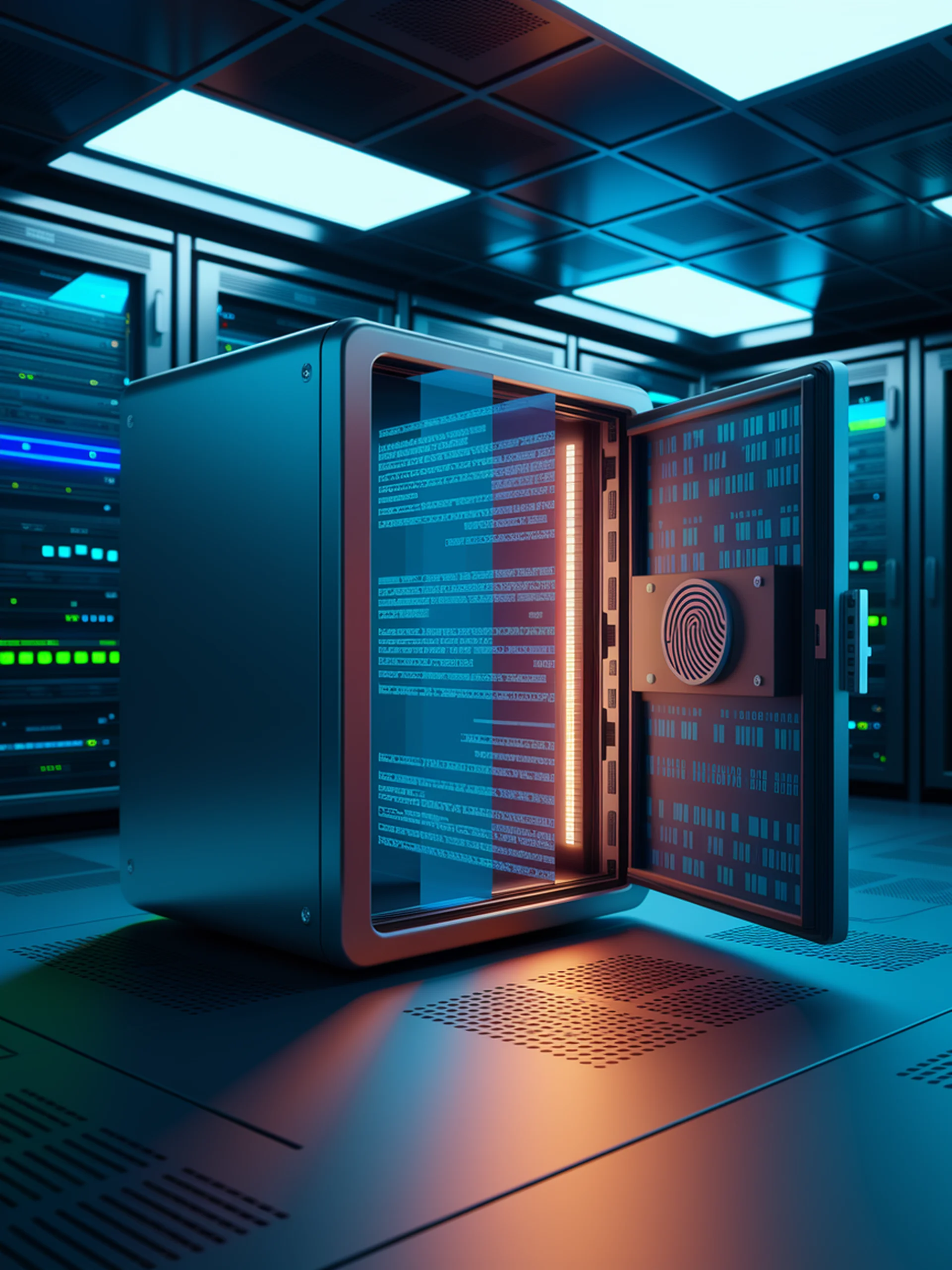
Securing RAG Systems with Privacy Guarantees
Protecting Sensitive Data in Retrieval-Augmented Generation
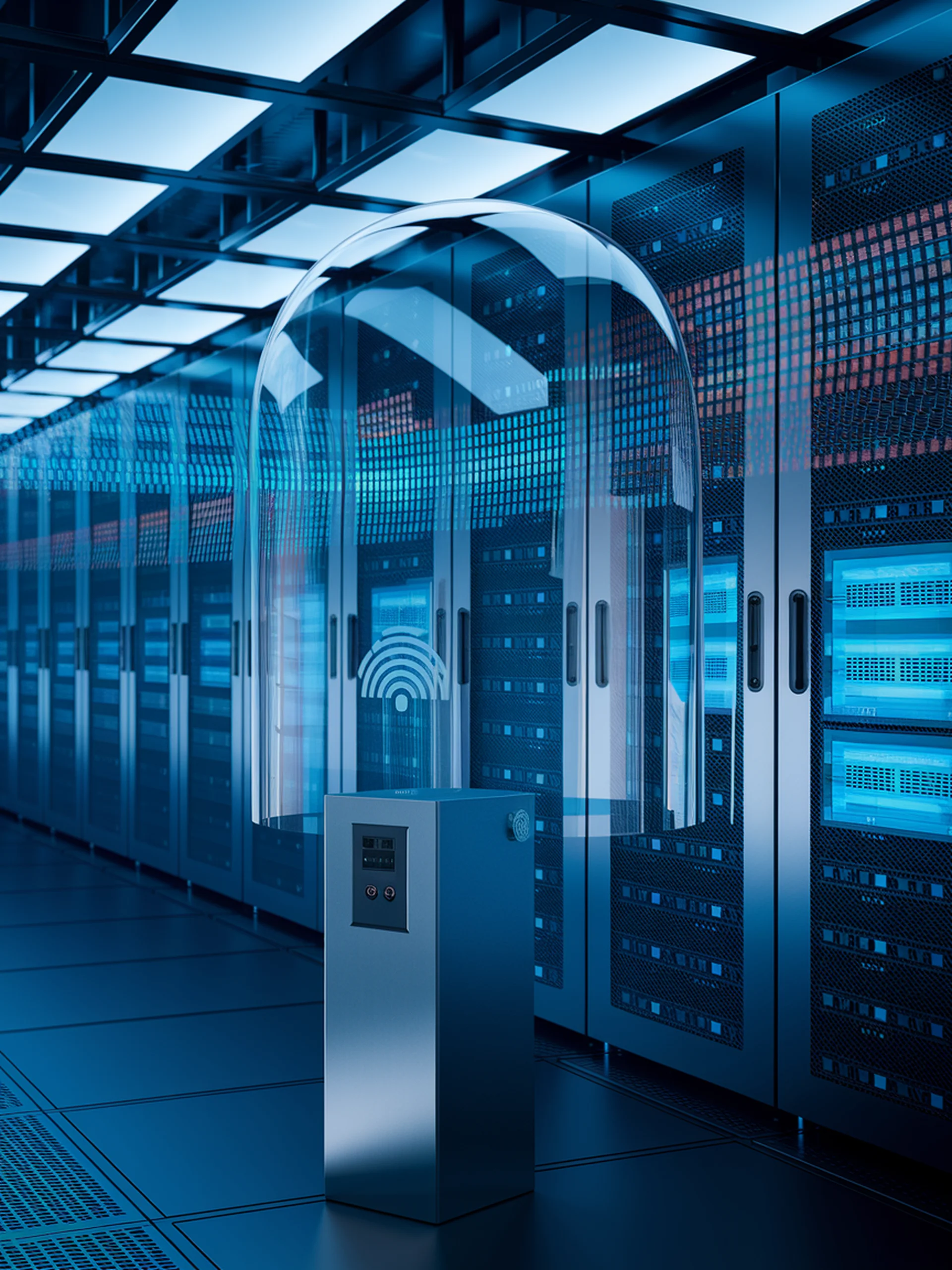
Protecting Privacy in LLM Fine-tuning
Understanding vulnerabilities and defenses for sensitive data protection
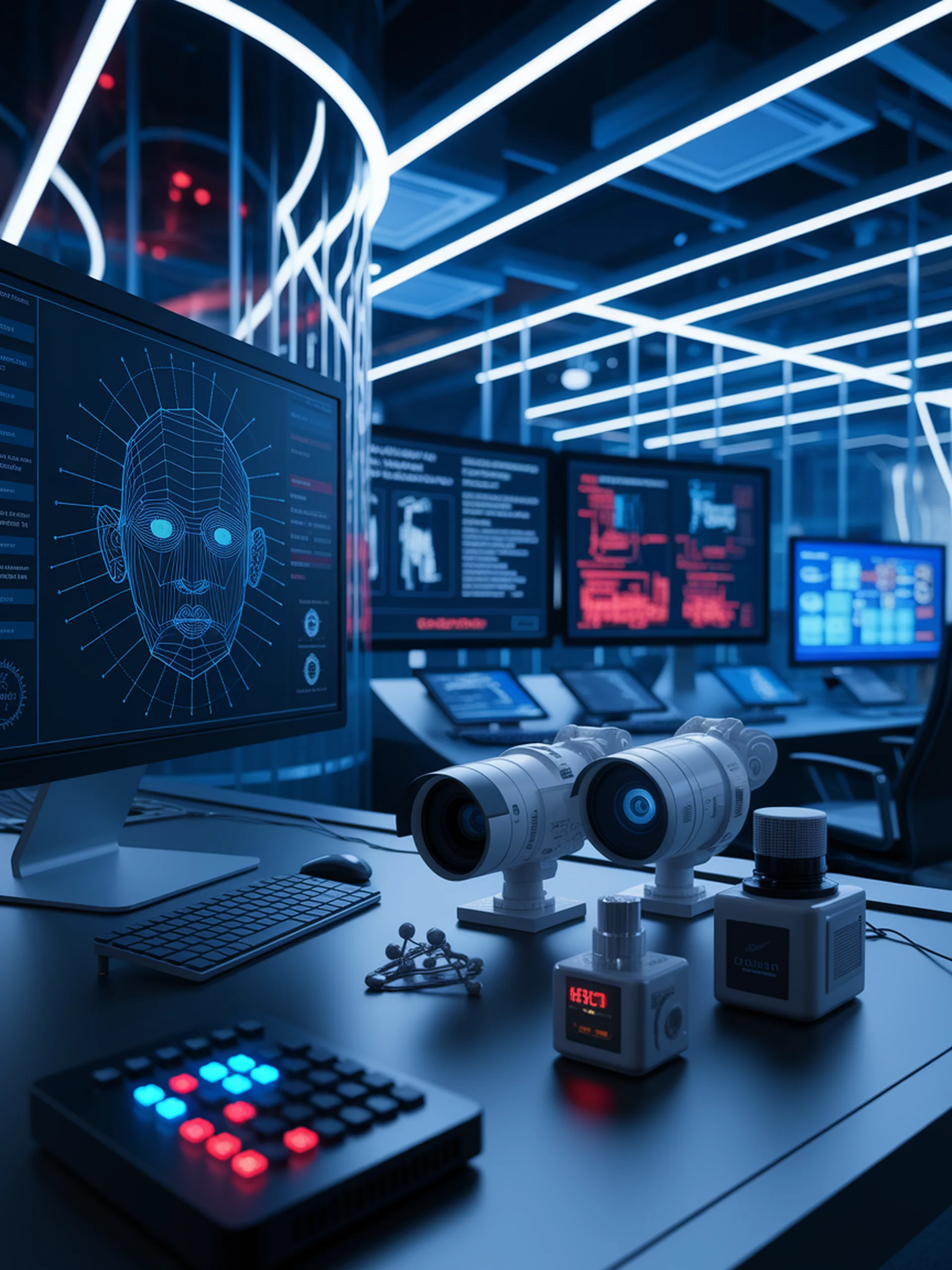
Privacy Assessment for Vision-Language AI
A multi-perspective benchmark for evaluating privacy risks in LVLMs
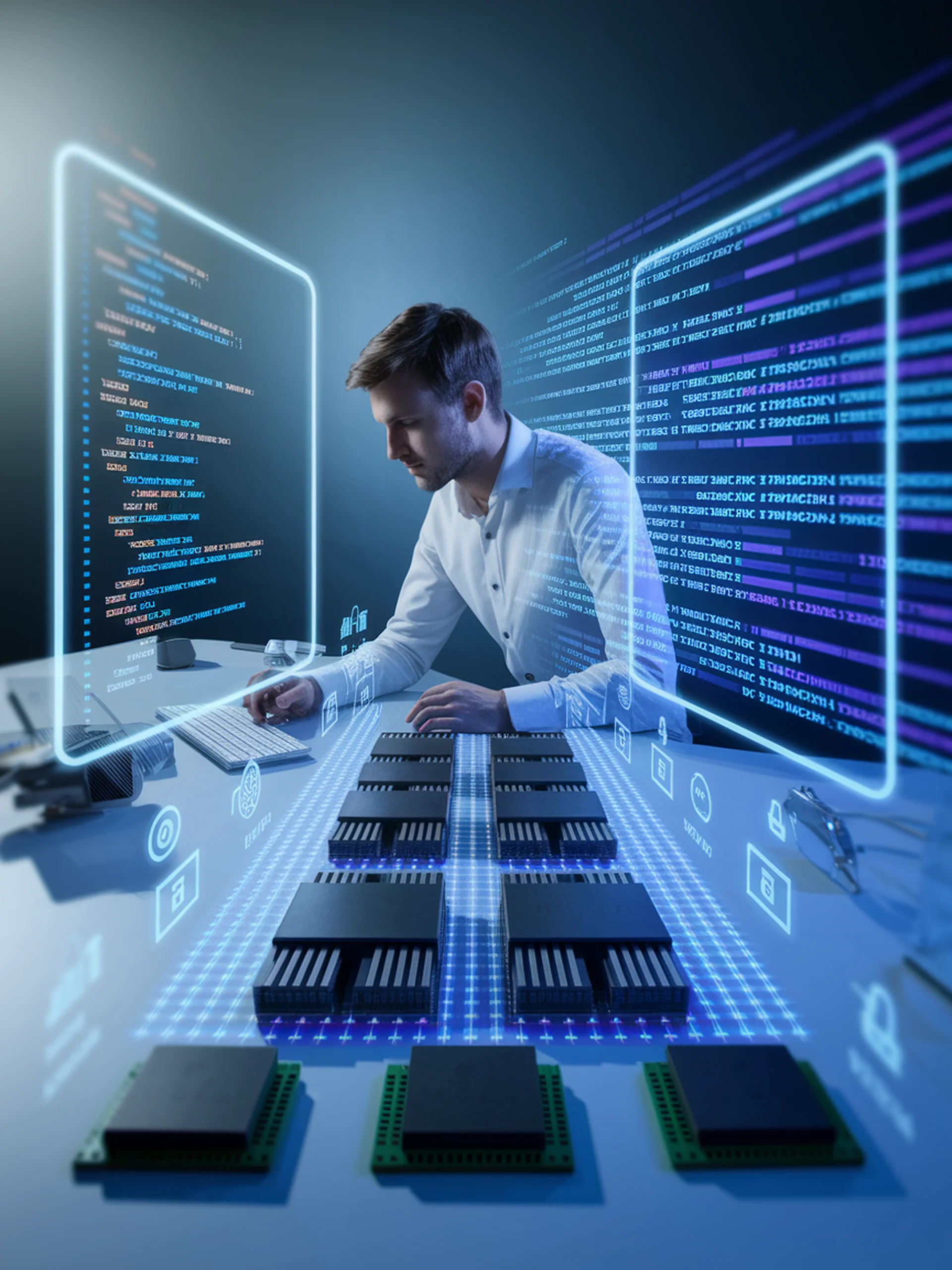
AI-Powered Privacy Code Generation
Bridging the gap between conventional and privacy-preserving programming
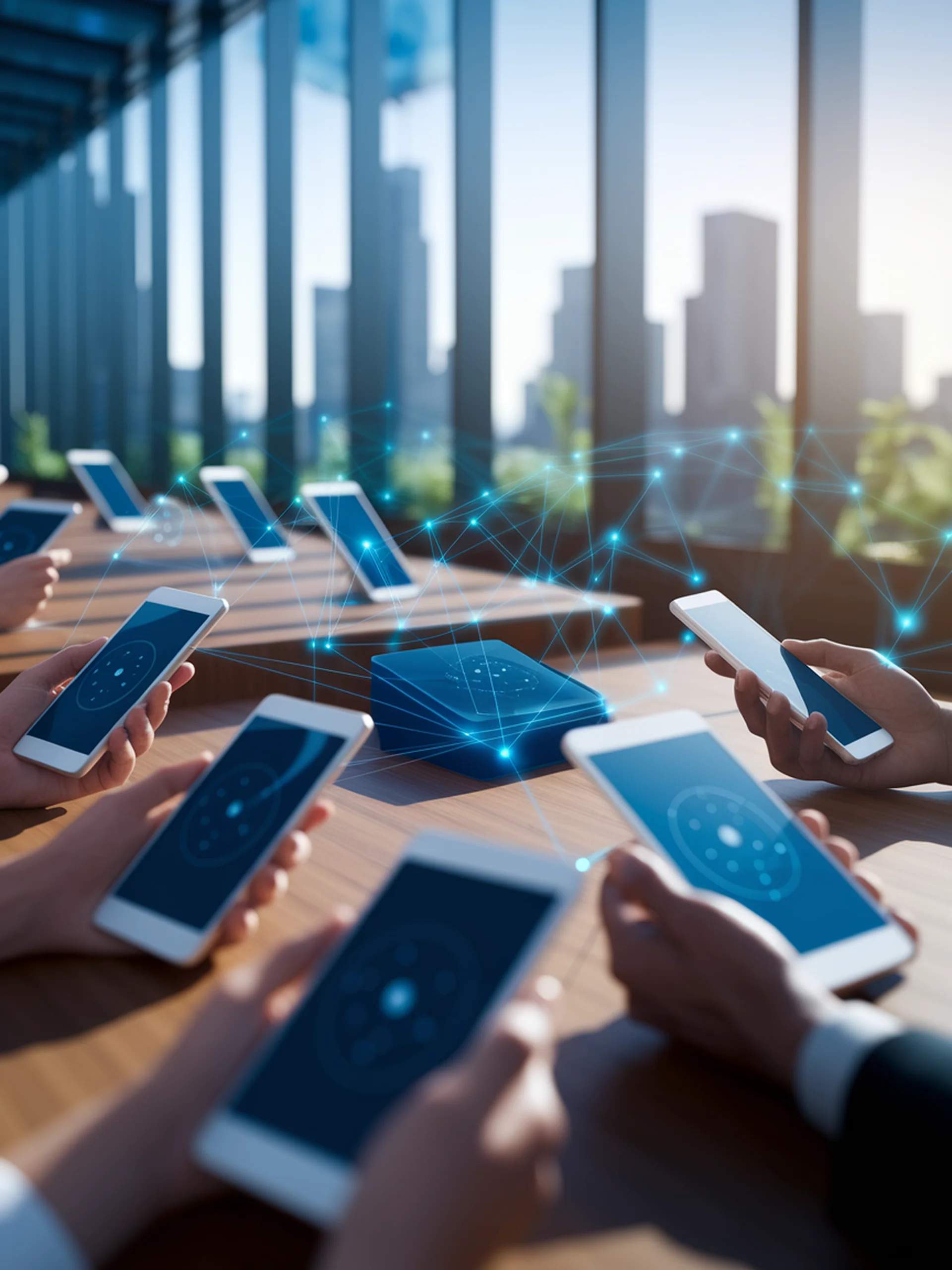
Decentralized Learning at the Edge
Privacy-Preserving Collaborative ML for Mobile Devices
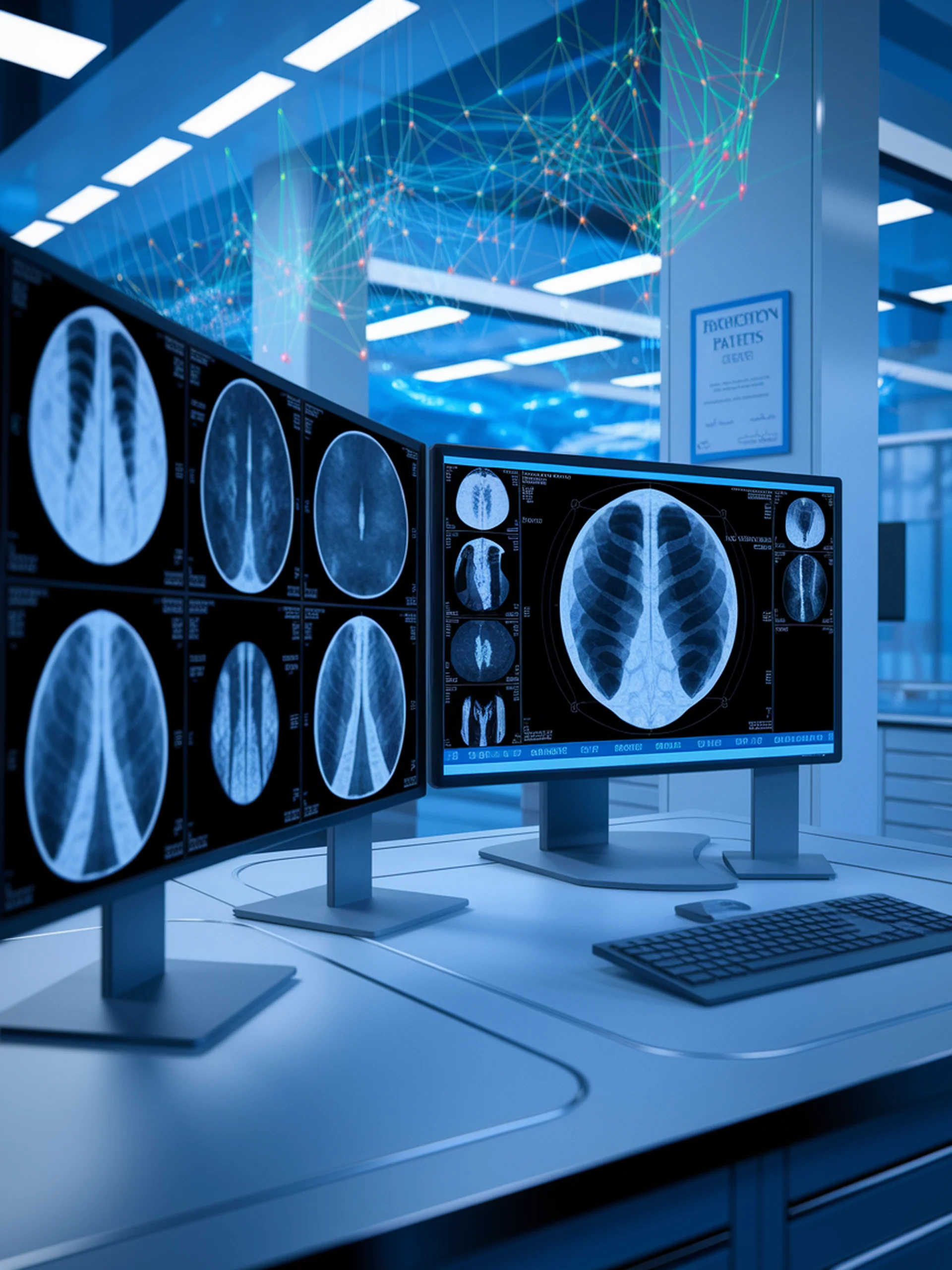
AI for Protected Health Information Detection
Securing Medical Images through Automated PHI Detection
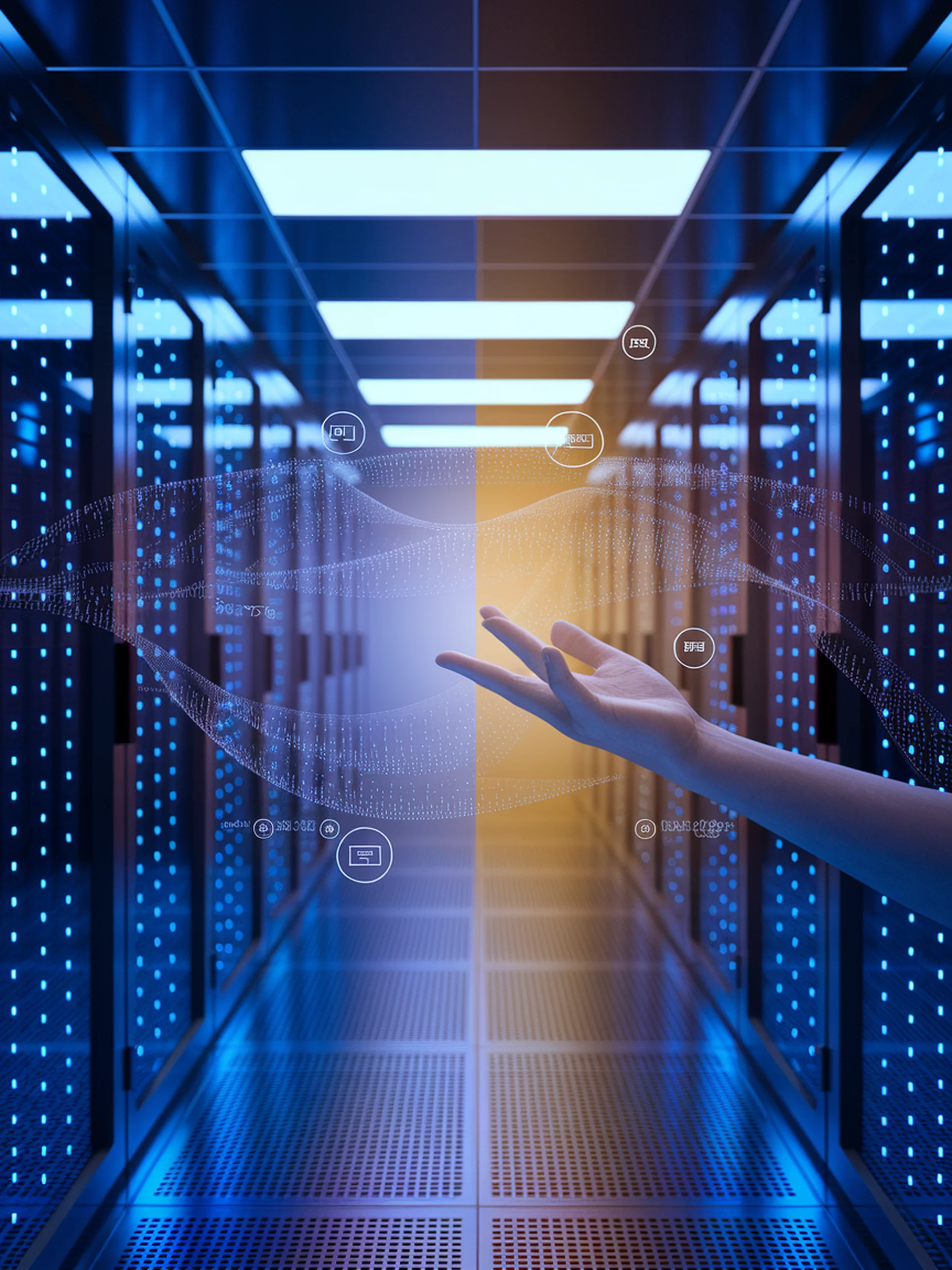
Privacy-First Personalized AI Support
Balancing Personalization and Privacy in Multimodal LLMs
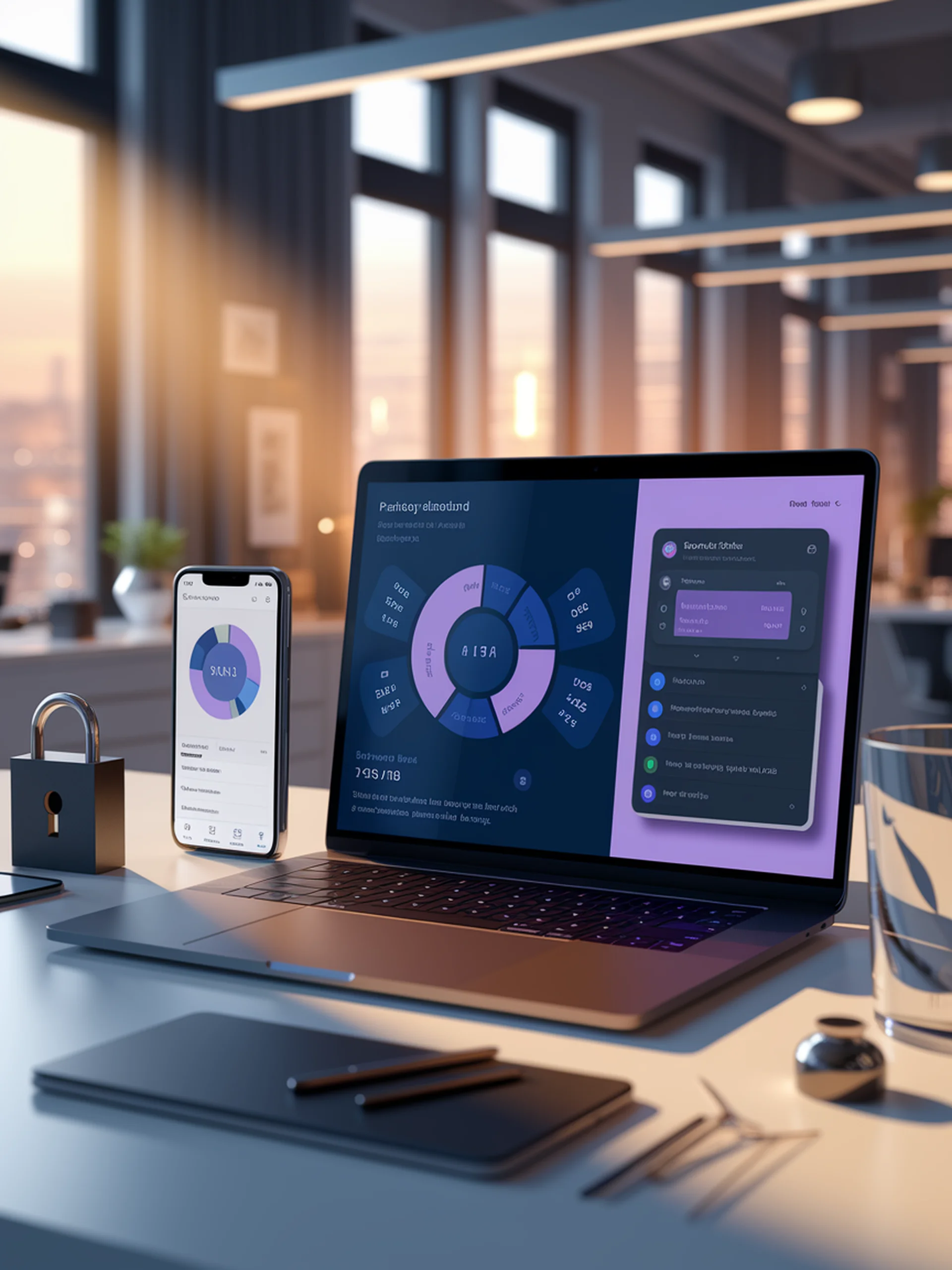
PRISMe: Making Privacy Policies Accessible
AI-powered browser extension for real-time privacy risk assessment
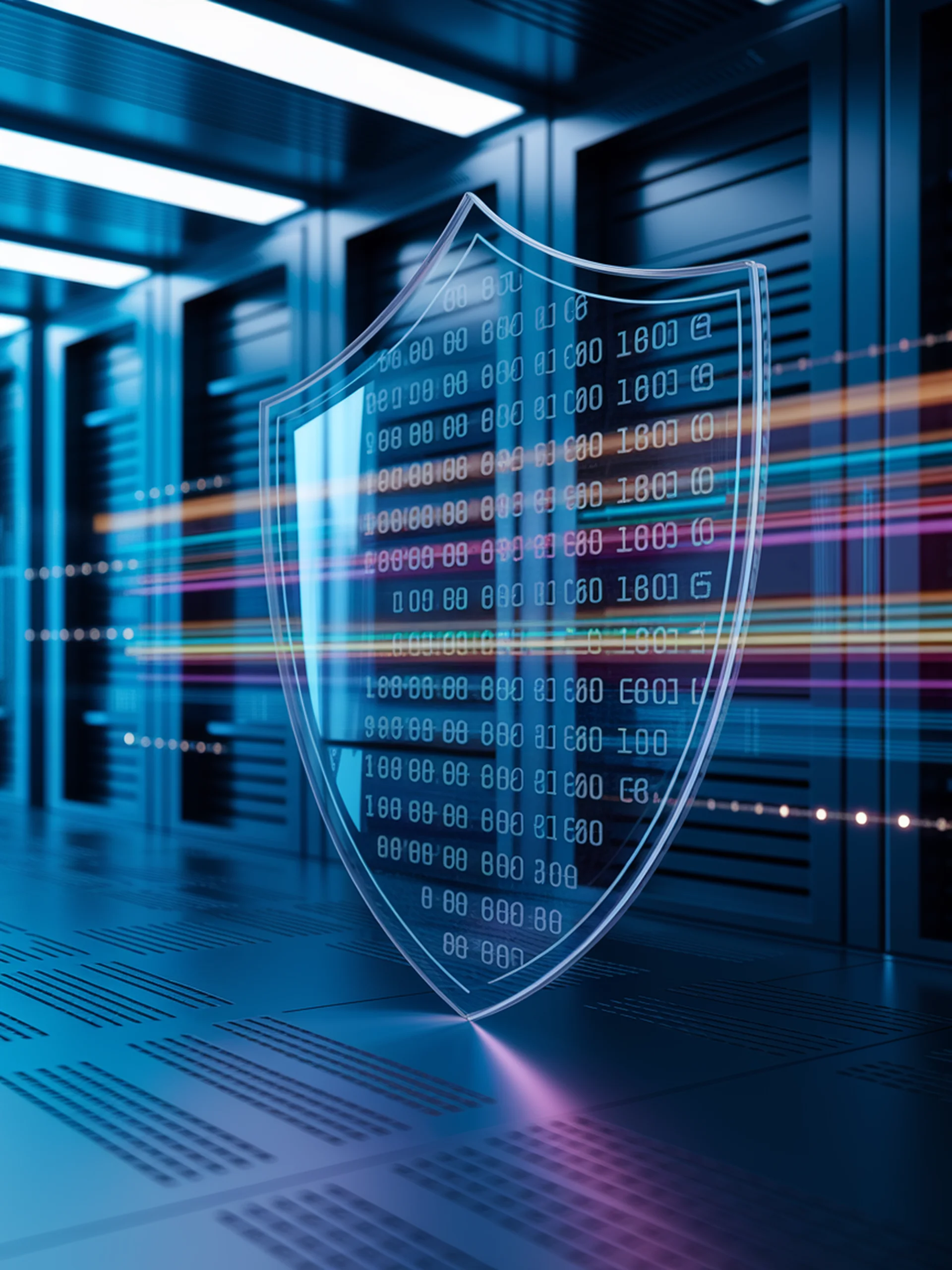
Privacy-Preserving LLM Alignment
Steering language models safely with differential privacy
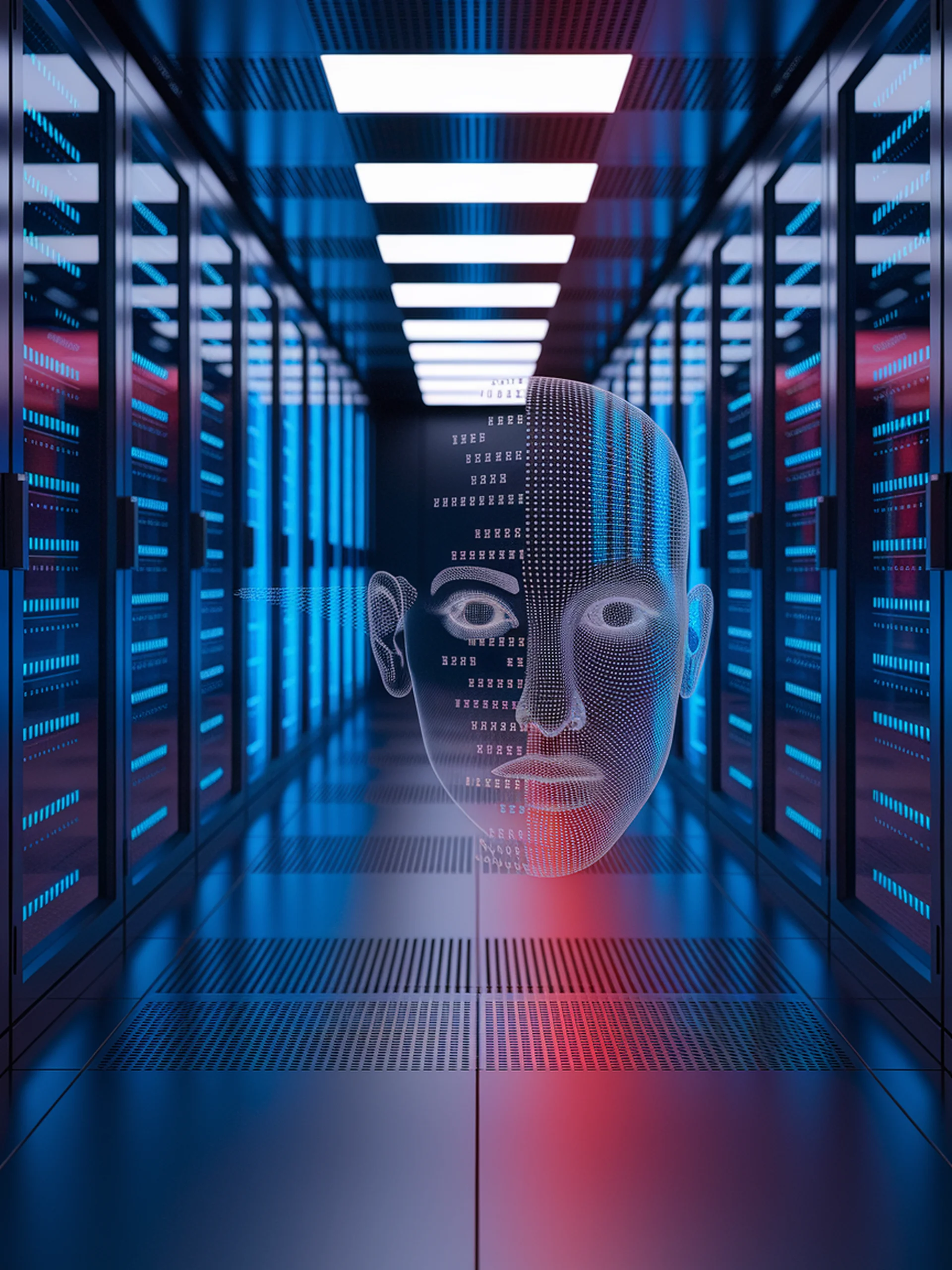
Privacy Vulnerabilities in VLMs
Detecting Data Leakage in Vision-Language Models
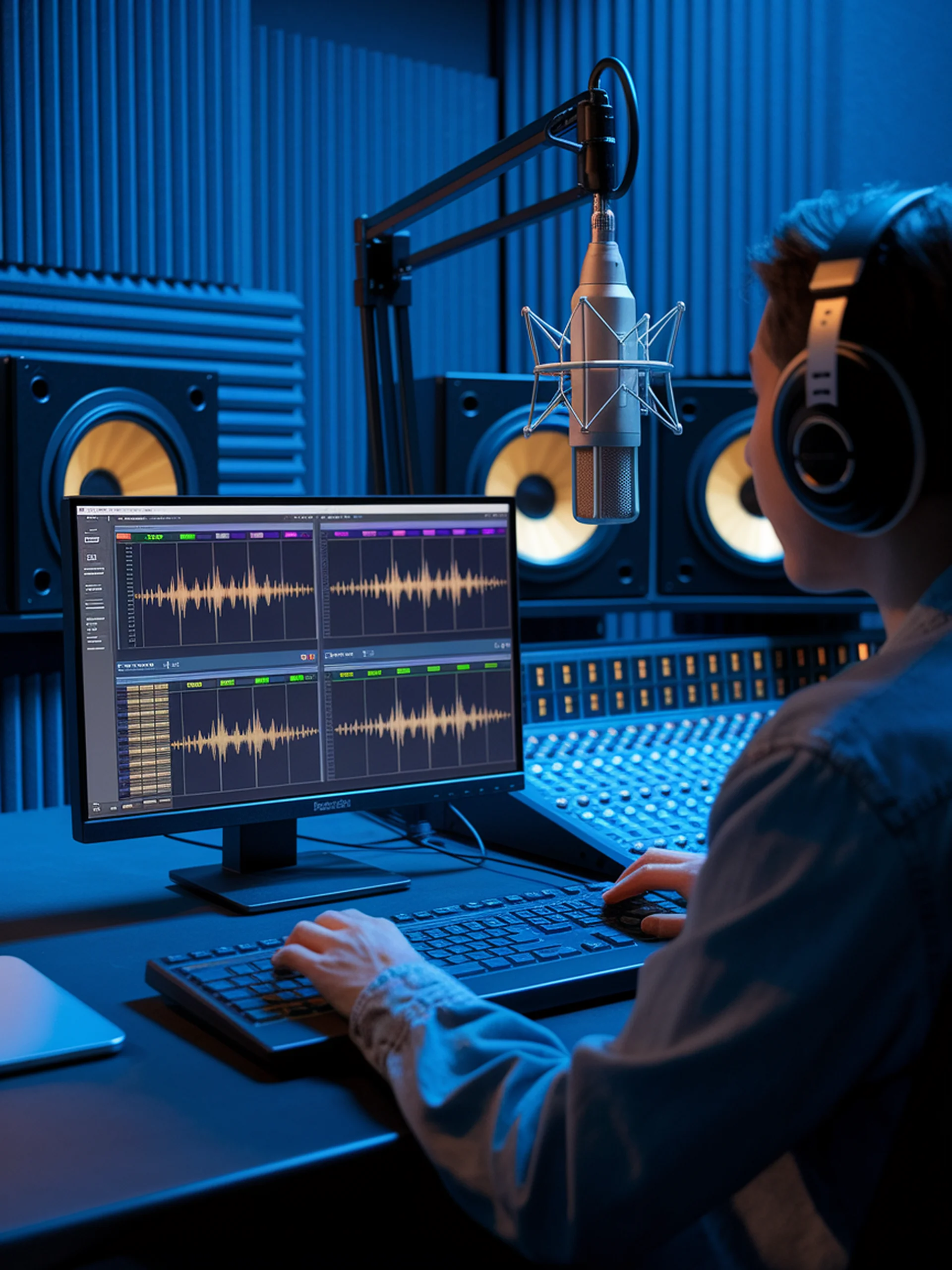
Protecting Emotional Privacy in Voice Data
Audio Editing as User-Friendly Defense Against LLM Inference Attacks
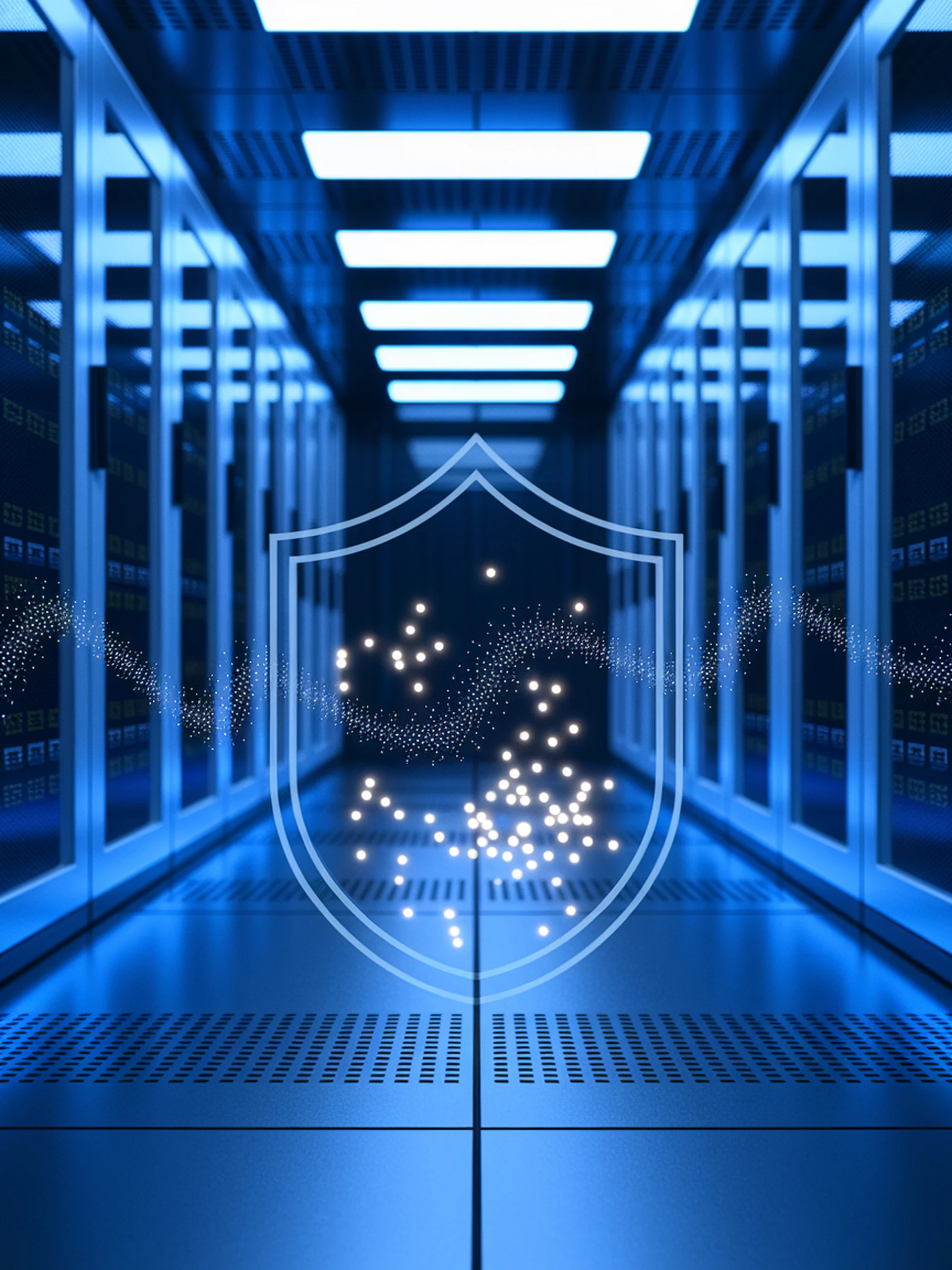
Privacy-Preserving LLM Scaling
New scaling laws for differentially private language models
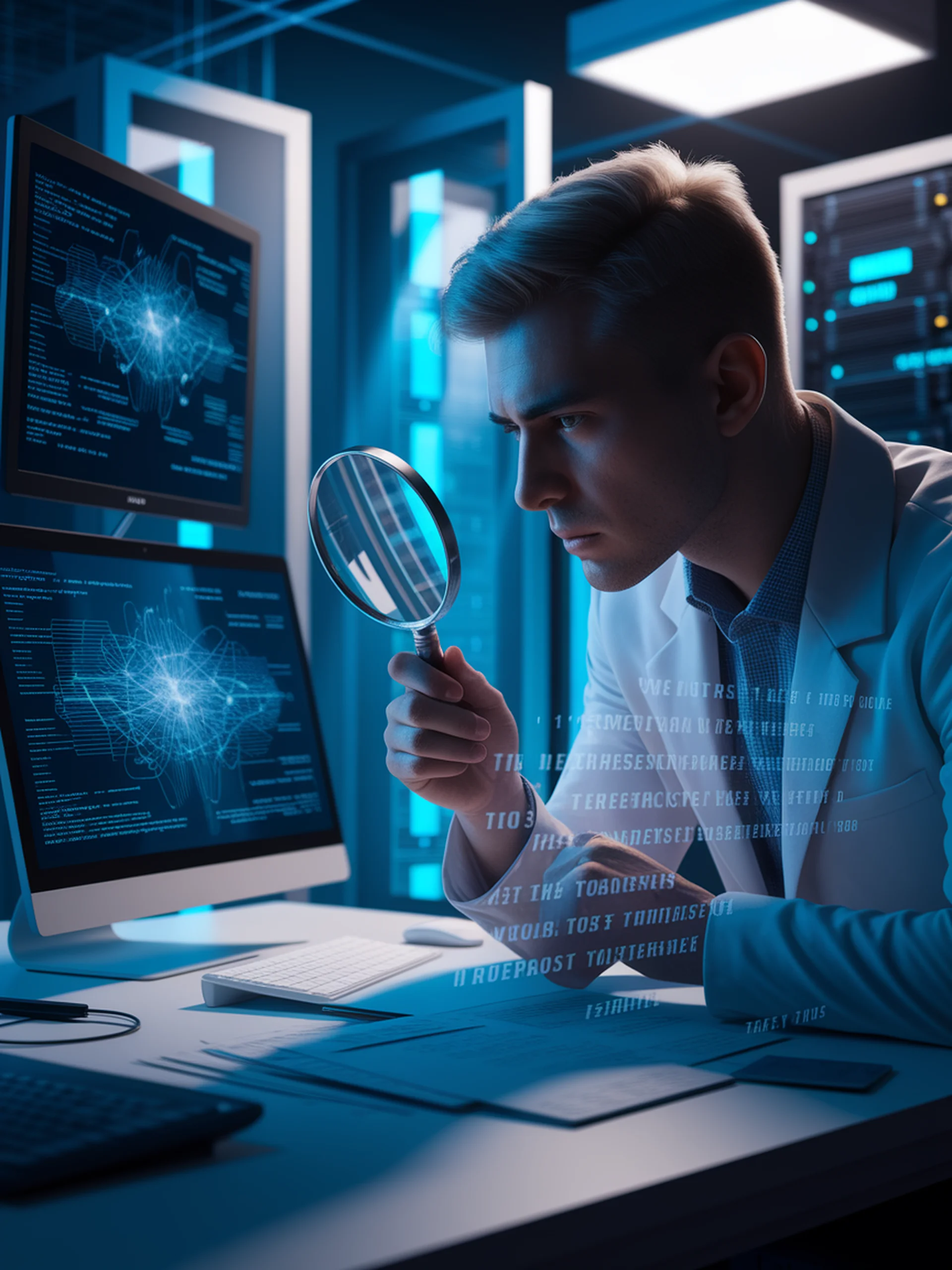
Beyond the Hype: Contextual Integrity in LLMs
Examining the superficial application of privacy frameworks in language models
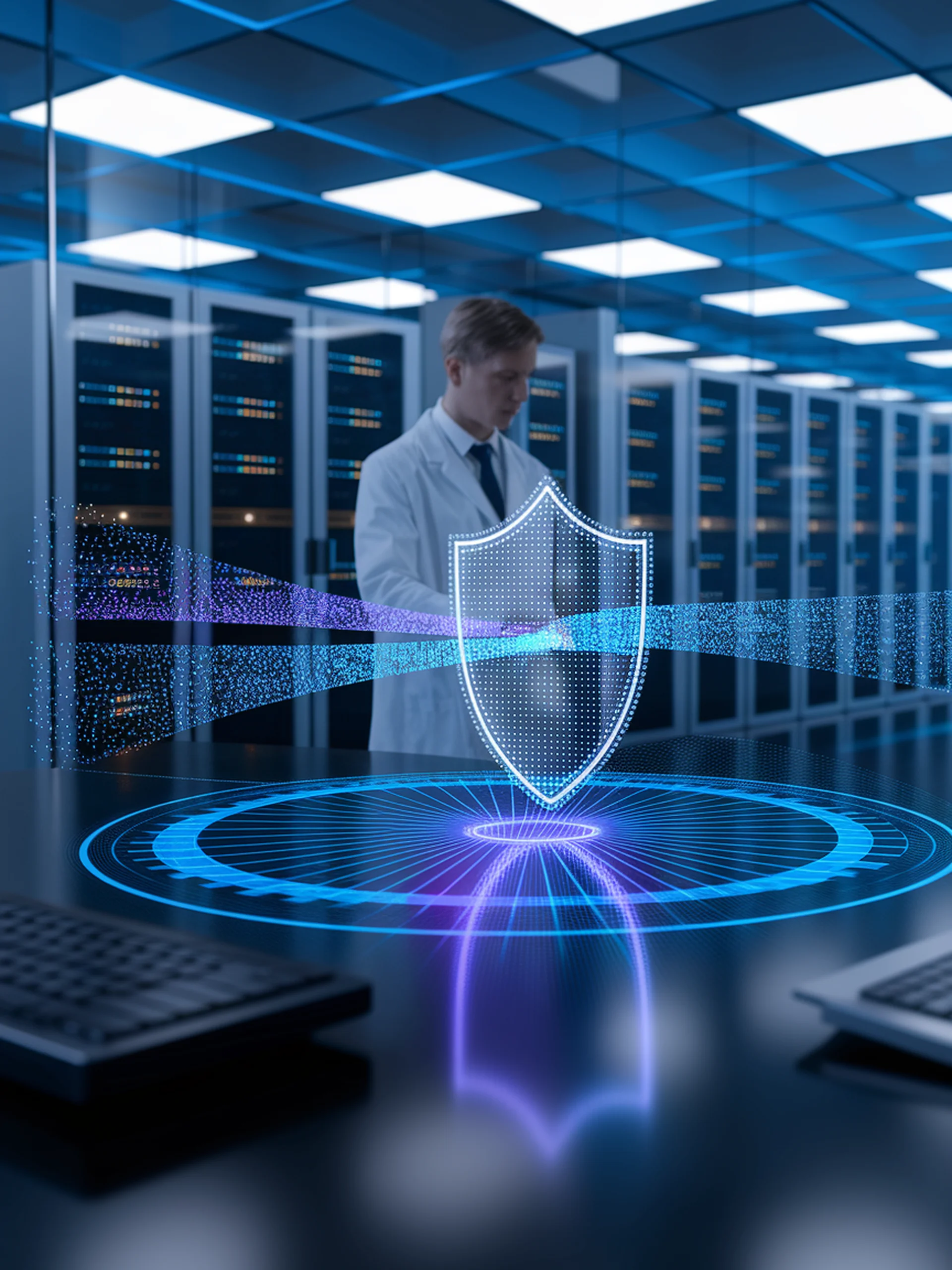
Privacy-Preserving Synthetic Data
Generating high-quality data while protecting privacy through multi-model fusion
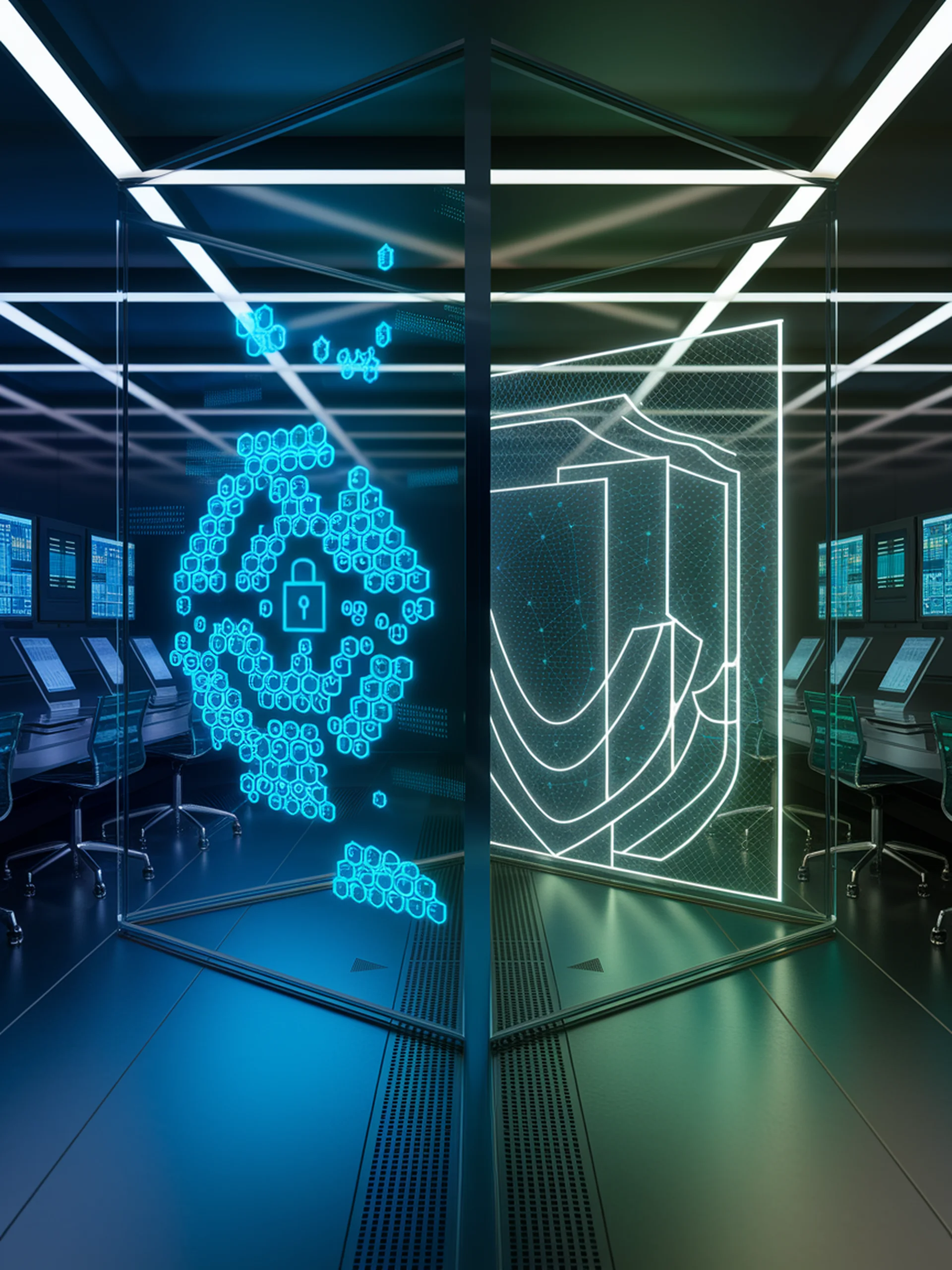
Securing LLMs on Two Fronts
A novel approach combining privacy protection and adversarial robustness
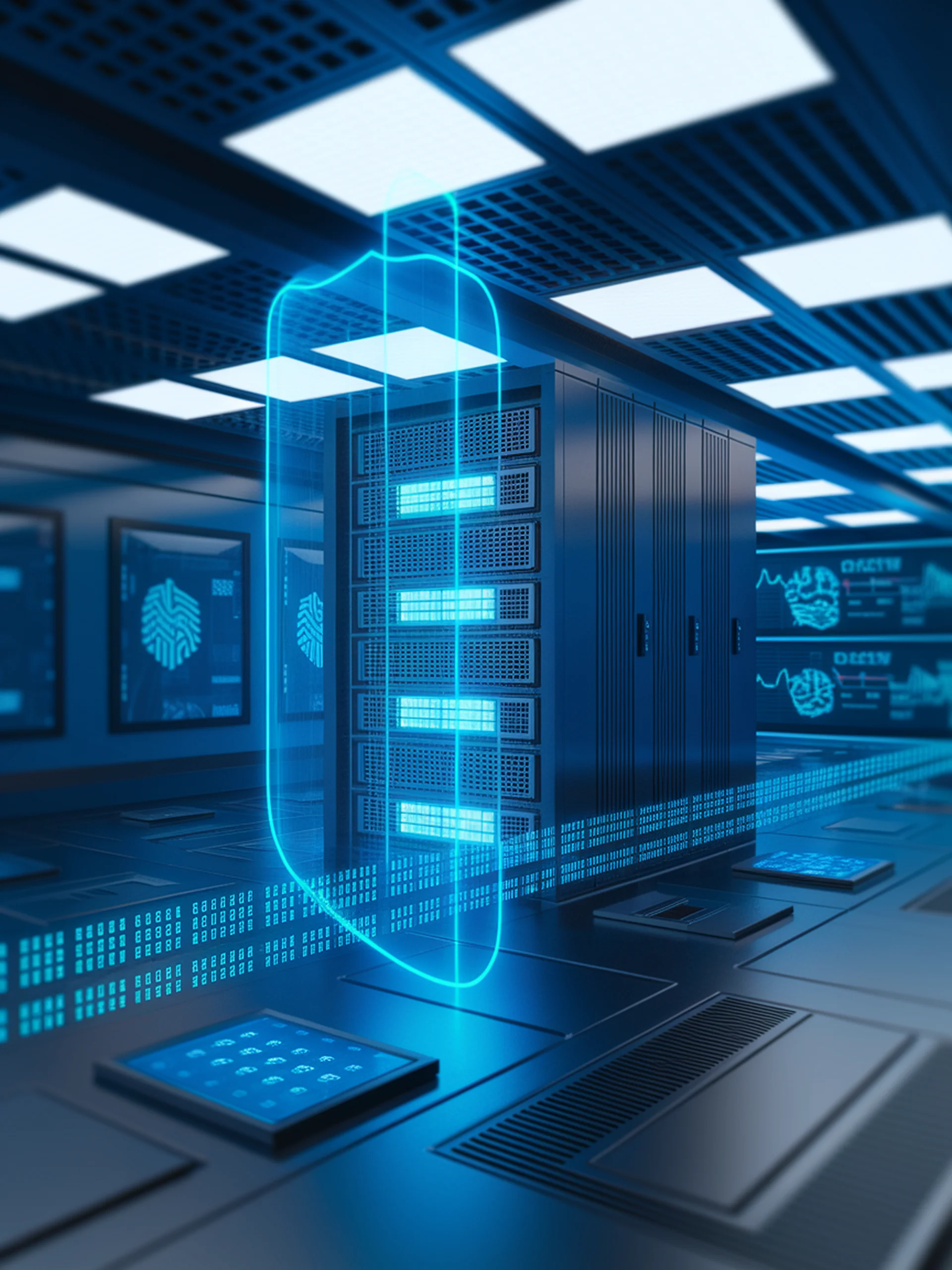
Securing AI: The Encrypted Inference Revolution
How Equivariant Encryption enables privacy-preserving model deployment
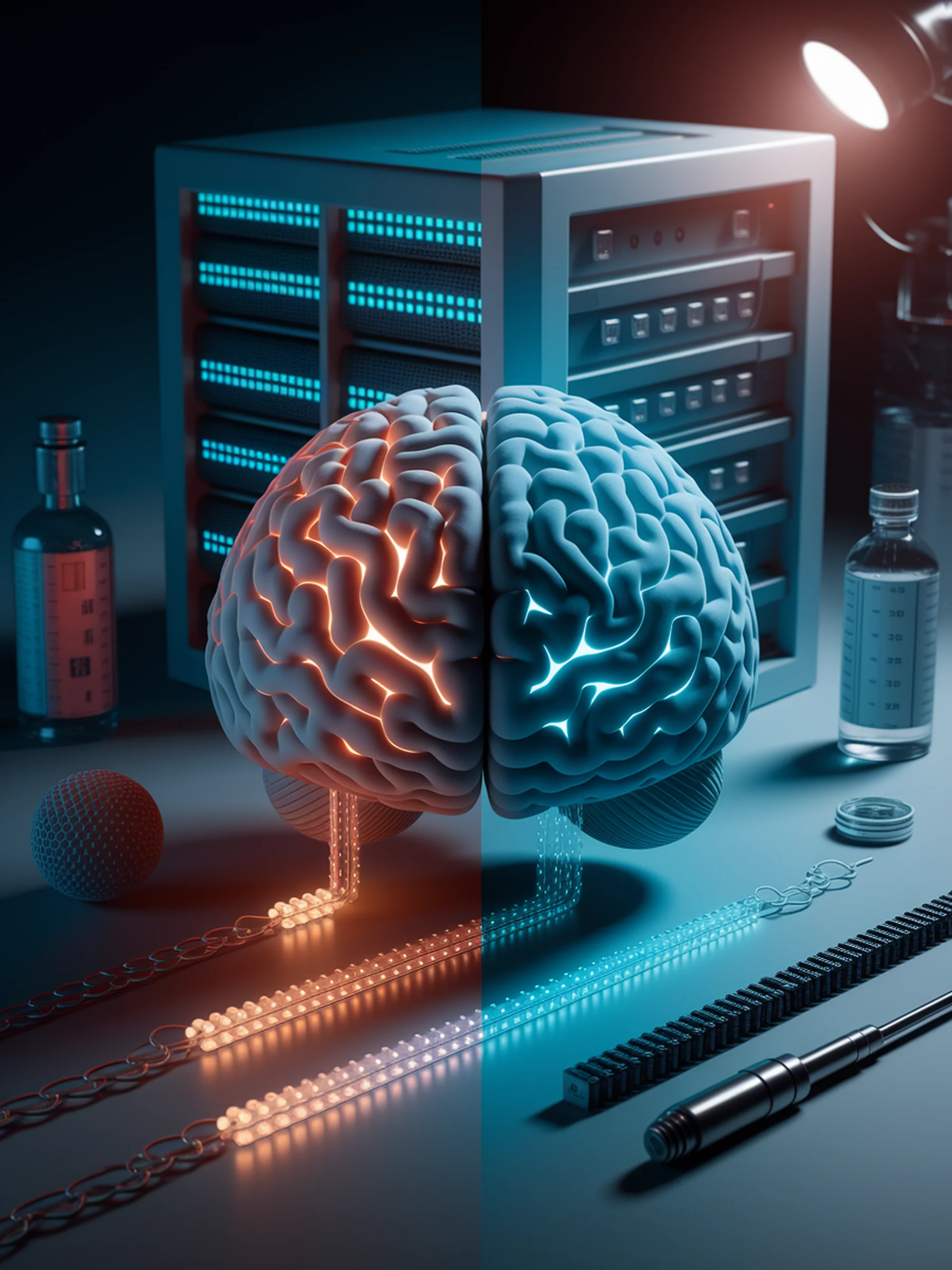
The Hidden Memory Problem in LLMs
Understanding skewed memorization patterns and their security implications
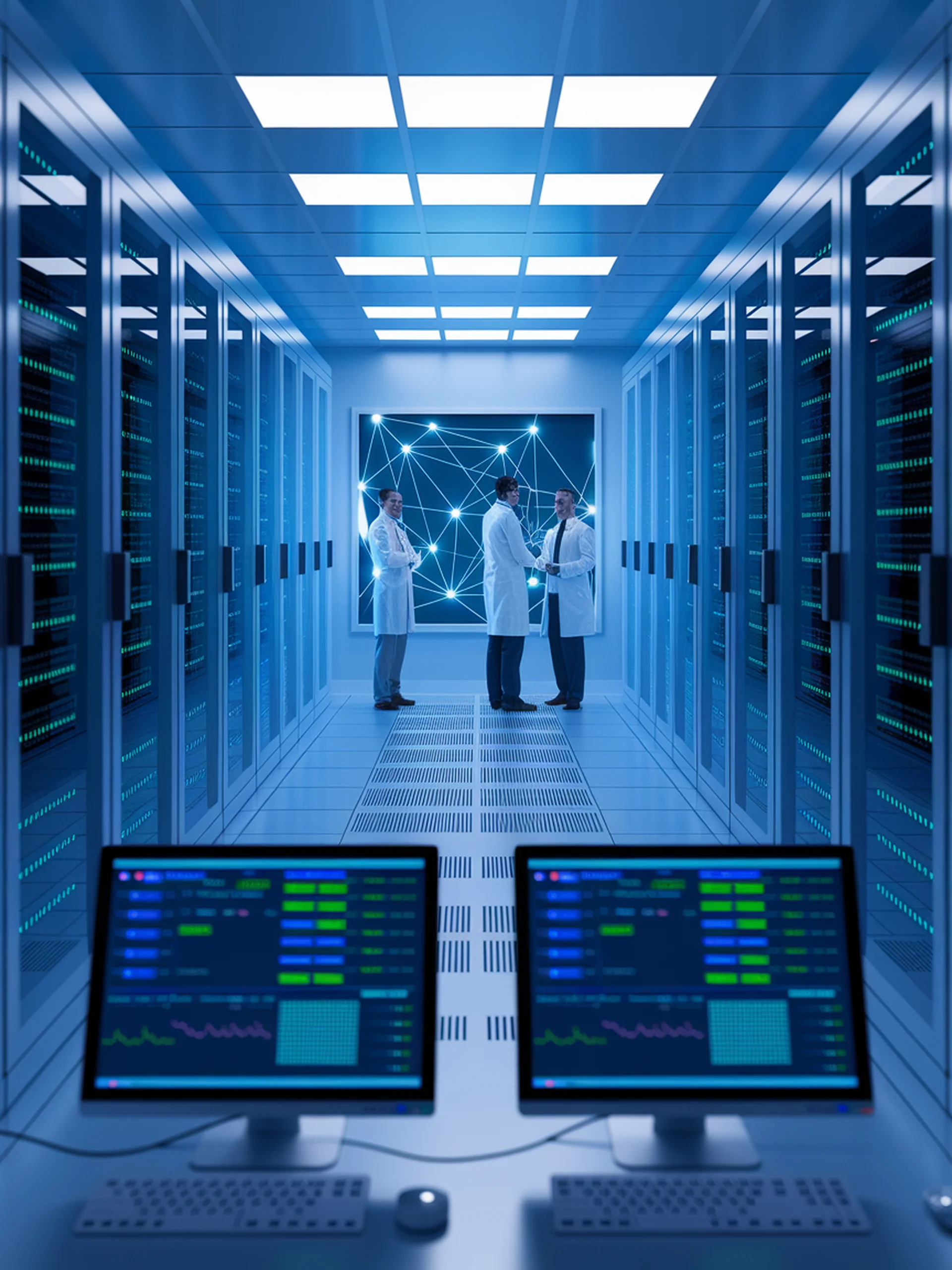
Protecting Patient Data in Collaborative AI
Using LoRA to reduce unintended data memorization in federated learning
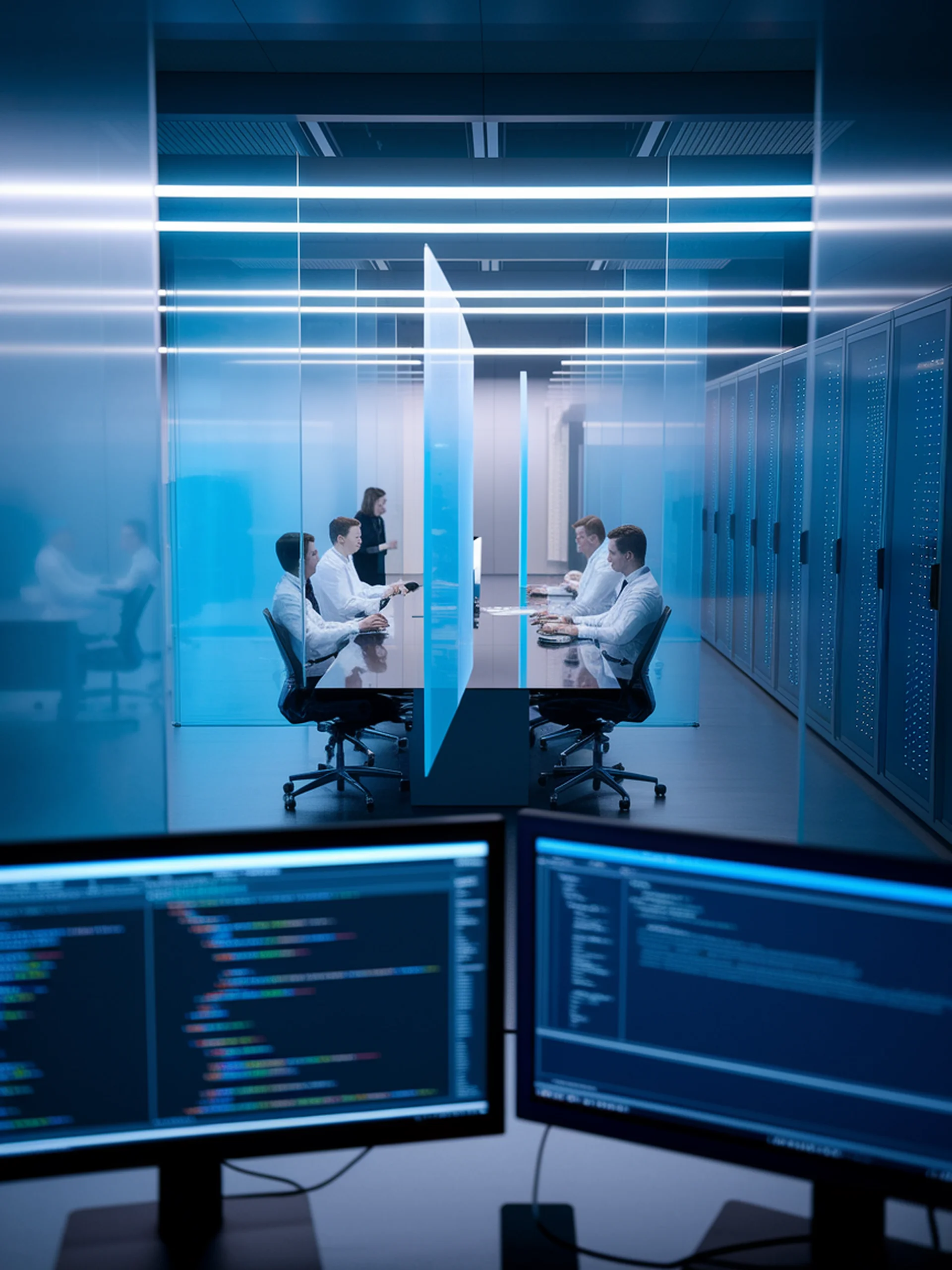
Securing AI Code Generators
Protecting Sensitive Data through Machine Unlearning
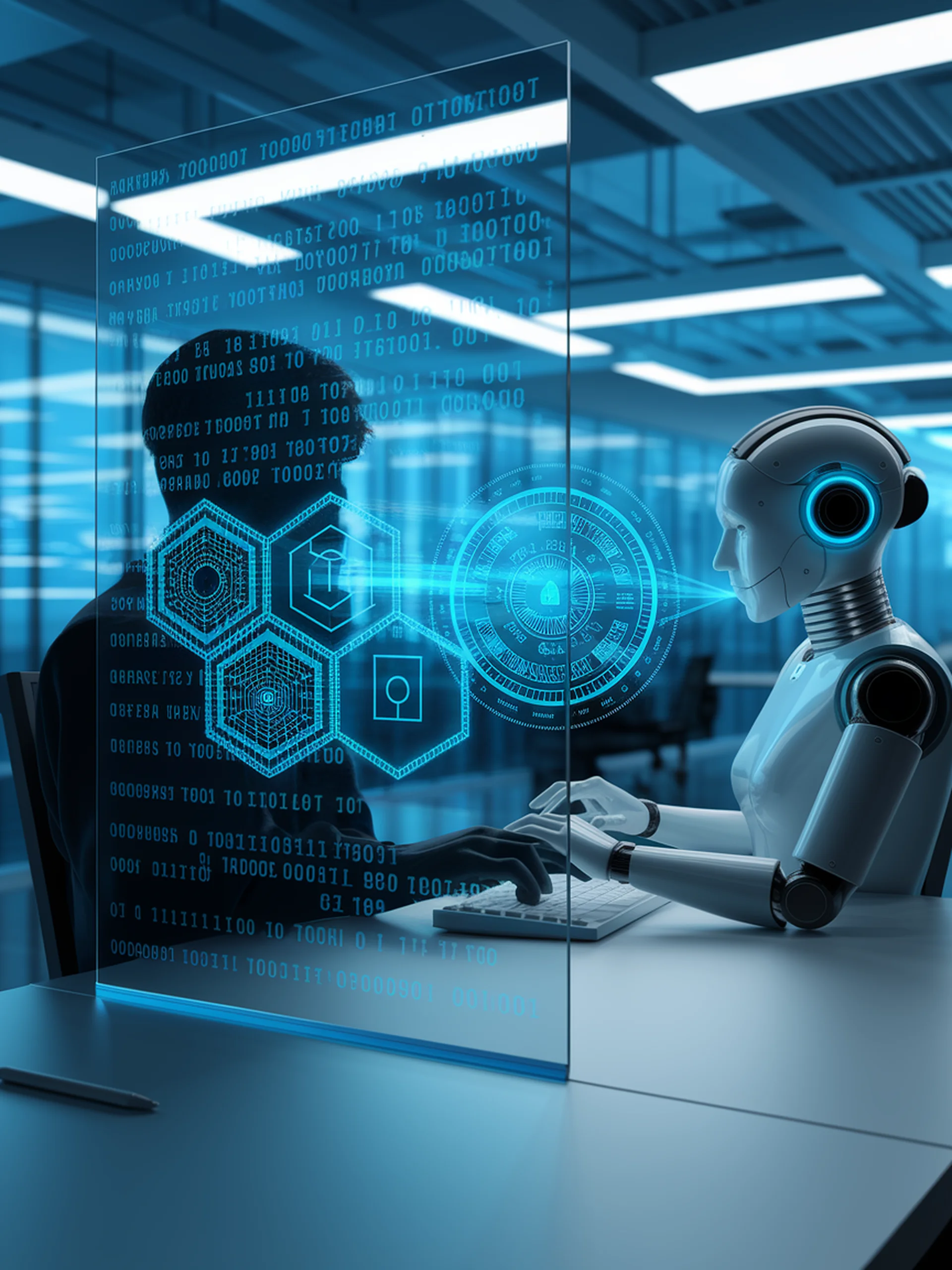
Privacy-First AI Advisory Systems
Combining Zero-Knowledge Proofs with LLMs for Secure Personalization
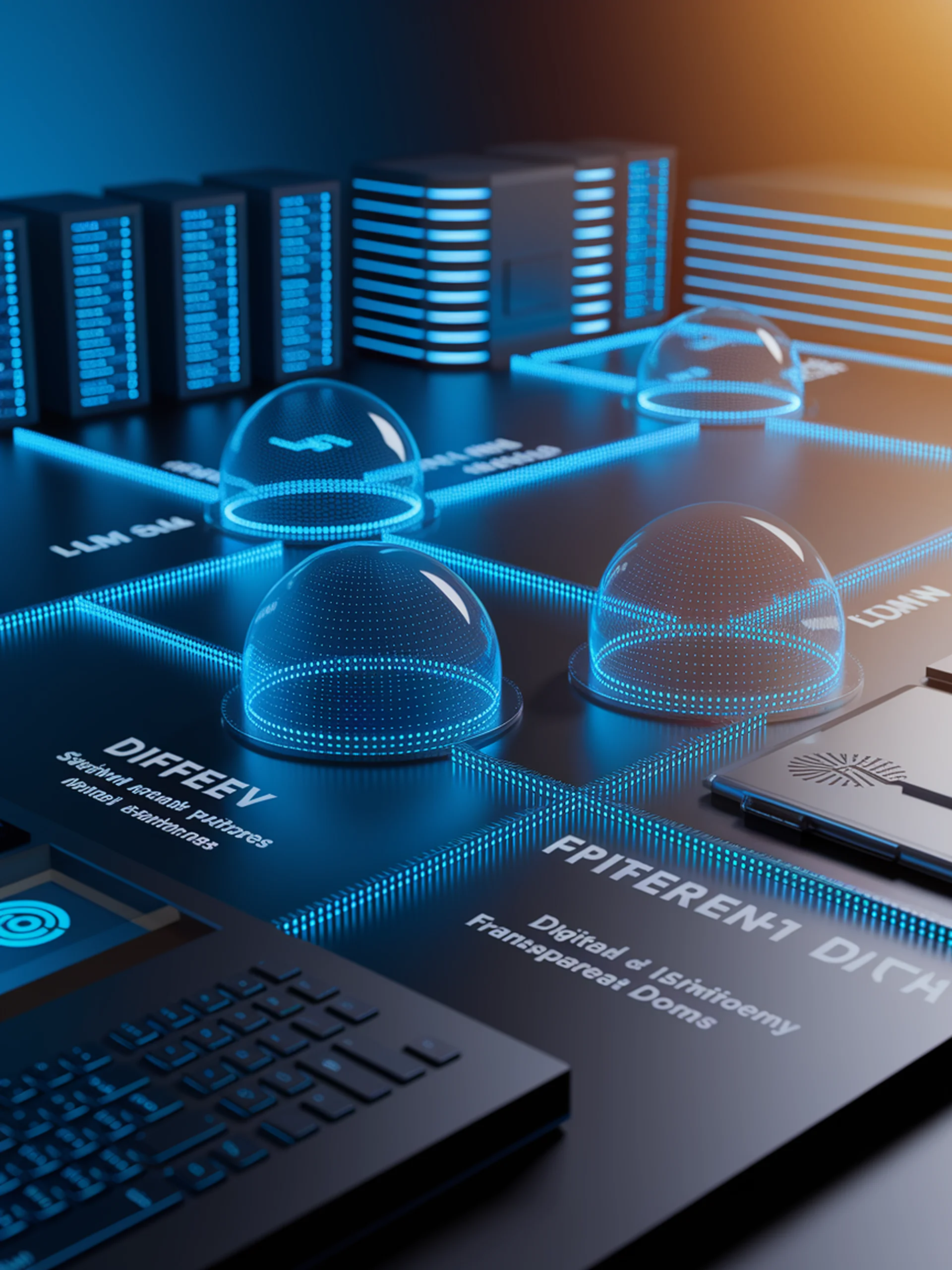
Securing Federated Learning for LLMs
Privacy-Preserving Framework Balances Security and Performance
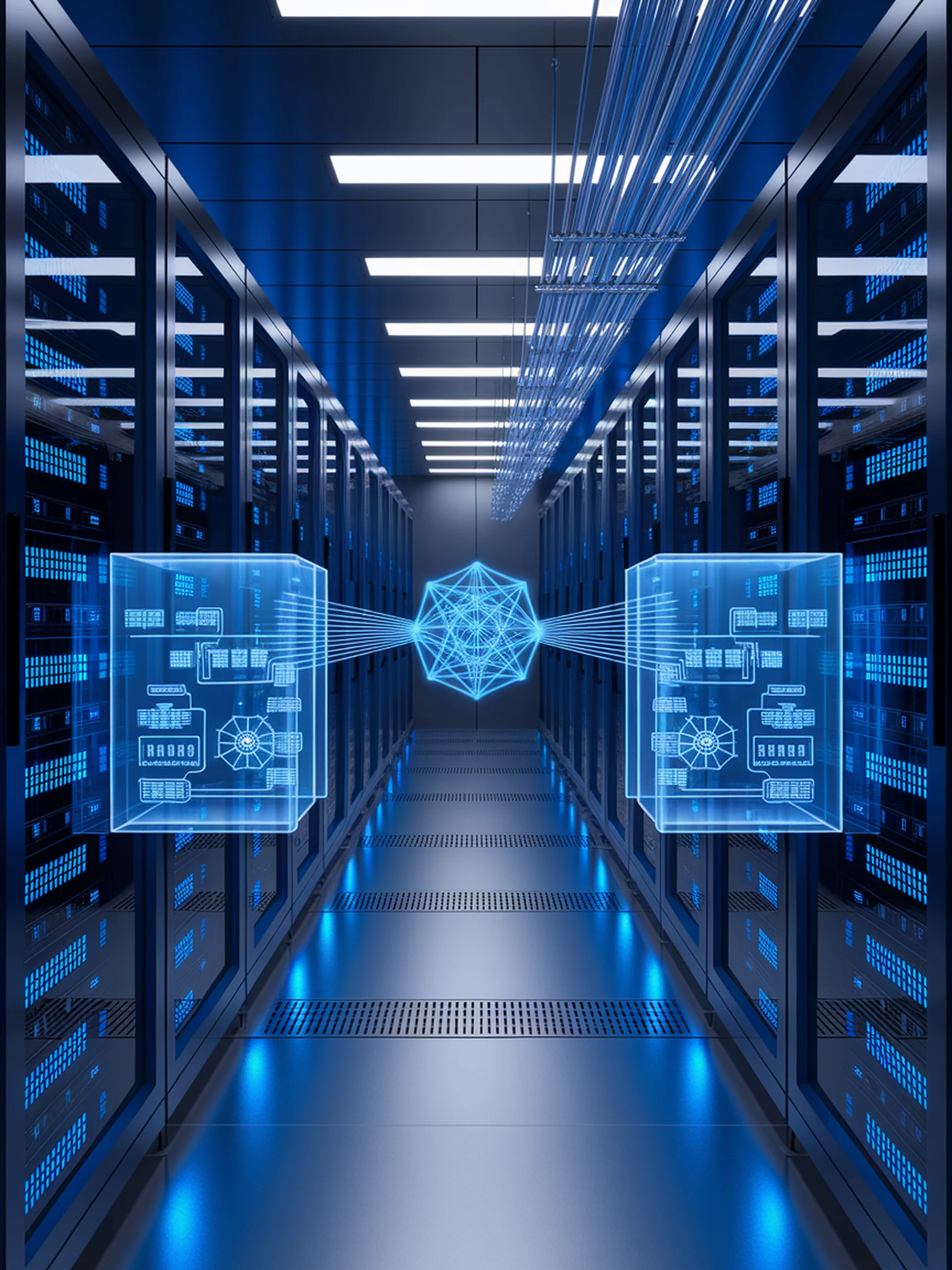
Democratizing AI Through Open-Source LLM Training
A scalable framework for training large language models on GPU supercomputers
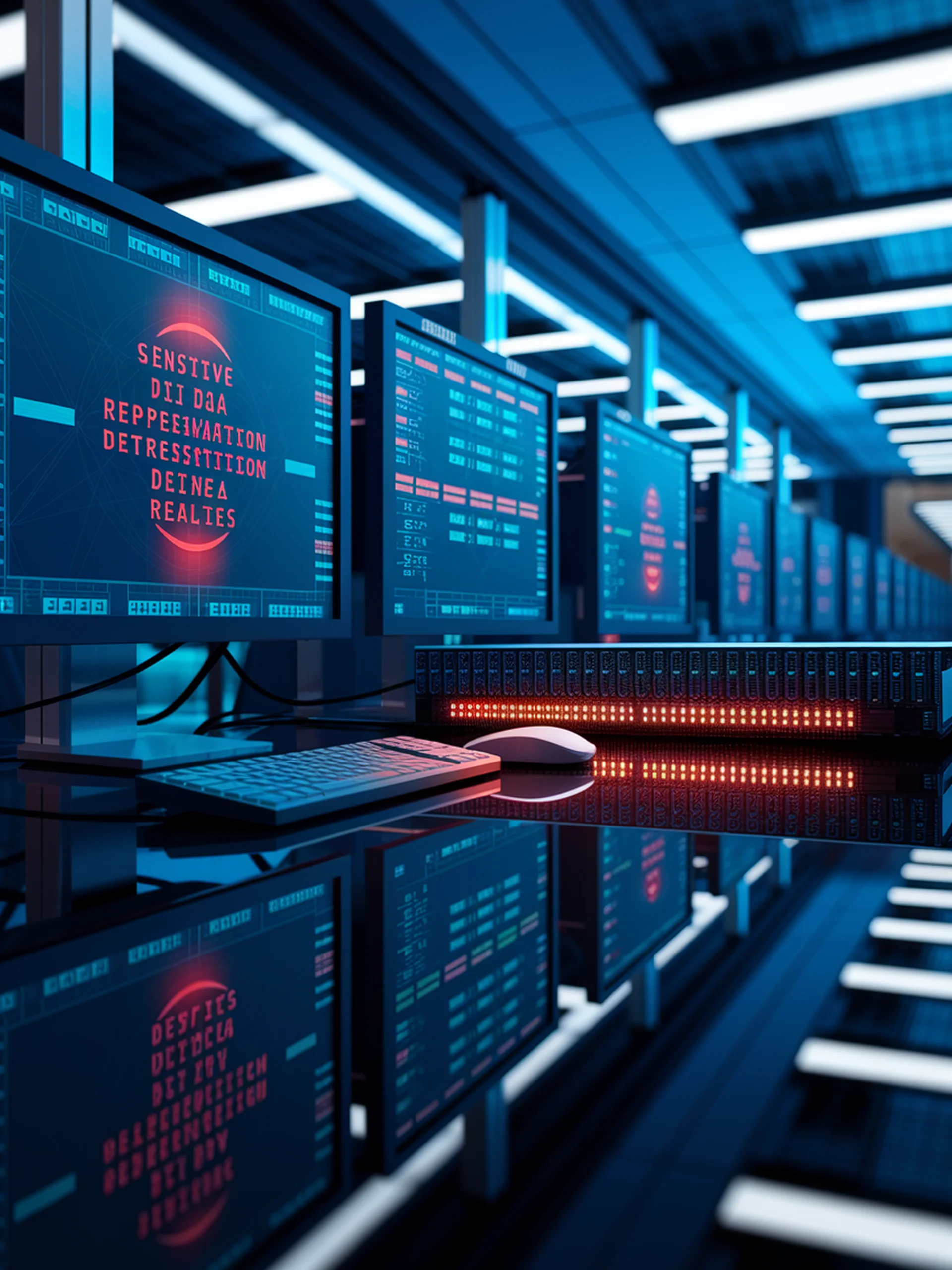
Smarter PII Detection in Network Traffic
Fine-tuning embeddings with triplet loss for enhanced security
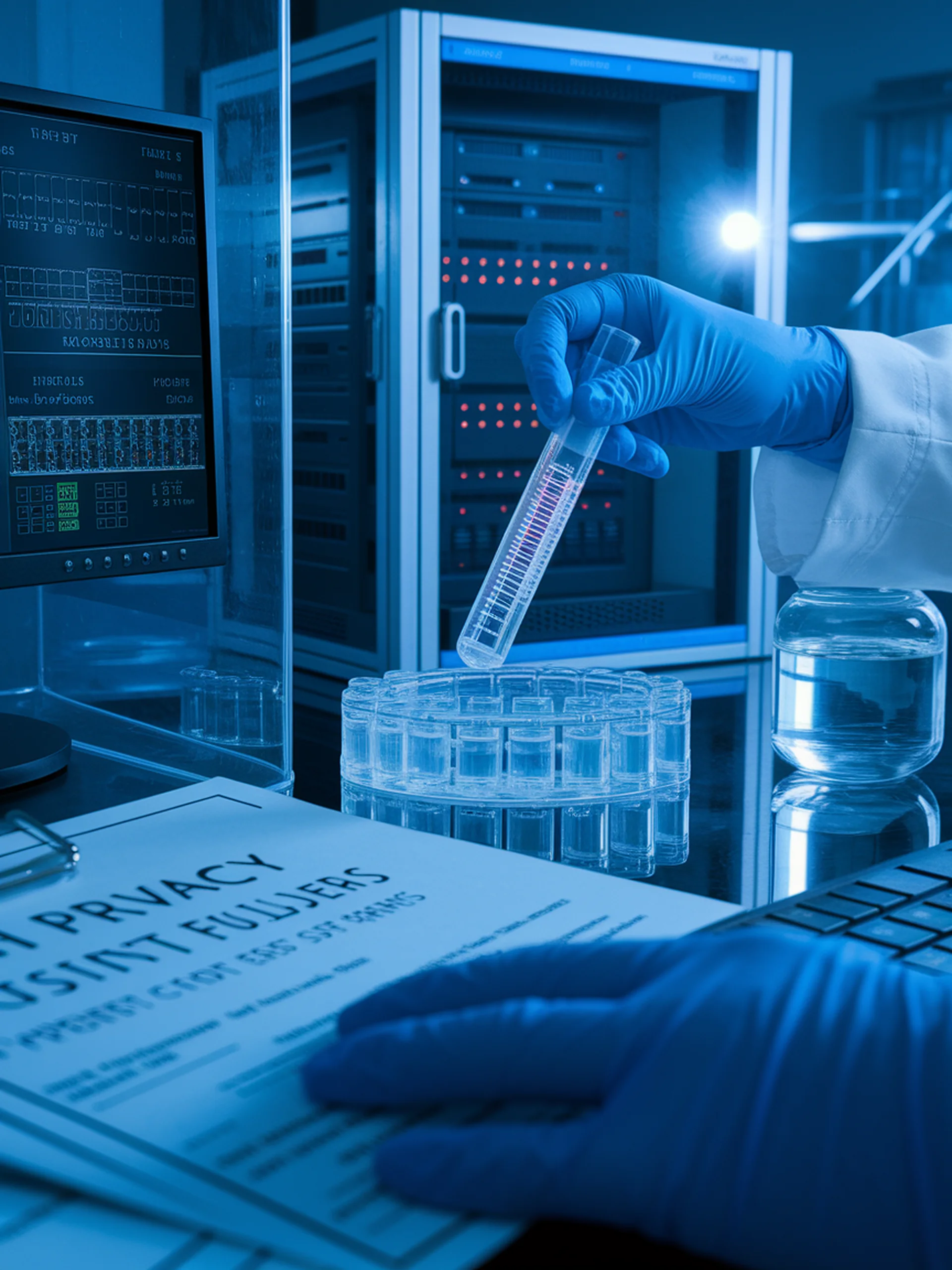
Genetic Data: Crisis and Solutions
Policy frameworks to protect privacy and prevent discrimination
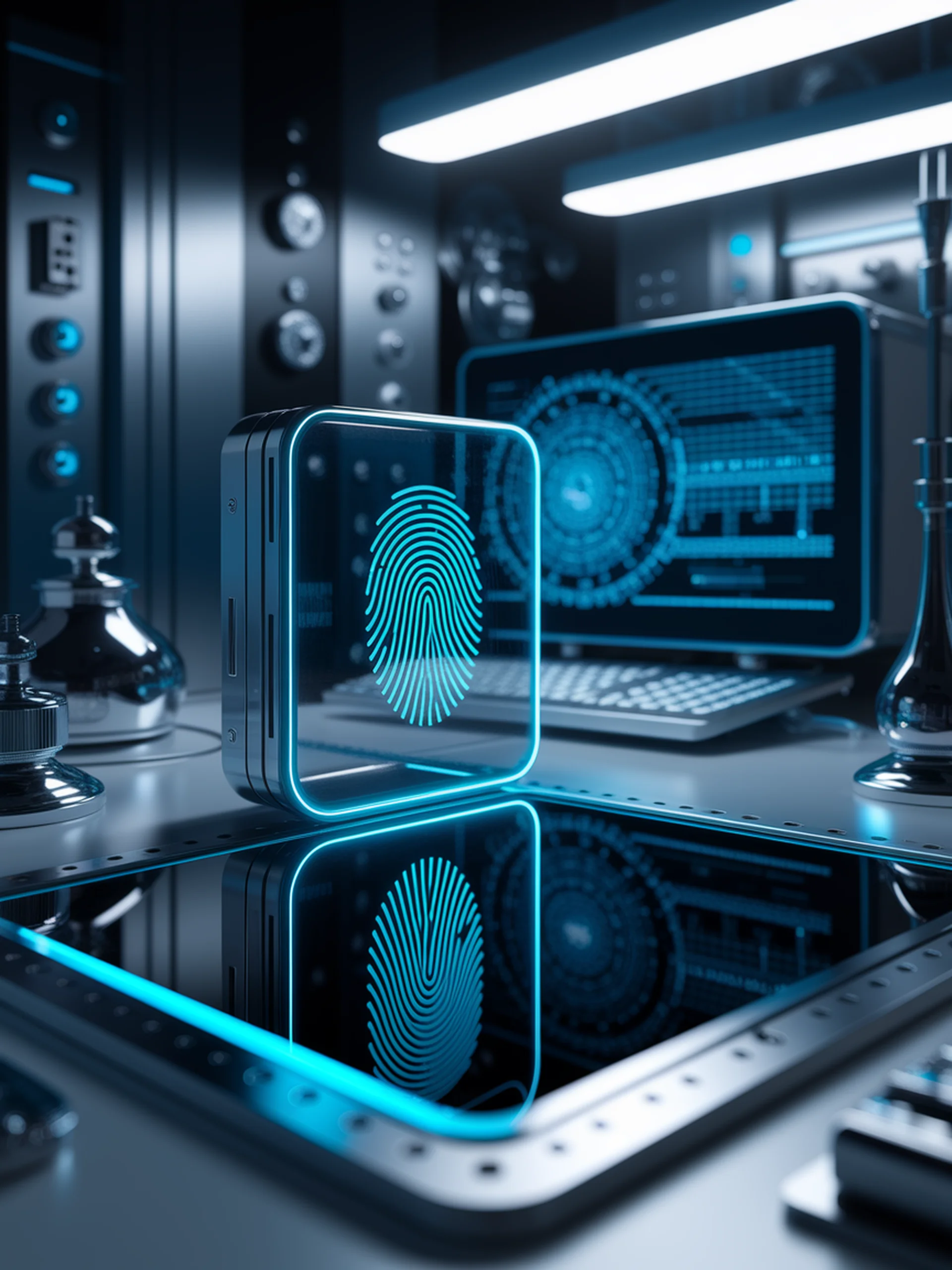
Prompt Theft Detection
Protecting Proprietary System Prompts from Unauthorized Use
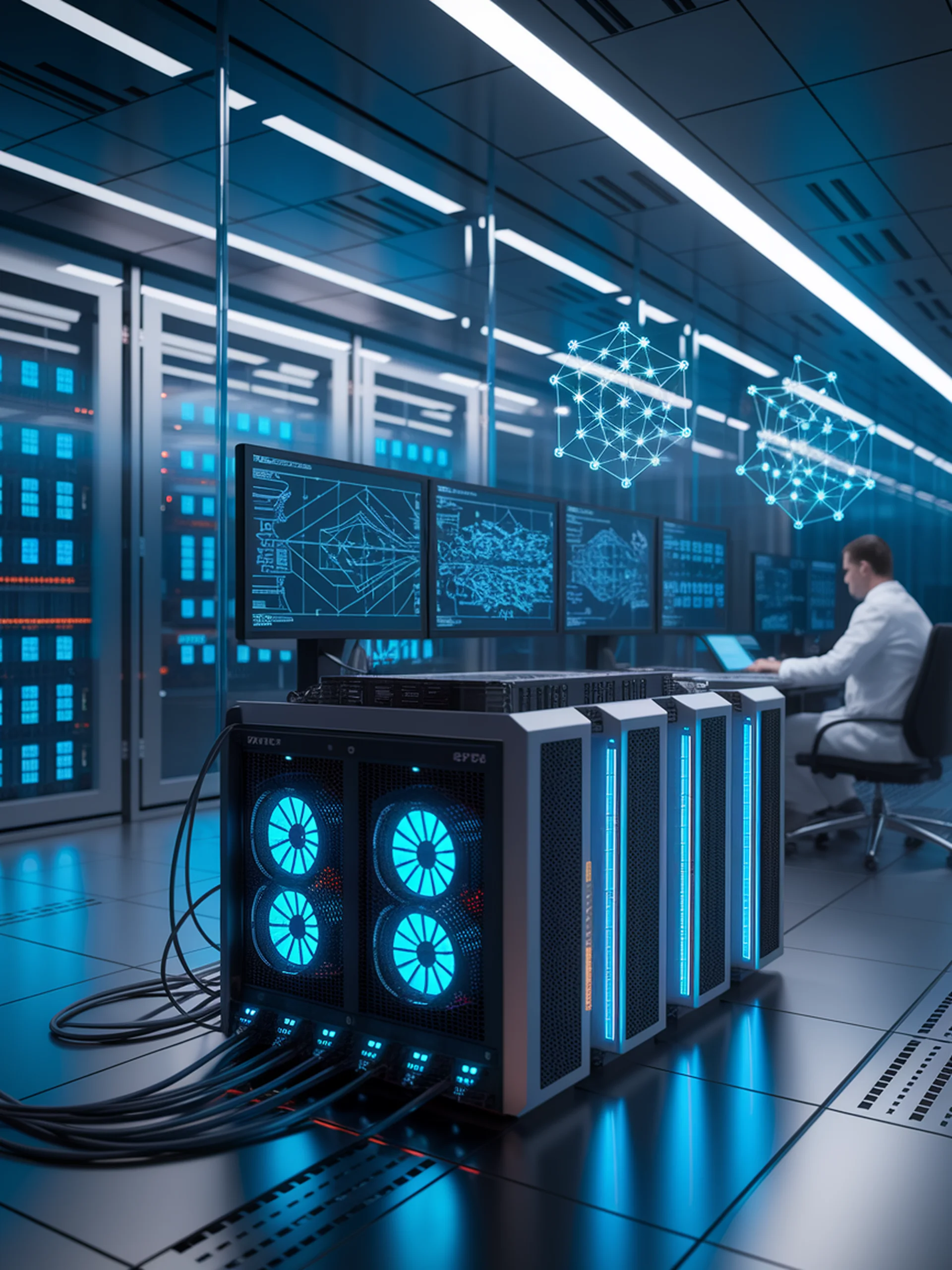
Accelerating Homomorphic Encryption with AI
GPU-powered algorithms and LLM coding for faster privacy-preserving computation
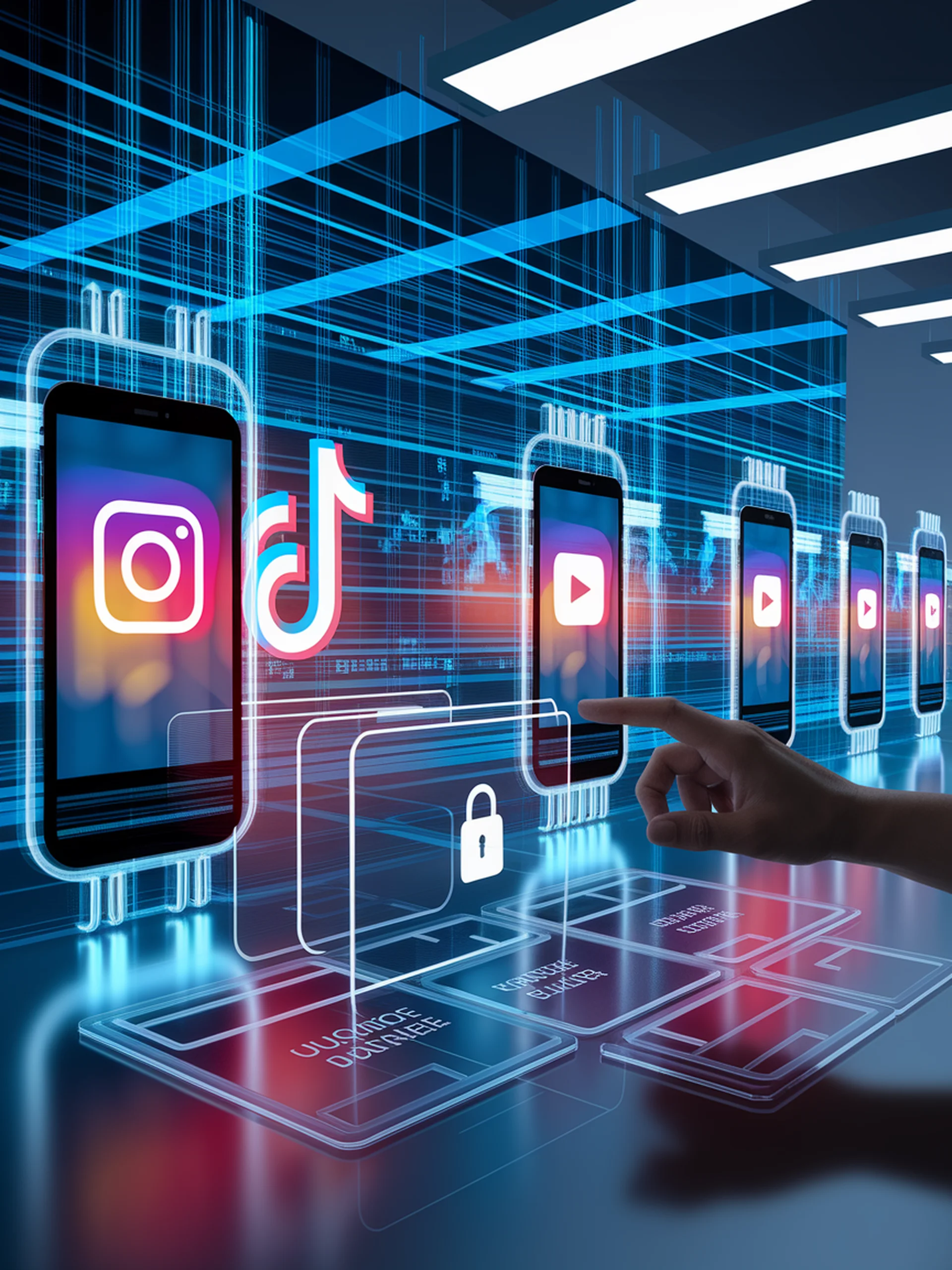
Social Media's GDPR Compliance Gap
Evaluating how Instagram, TikTok, and YouTube fail to provide complete data access
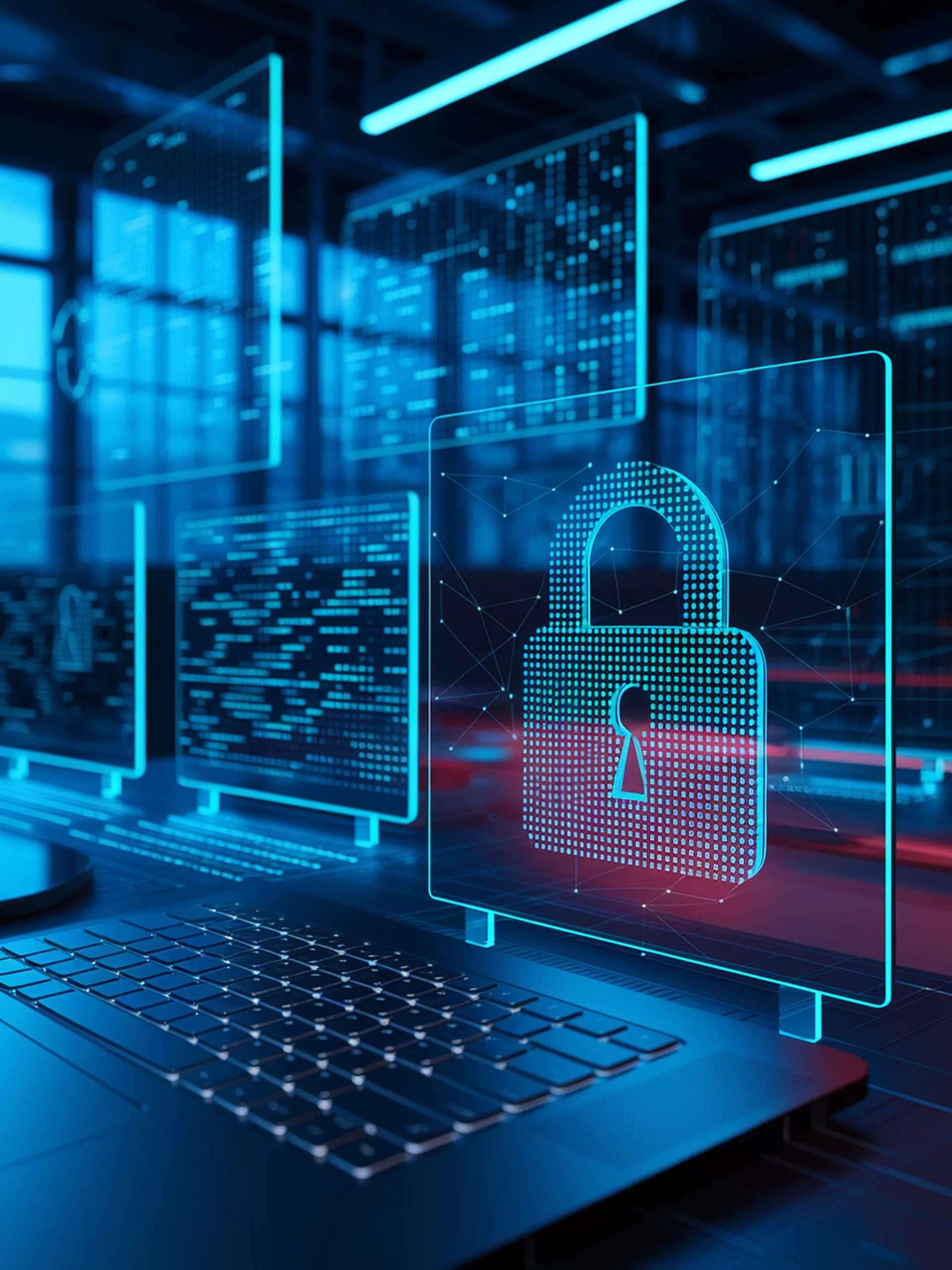
Cracking the Code: Text Embedding Vulnerabilities
Reconstructing private text with minimal training data
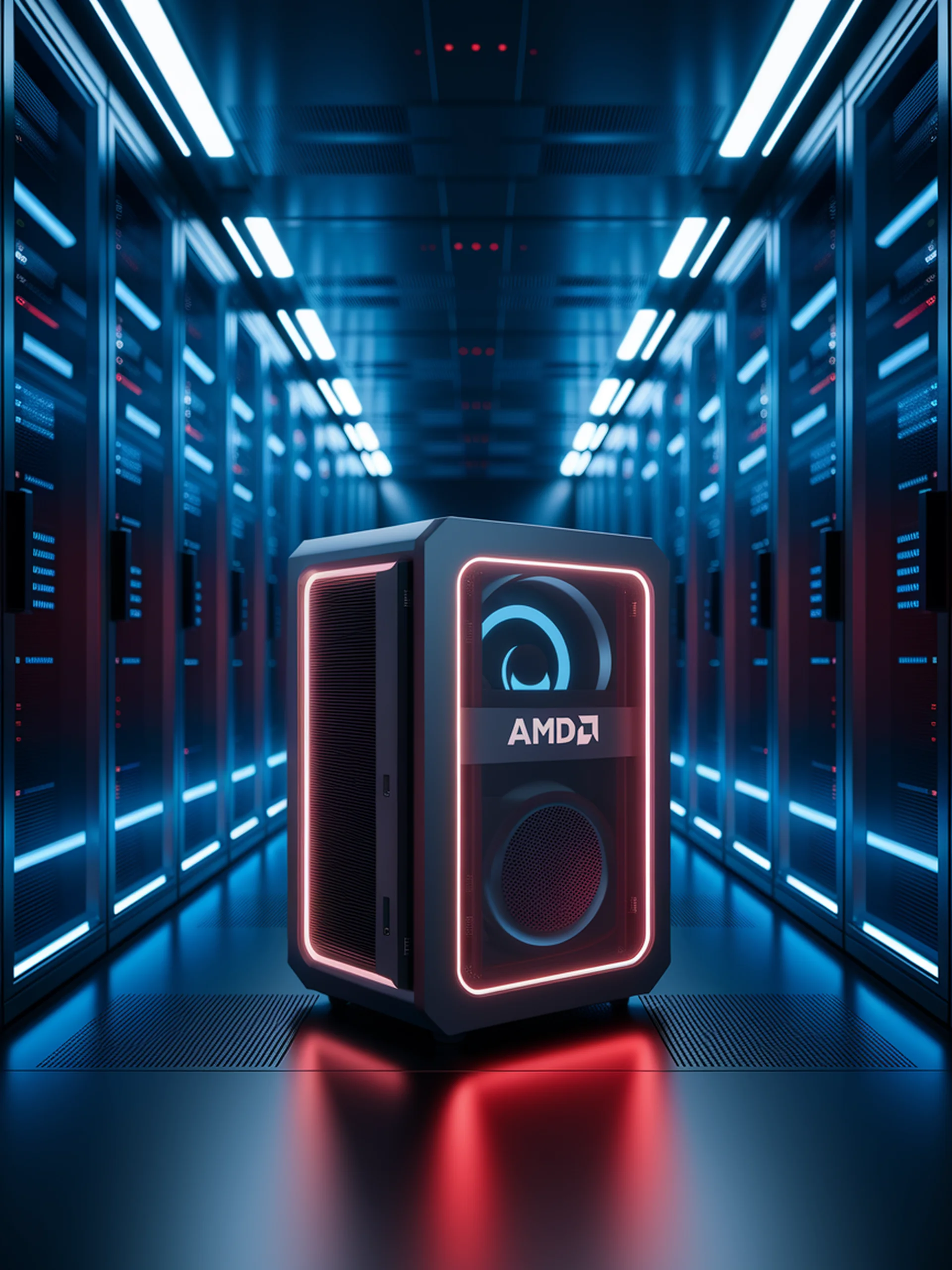
Secure LLMs in Confidential Computing
First evaluation of DeepSeek LLM in GPU-based Trusted Execution Environments
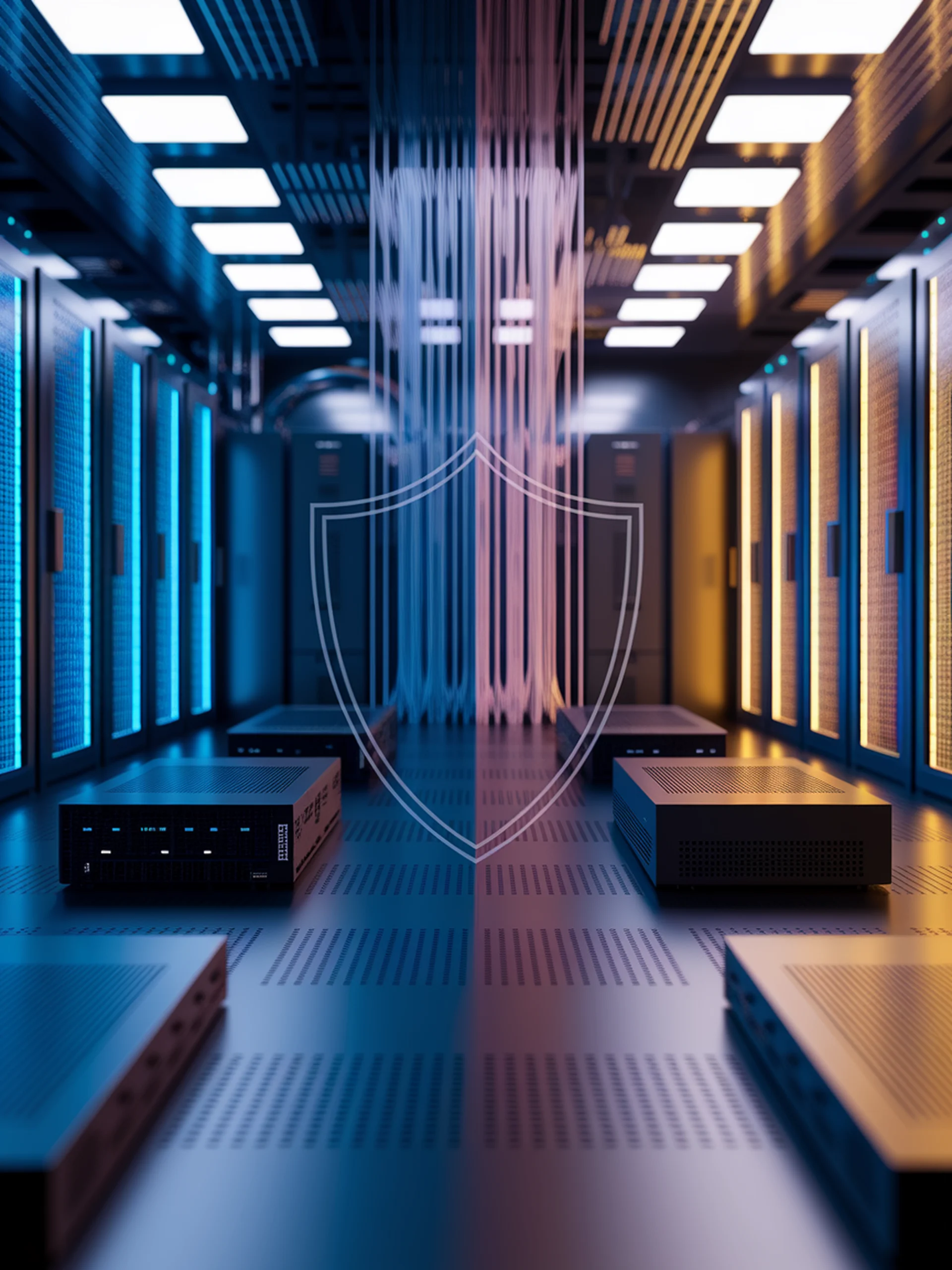
Balancing Privacy and Performance in LLM Fine-Tuning
Analyzing trade-offs between data security, model utility, and computational efficiency
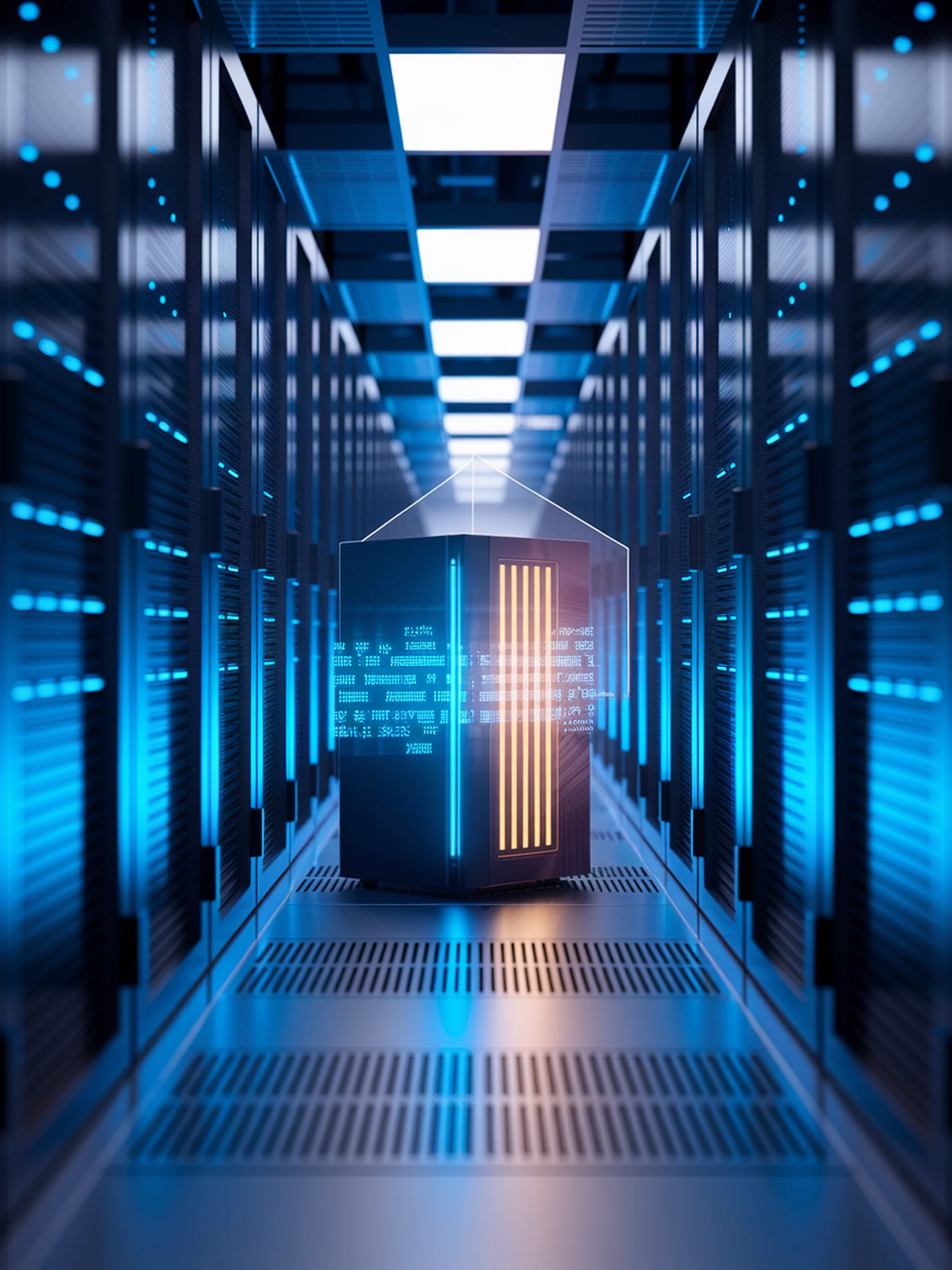
Securing User Privacy in LLM Interactions
A novel privacy preservation pipeline for cloud-based LLMs
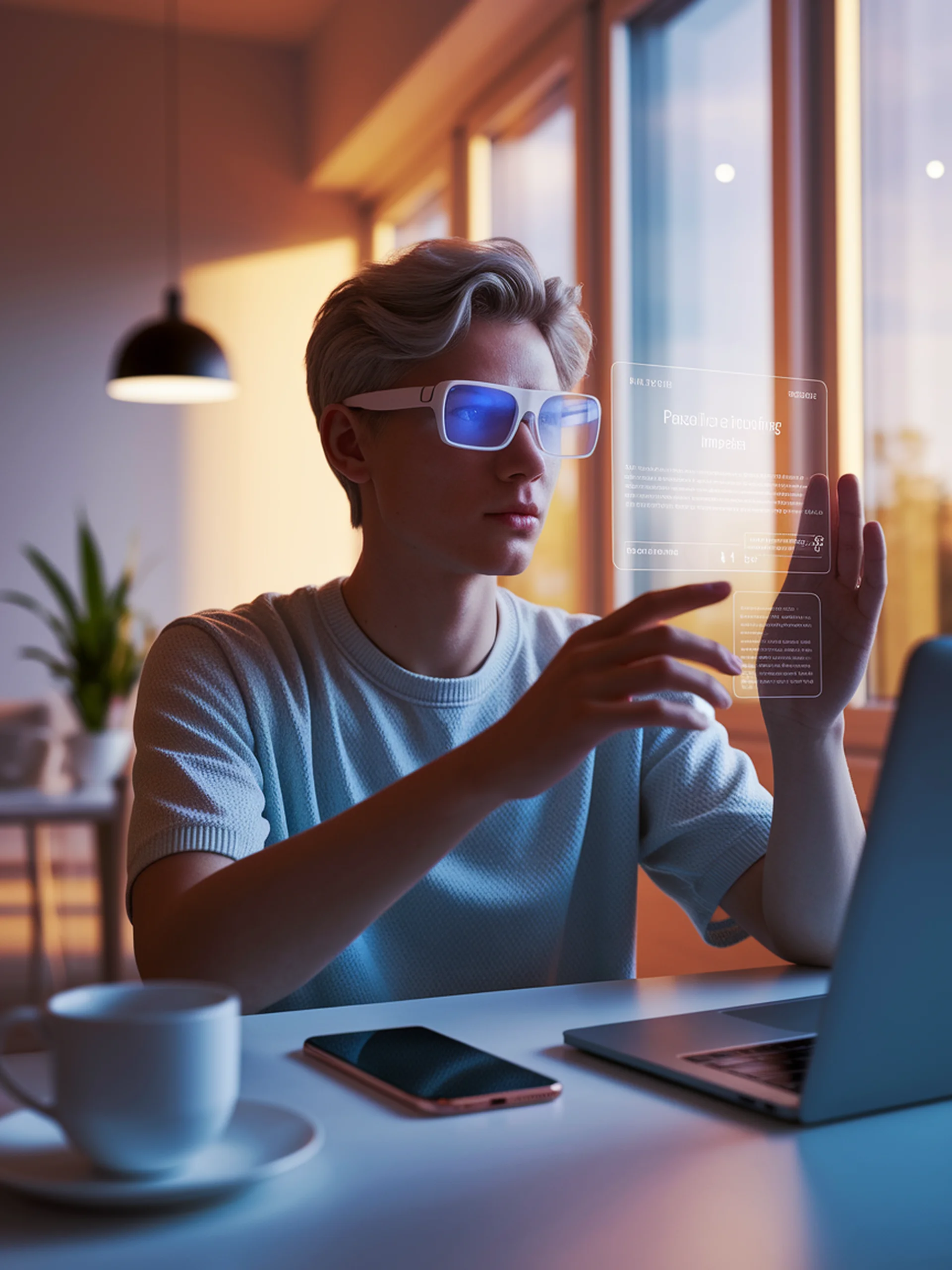
ReVision: Privacy-First Visual Interactions
Enabling on-device visual instruction processing without compromising privacy
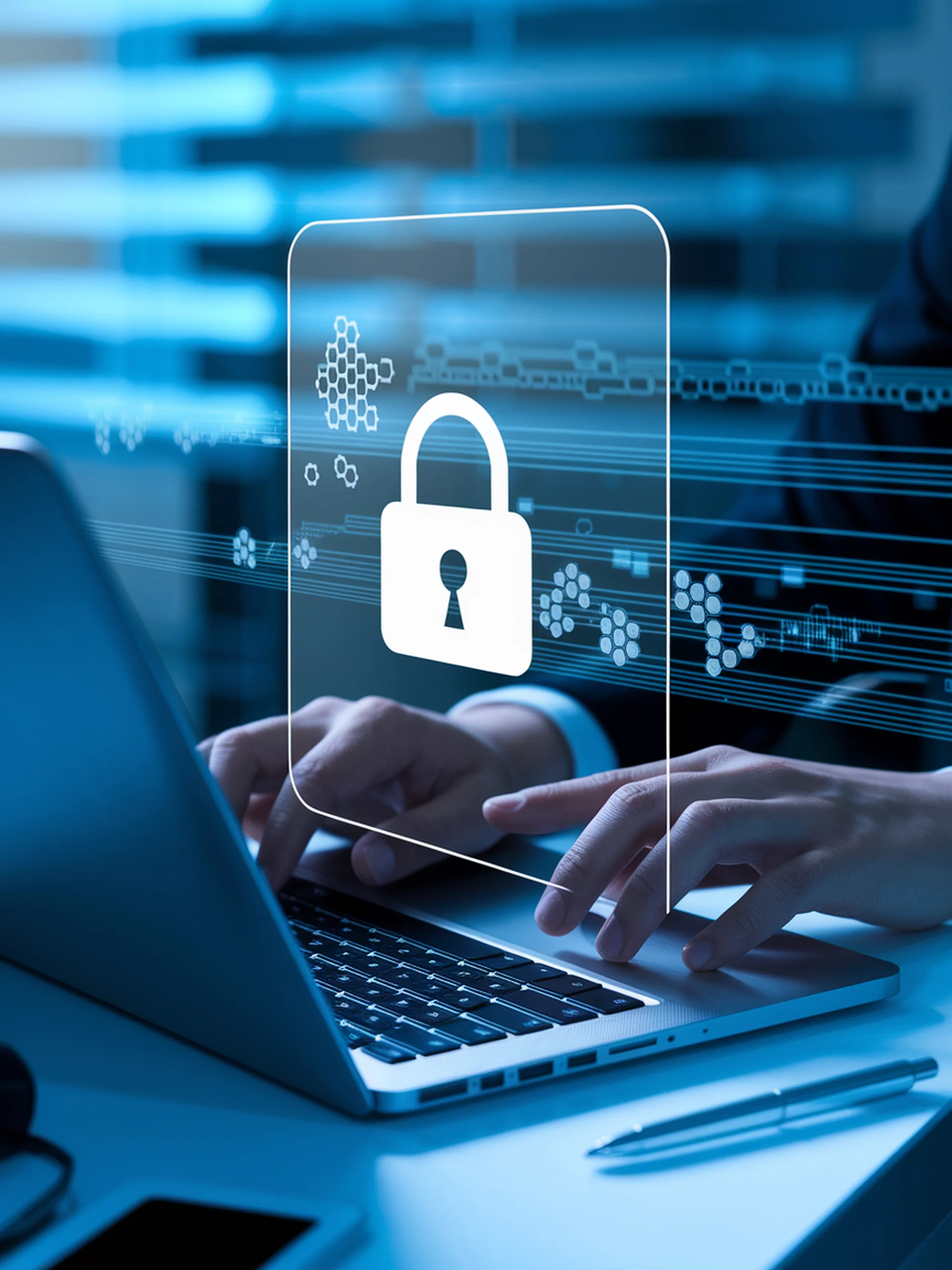
Protecting User Privacy in Cloud LLMs
A framework for pseudonymizing sensitive information in LLM prompts
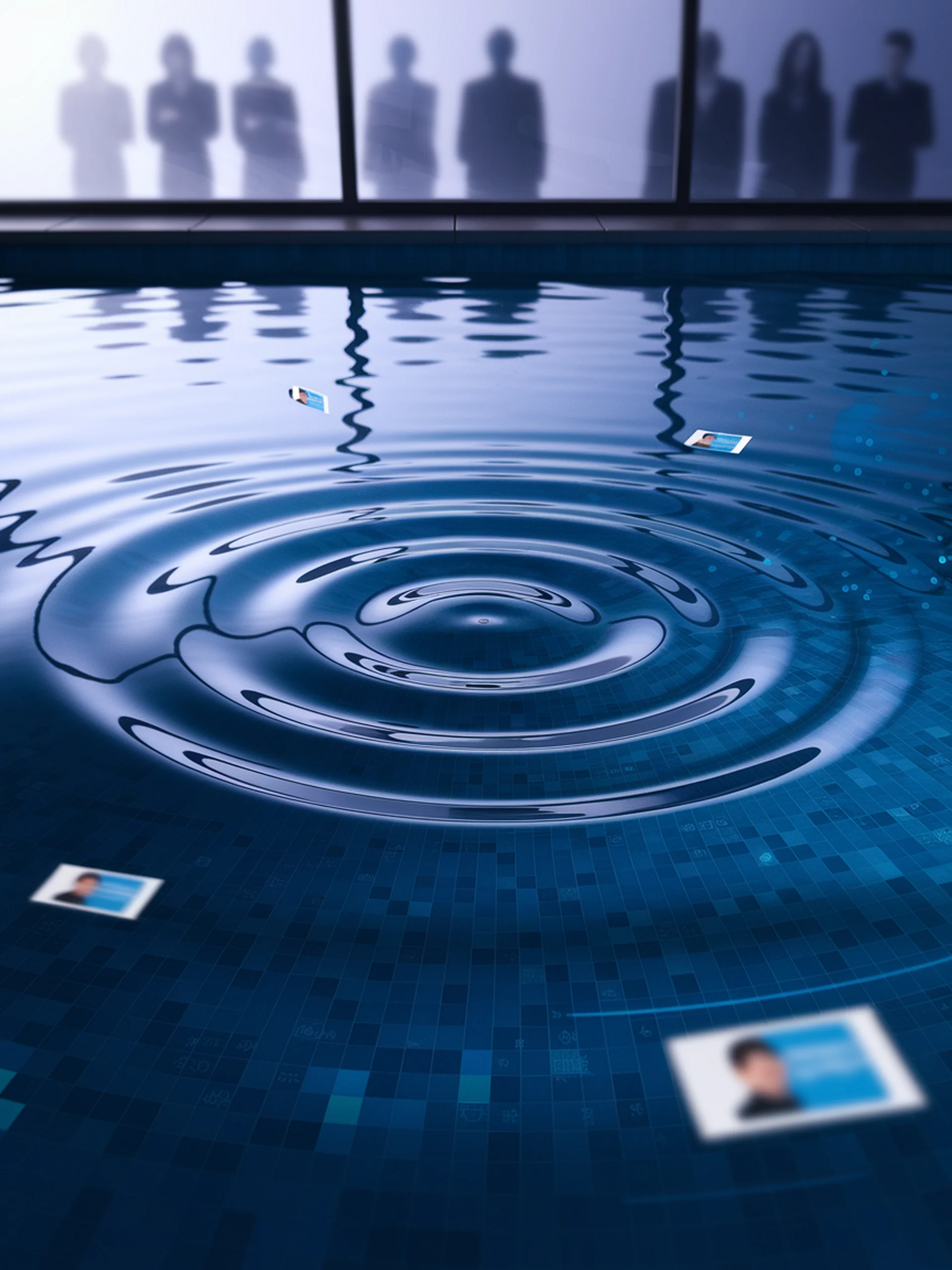
Privacy Ripples in Language Models
How adding or removing personal data impacts LLM privacy beyond the affected individual
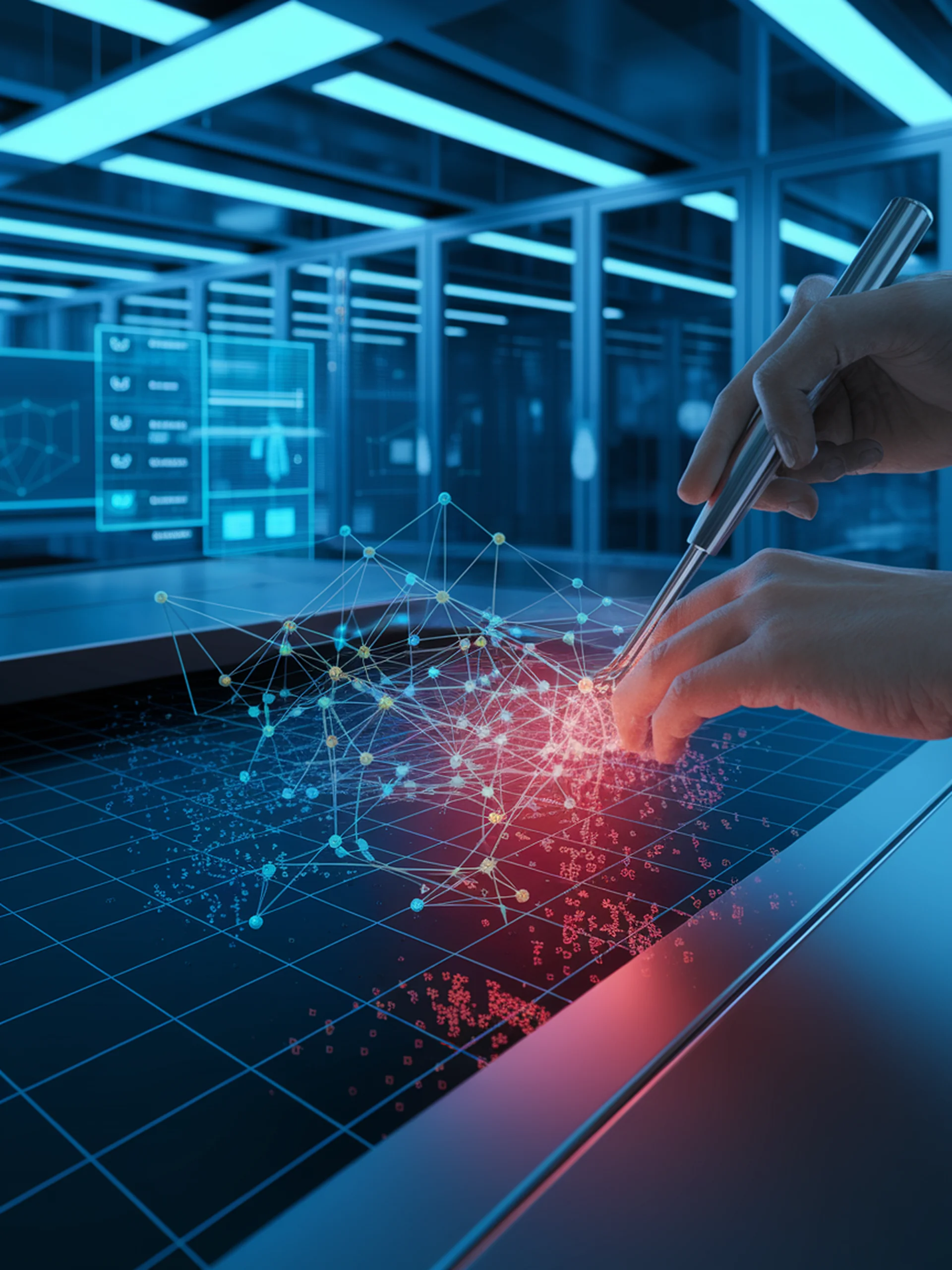
Pruning: A Simple Defense Against AI Memory Leaks
How model pruning reduces data memorization in Large Language Models
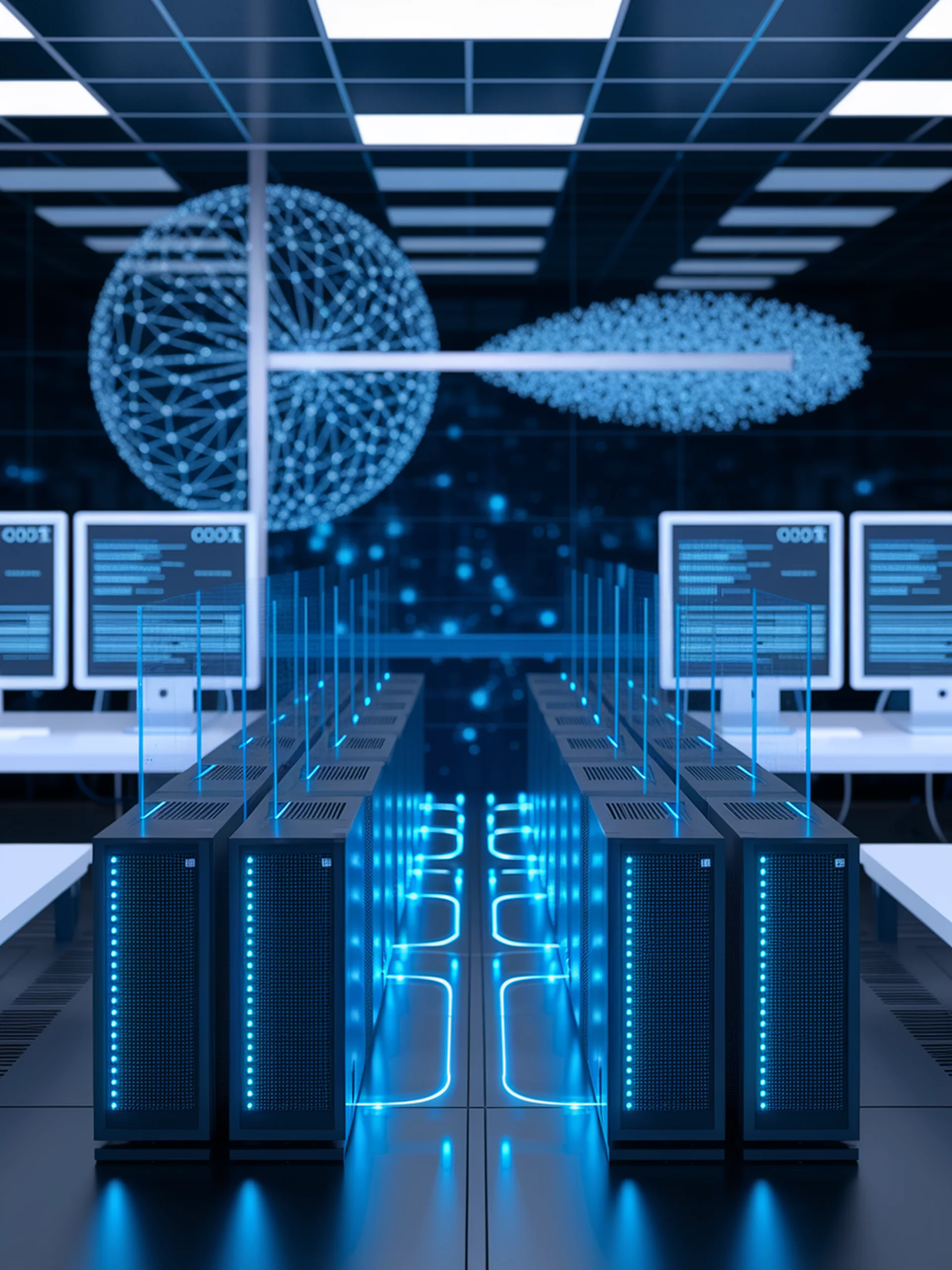
Private Compression of Large Language Models
Federated learning approach to create secure, task-specific small models
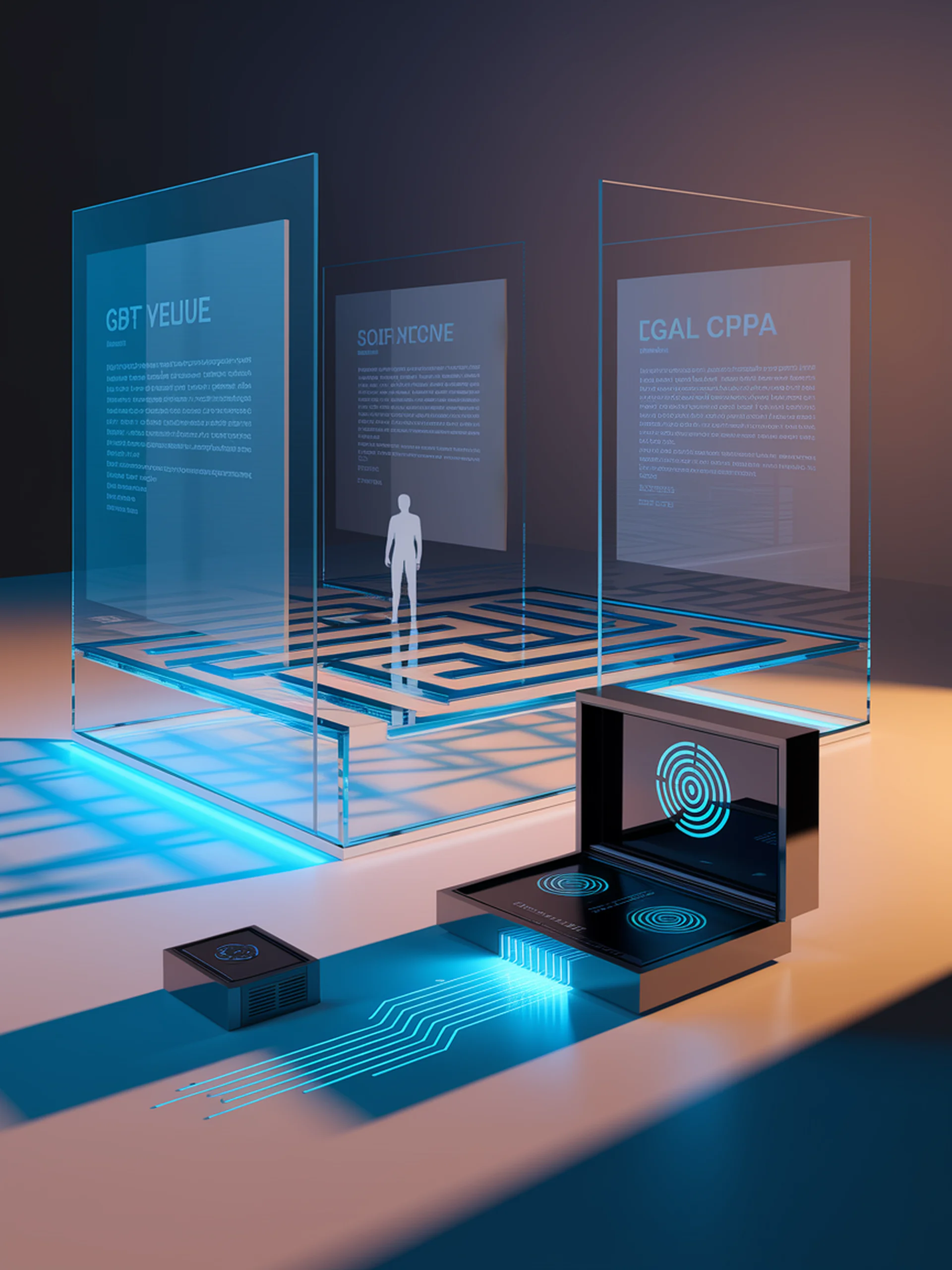
Evaluating AI Privacy: Beyond the Basics
A comprehensive framework for privacy evaluation in LLMs
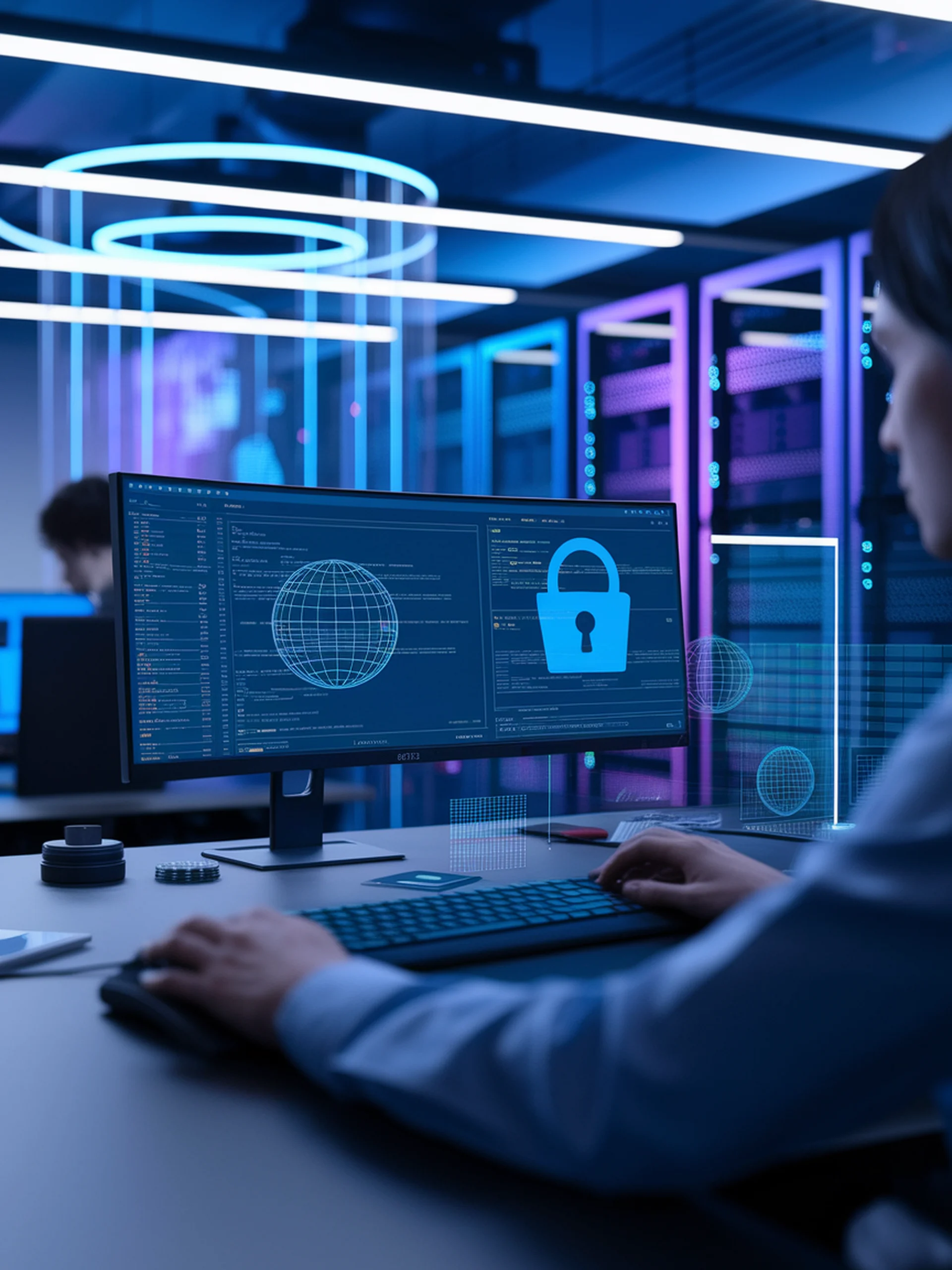
Protecting User Privacy in AI Feedback Systems
A novel approach to user-level privacy in RLHF for language models
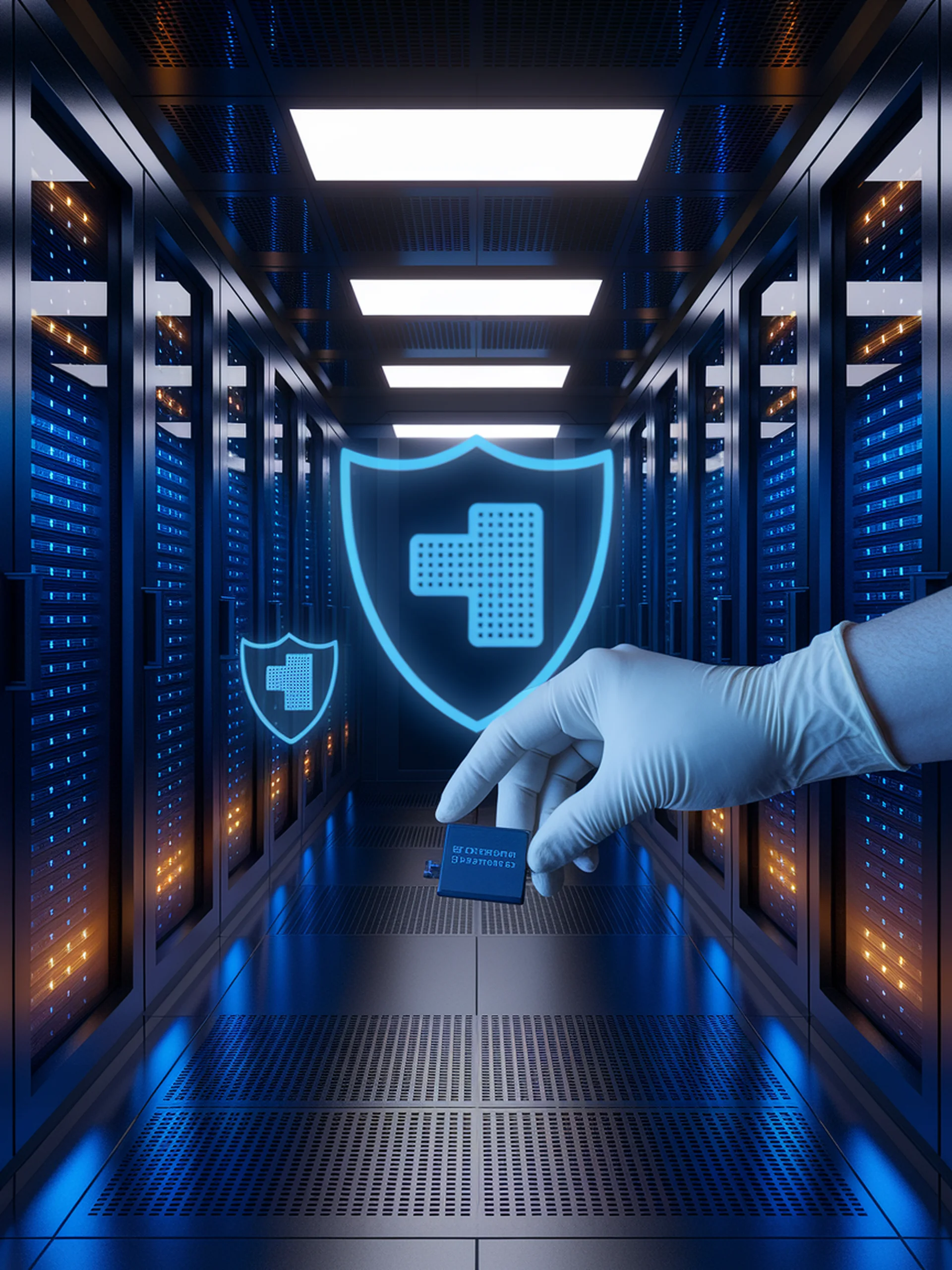
Protecting Privacy in LLMs
Achieving robust PII protection without sacrificing model performance
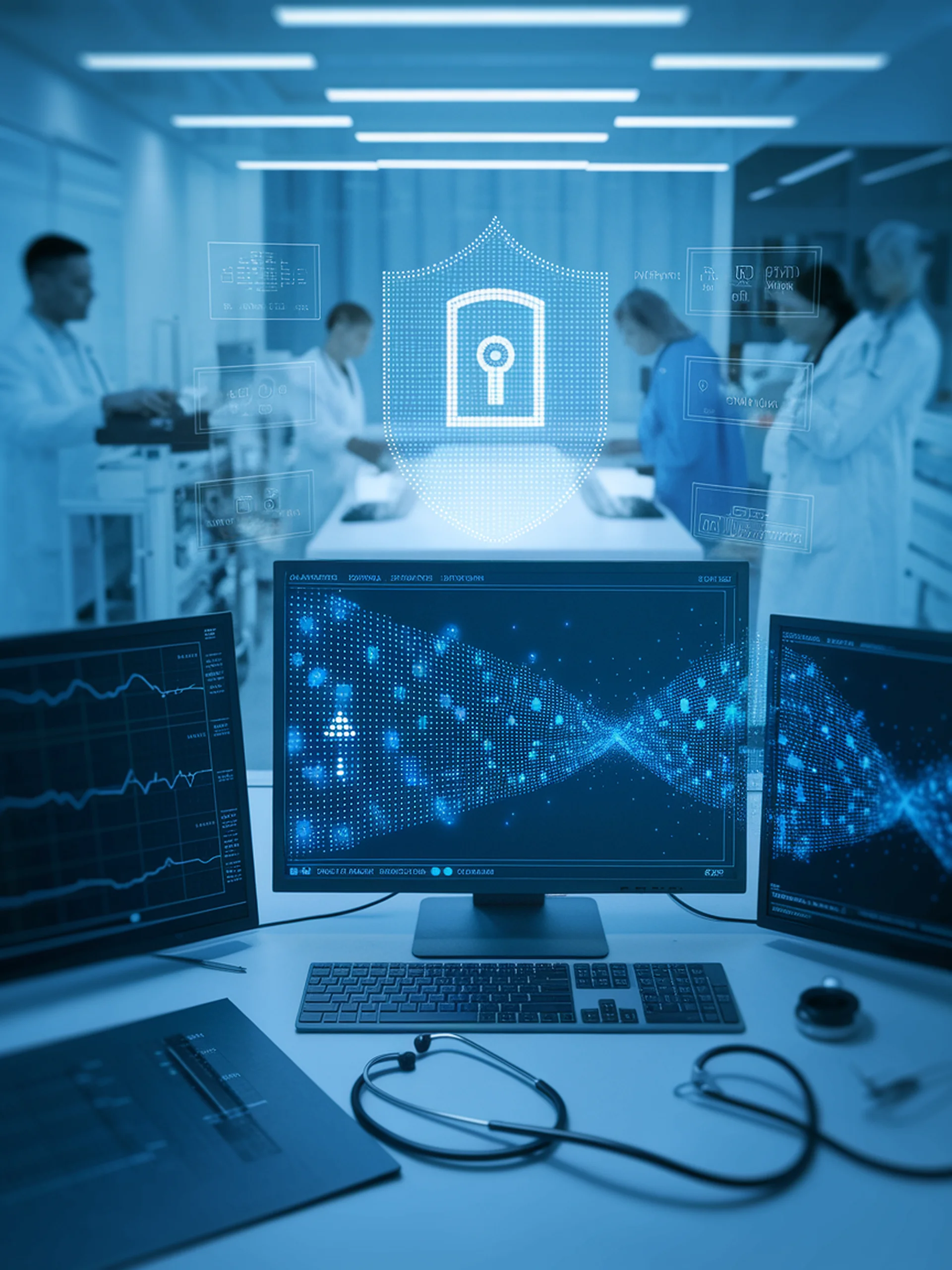
Privacy-Preserving LLMs in Healthcare
Generating high-quality synthetic data for sensitive domains
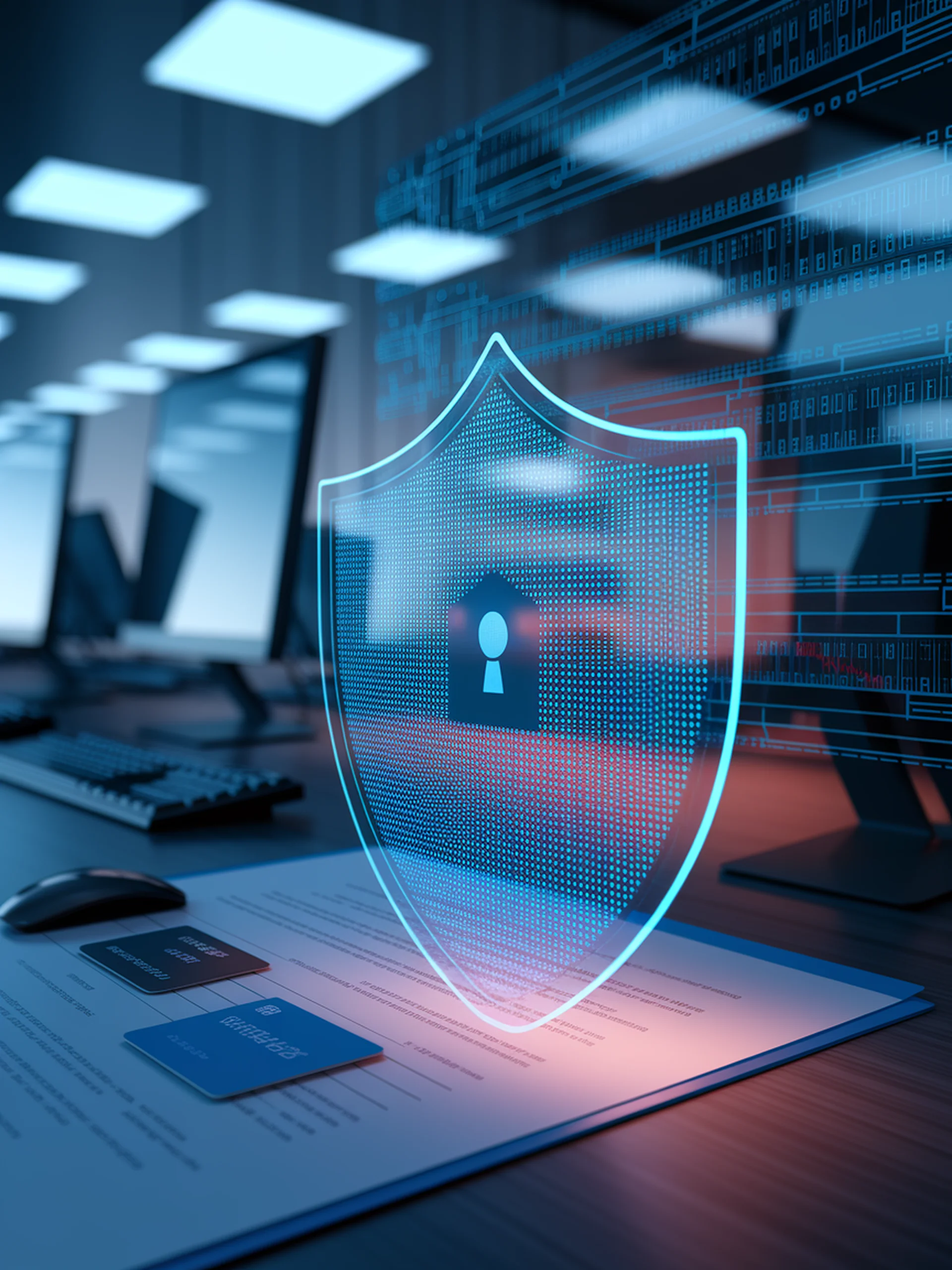
Protecting Privacy in LLM Interactions
First benchmark for evaluating PII protection systems
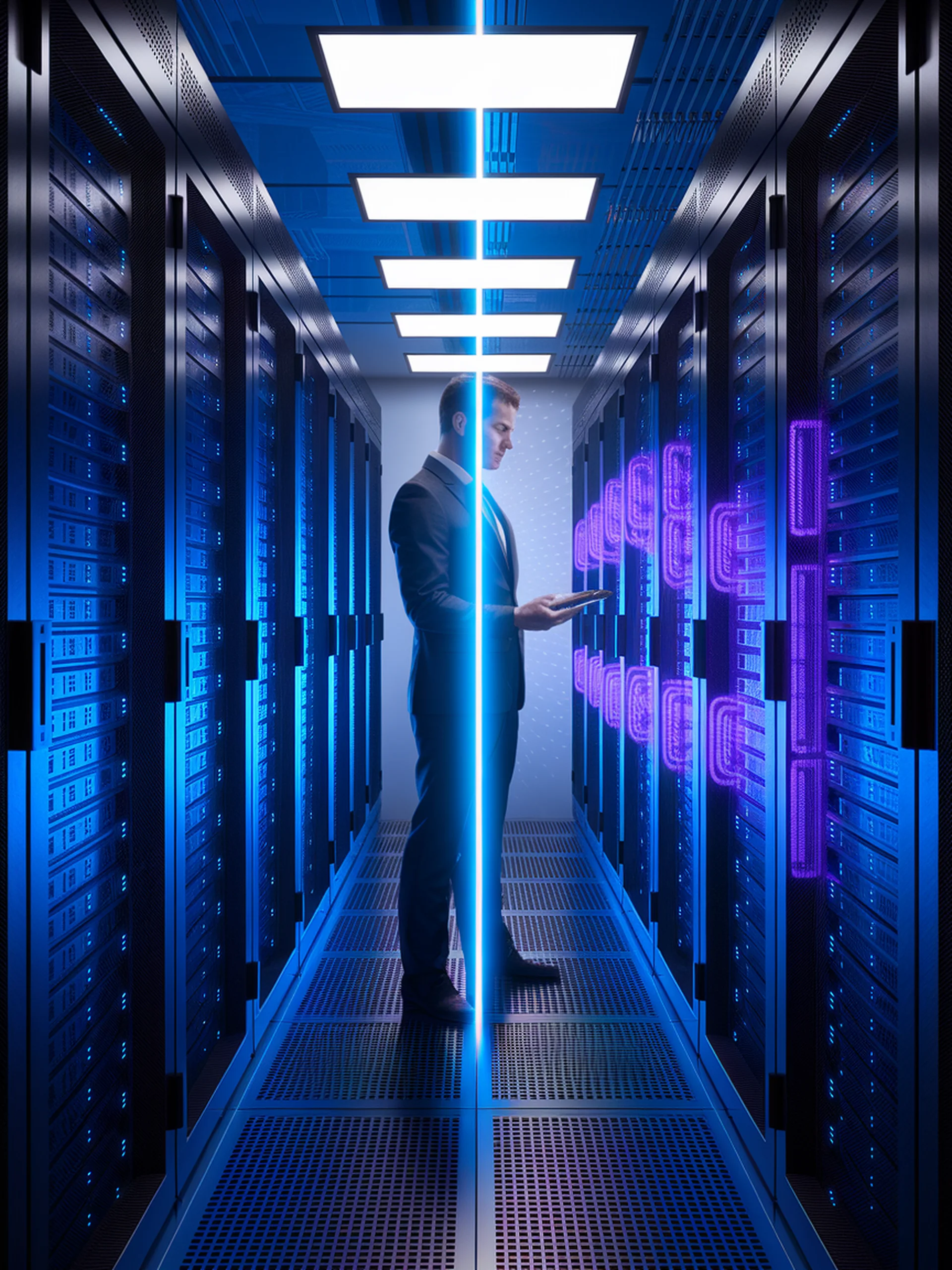
Defending LLMs Against Privacy Attacks
A novel dual-purpose token approach to protect sensitive training data
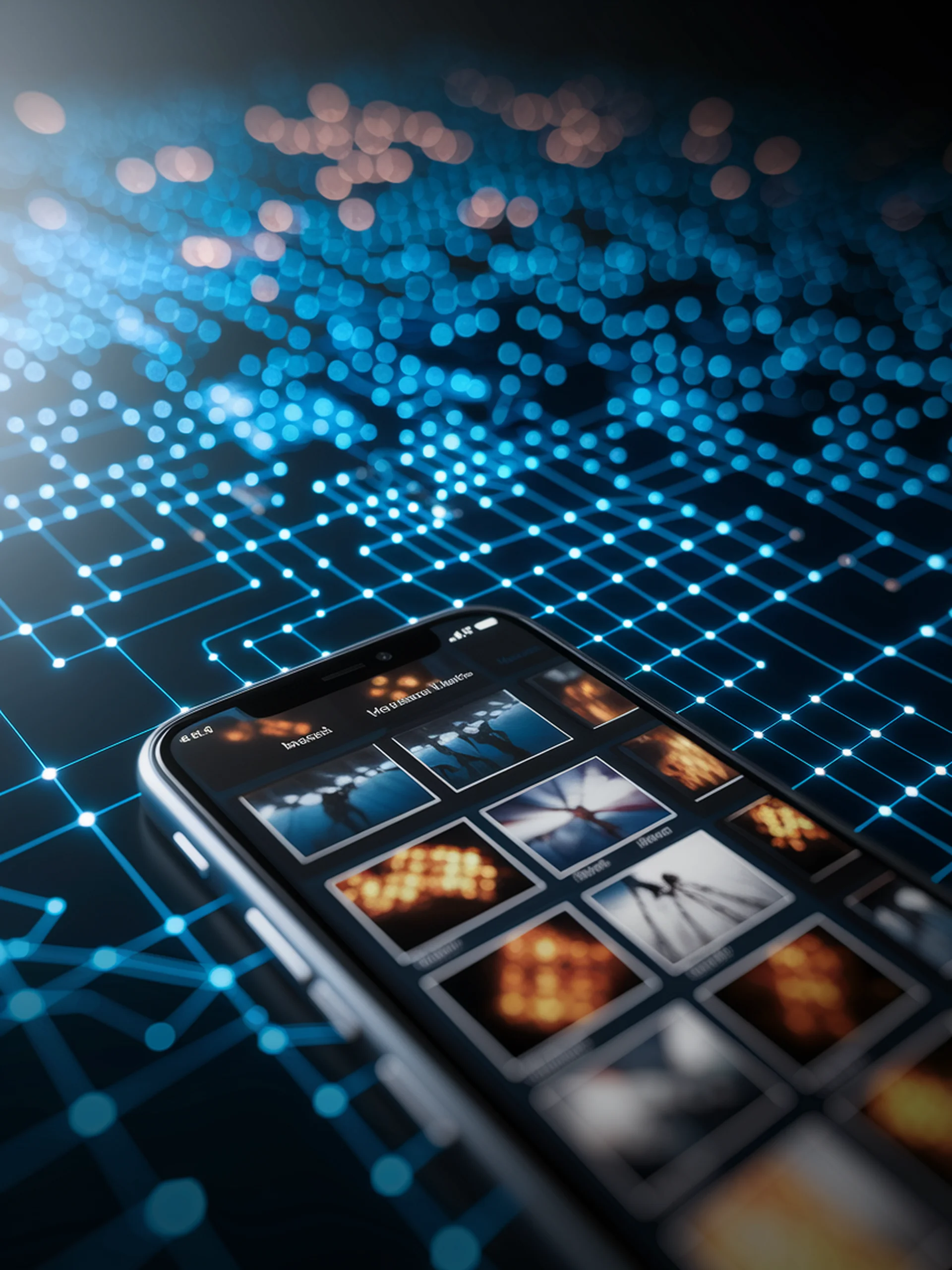
The Hidden Memory of AI Models
How MLLMs Inadvertently Memorize Your Private Images
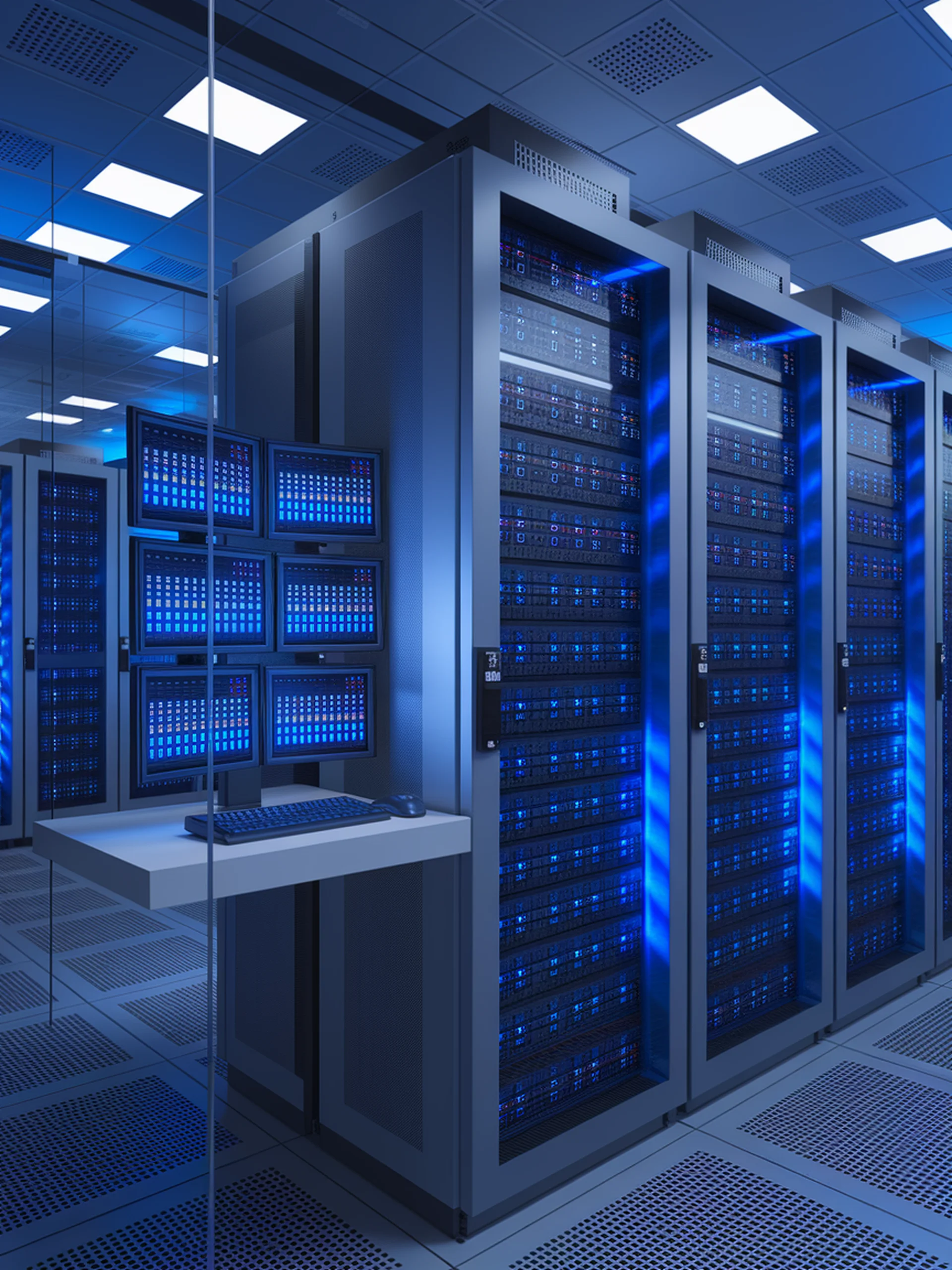
Secure LLM Implementation Architecture
A data-protection compliant framework for enterprise LLM deployment
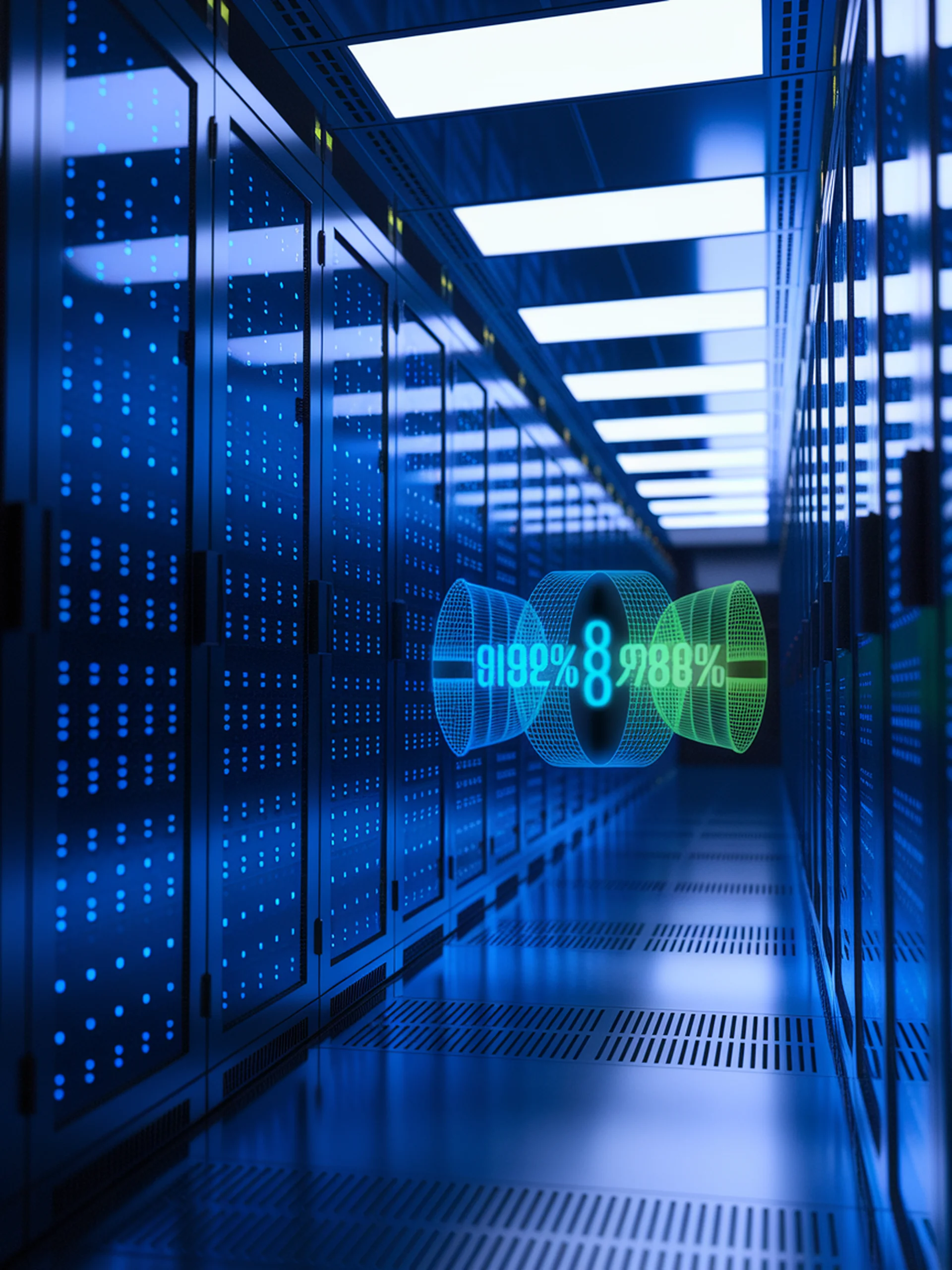
Smarter Model Storage for Privacy-Preserving AI
Balancing Storage Efficiency with Privacy Guarantees
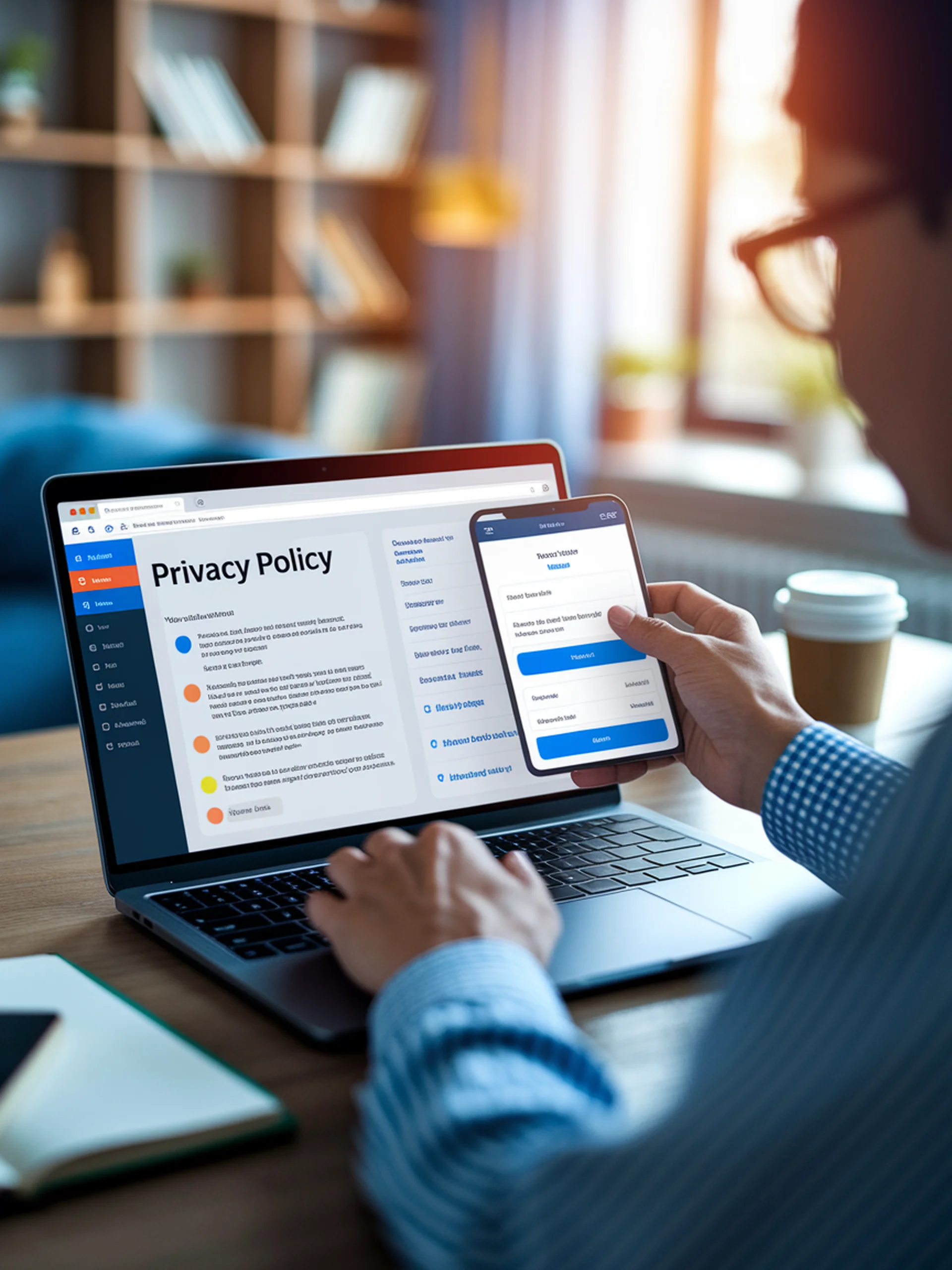
Making Privacy Policies Accessible with AI
LLM-powered browser extension to demystify complex privacy terms
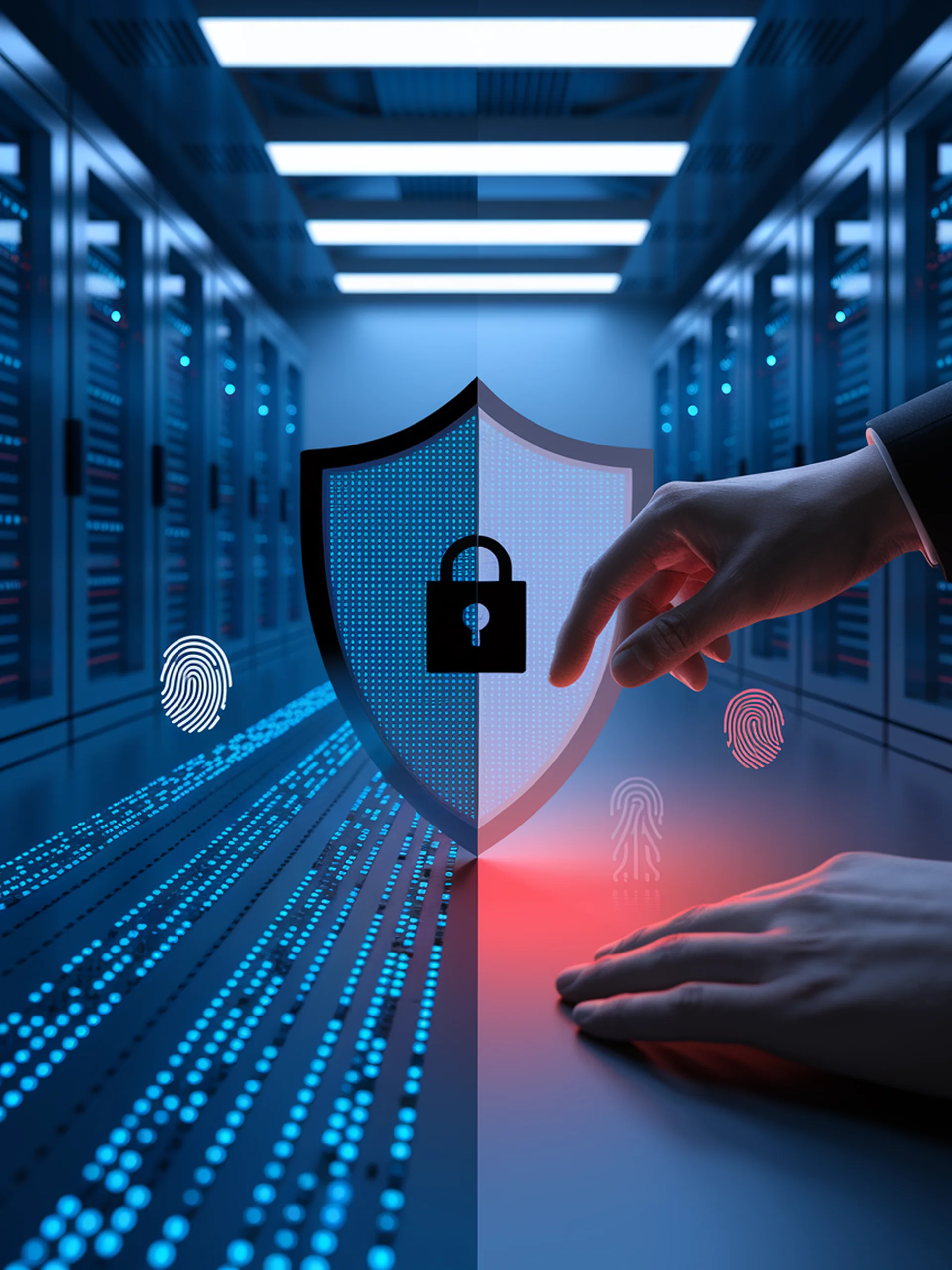
Safeguarding Privacy in LLM Interactions
A token-level approach to protect sensitive data
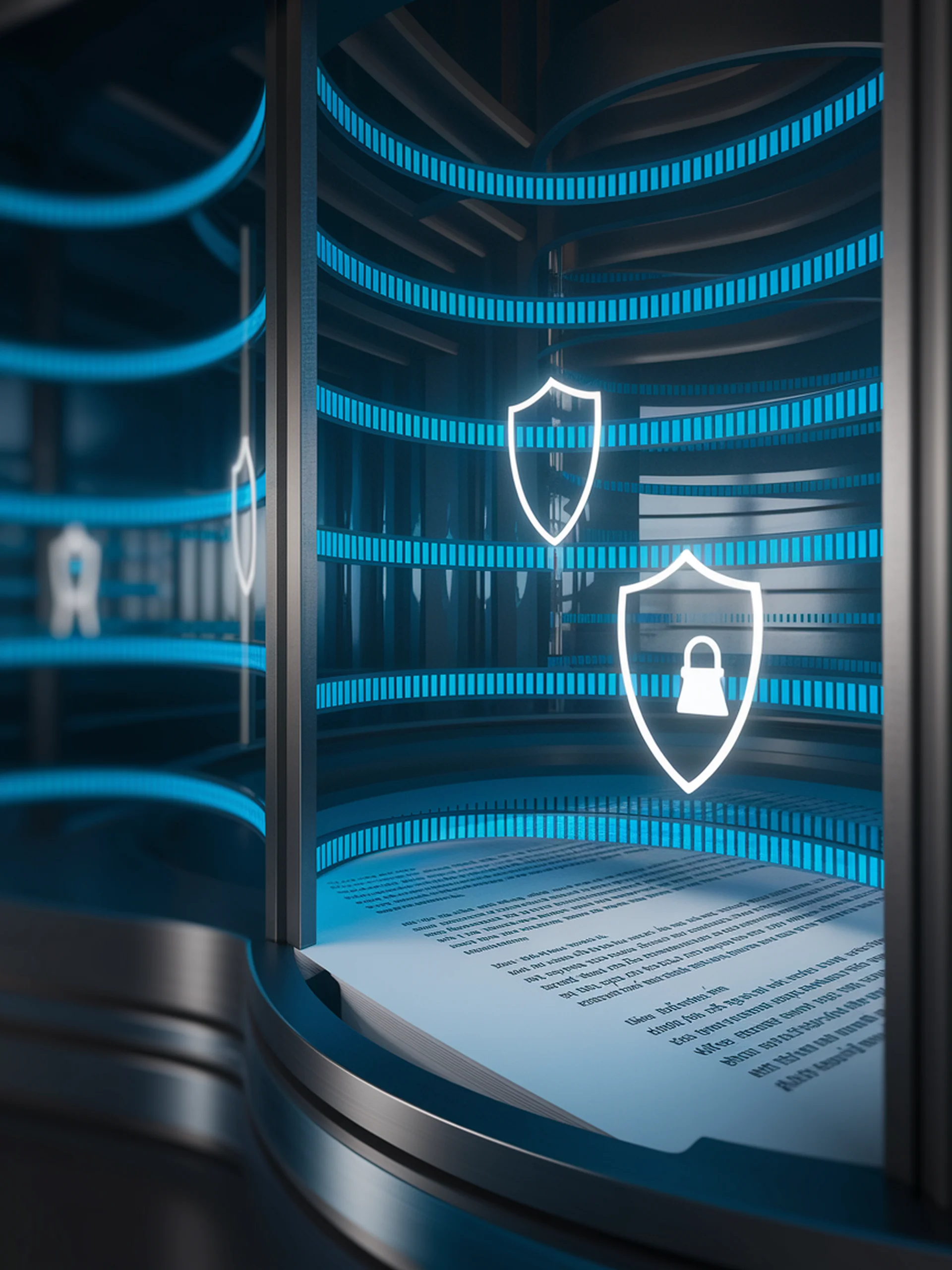
Securing Your LLM Prompts
Protecting sensitive information through multi-level text rewriting
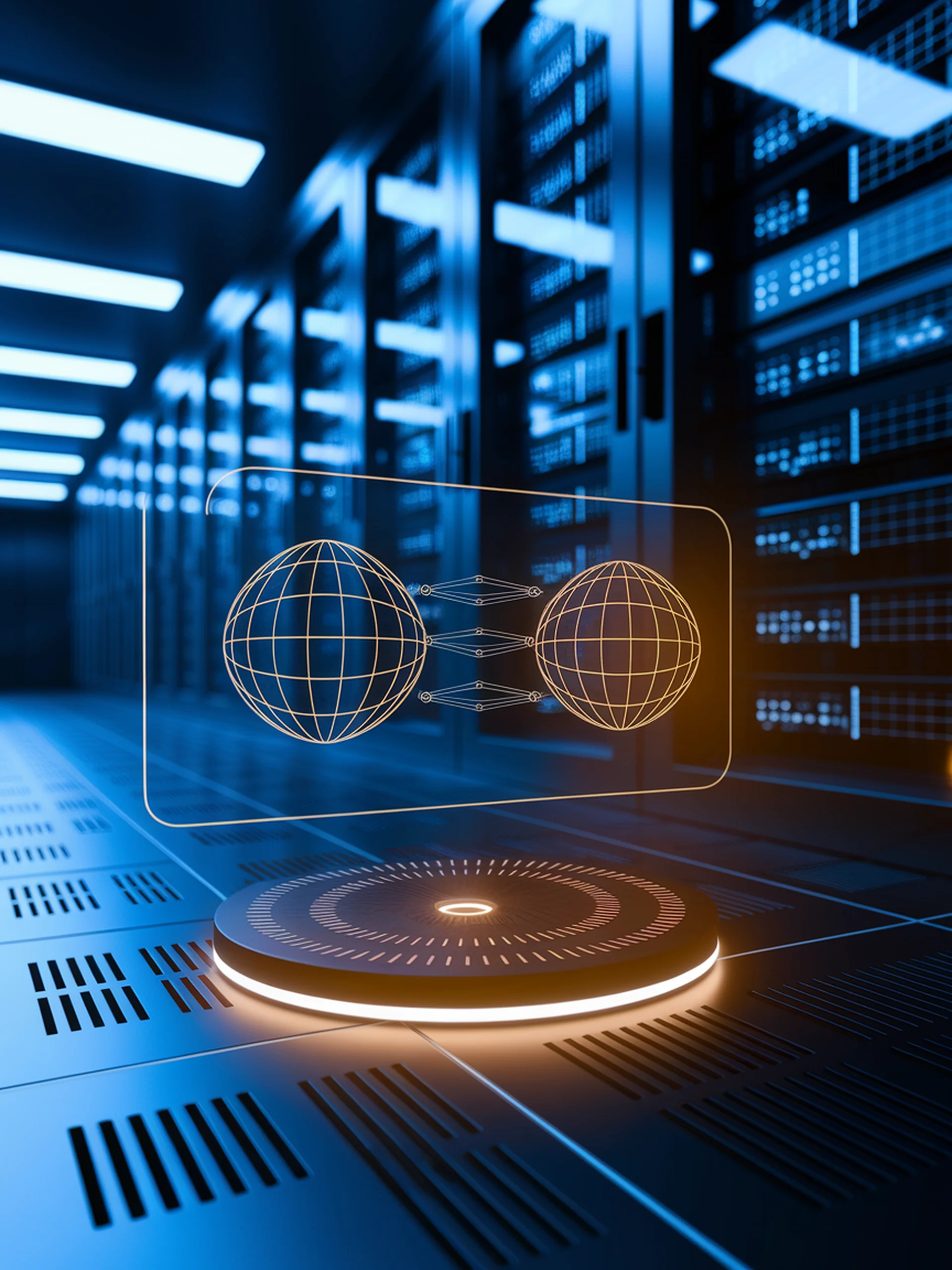
Securing Vector Similarity Search
Enabling Privacy-Preserving AI with Partially Homomorphic Encryption
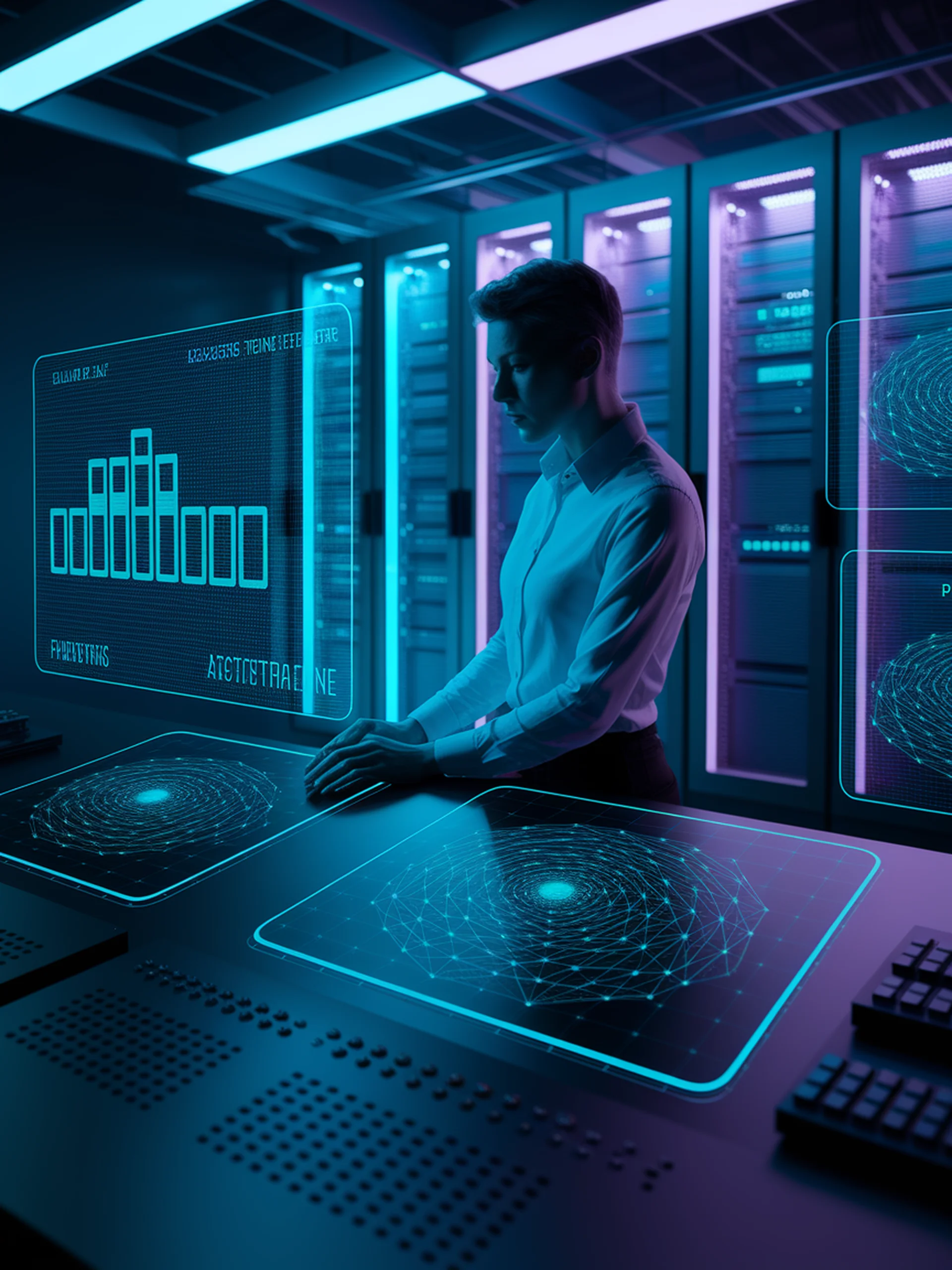
Securing LLMs Against Data Leakage
Using Activation Steering to Reduce Memorization While Preserving Performance
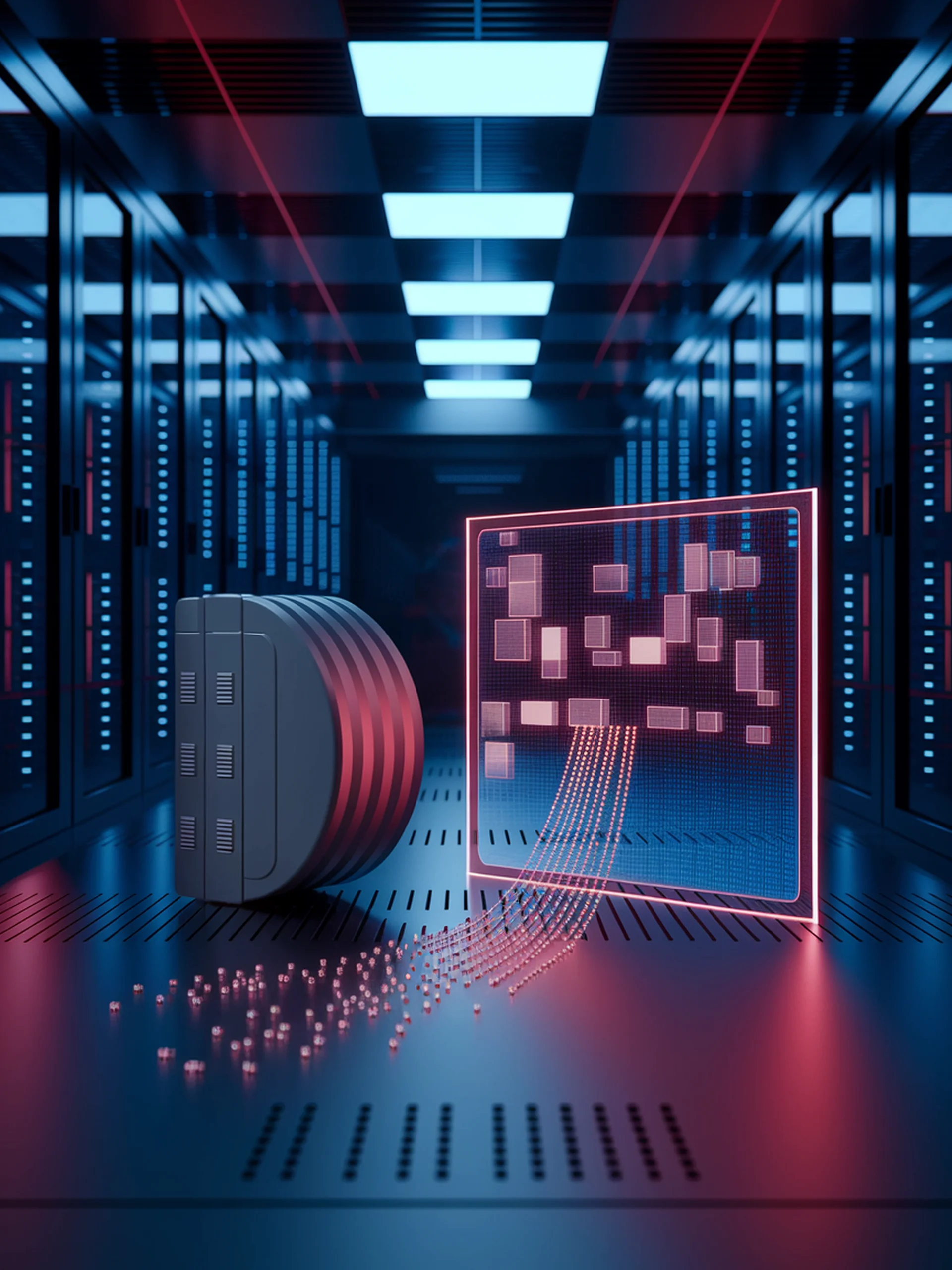
Exposing Privacy Vulnerabilities in LLMs
Advanced techniques to audit and measure privacy leakage in language models
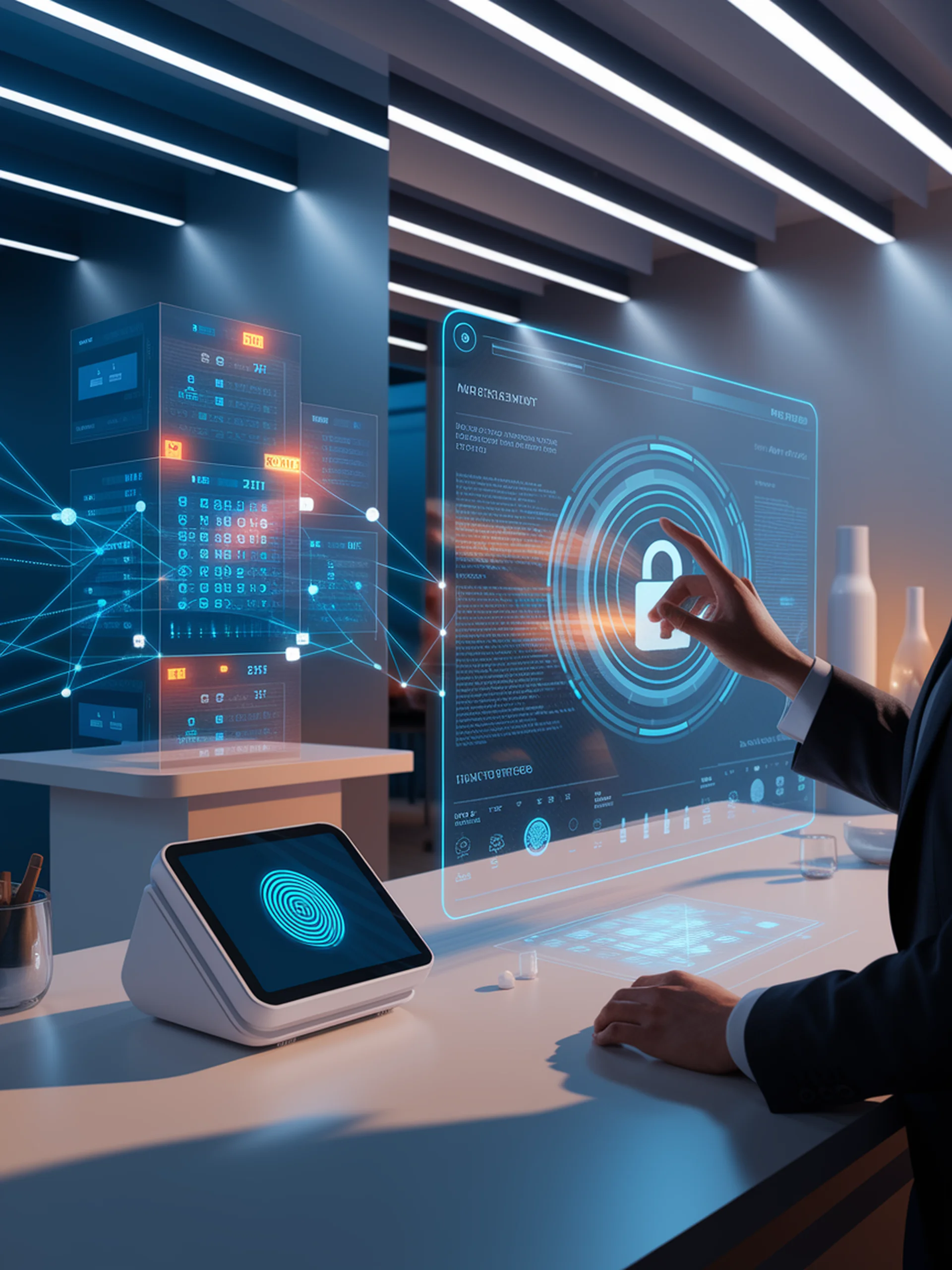
SECOND ME: Reinventing Personal Digital Identity
AI-powered memory system that reduces repetitive information sharing
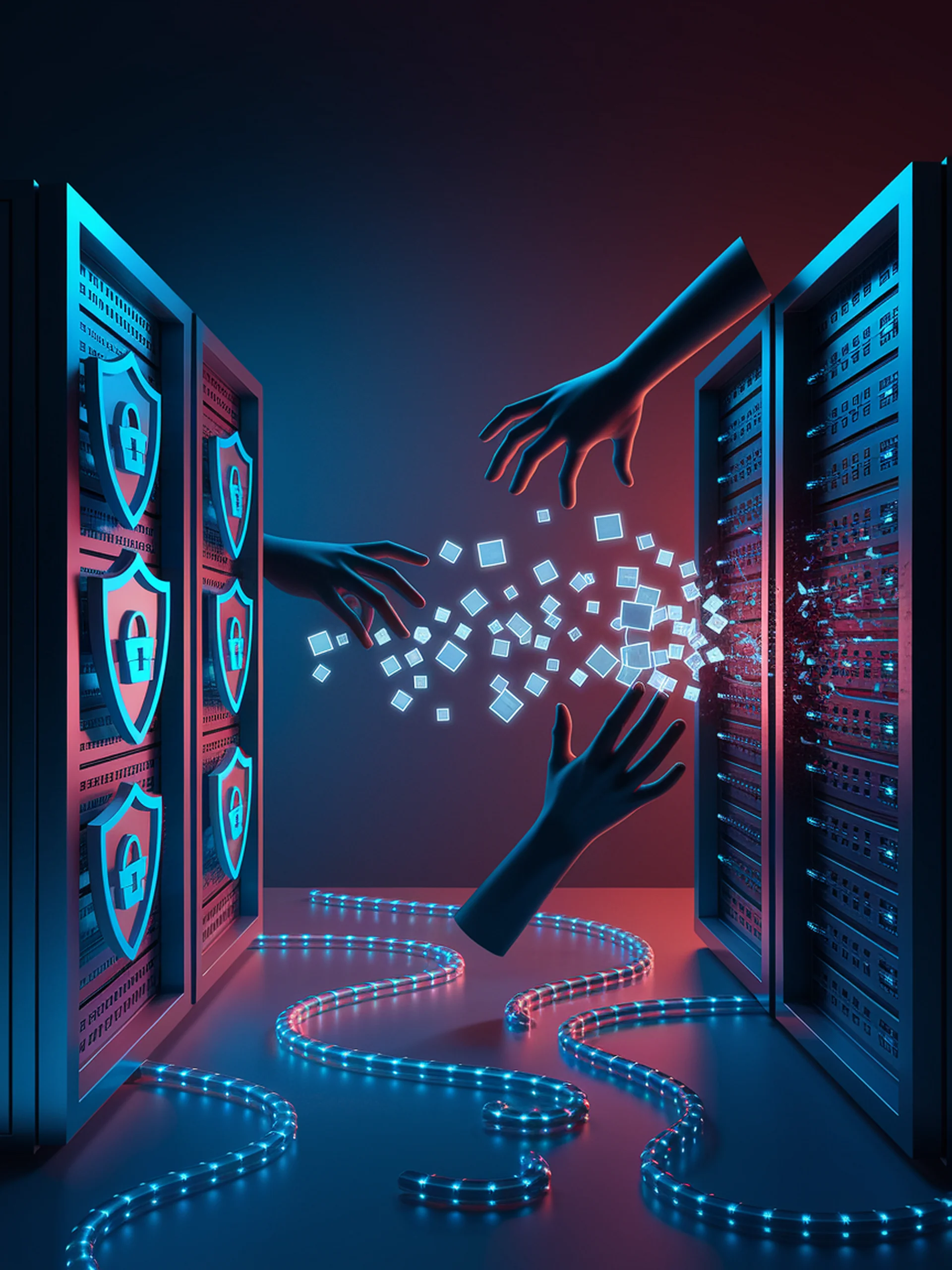
Security Threat: Exposing Vulnerabilities in LLM Collaboration
How attackers can recover sensitive prompts in distributed LLM systems
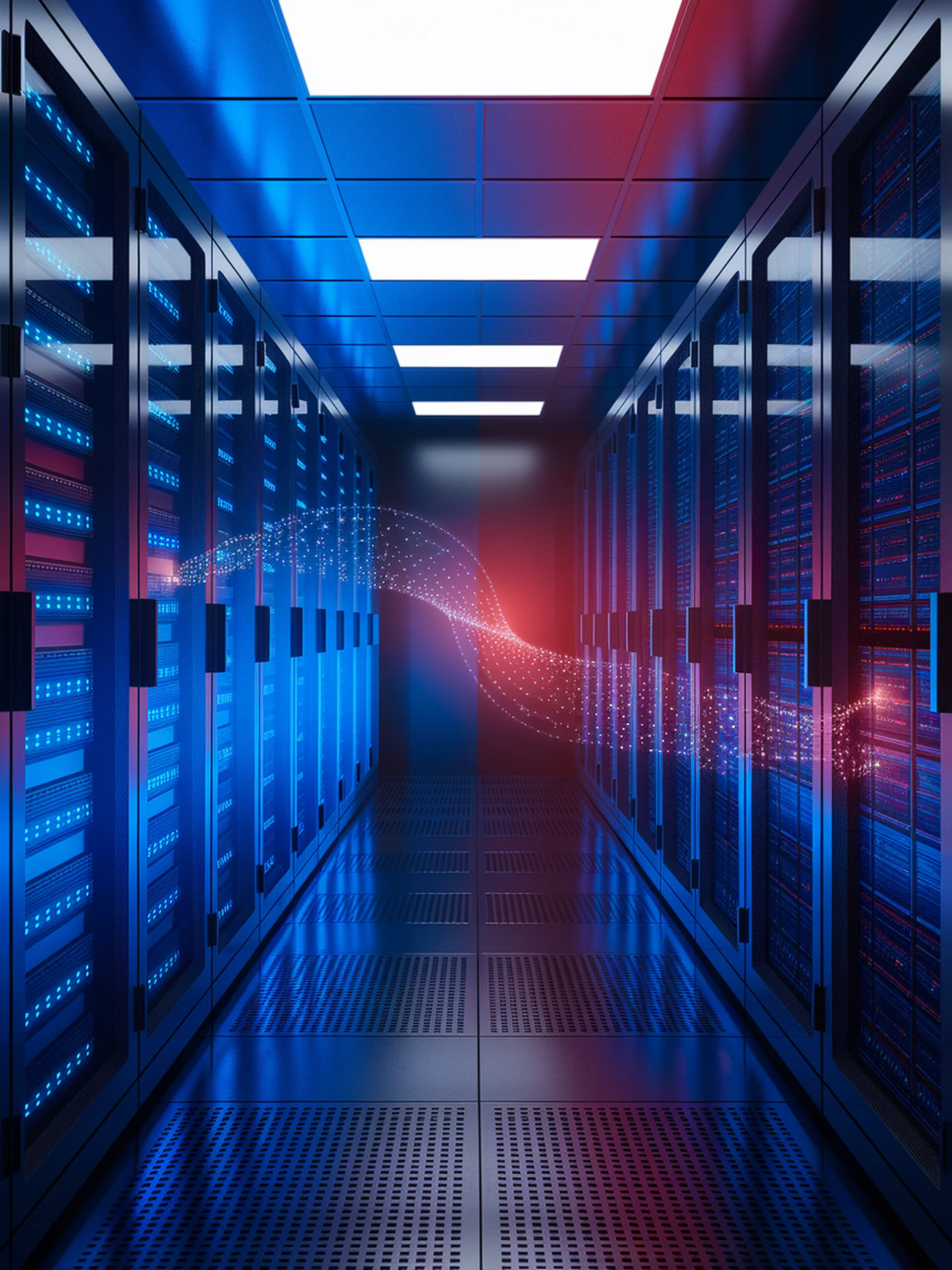
Security Vulnerabilities in Distributed LLM Inference
How attackers can reconstruct private prompts from intermediate outputs
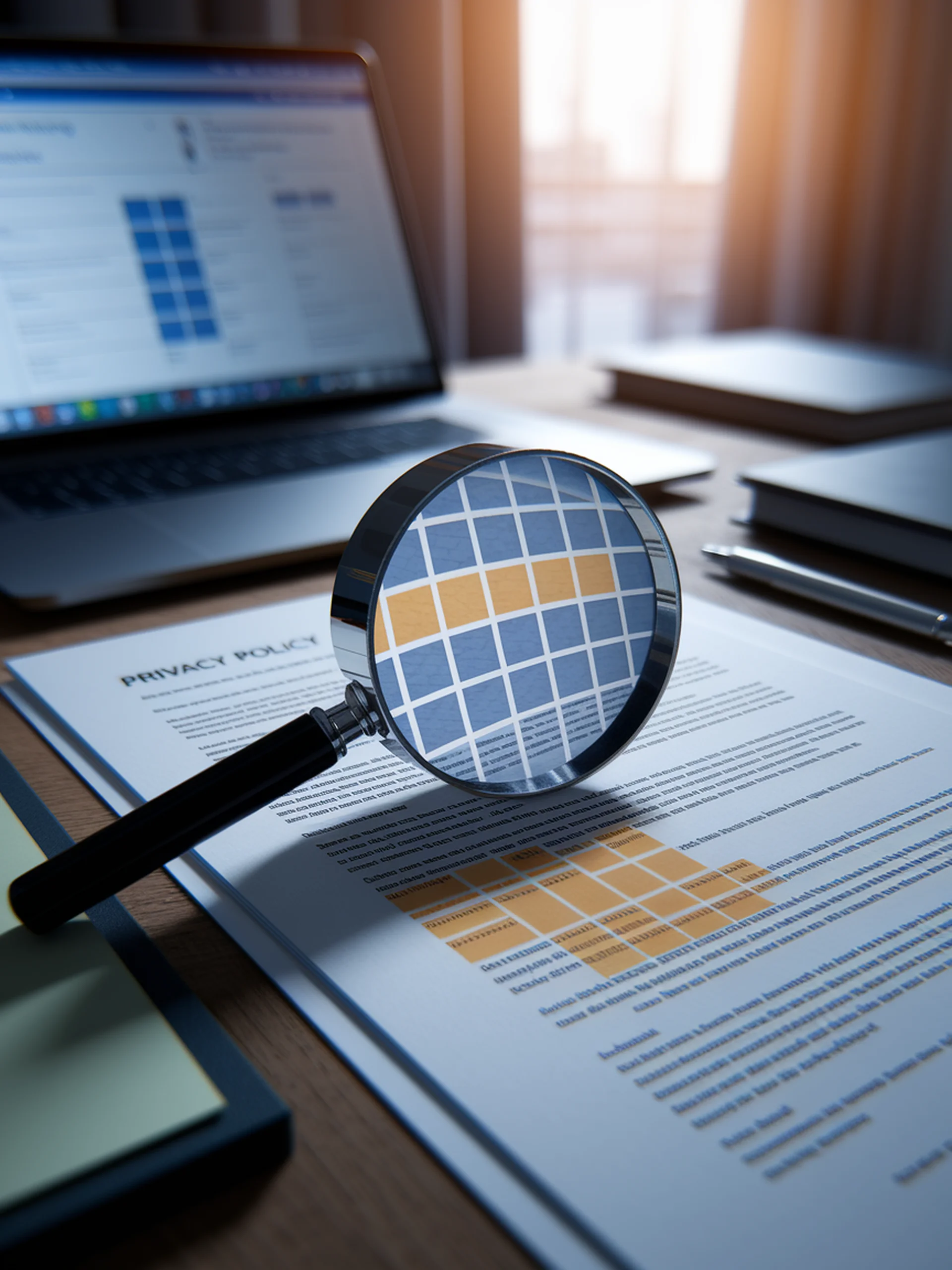
Mind the Gap: LLMs and Privacy Documents
Identifying interpretation problems when AI simplifies privacy policies
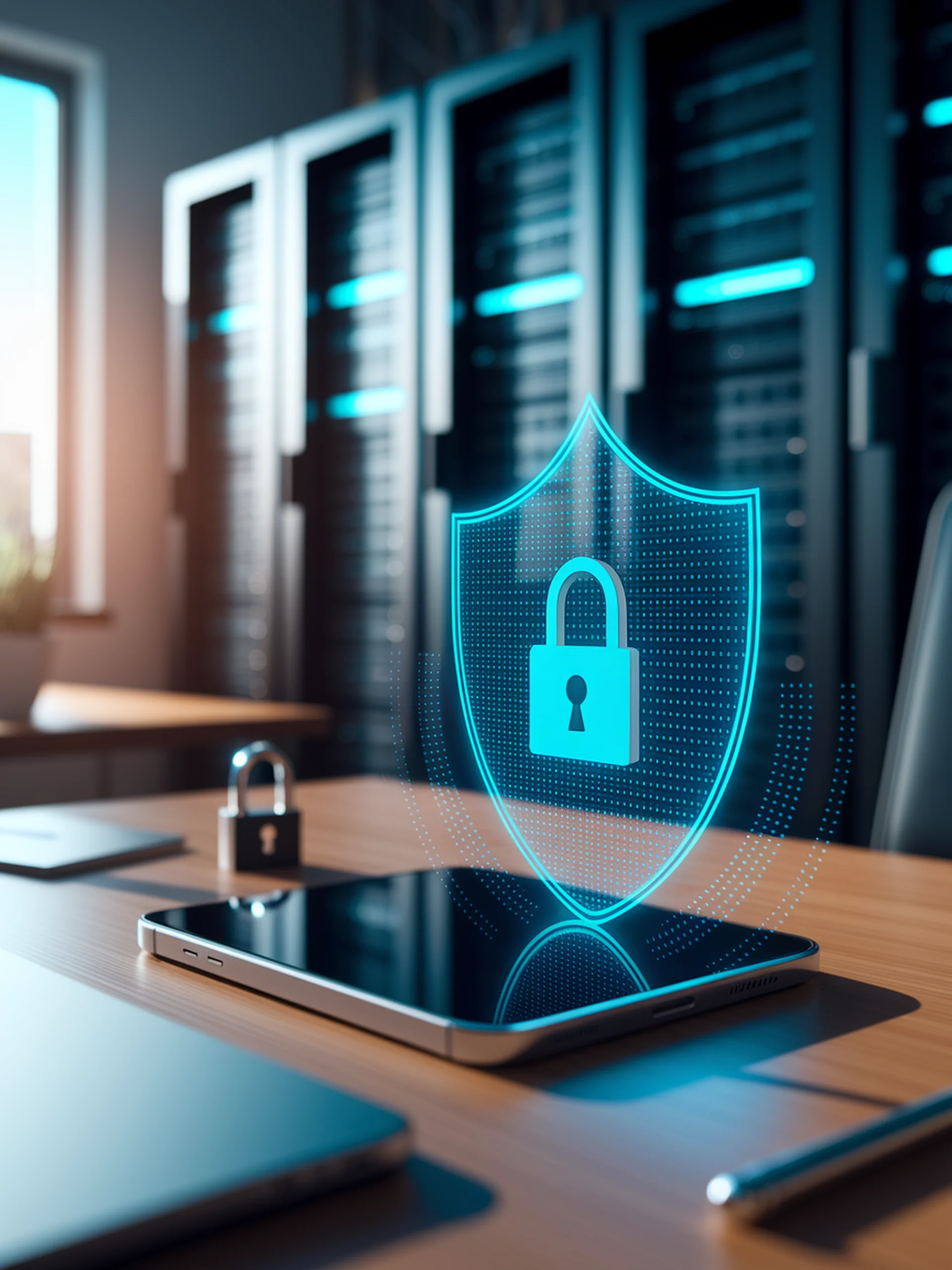
Secure LLM Adaptation on Edge Devices
Privacy-Preserving AI Customization with Limited Resources
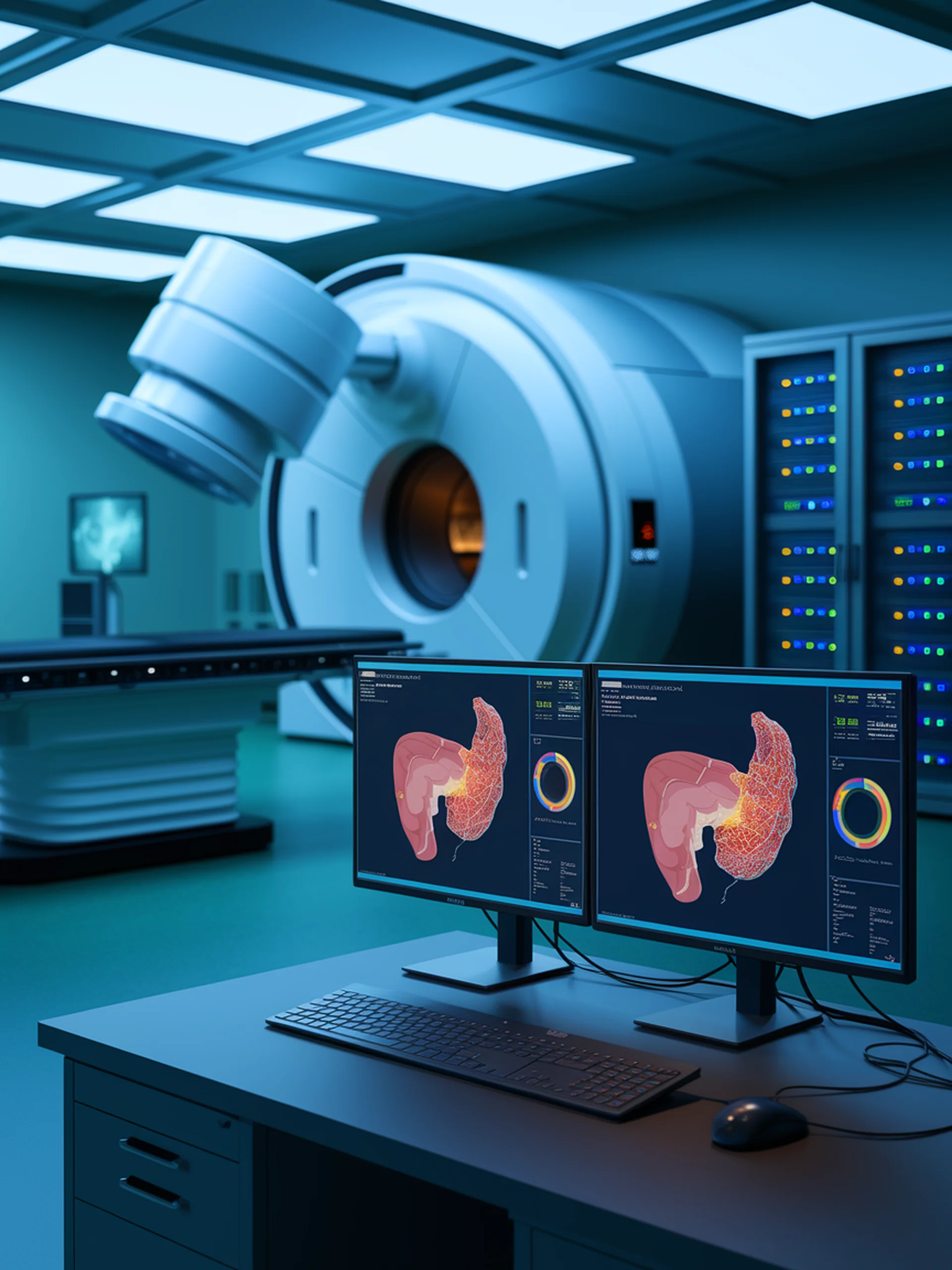
AI-Powered Radiotherapy Planning
Autonomous LLM agent optimizes cancer treatment while preserving privacy
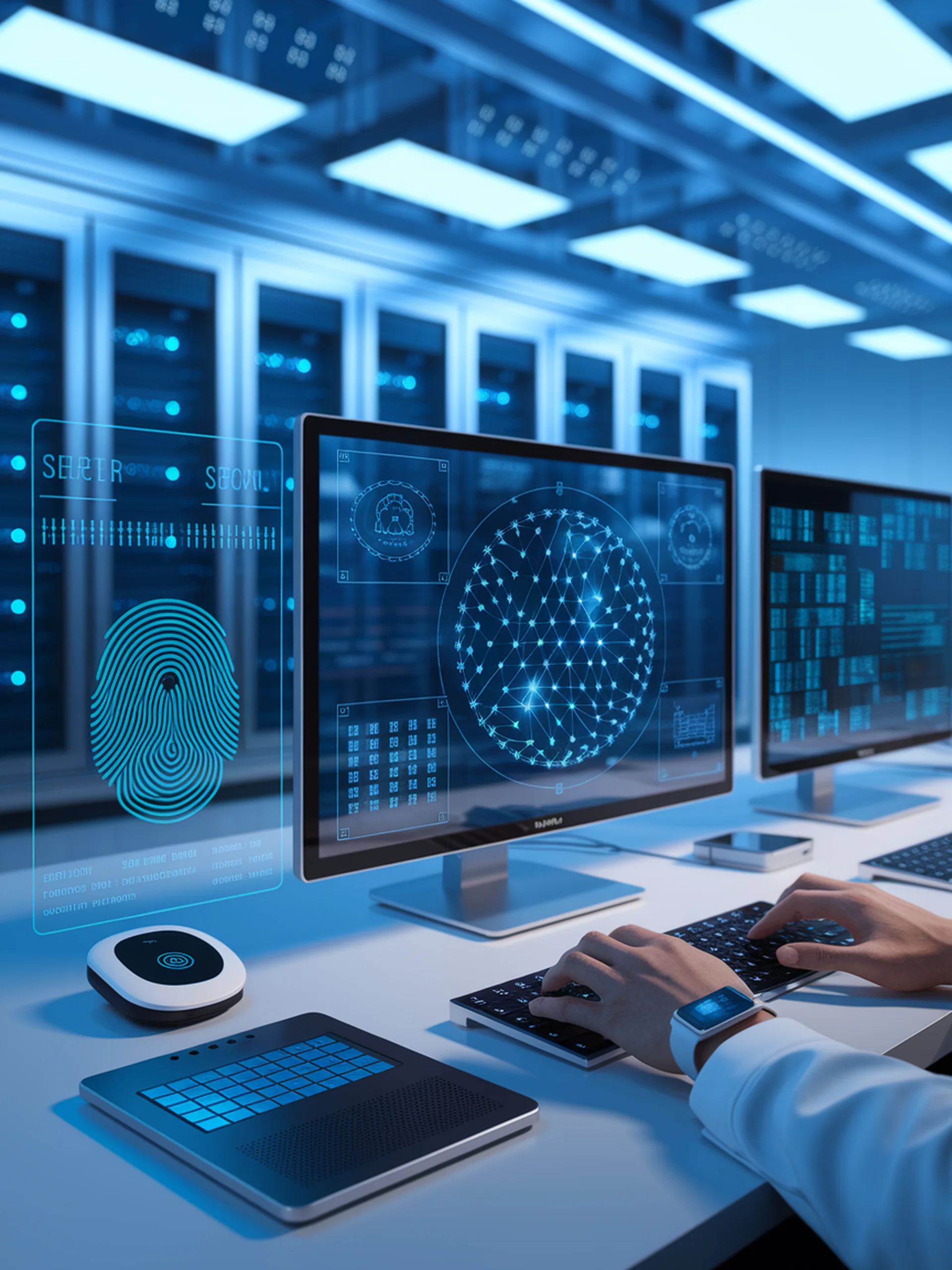
Privacy-First Personalized AI
Evolutionary Model Merging for Secure LLM Customization
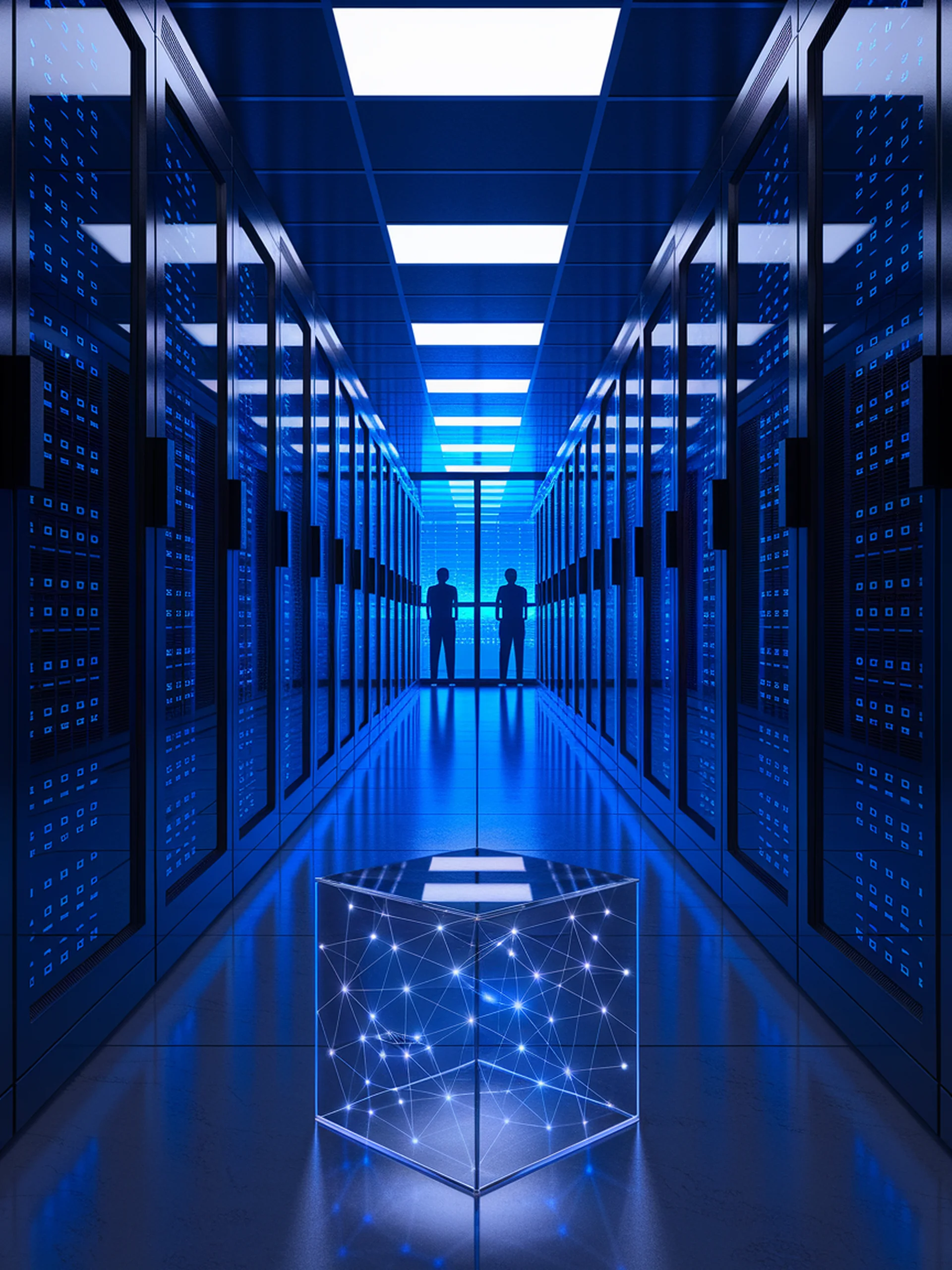
Privacy Vulnerabilities in AI Models
Understanding and Addressing Membership Inference Attacks
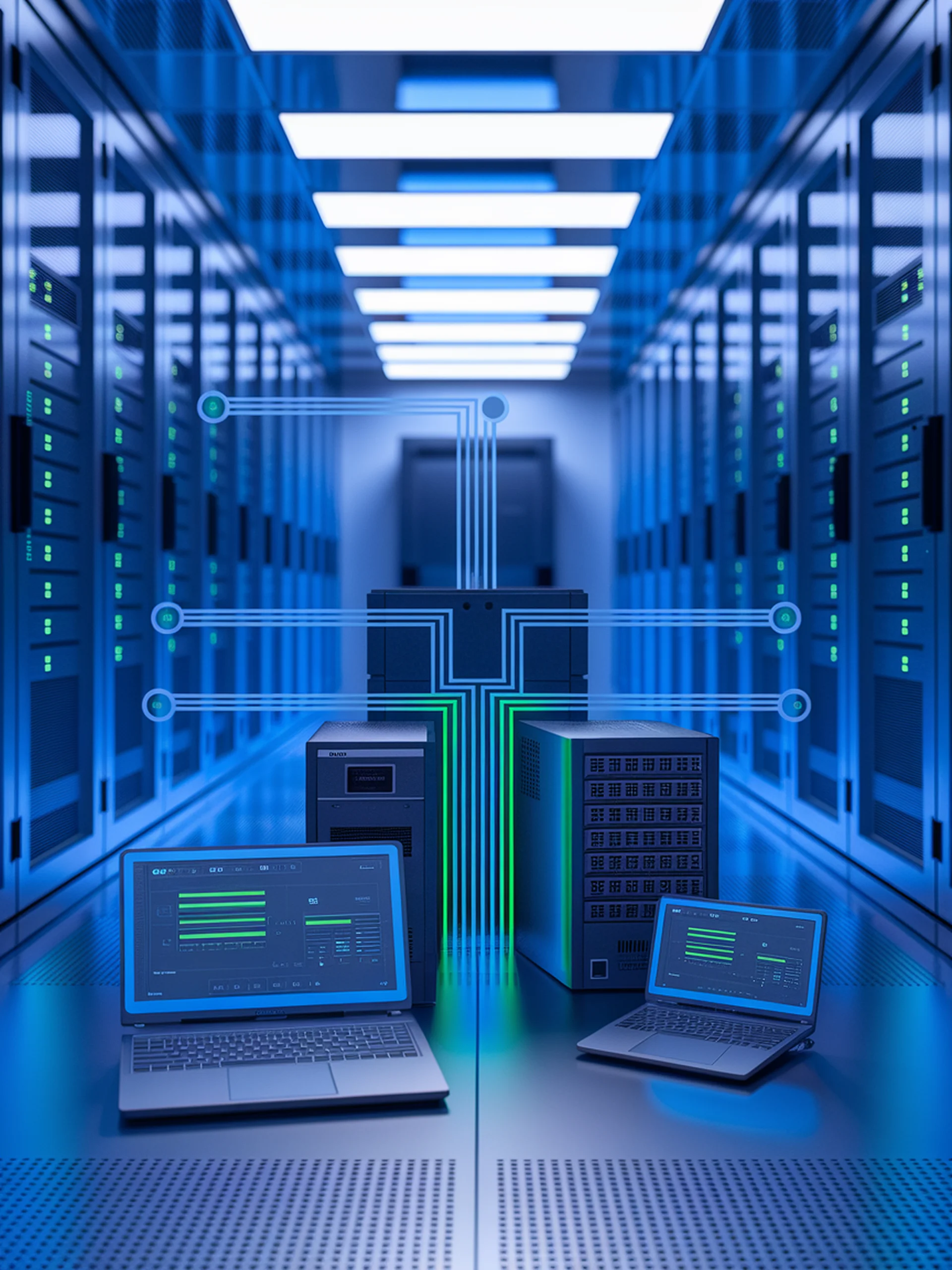
Efficient Federated LLM Fine-Tuning
Solving resource constraints and data heterogeneity across devices
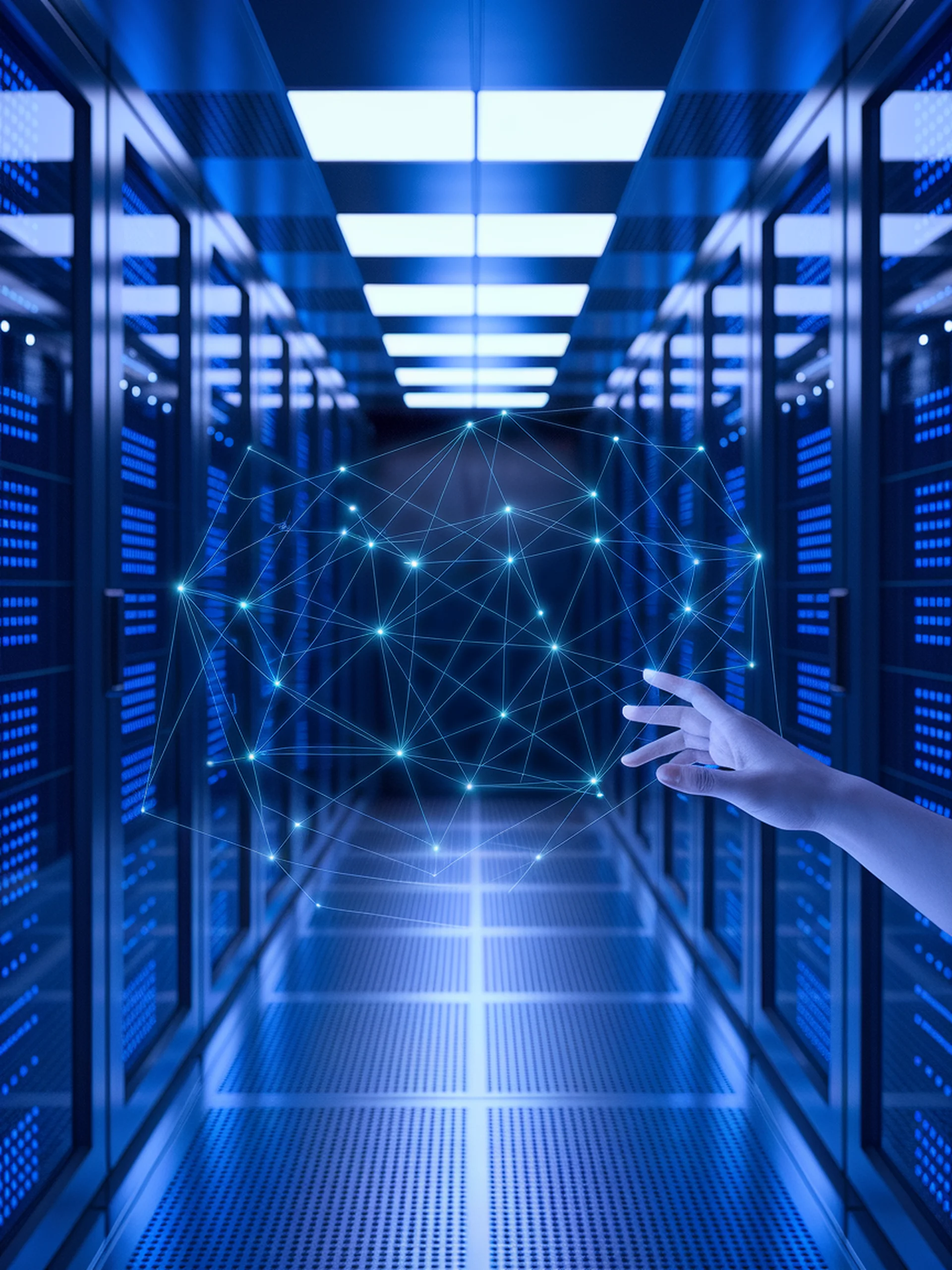
AI-Powered Data Enrichment Without Compromising Privacy
Exploring How LLMs Can Enhance Anonymized Data While Preserving Security
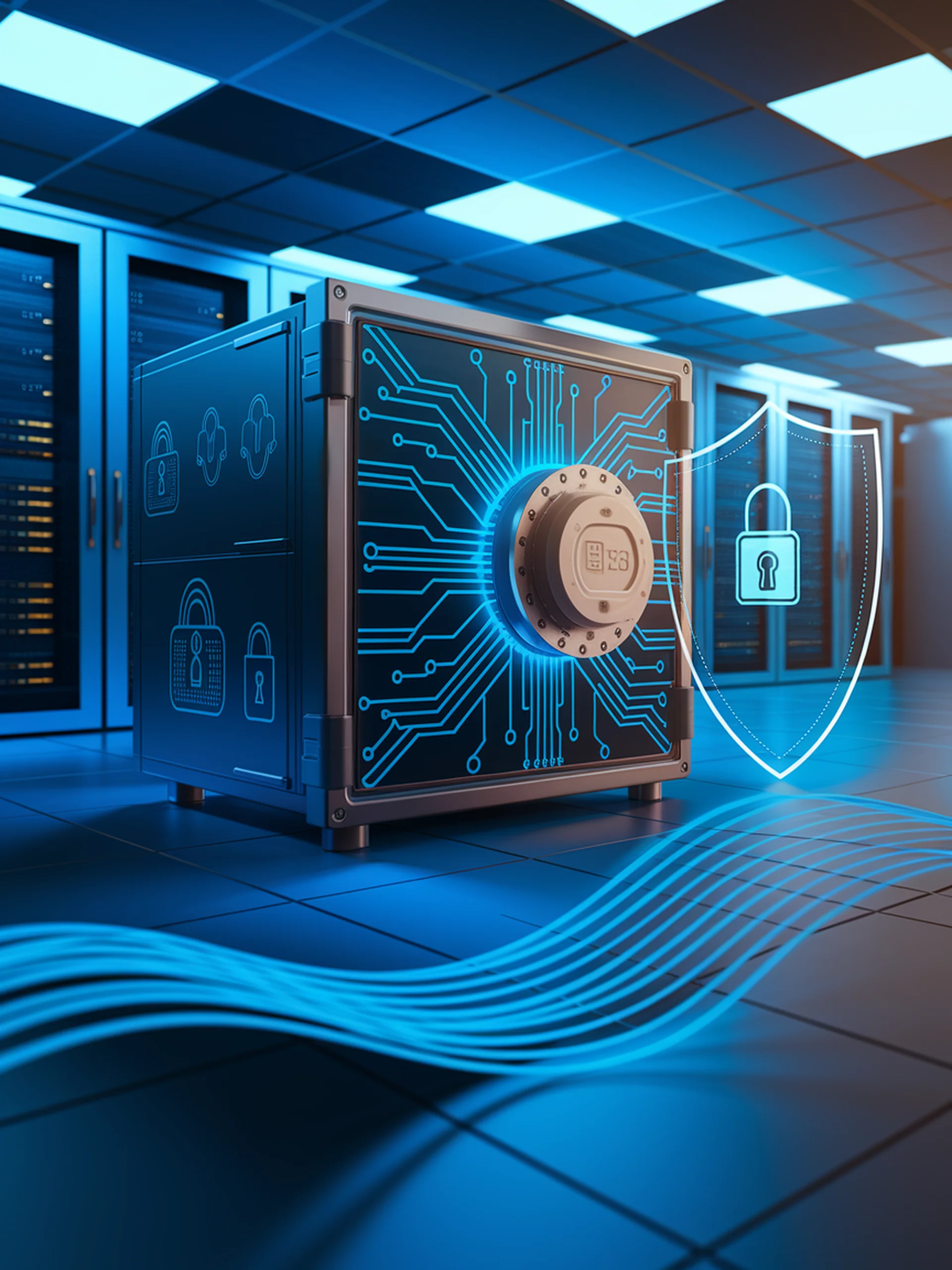
Securing LLM Interactions
A cryptographic approach to protecting sensitive information in prompts
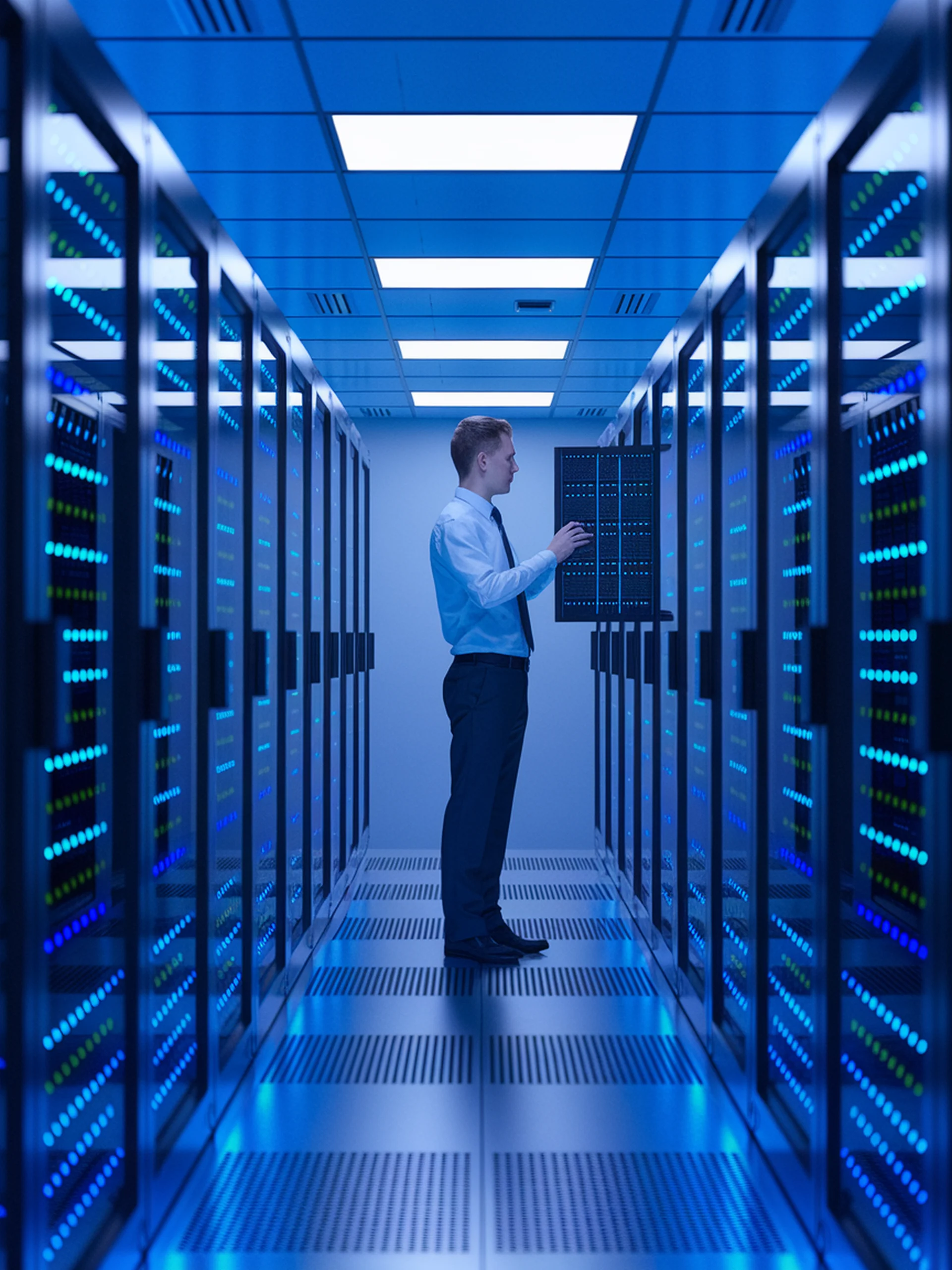
Securing LLMs: The On-Premise Migration Challenge
Moving from ChatGPT to controlled environments for enhanced data privacy
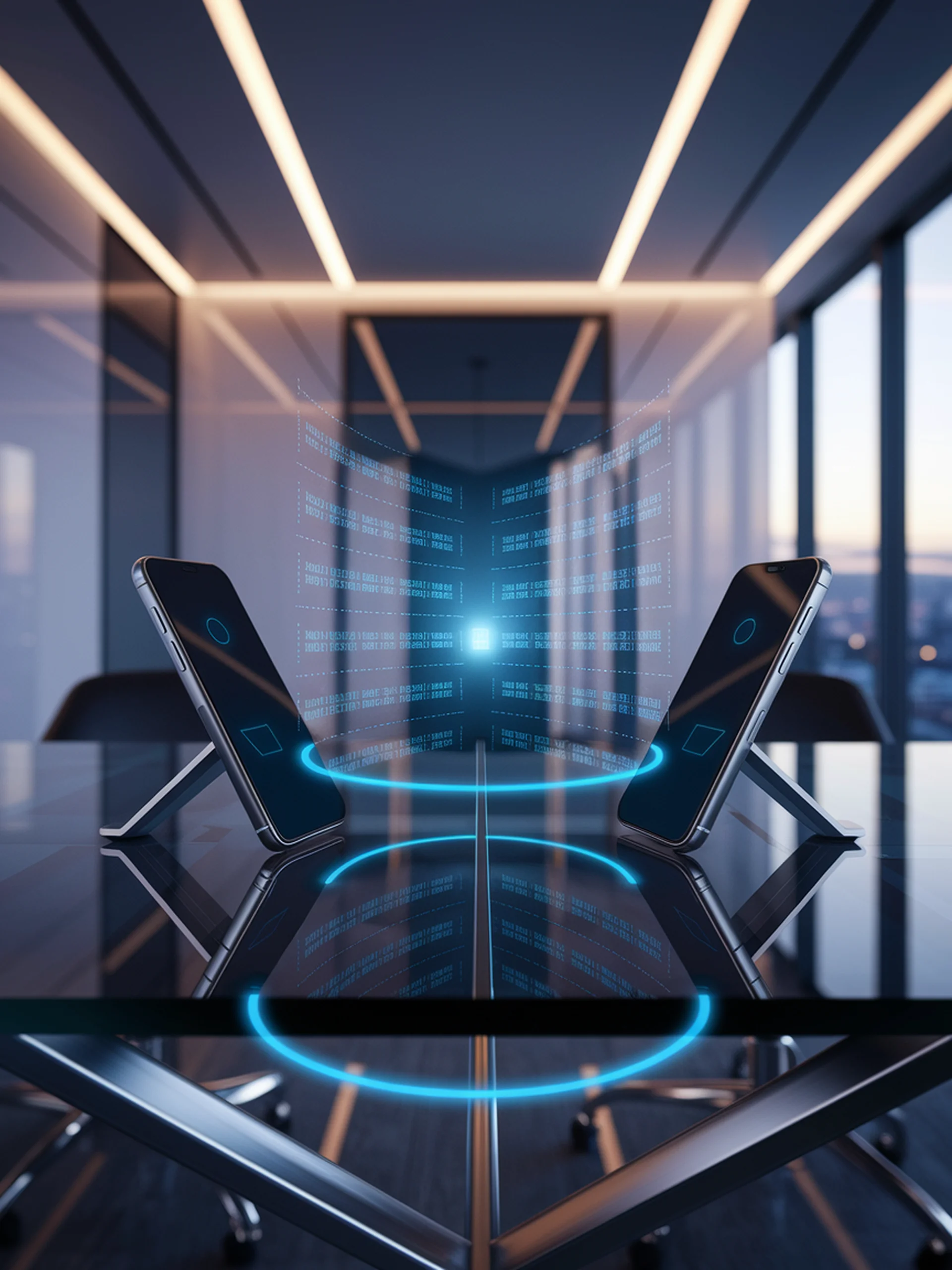
Secure Messaging Through LLMs
A novel framework for covert communication over public channels
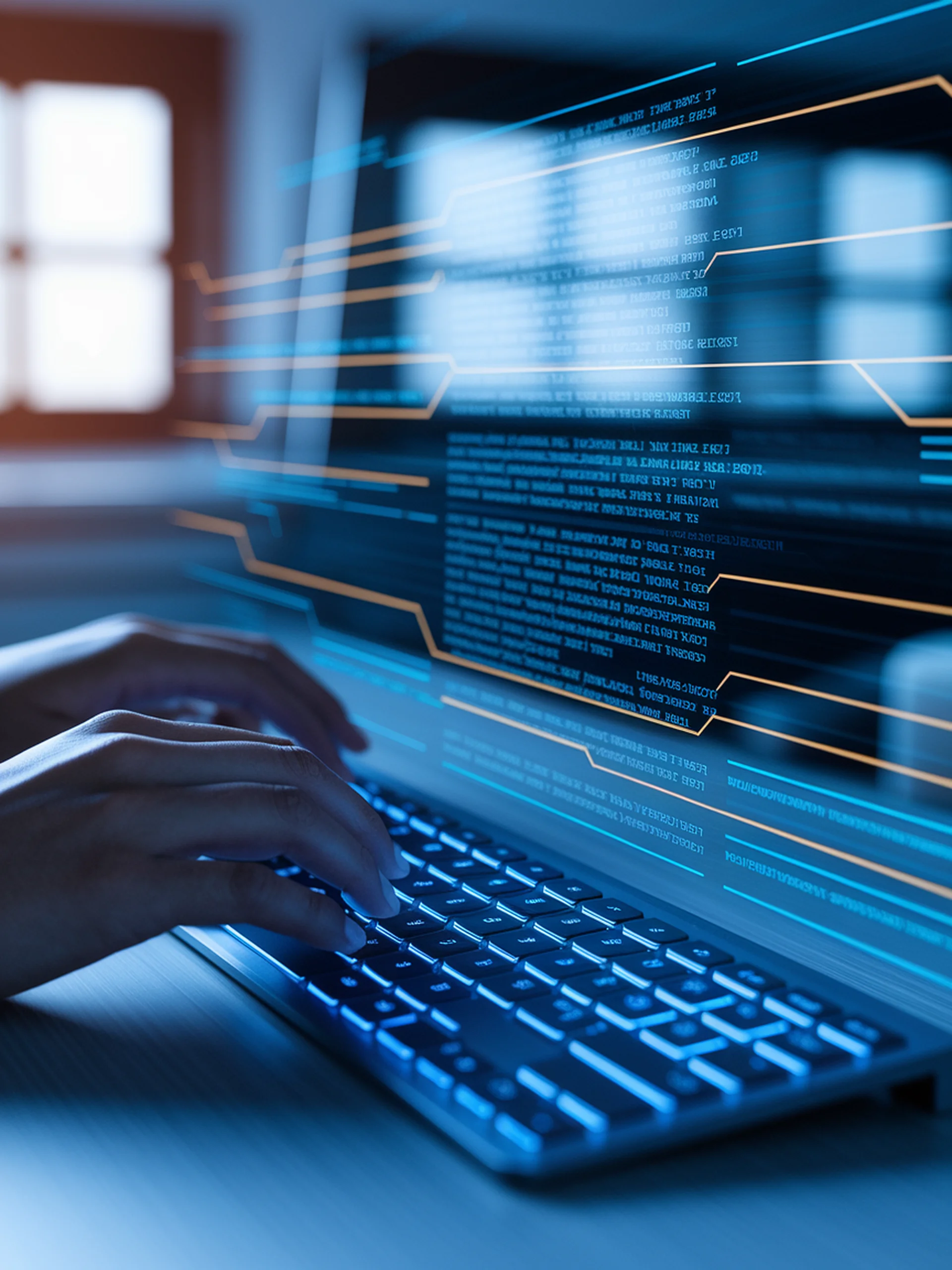
Fortifying Hidden Messages in LLM Text
Making steganography robust against disruption attacks
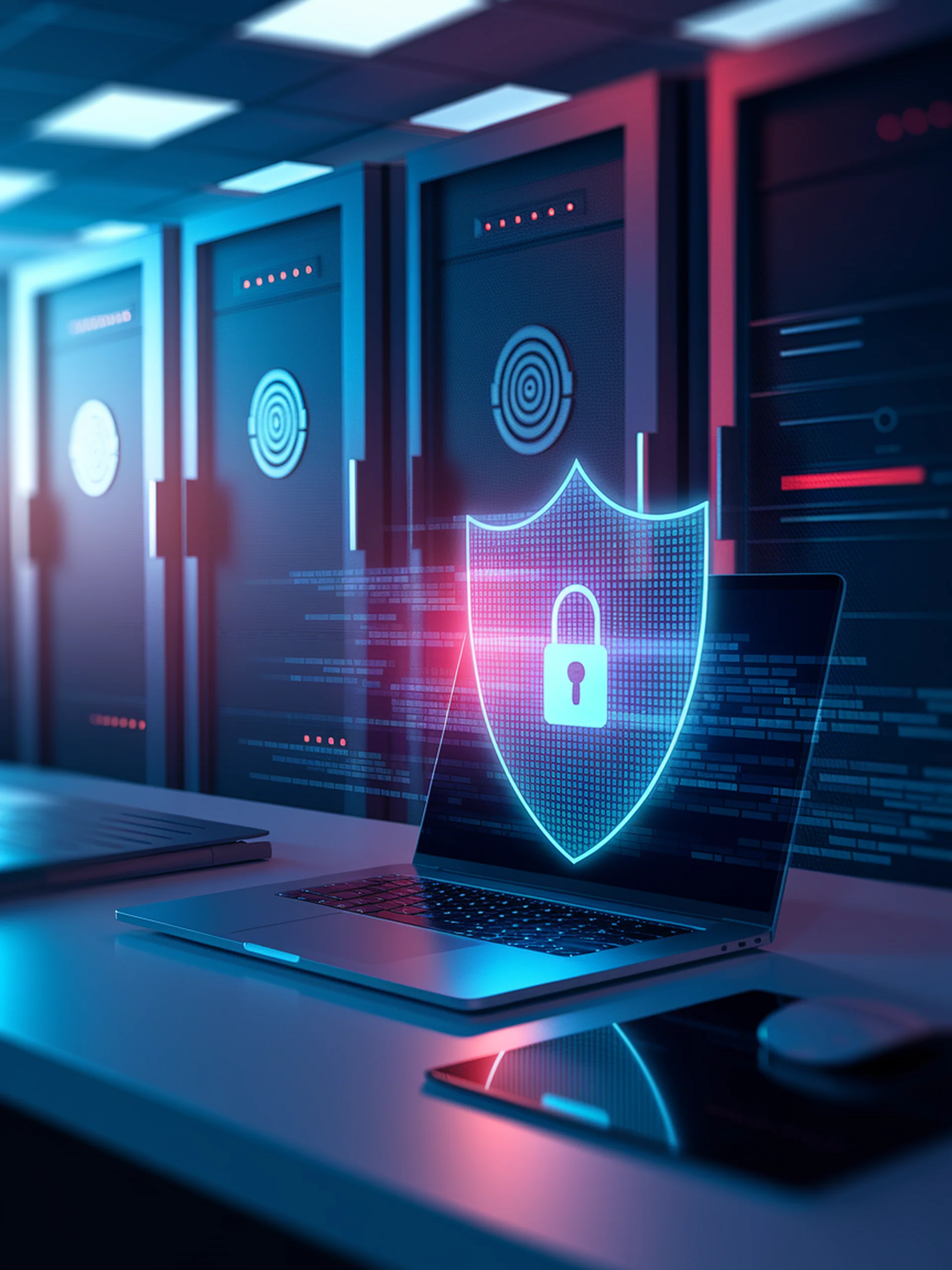
Safeguarding Privacy in AI Language Models
Novel approaches to protect personal data in LLM applications
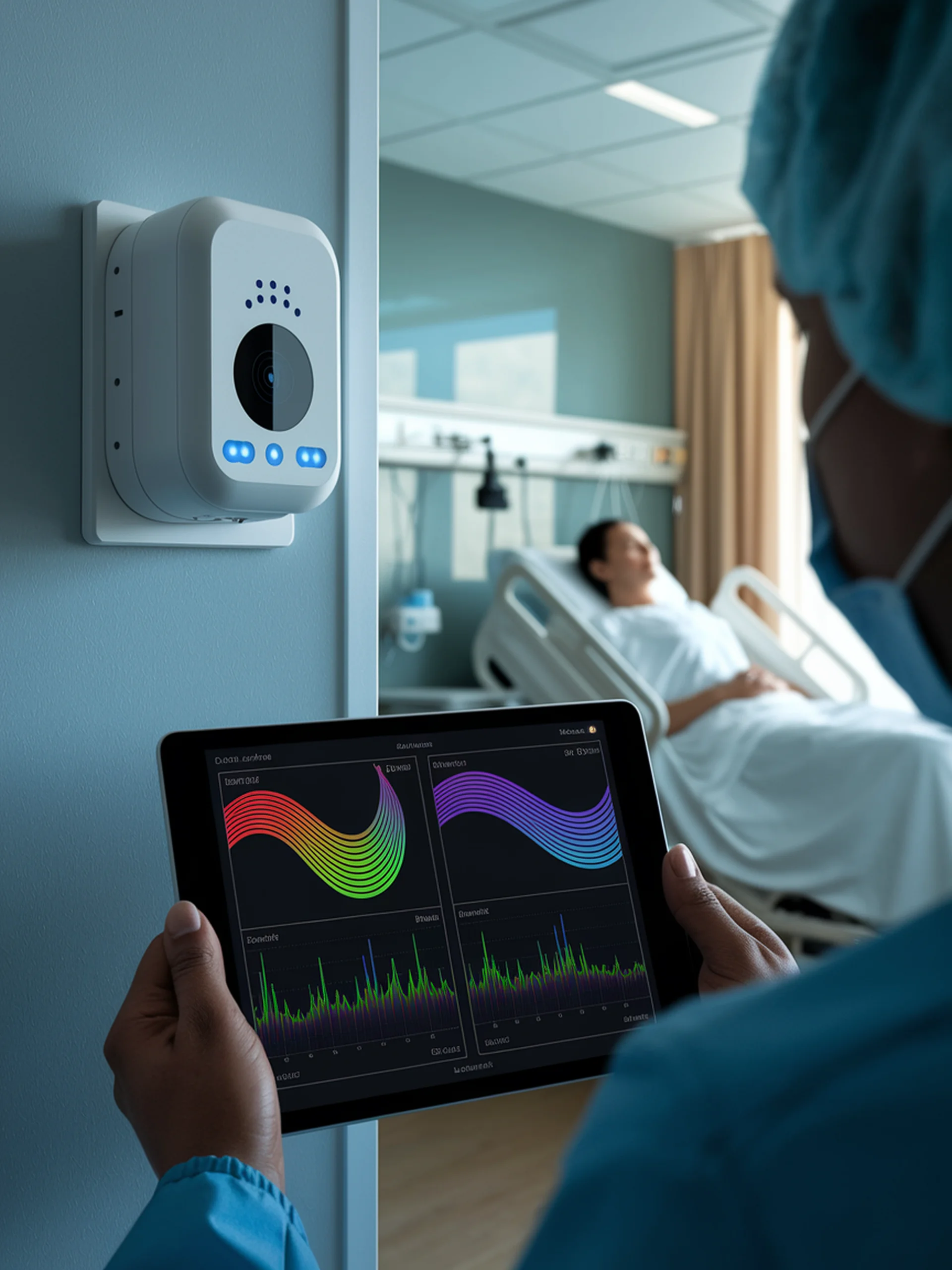
RadarLLM: Privacy-Preserving Motion Analysis
Leveraging LLMs to interpret human movement from radar data
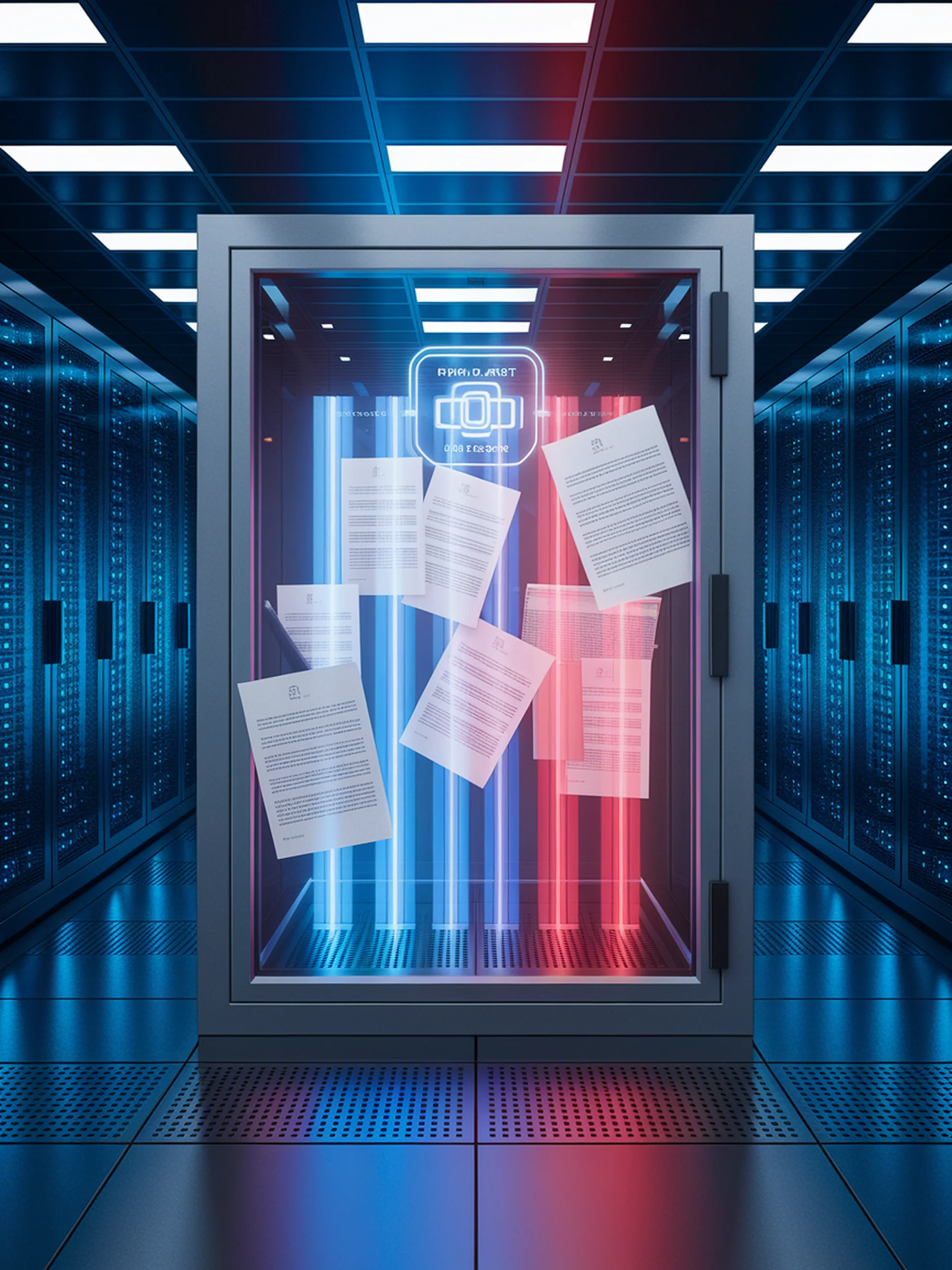
Securing RAG Systems Against Privacy Leaks
A novel approach to erasing private information while preserving utility
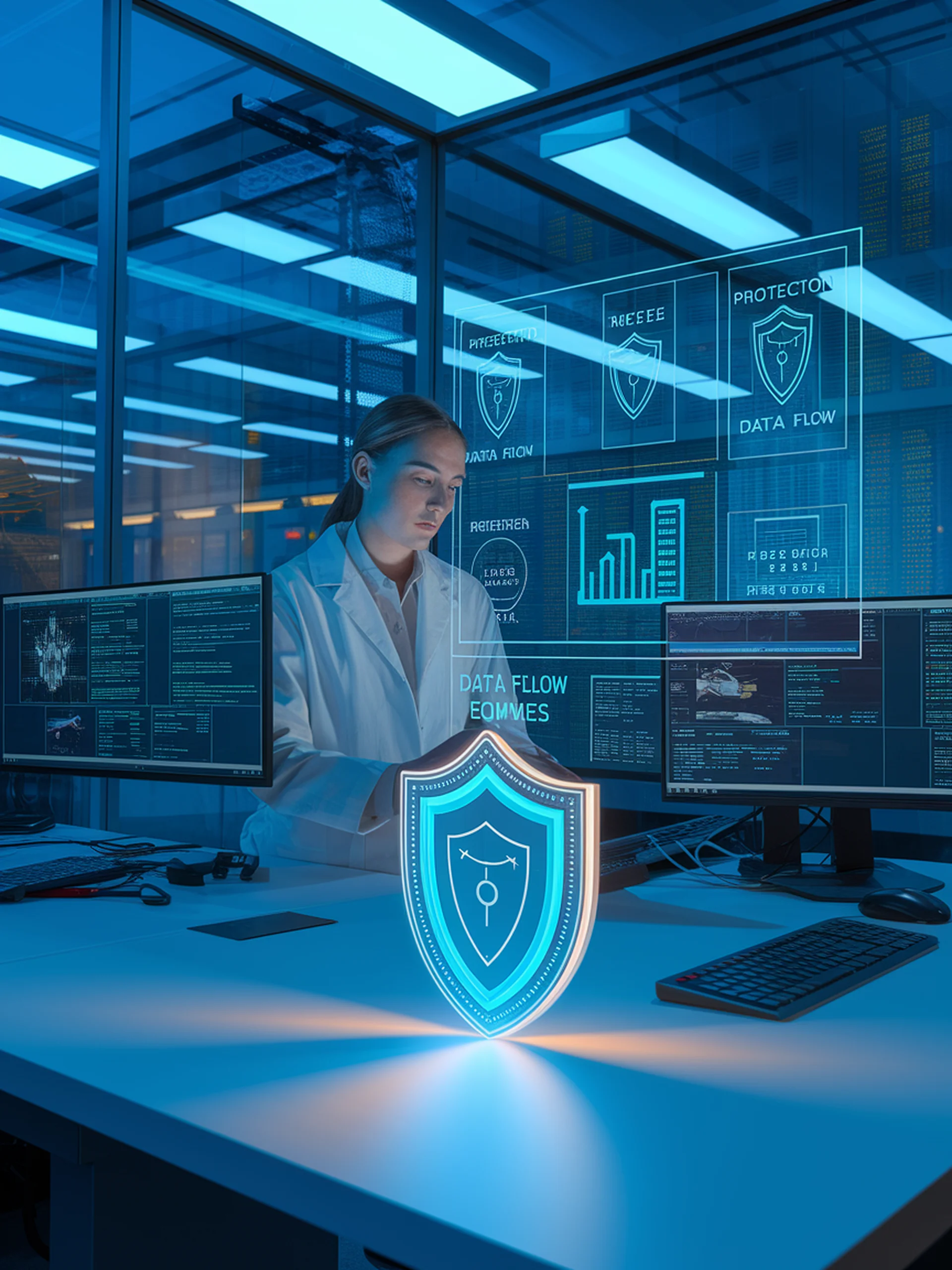
Protecting Confidential Data in LLM-Powered Science
DataShield: Managing privacy and transparency in AI-driven research
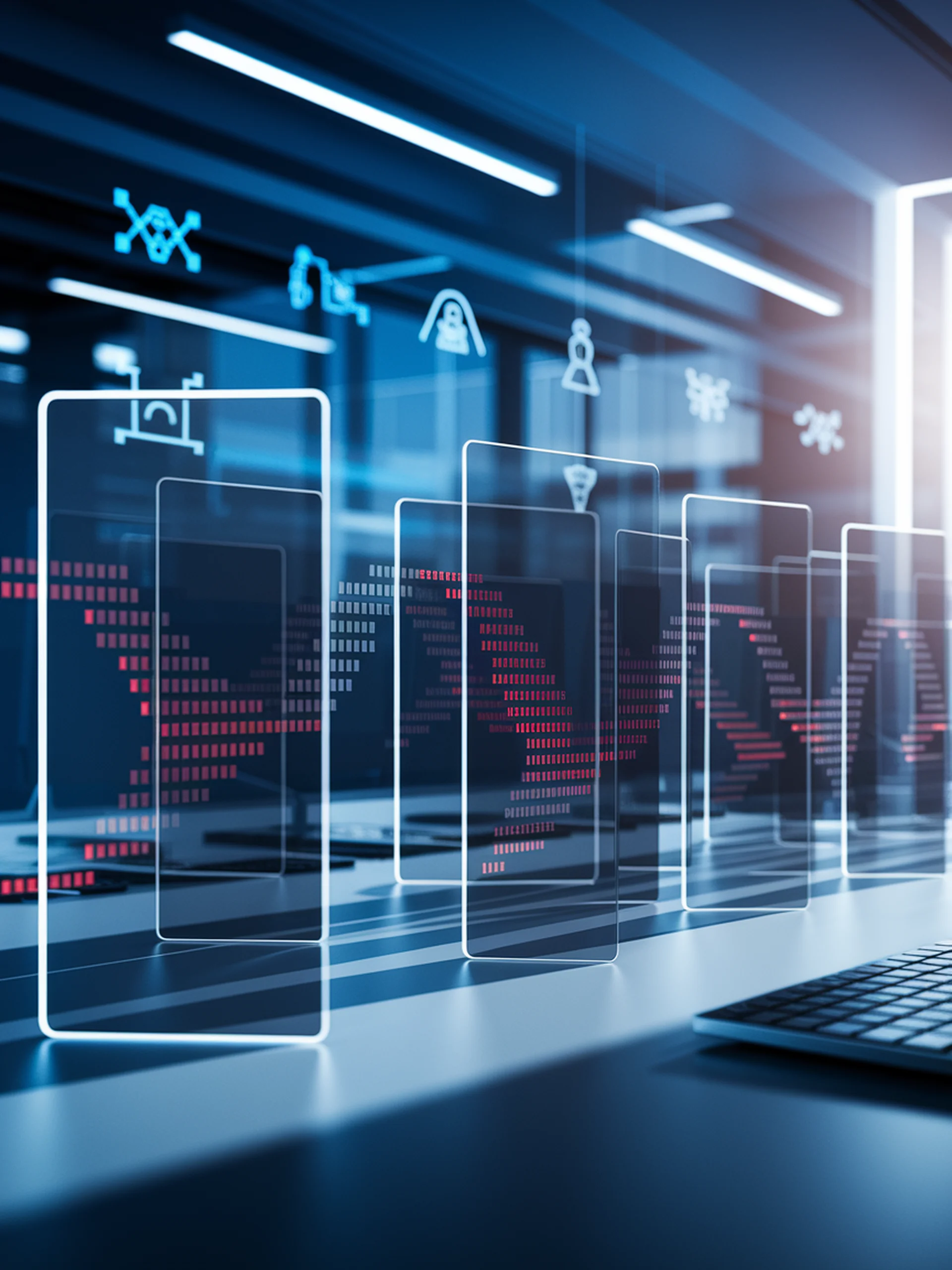
Redefining Privacy for AI Decision-Making
Why traditional privacy frameworks fail in the age of LLMs
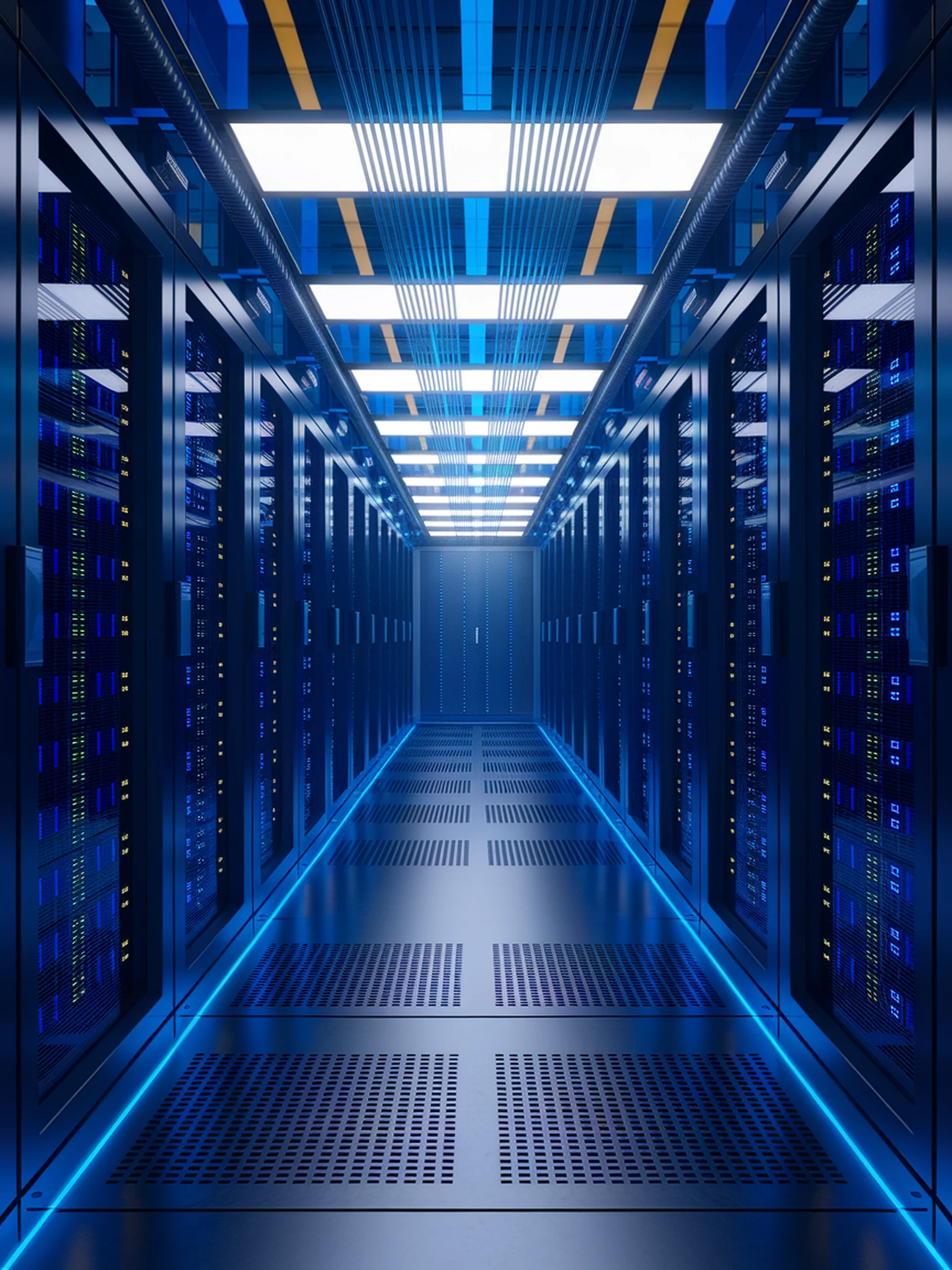