LLM Optimization and Efficiency
Techniques for improving LLM performance, reducing computational resources, and enhancing model deployment efficiency
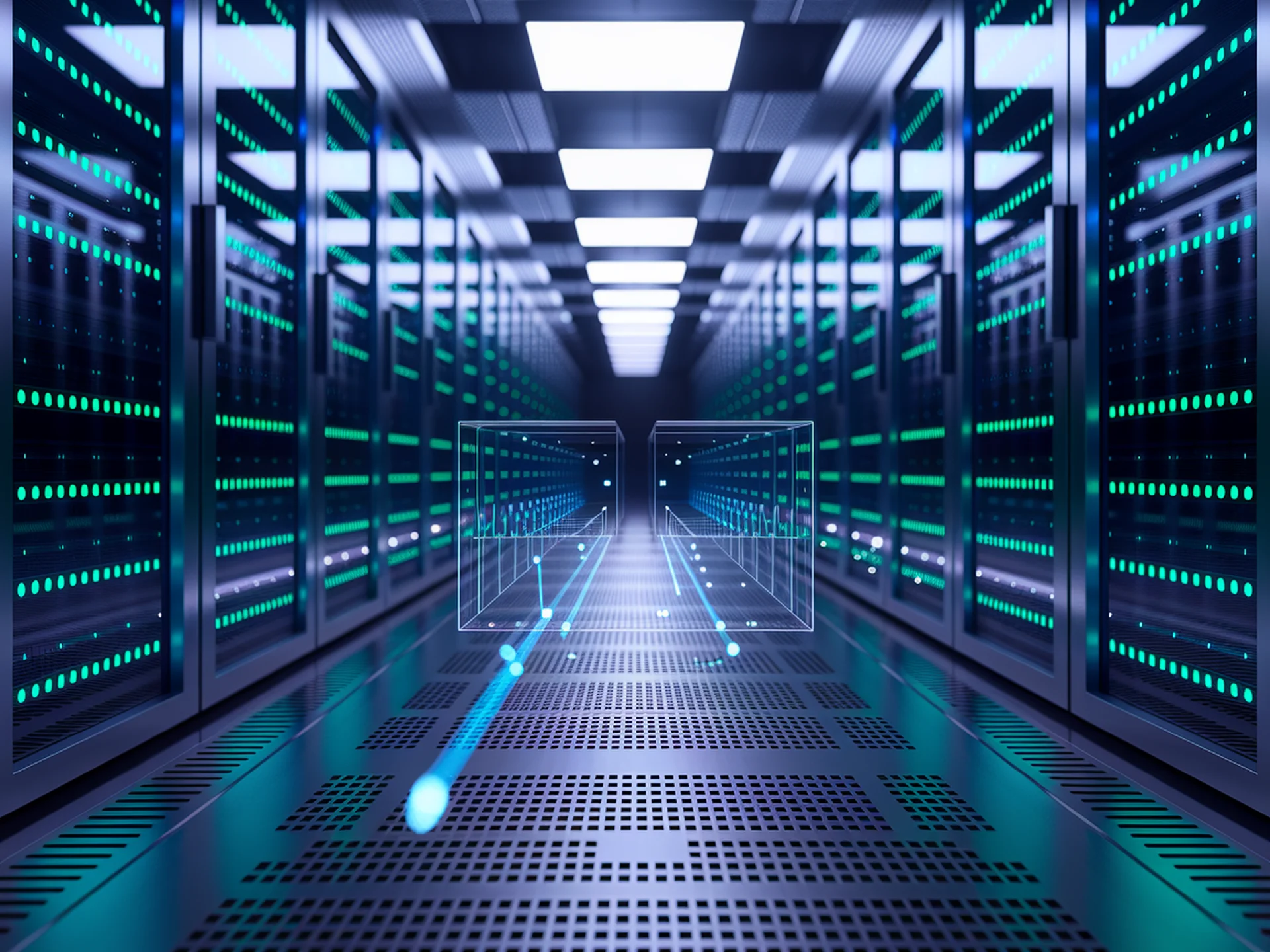
LLM Optimization and Efficiency
Research on Large Language Models in LLM Optimization and Efficiency
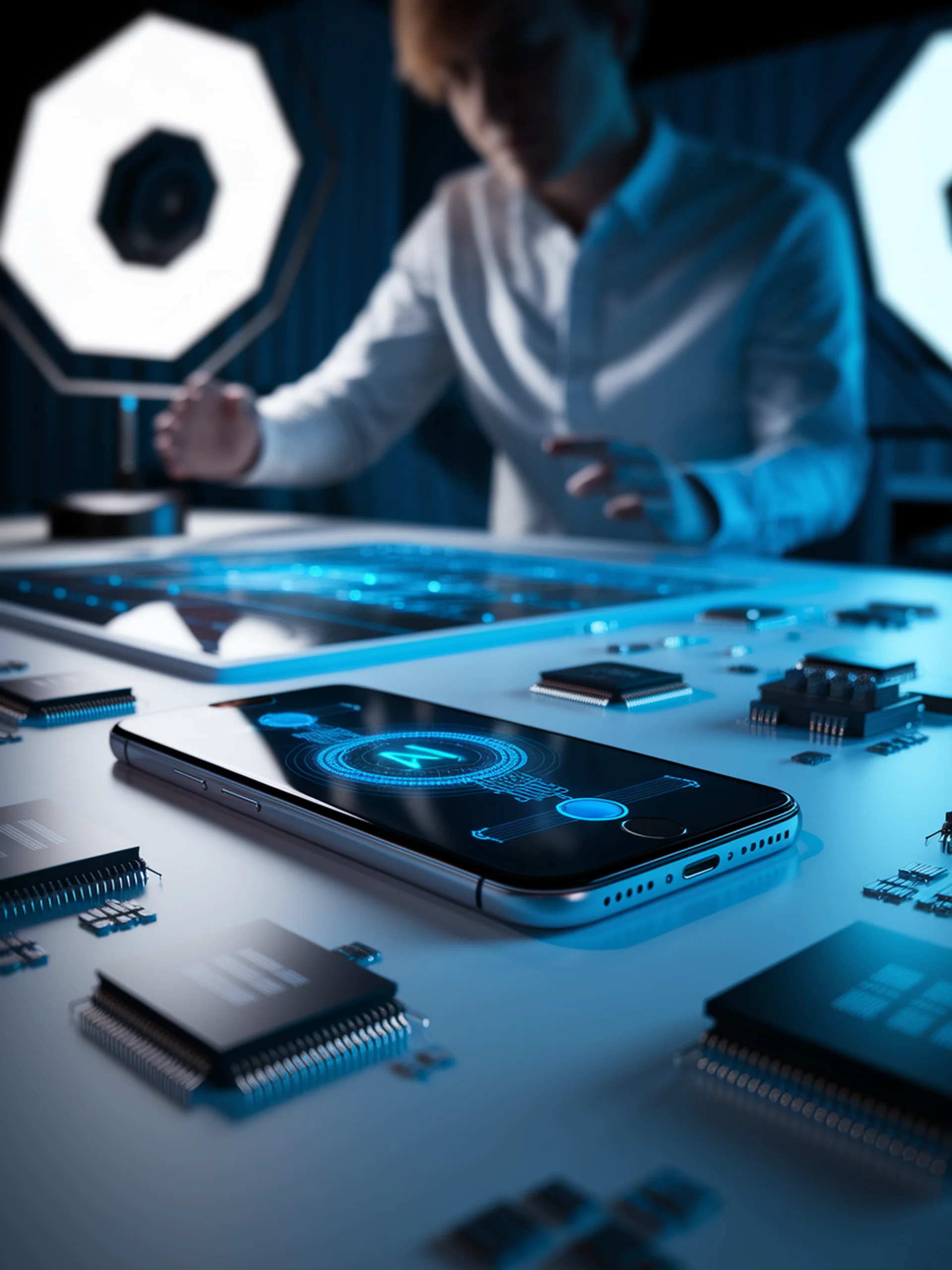
Bringing LLMs to Mobile Devices
EdgeMoE: An efficient inference engine for sparse LLMs
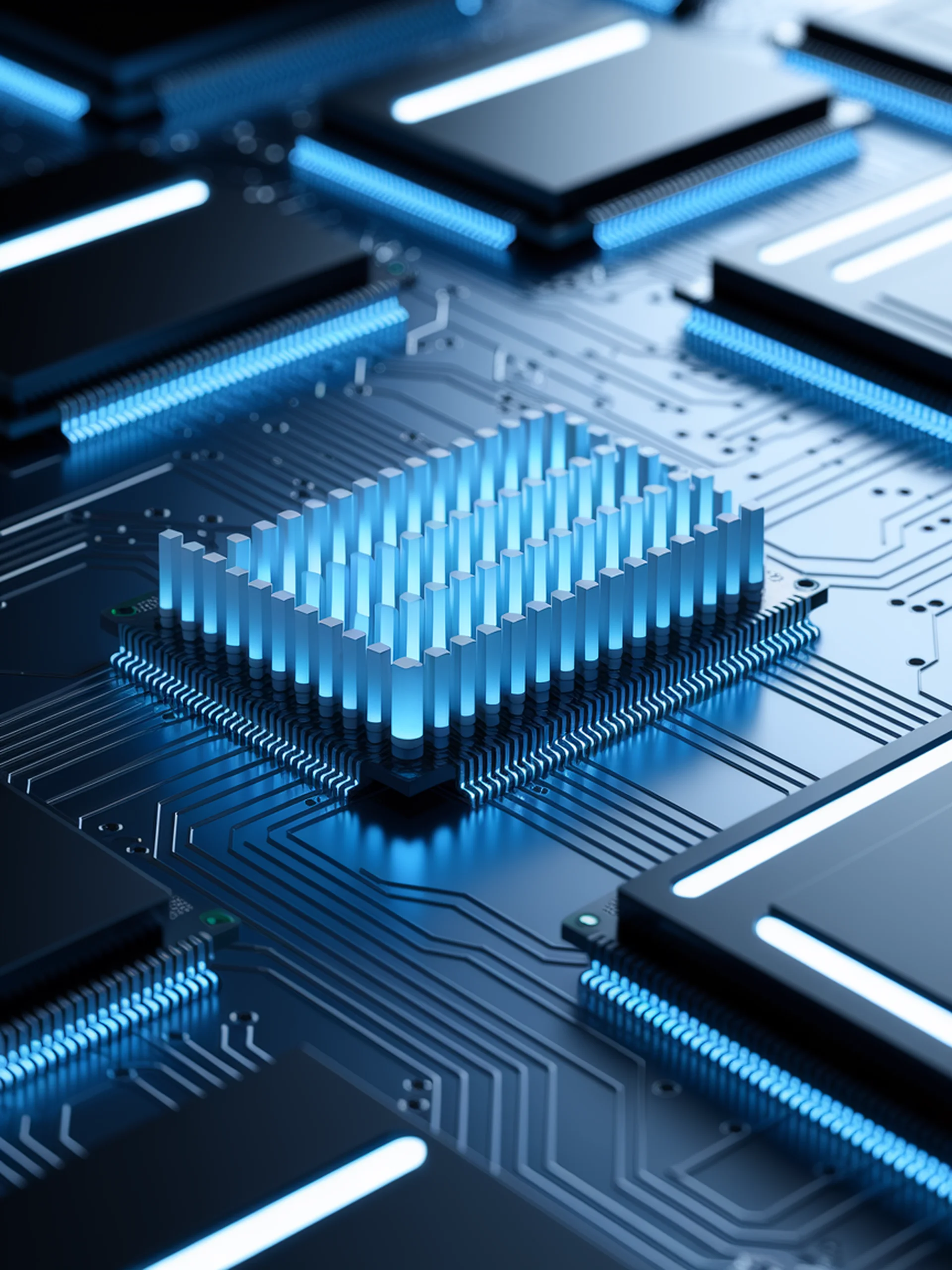
Smarter LLM Compression
Isolating Outliers for Efficient Low-bit Quantization
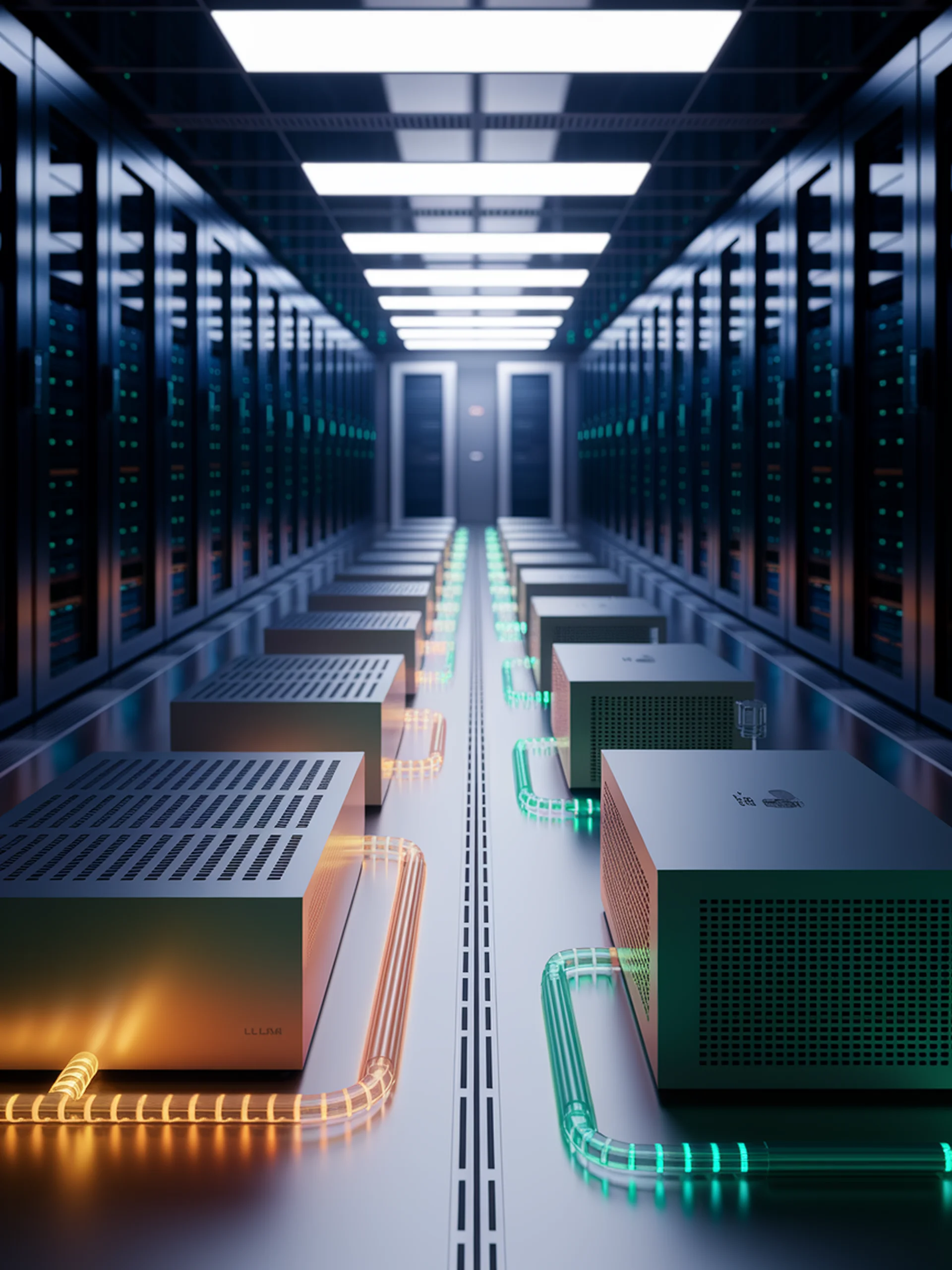
Chameleon: Next-Gen Infrastructure for RALMs
A heterogeneous accelerator system optimizing retrieval-augmented LLMs
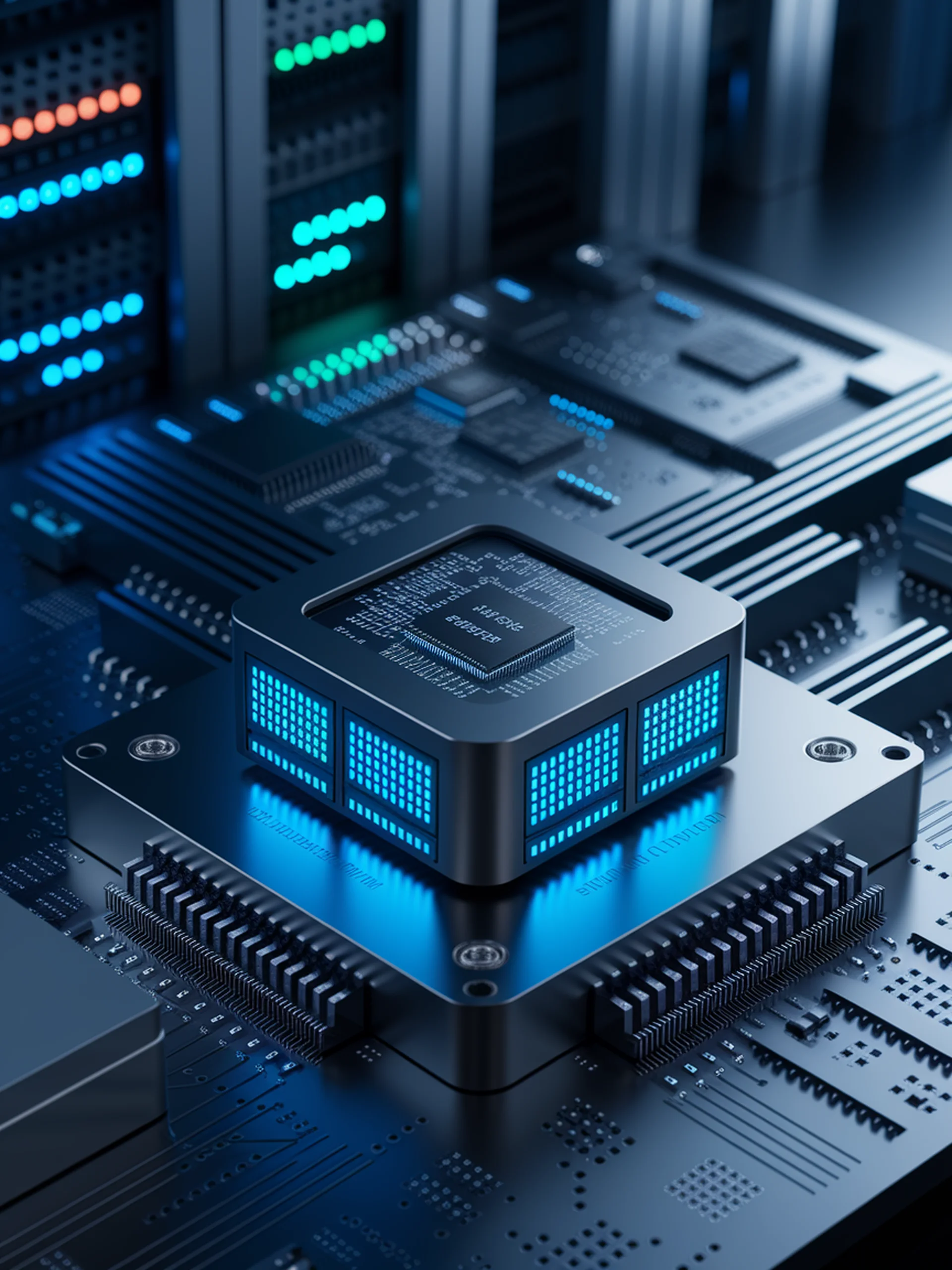
Next-Gen AI Hardware: The All-rounder Accelerator
Flexible ASIC design supporting multiple AI workloads simultaneously
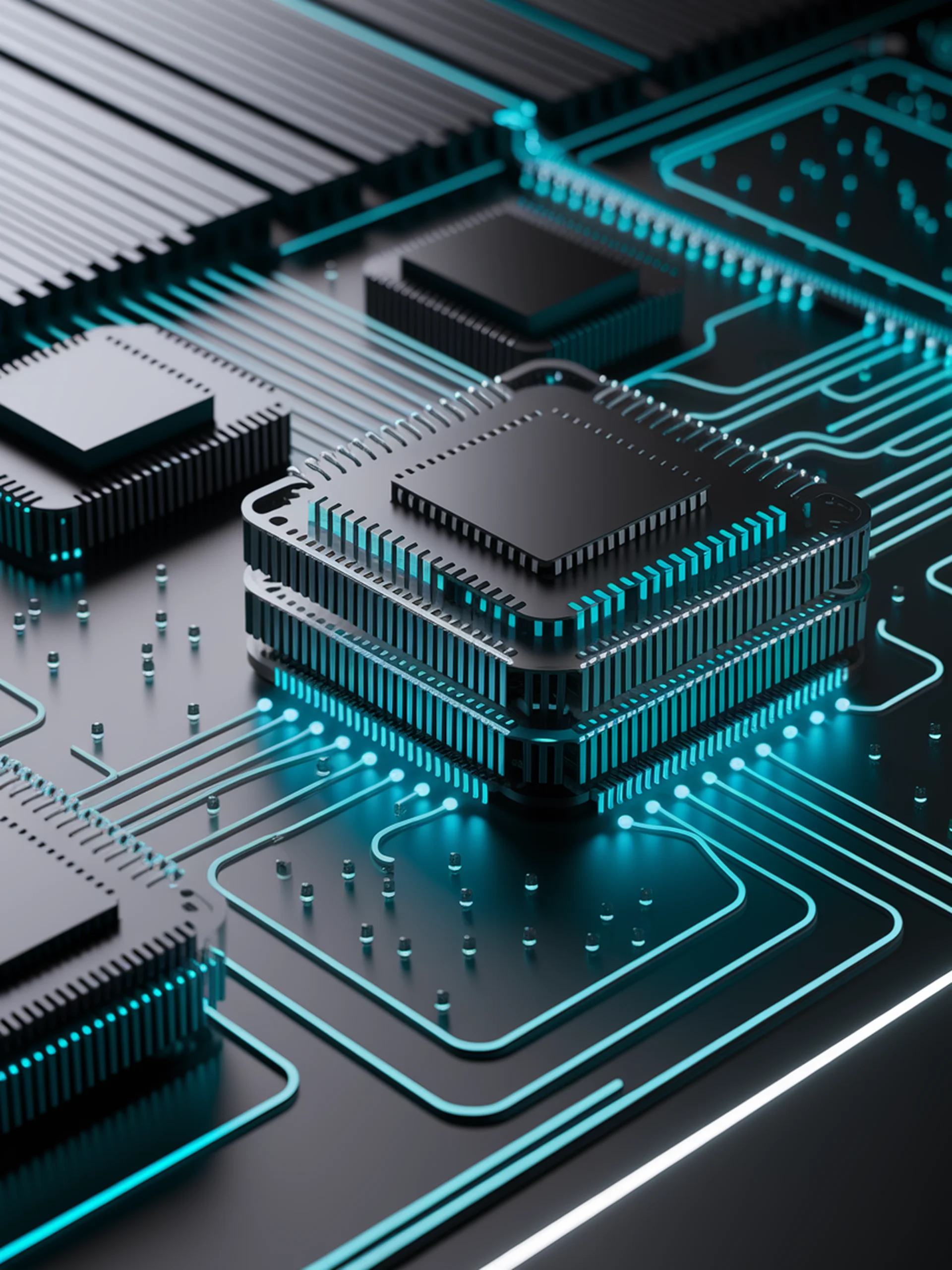
Optimizing Dynamic ML Systems
A Next-Generation Compiler Framework for LLMs
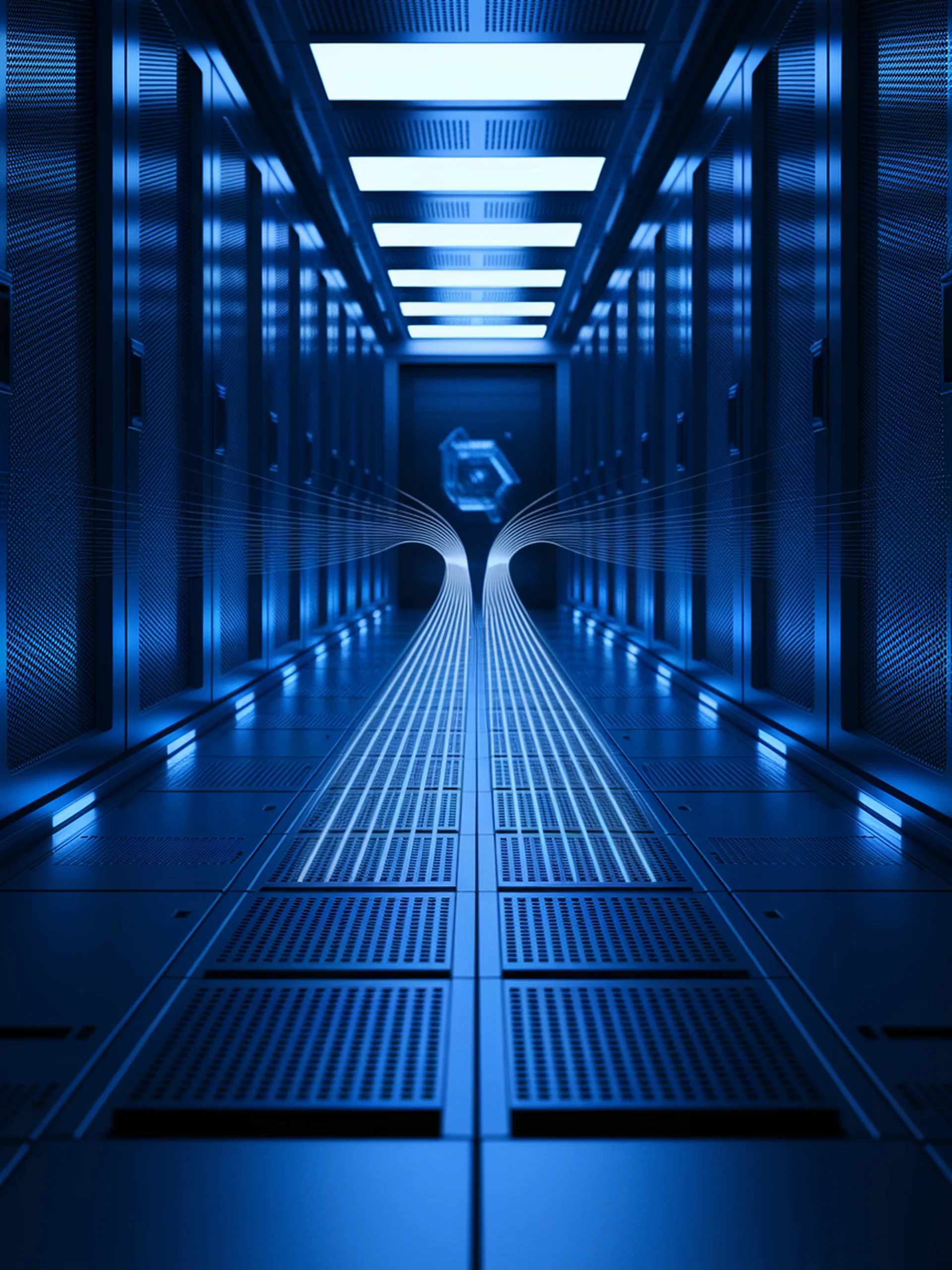
DeltaZip: Serving Multiple Fine-tuned LLMs Efficiently
10x compression with quality preservation for concurrent LLM deployment
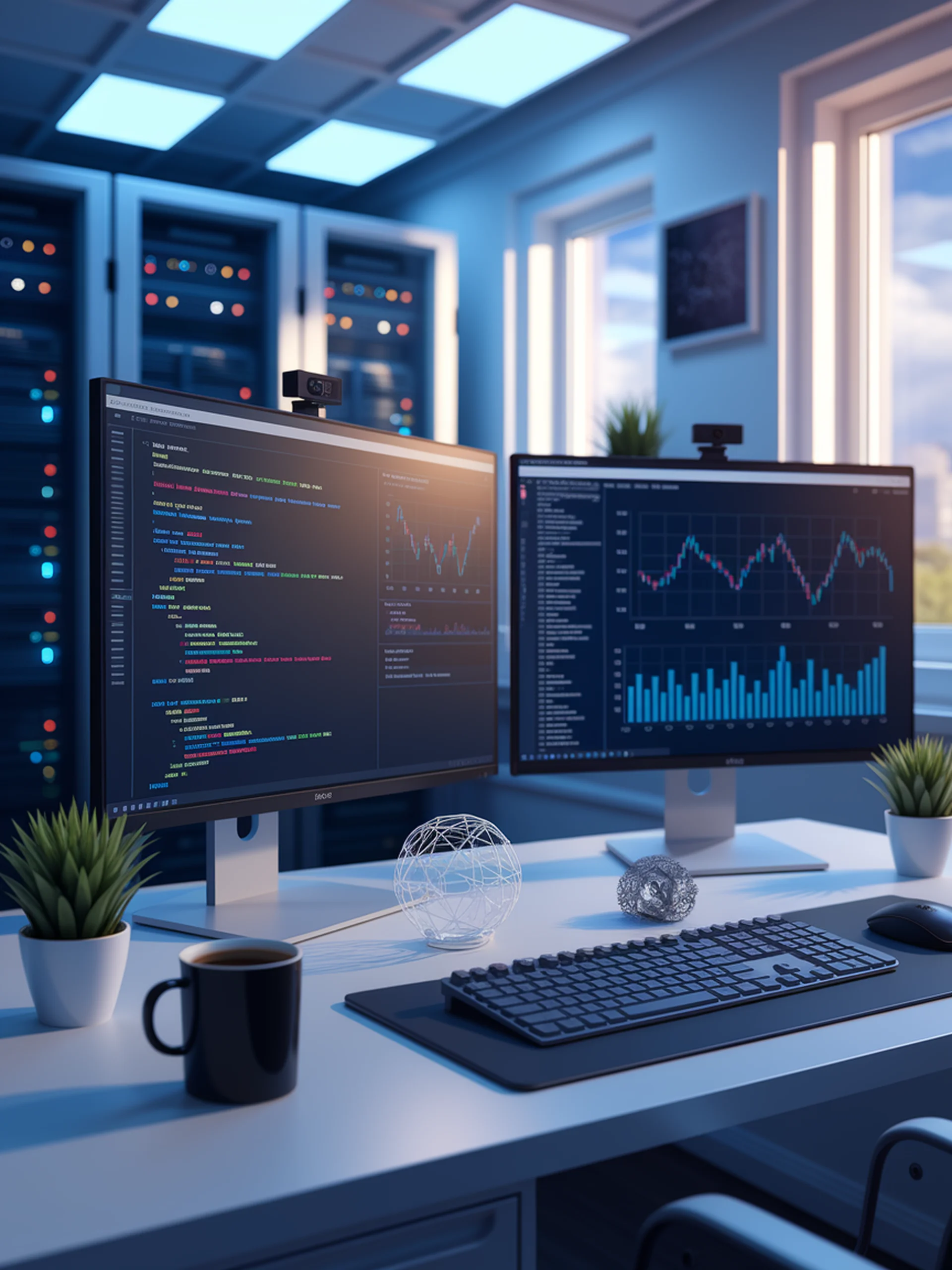
Optimizing Code with AI & Small Language Models
PerfRL - A Framework for Automated, Efficient Code Optimization
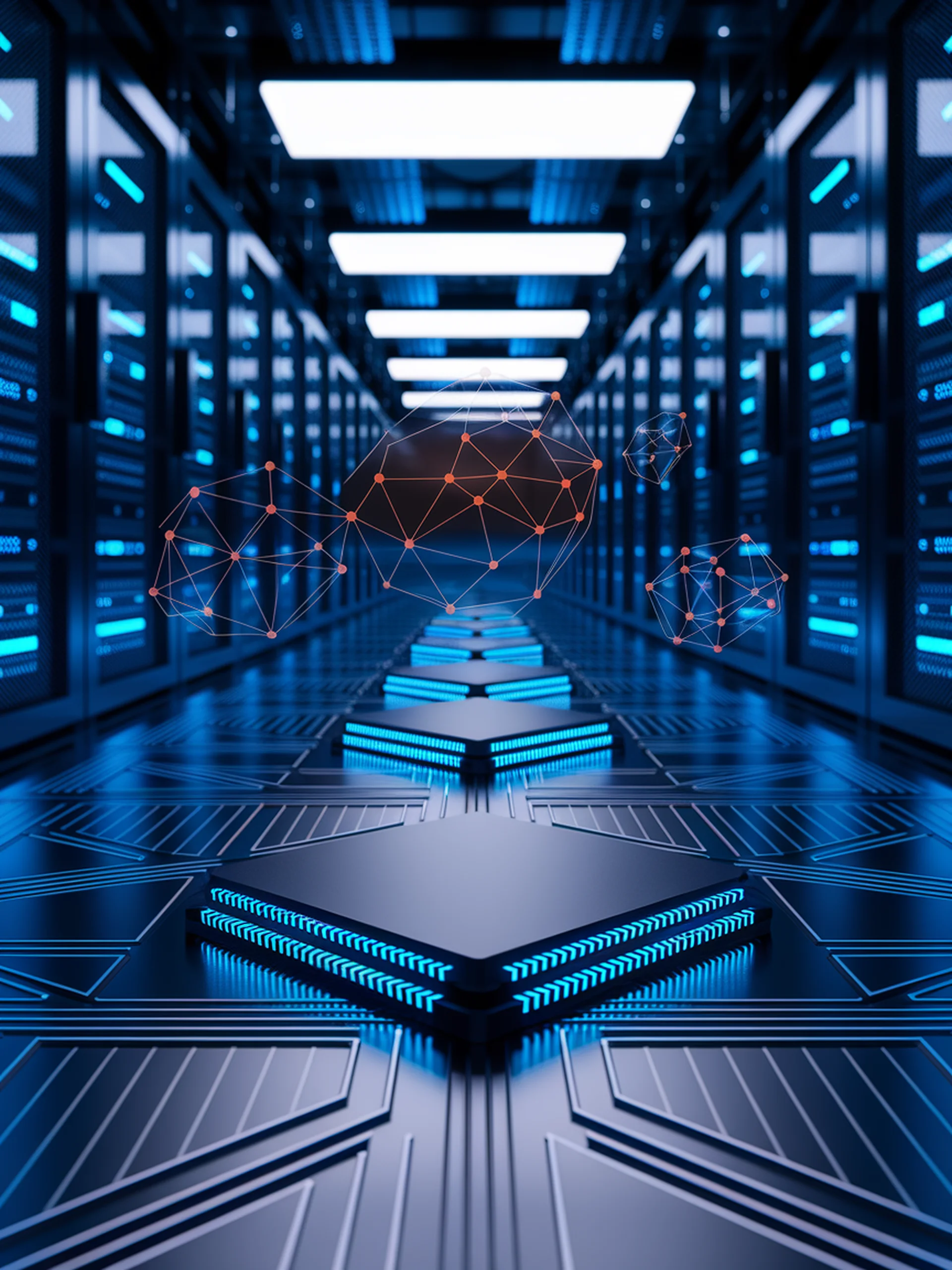
Smarter LLM Compression with CBQ
Leveraging cross-block dependencies for efficient model quantization
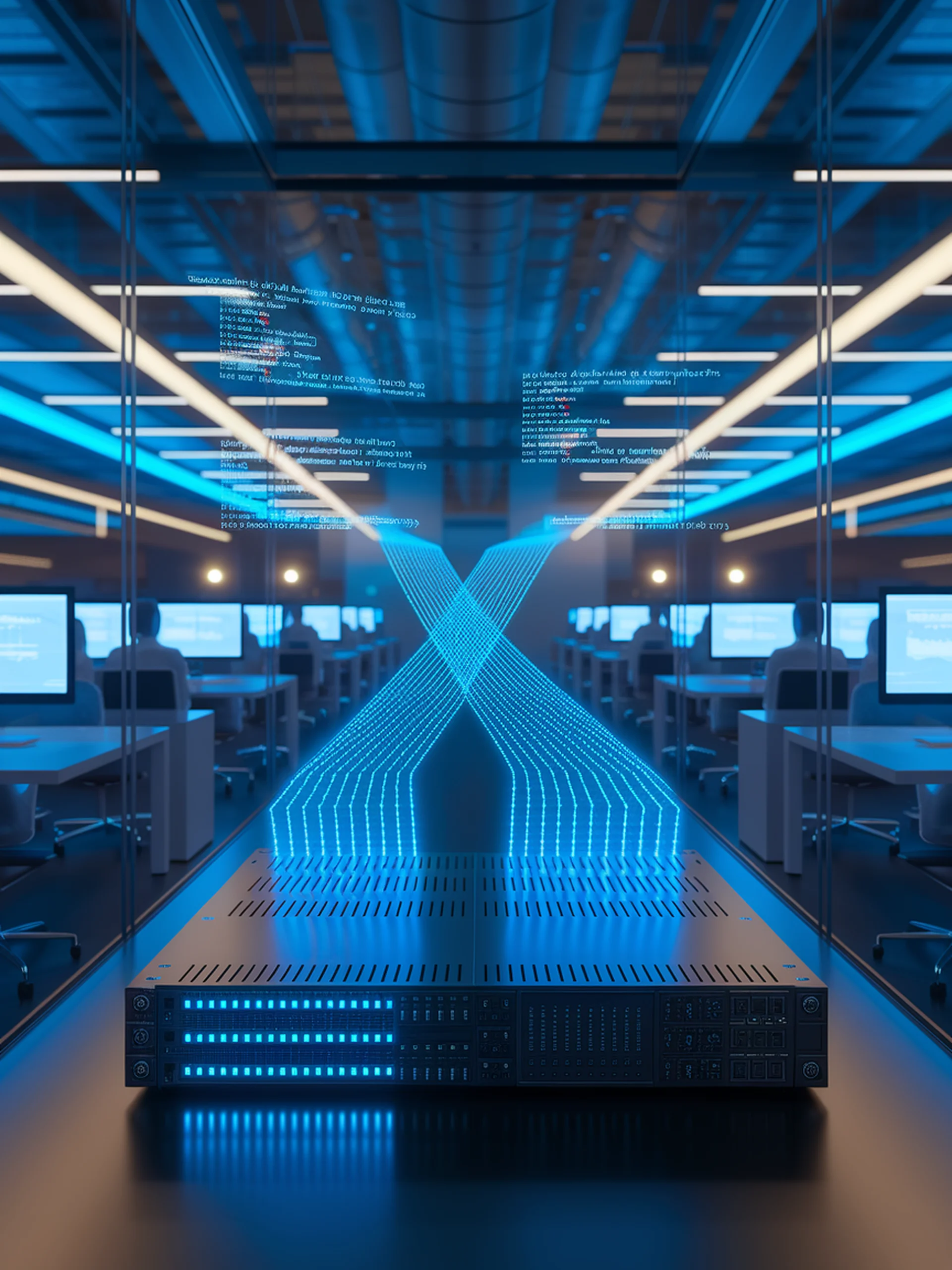
Teaching LLMs to Understand SQL Equivalence
Leveraging AI to solve a decades-old database challenge
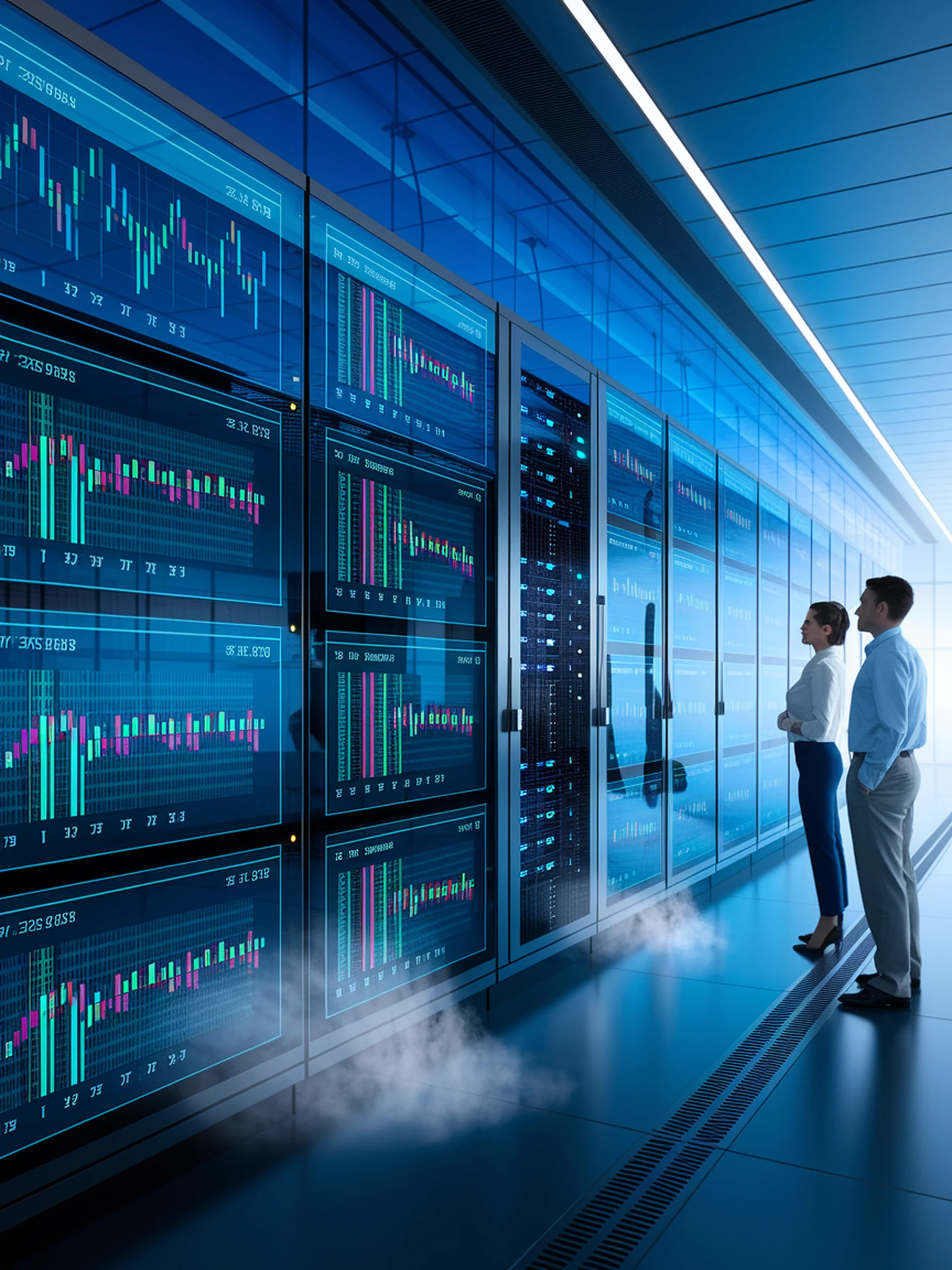
Optimizing LLM Inference Speed
Unlocking Efficient Speculative Decoding Techniques
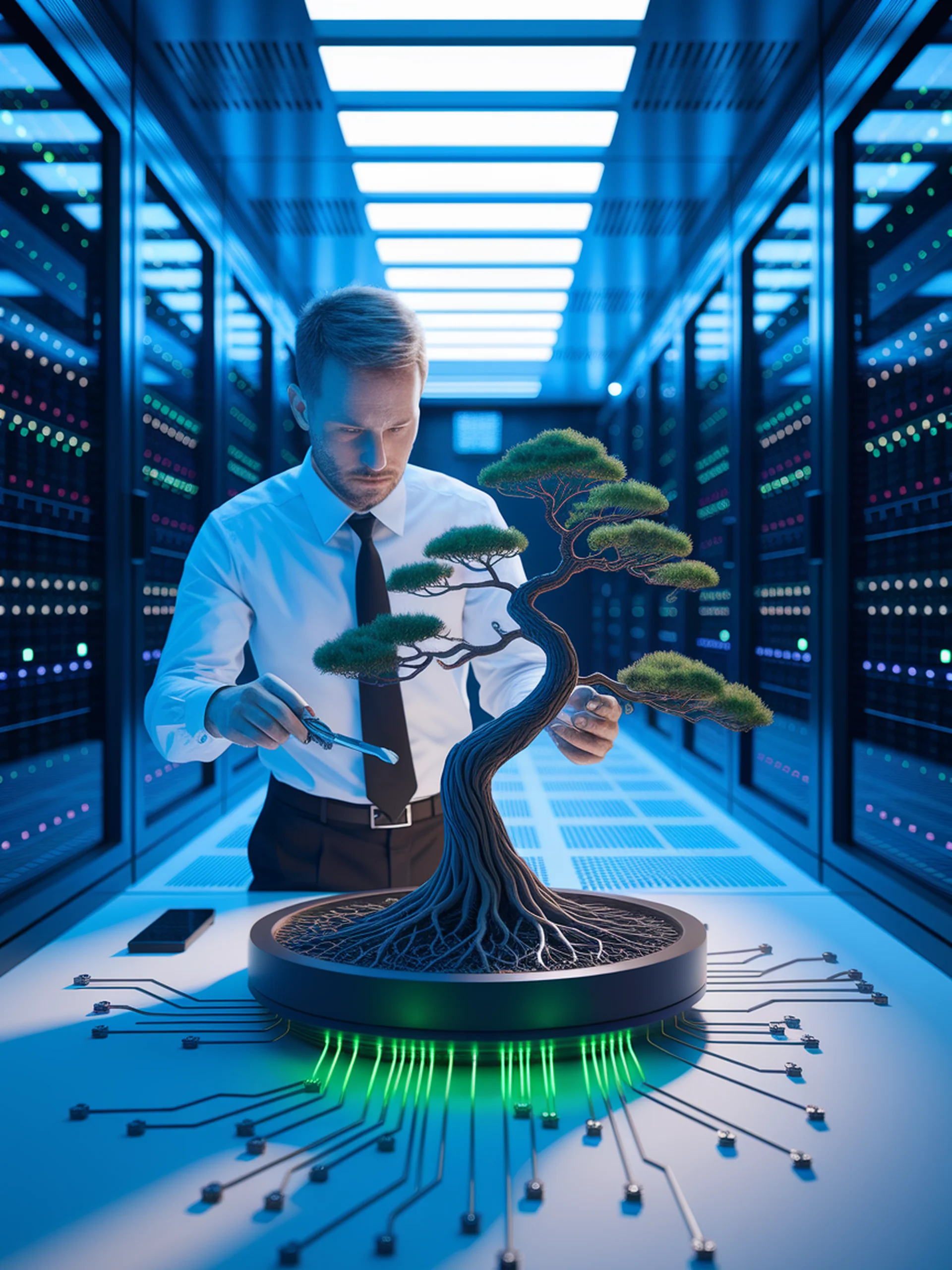
Revolutionizing LLM Pruning
Achieving Better Compression with Less Compute
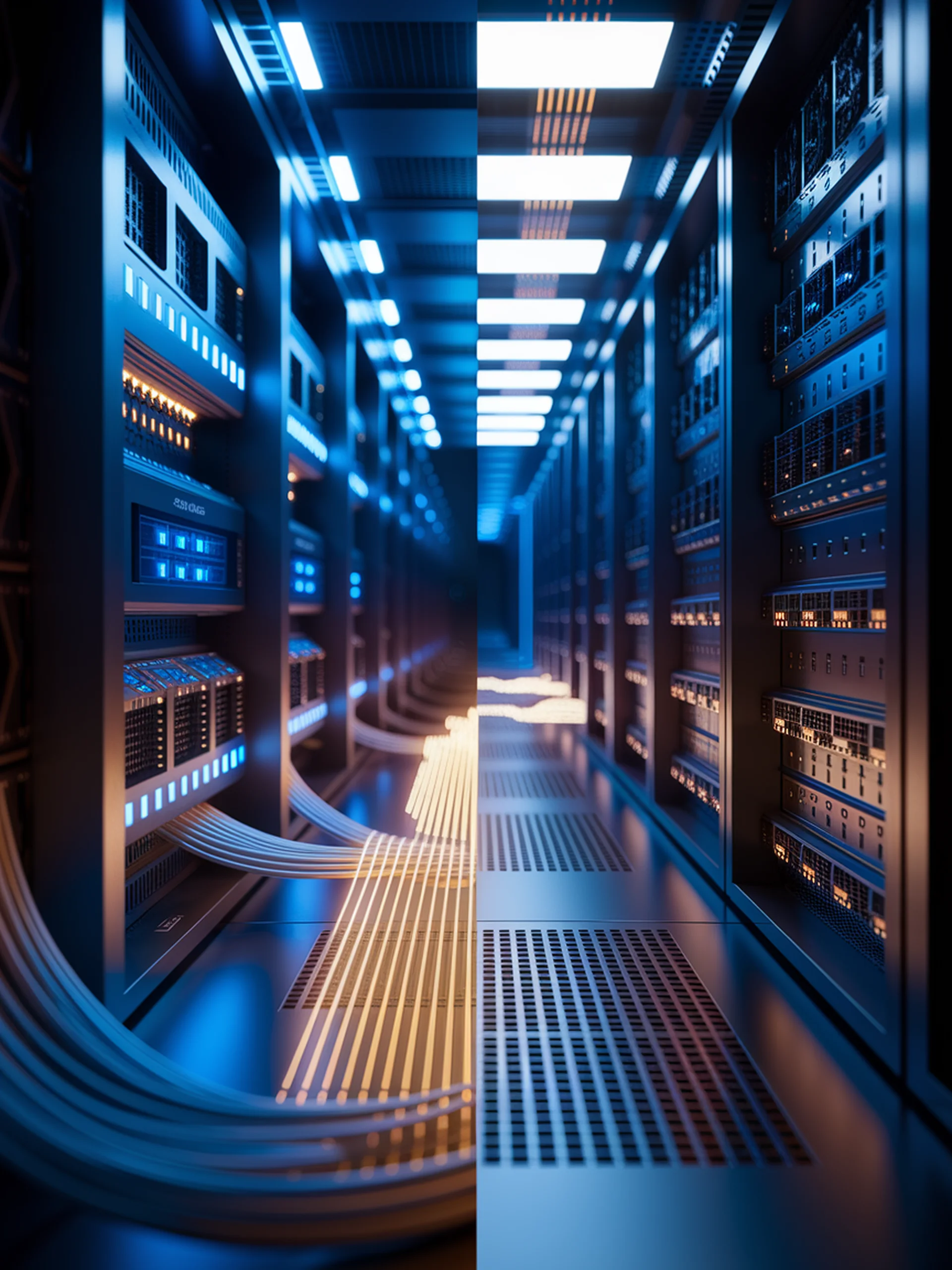
Accelerating MoE Models with Hybrid Computing
Smart CPU-GPU orchestration for faster LLM inference
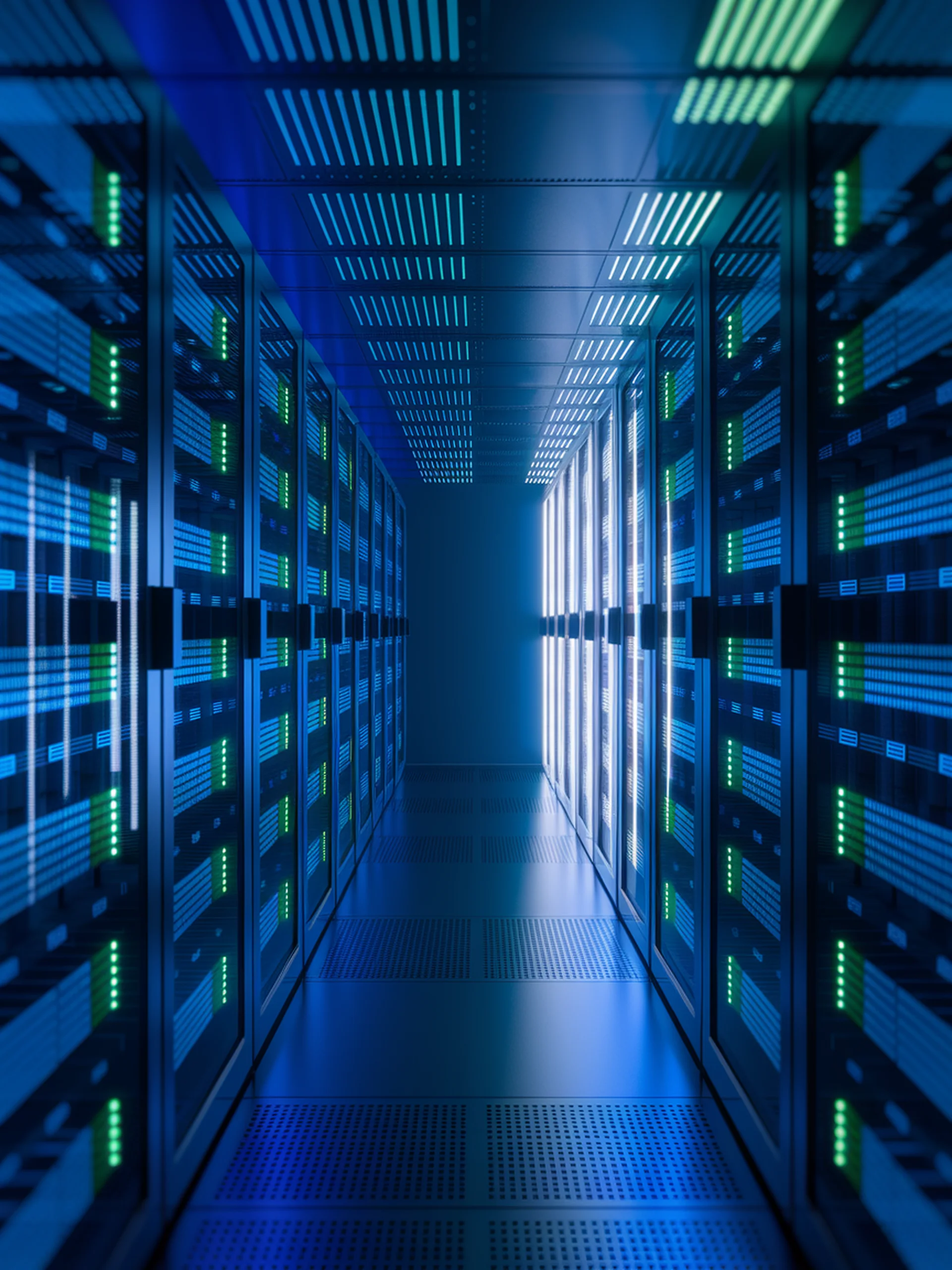
Accelerating LLM Inference with Plato
Efficient parallel decoding without compromising quality

DropBP: Accelerating LLM Training
A novel approach to reduce computational costs in fine-tuning
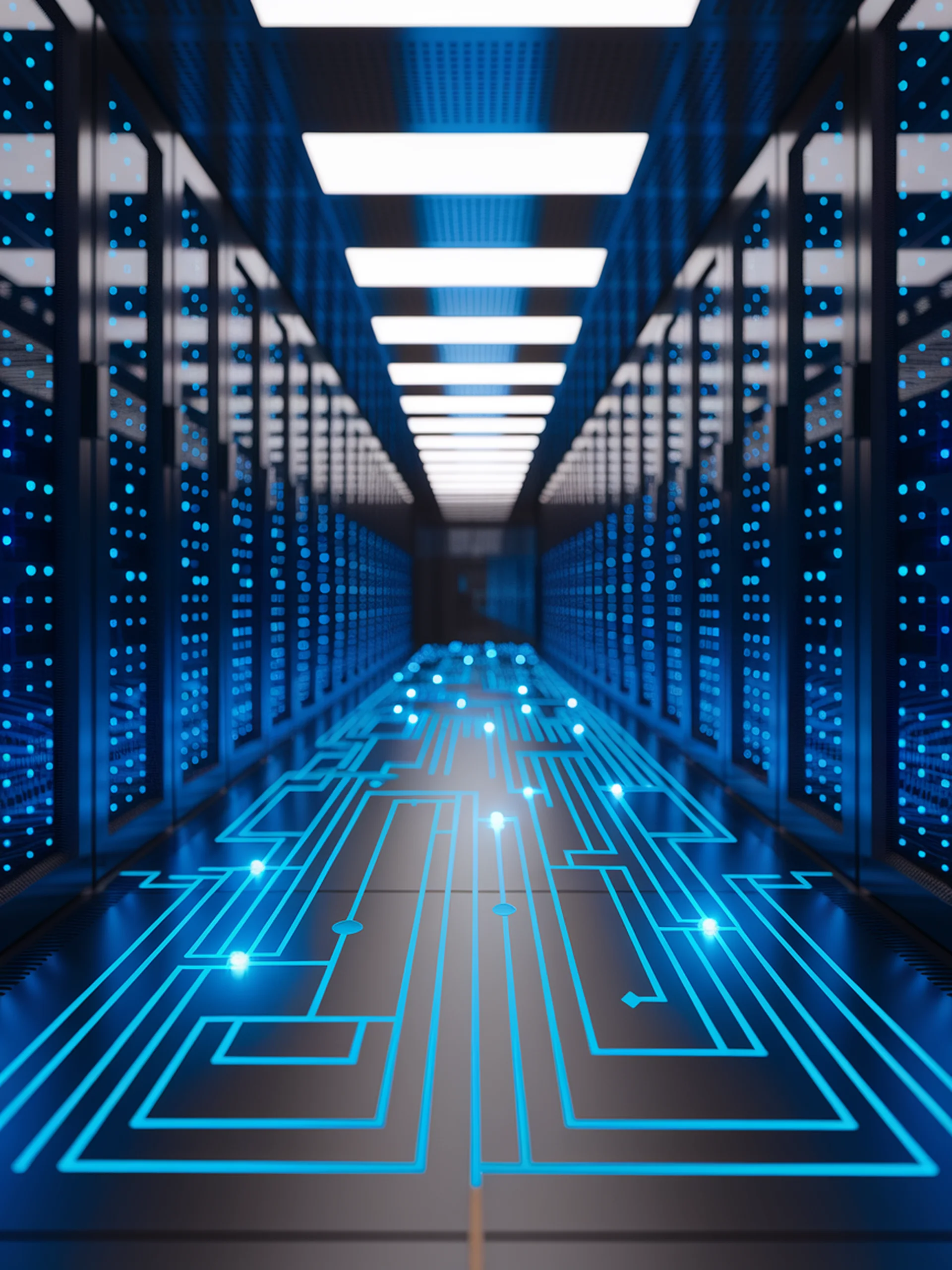
MeanCache: Cutting LLM Costs with Smart Caching
A user-centric semantic caching system that reduces computational costs by 31%
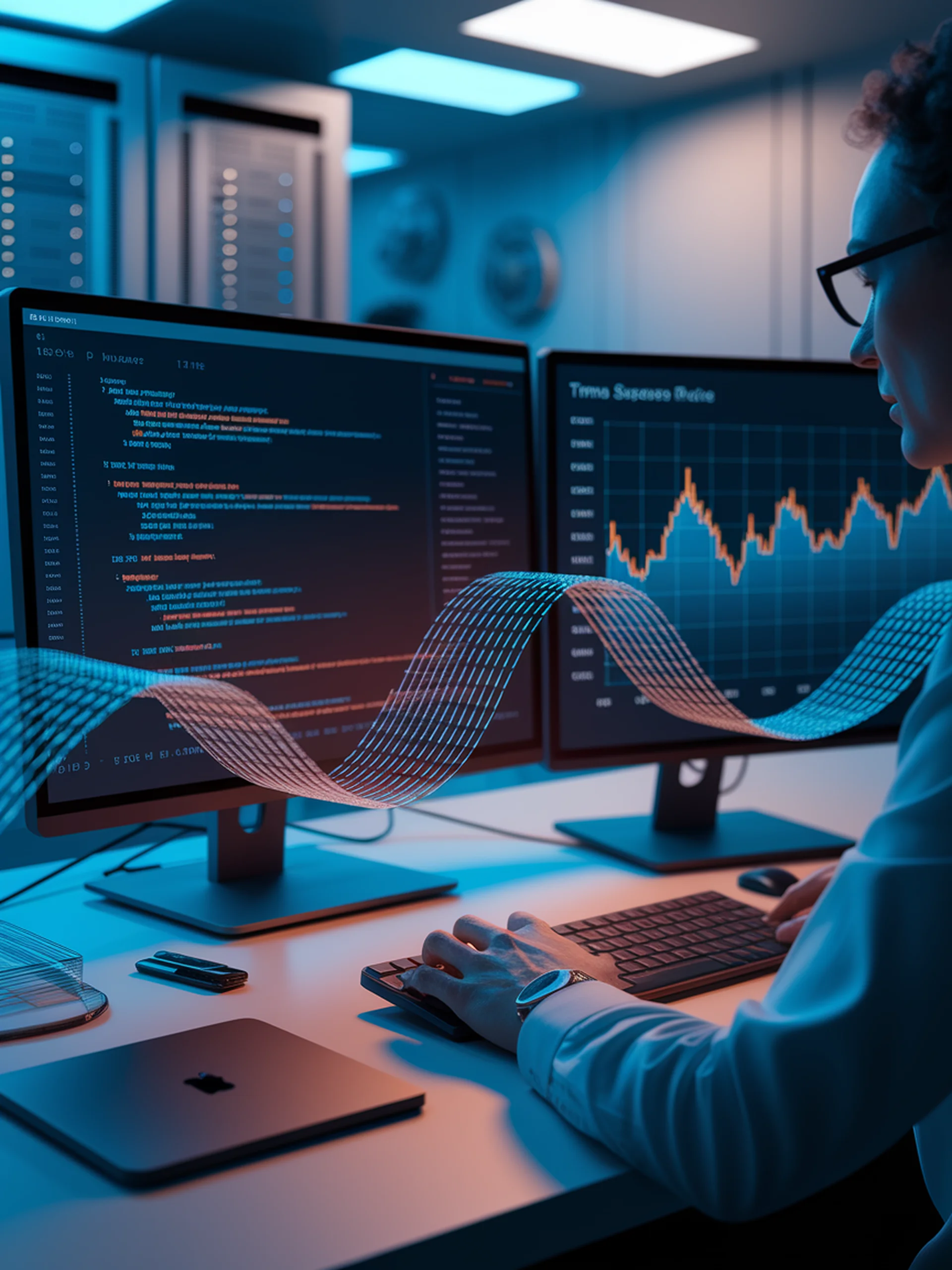
Bridging the Gap: LLMs for Time Series Forecasting
A novel cross-modal fine-tuning approach (CALF) that aligns language and numerical data distributions
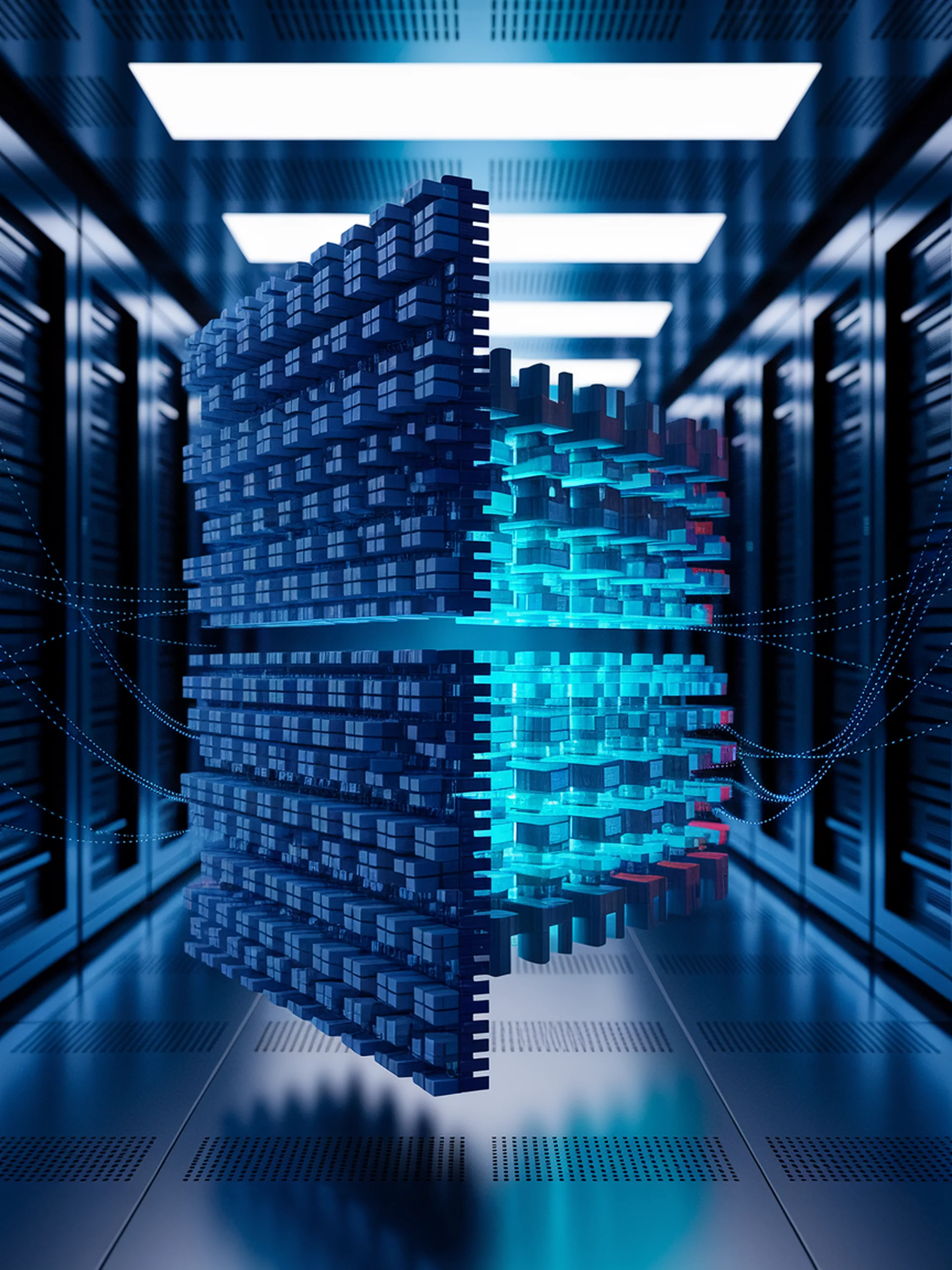
Smarter SVD for LLM Compression
Optimizing model size without sacrificing performance
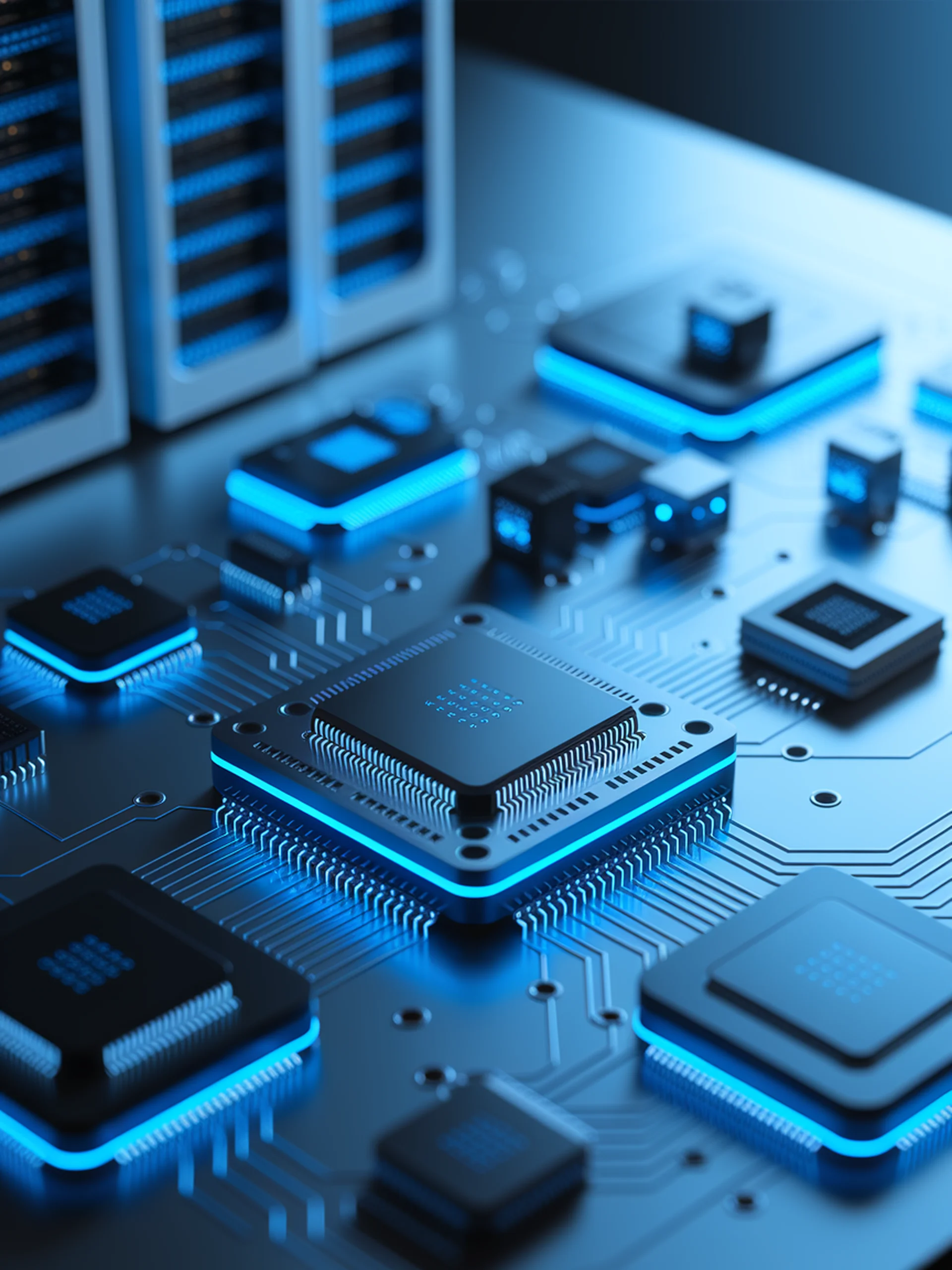
Optimizing LLMs for IoT Devices
An entropy-driven approach to deliver LLM power on resource-constrained hardware
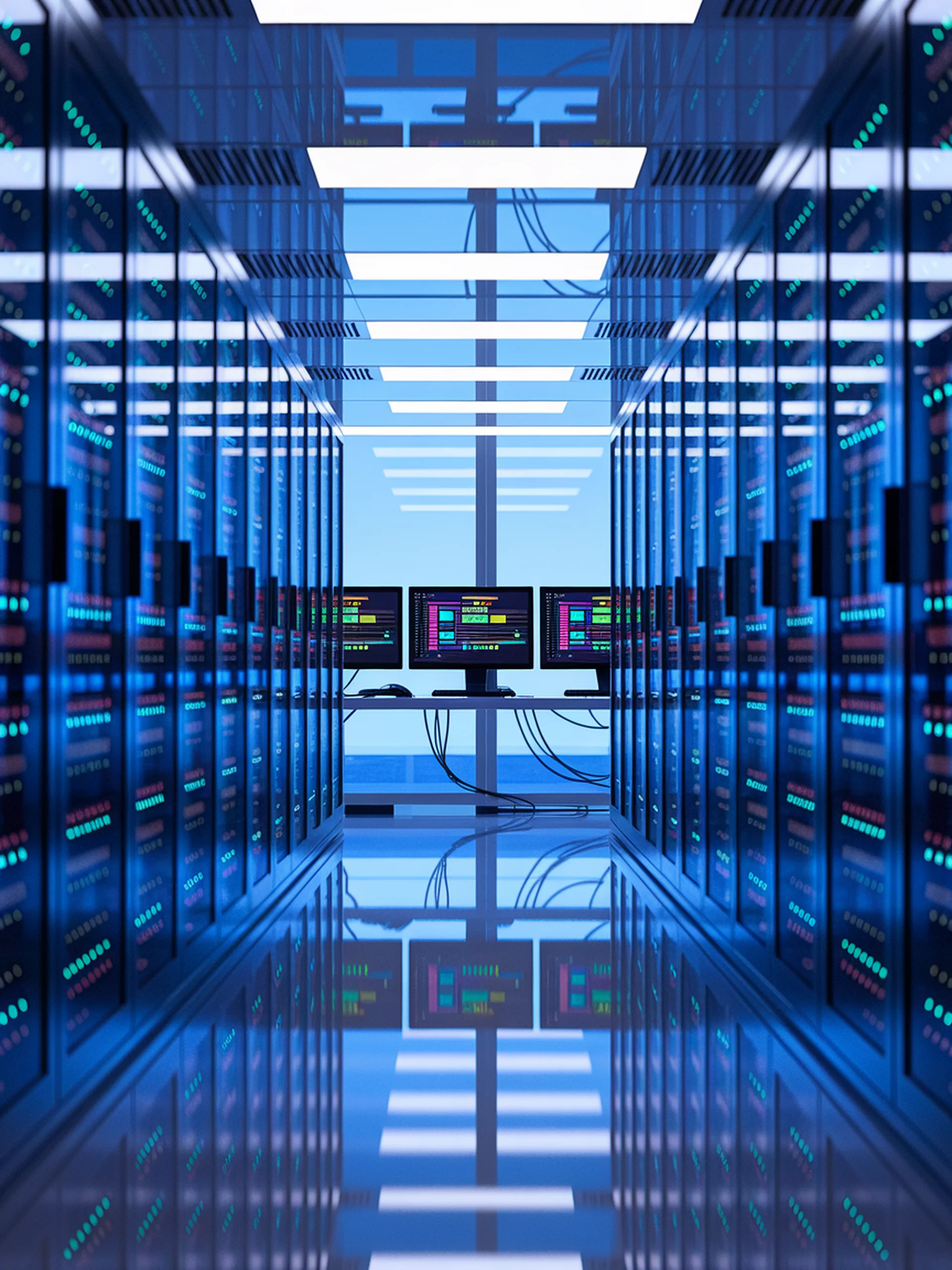
Dataverse: Streamlining Data Processing for LLMs
An Open-Source ETL Pipeline with User-Friendly Design
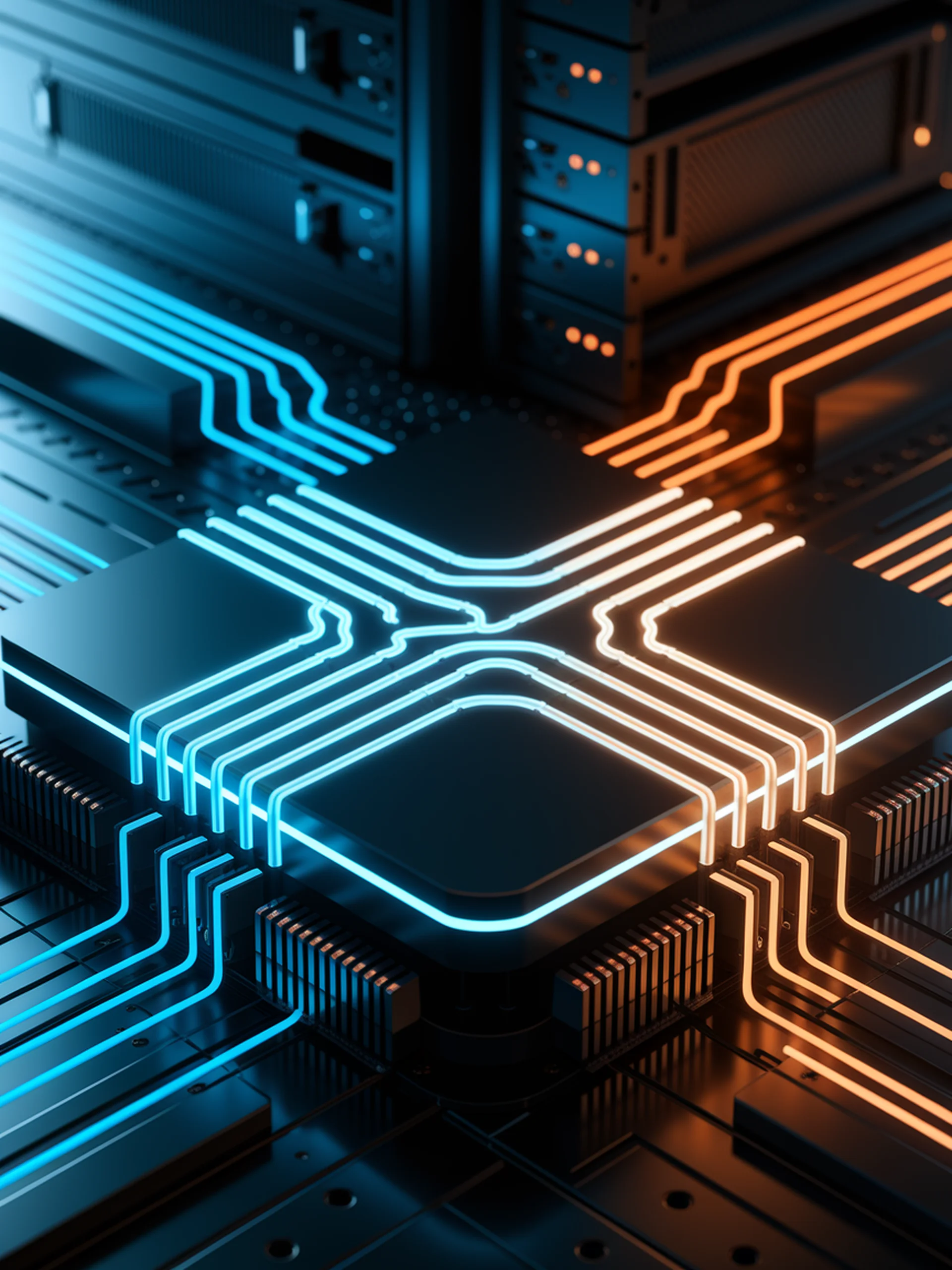
Accelerating Tree-structured LLM Inference
A novel approach to efficiently handle shared computations in complex LLM tasks
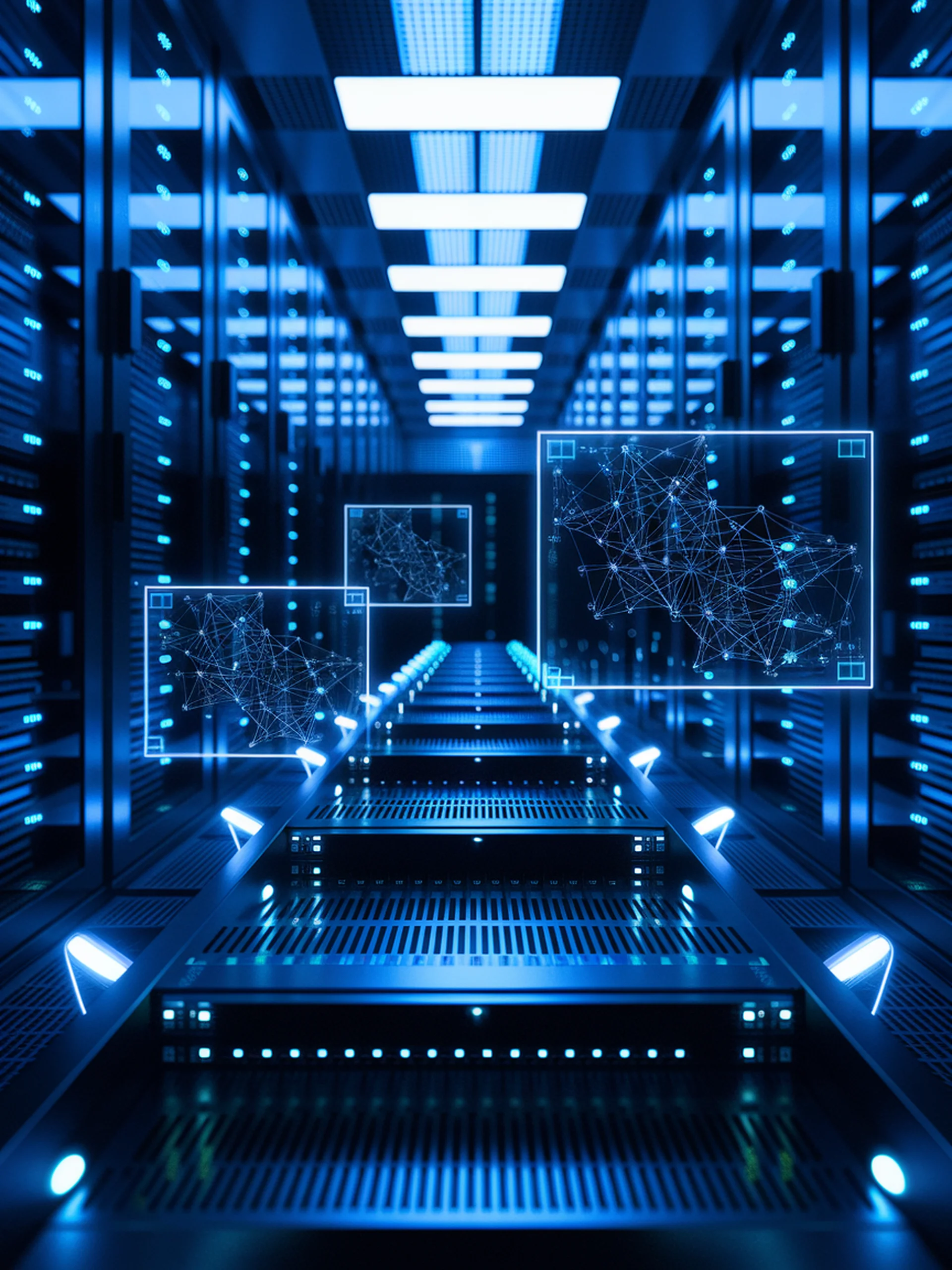
Unlocking Efficient Attention in LLMs
How Sparse Attention Achieves Sub-Quadratic Complexity
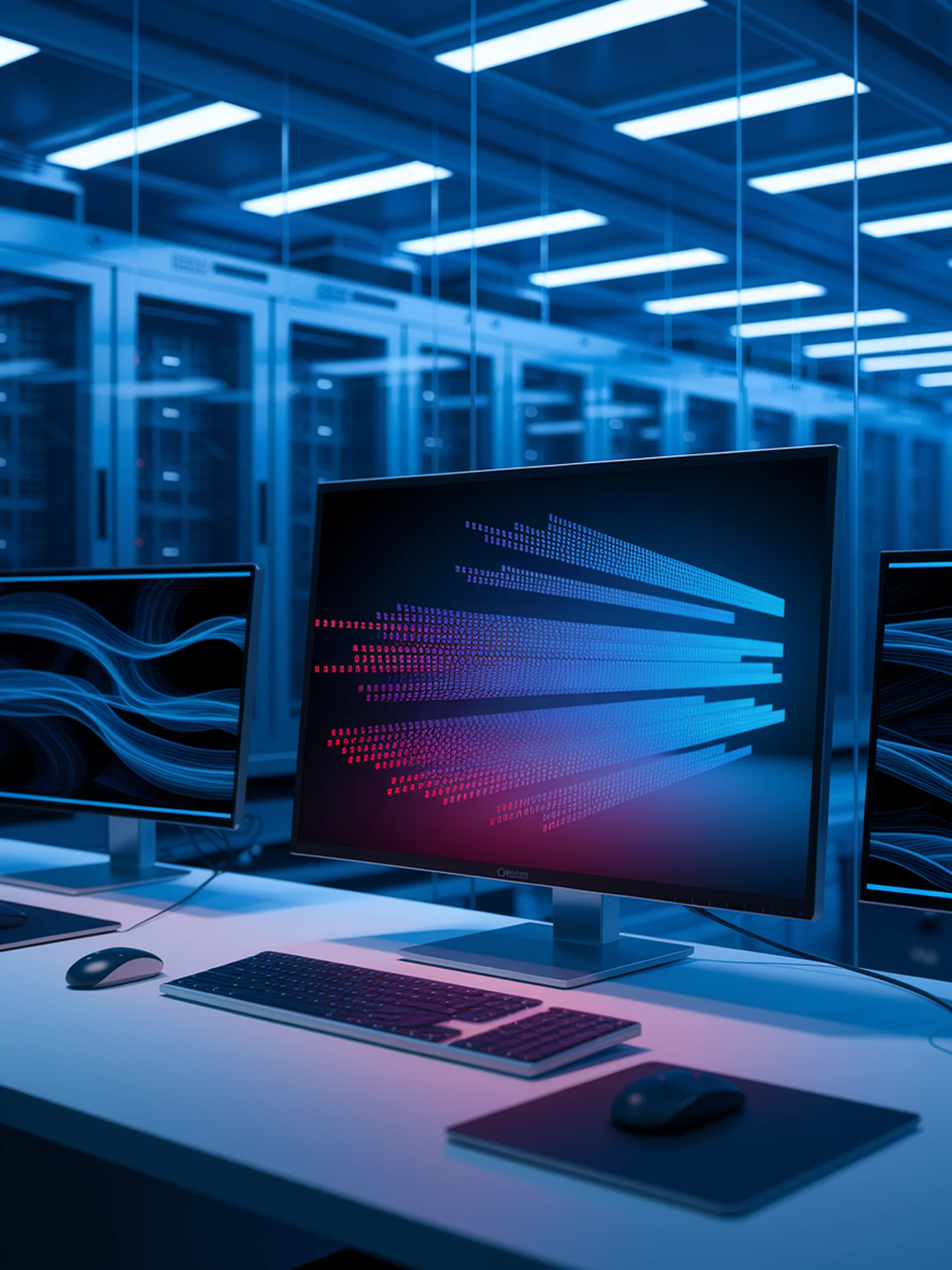
Supercharging LLM Fine-tuning
PiSSA: A Smarter Approach to Parameter-Efficient Fine-Tuning
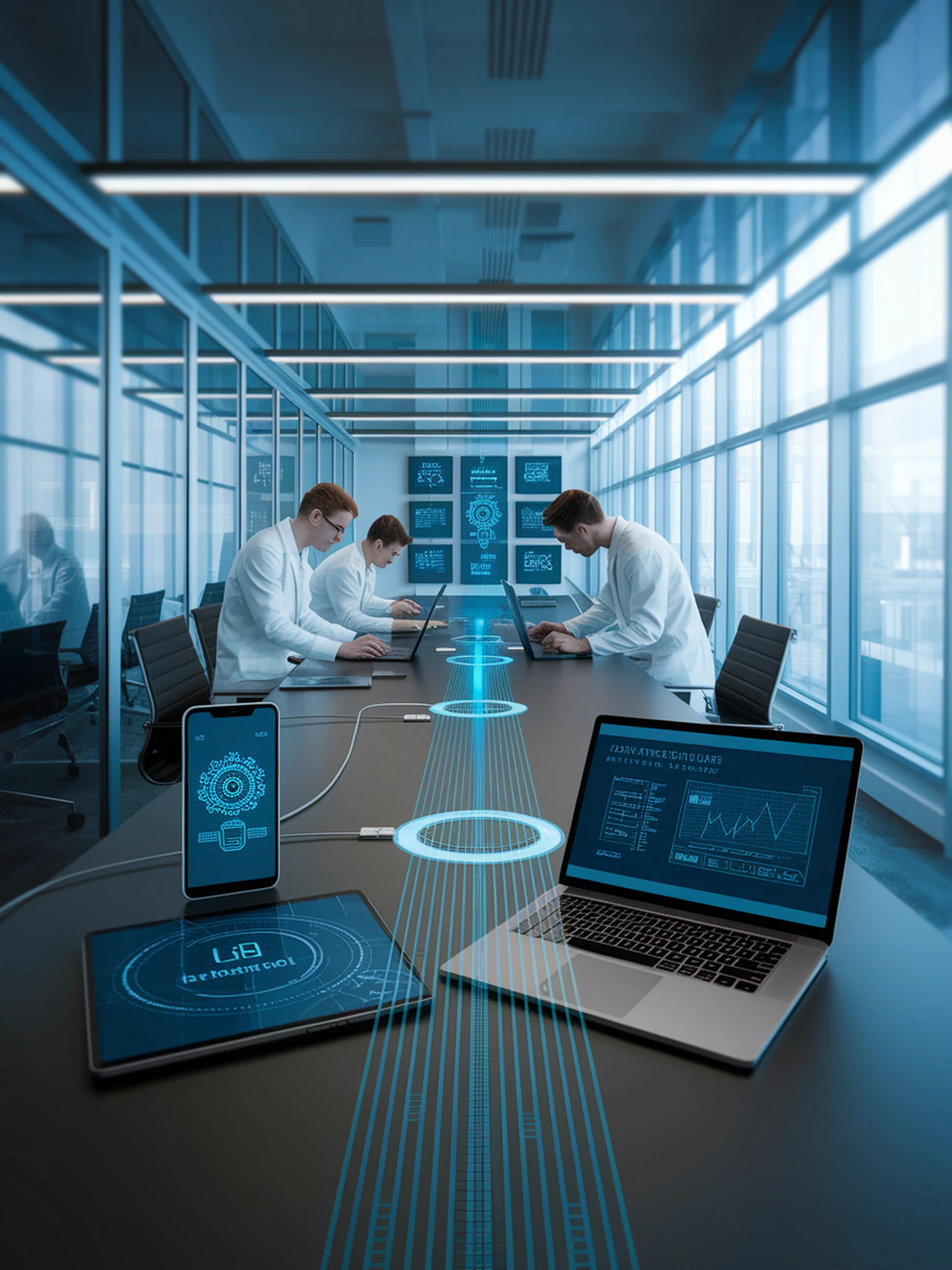
Bridging LLMs to New Platforms
A top-down testing approach for deploying AI on mobile, browsers & beyond

Boosting LLM Efficiency with Model-Attention Disaggregation
A novel architecture for optimized LLM deployment on heterogeneous hardware
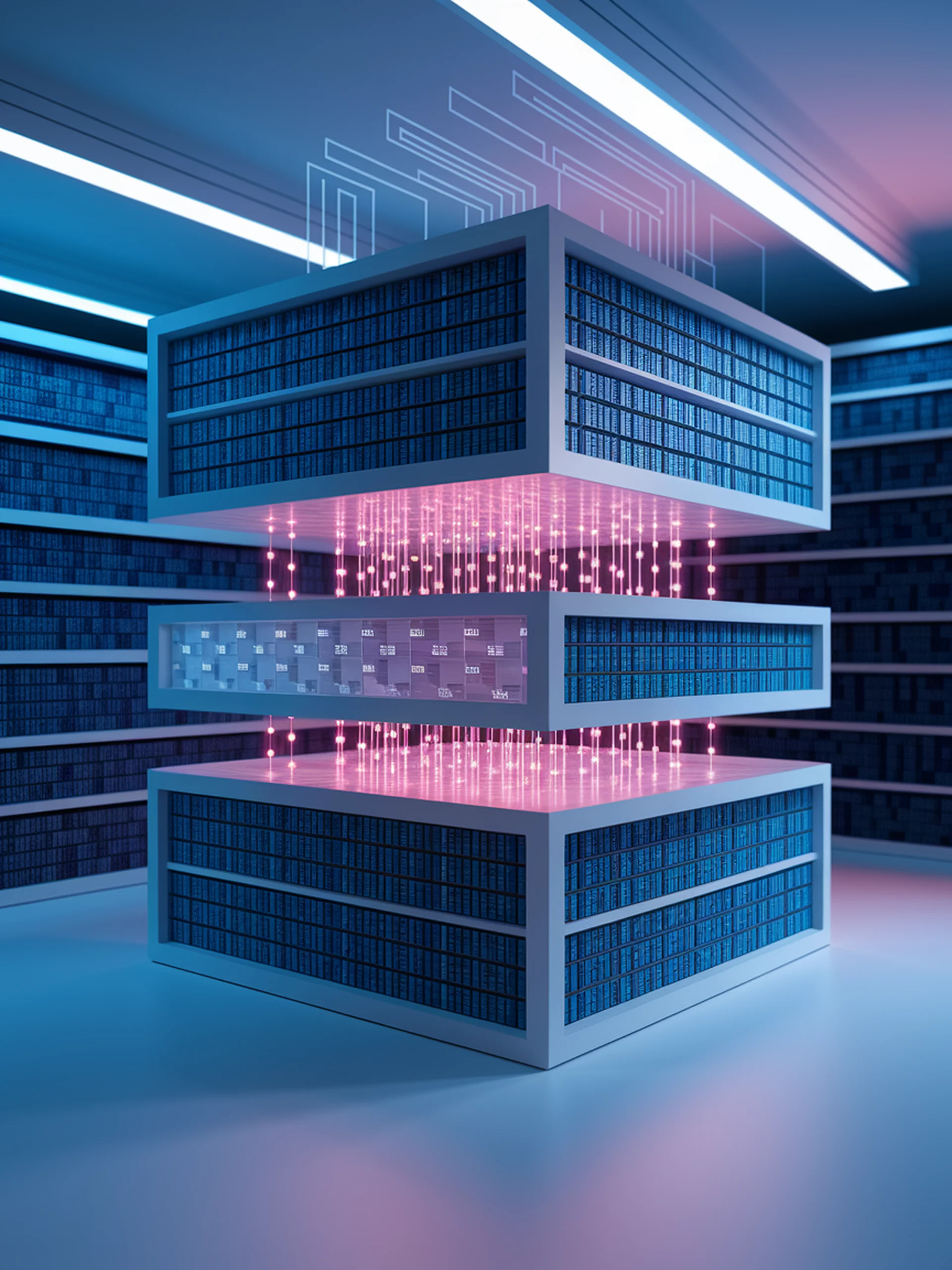
Hierarchical Memory for Language Models
A novel approach for efficient processing of long contexts
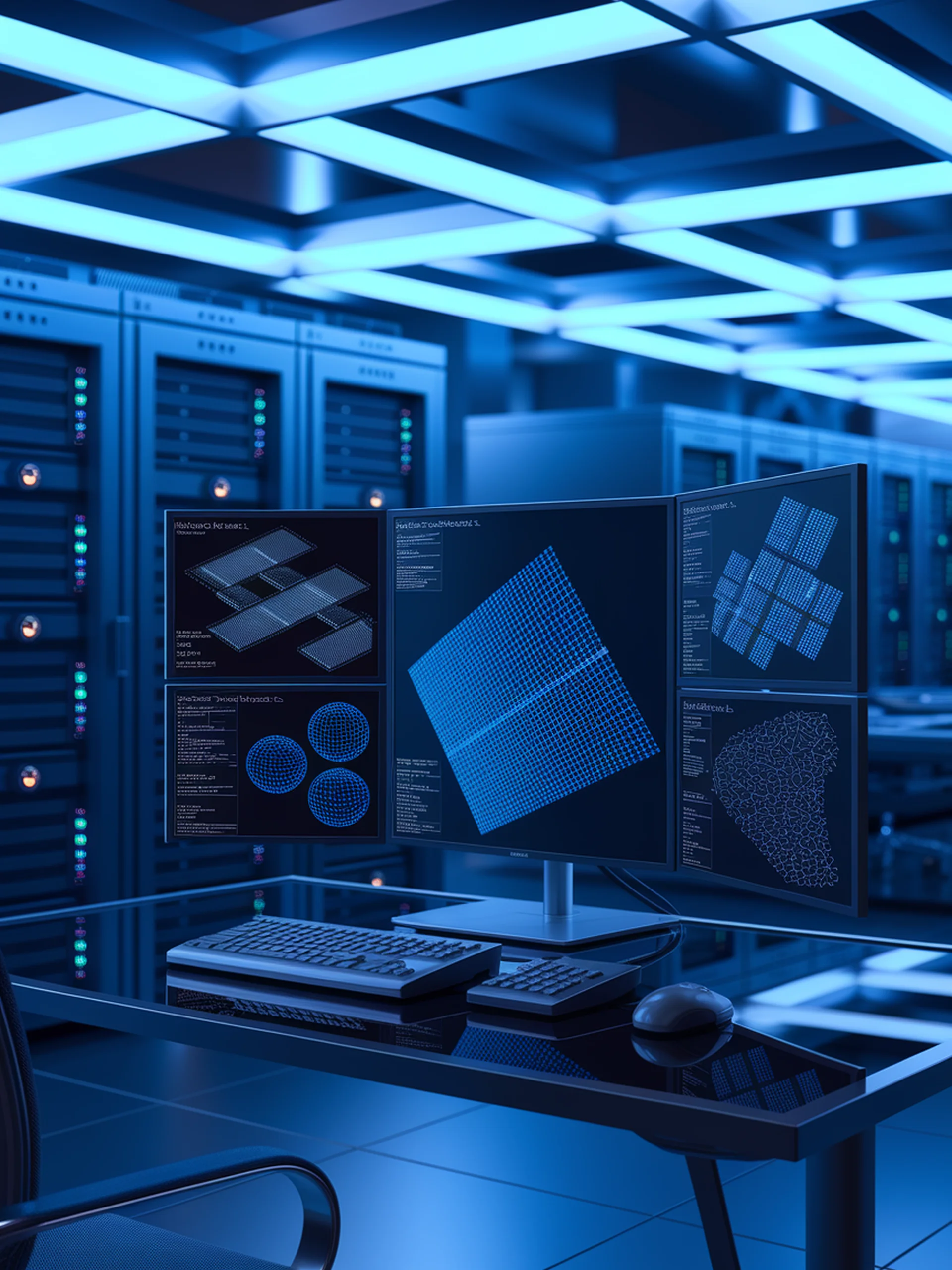
Smarter LLM Compression
Adaptive Low-Rank Compression Using Bayesian Optimization
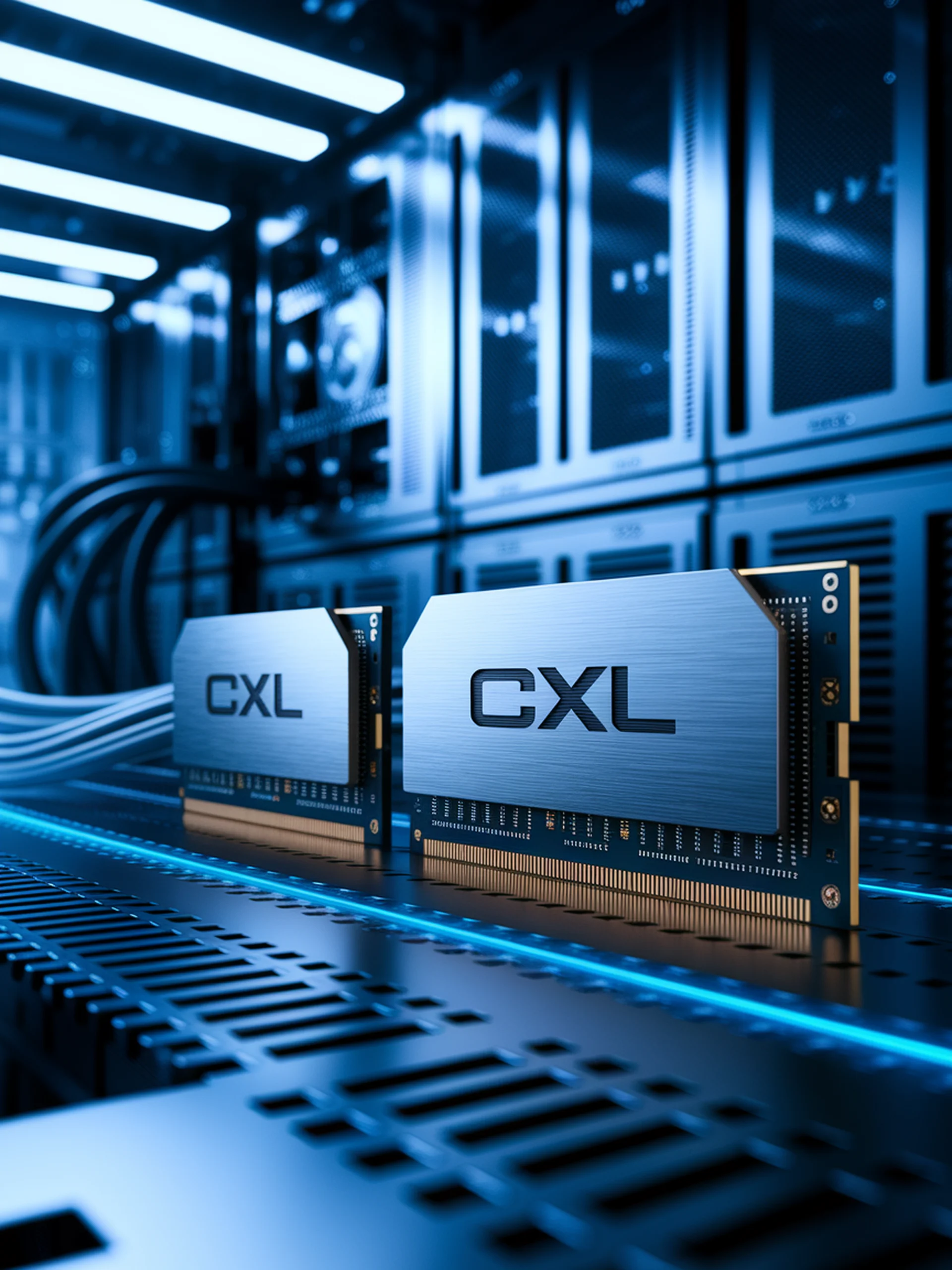
CXL Memory Technology: Real-World Performance Analysis
Evaluating practical adoption scenarios for Compute eXpress Link memory
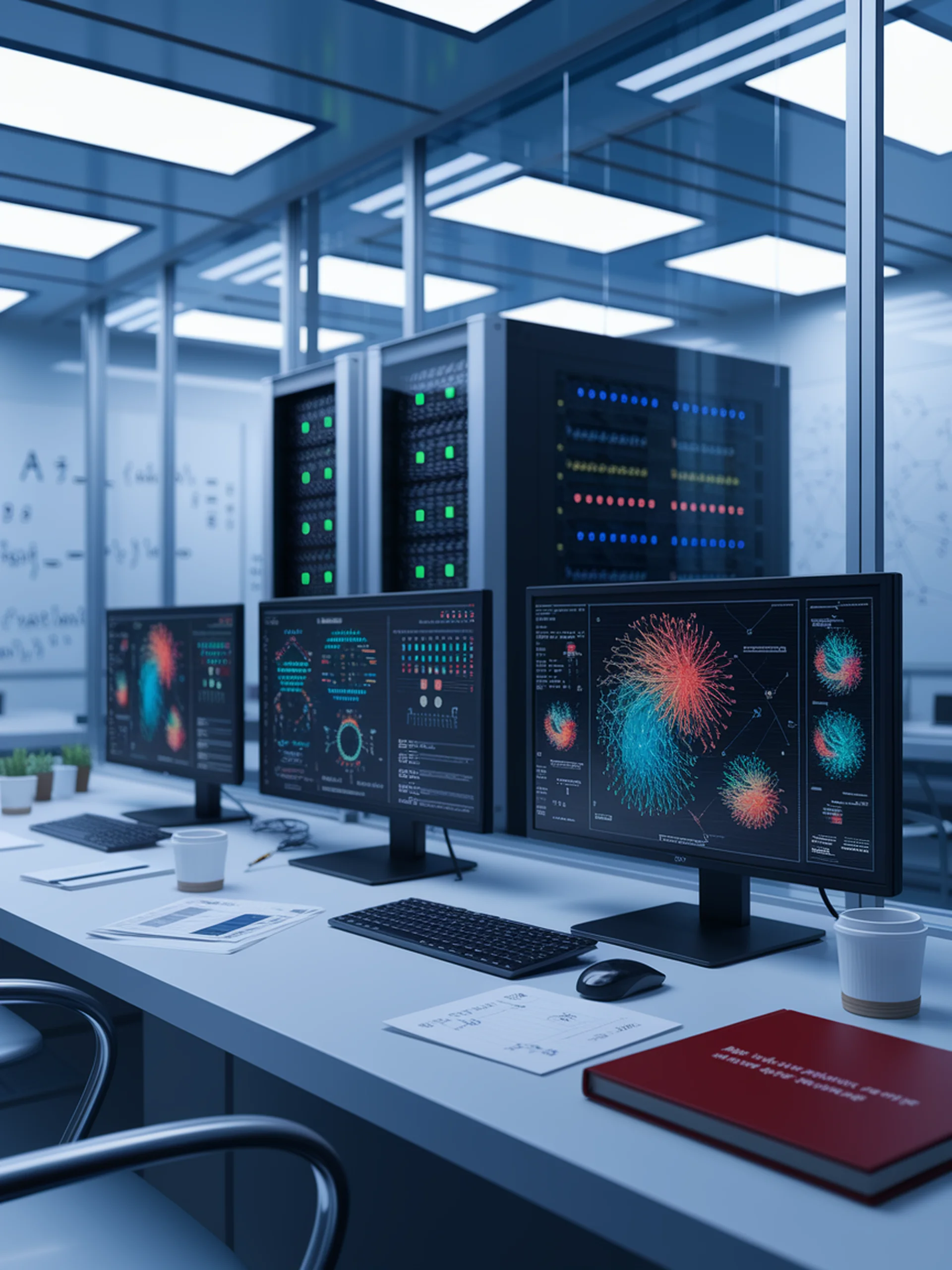
MallowsPO: Fine-Tune Your LLM with Preference Dispersions
By Haoxian Chen, Hanyang Zhao...
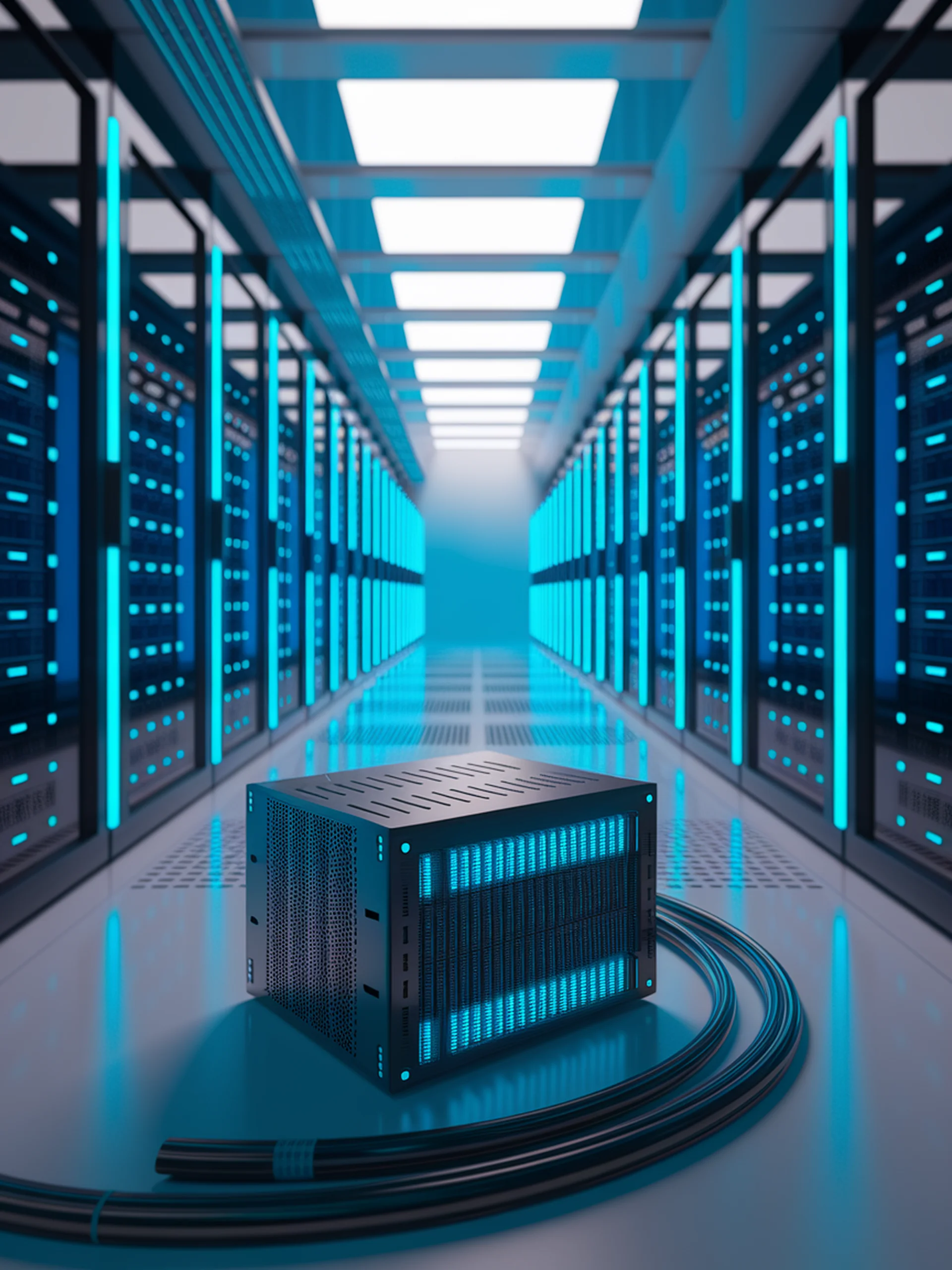
SpinQuant: Optimizing LLMs for Efficiency
Enhancing model quantization with learned rotations
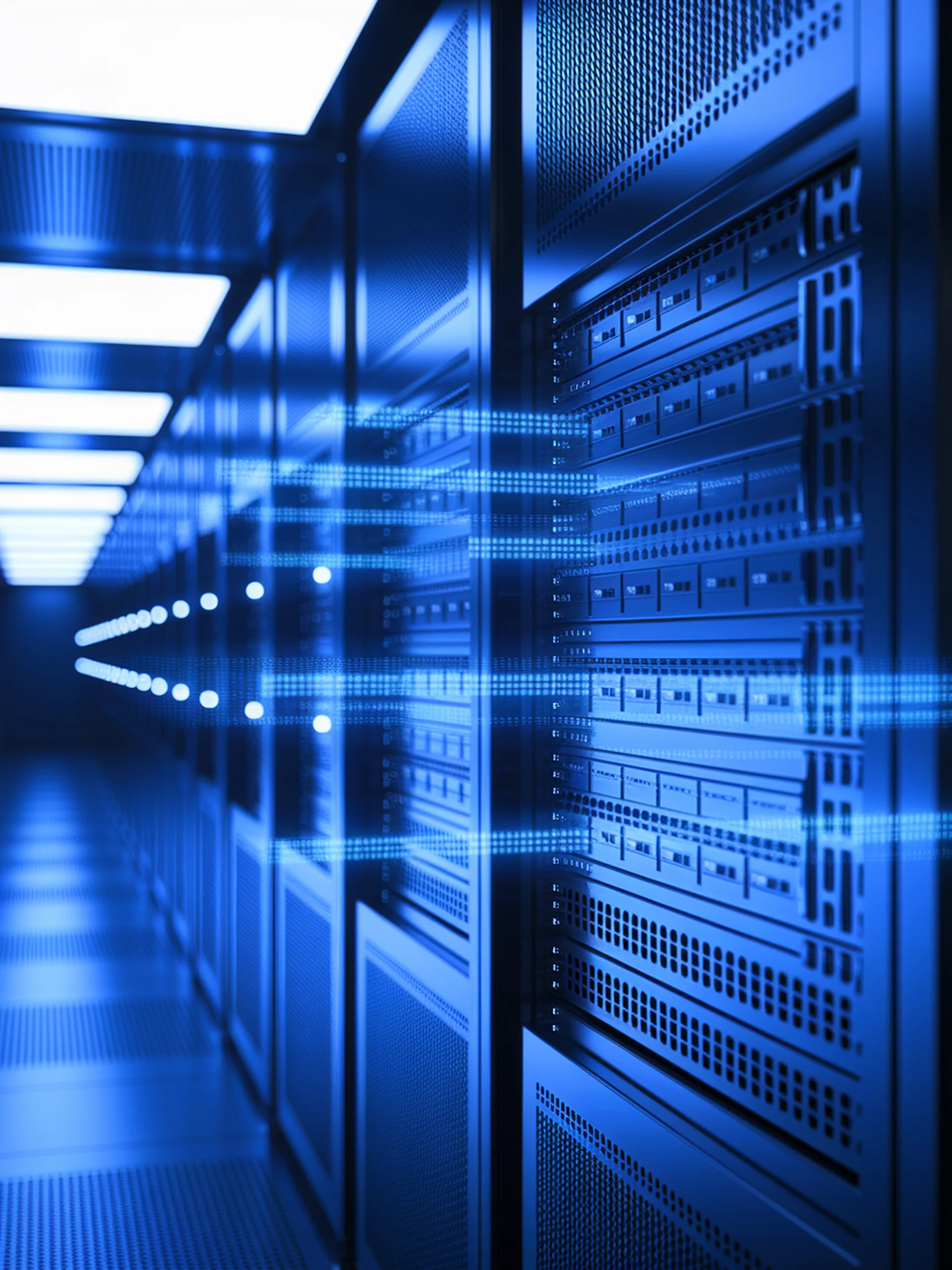
CacheBlend: Accelerating RAG Performance
Fusion of cached knowledge for faster LLM response times
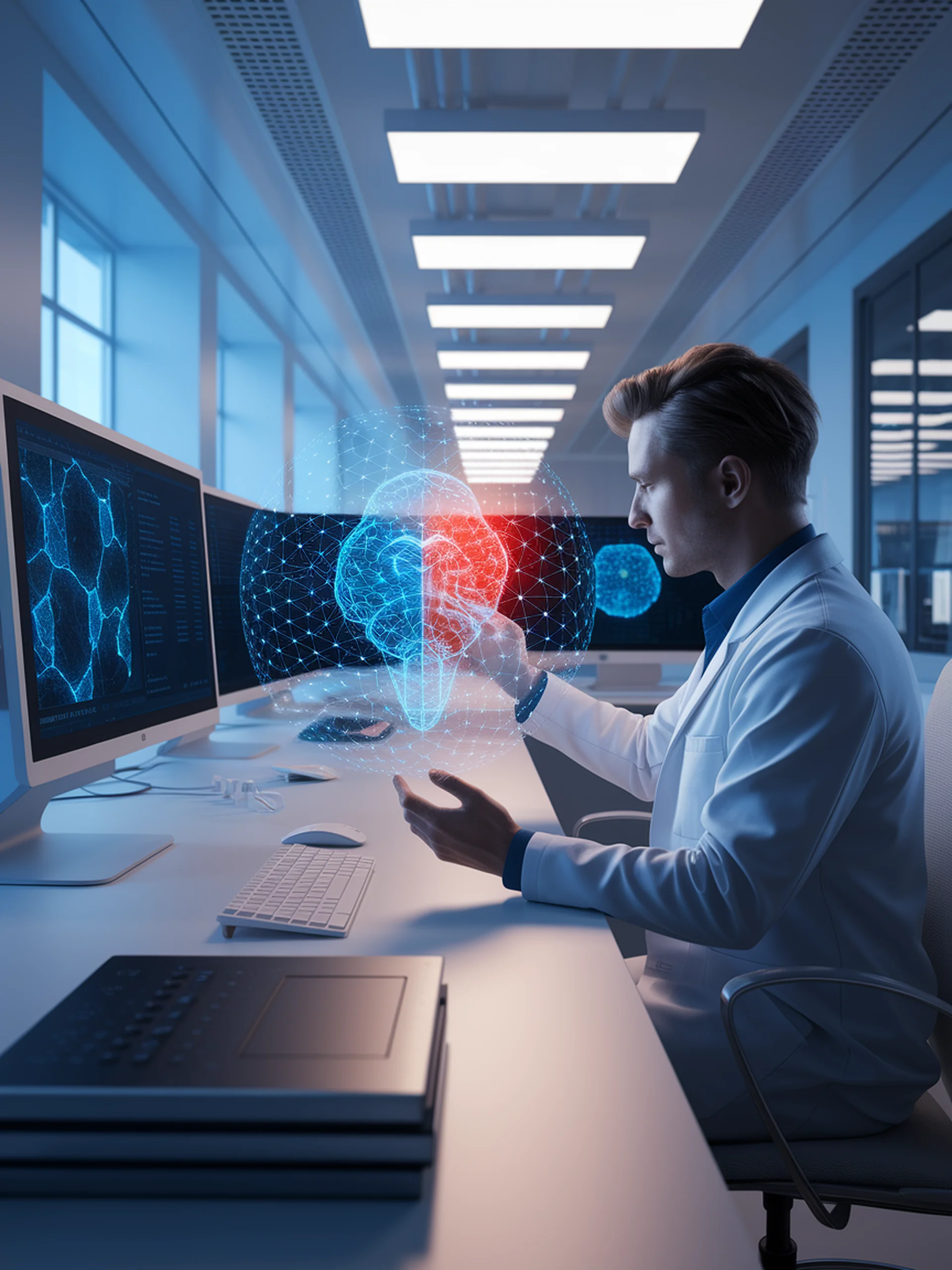
Smarter Model Editing for LLMs
Preserving capabilities while updating knowledge
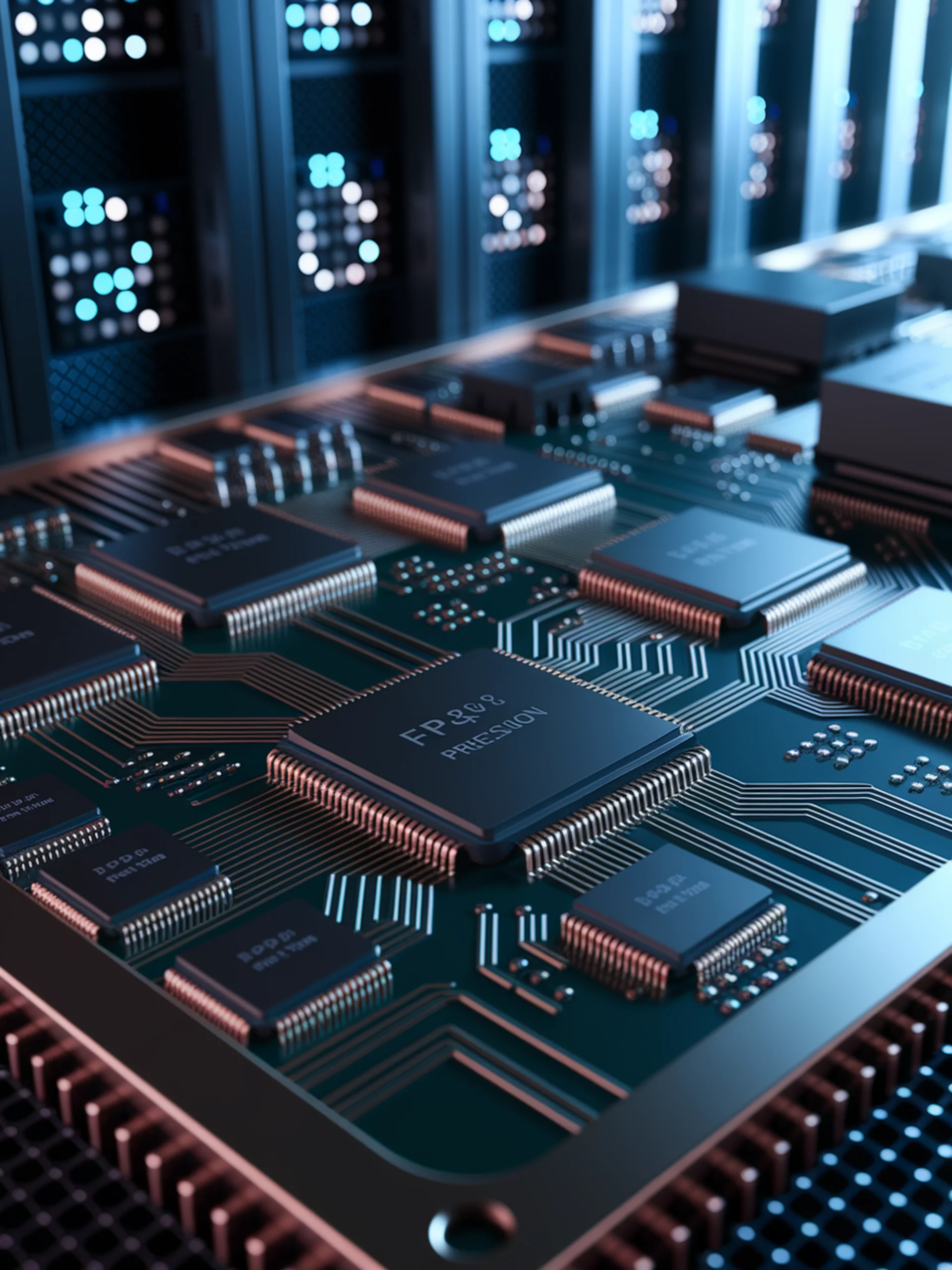
Optimizing LLM Training with FP8 Precision
Quantifying stability impacts of reduced-precision arithmetic

Optimizing Model Compression
Uncovering the non-orthogonal relationship between sparsity and quantization
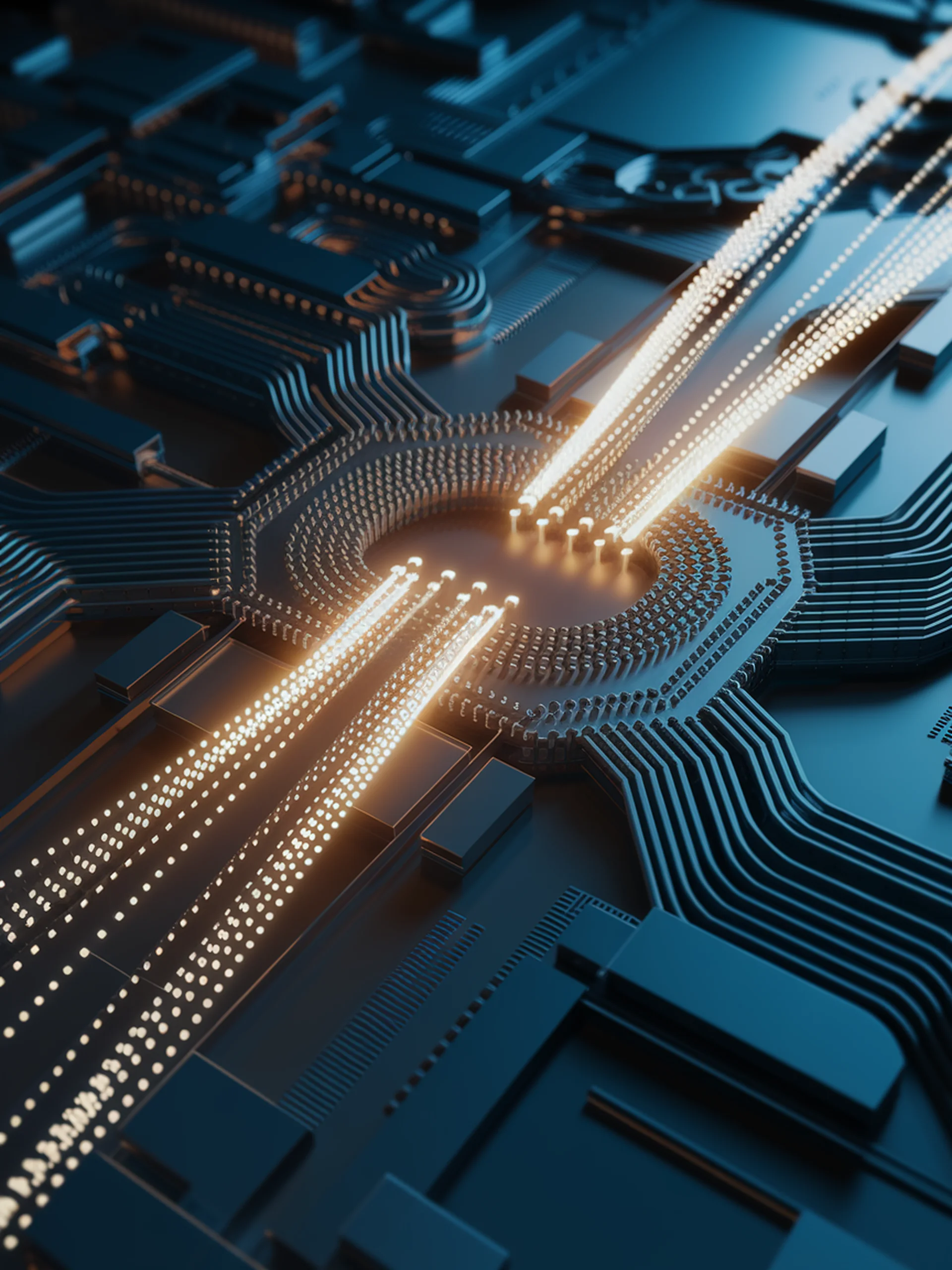
Smarter Expert Routing for LLMs
Bidirectional selection enhances efficiency in Mixture-of-Experts models
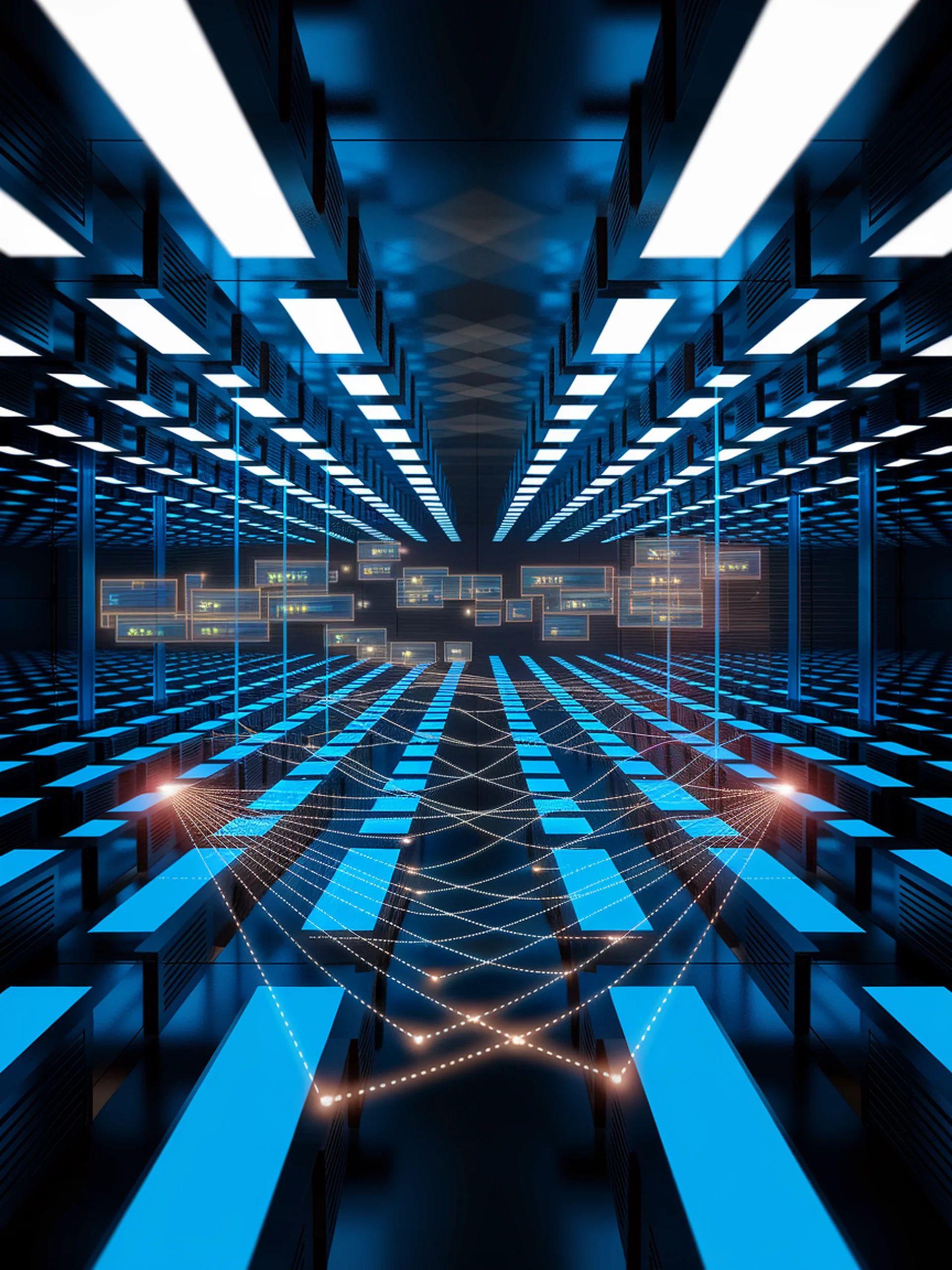
Optimizing LLM Serving With Helix
A Max-Flow Approach for Heterogeneous GPU Environments
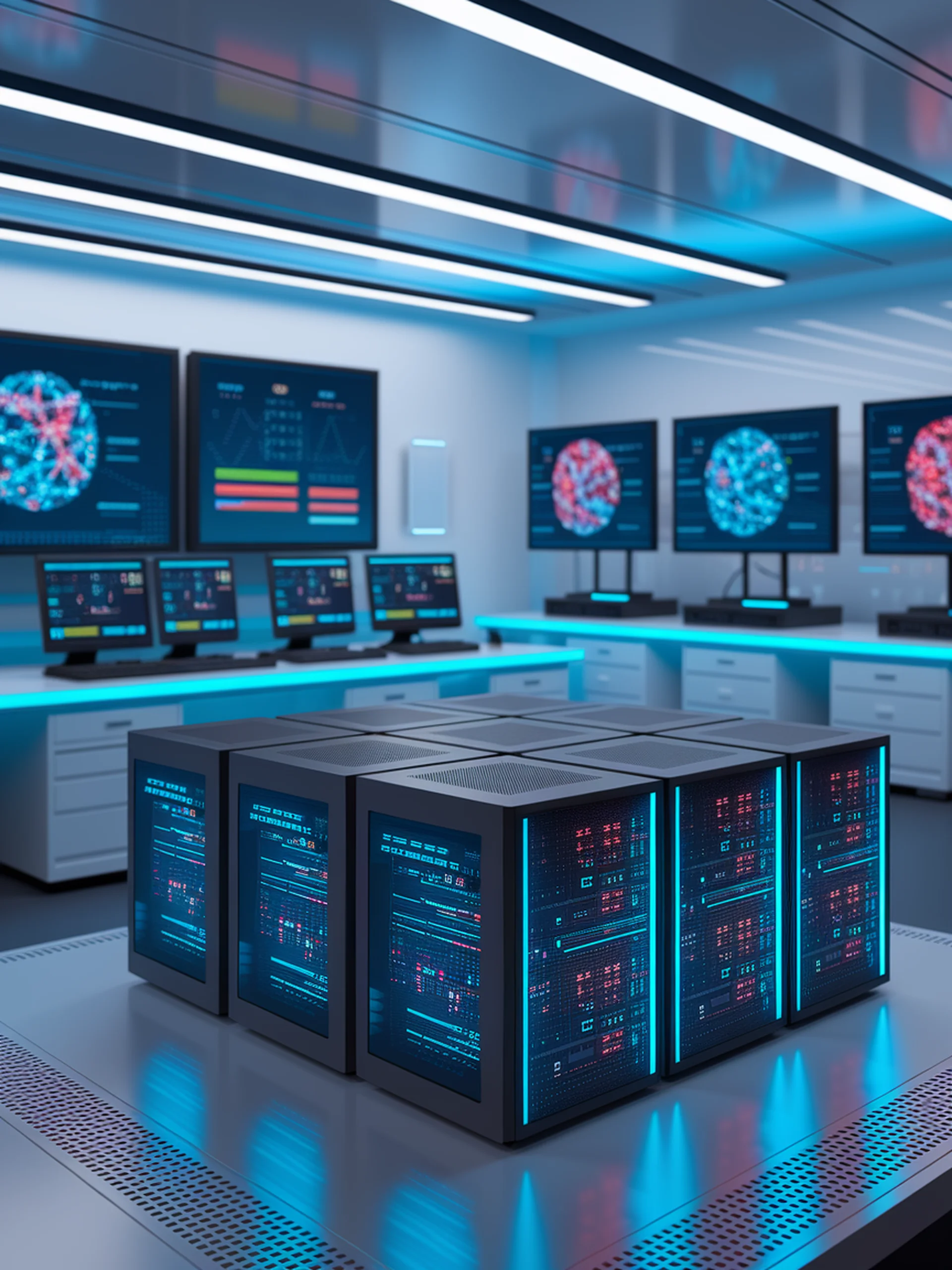
Optimizing MoE Models for Real-World Use
A systematic approach to compression for Mixture of Experts architectures
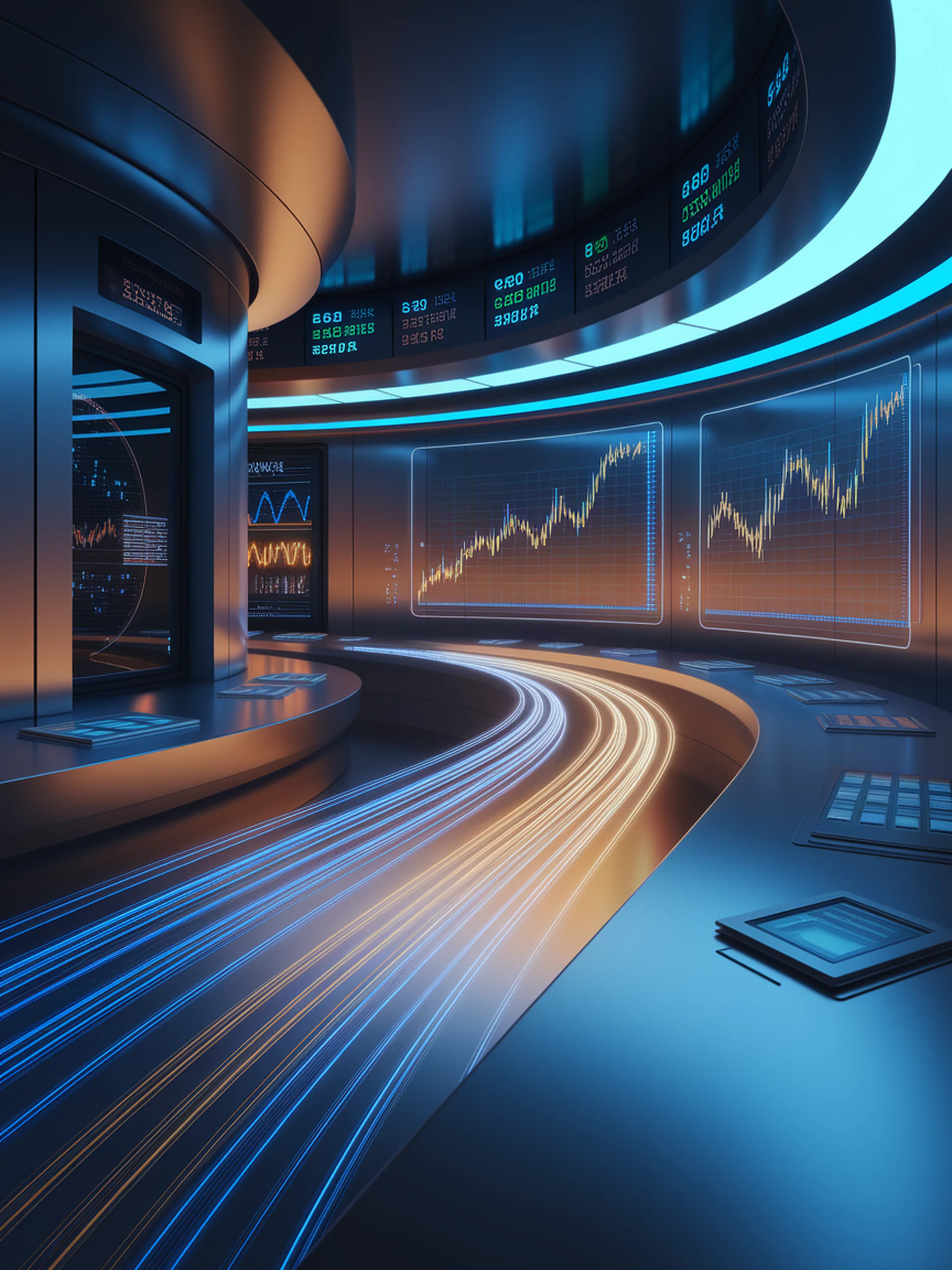
Smart Model Combining in Real-Time
Adaptive filtering outperforms static mixing of large language models
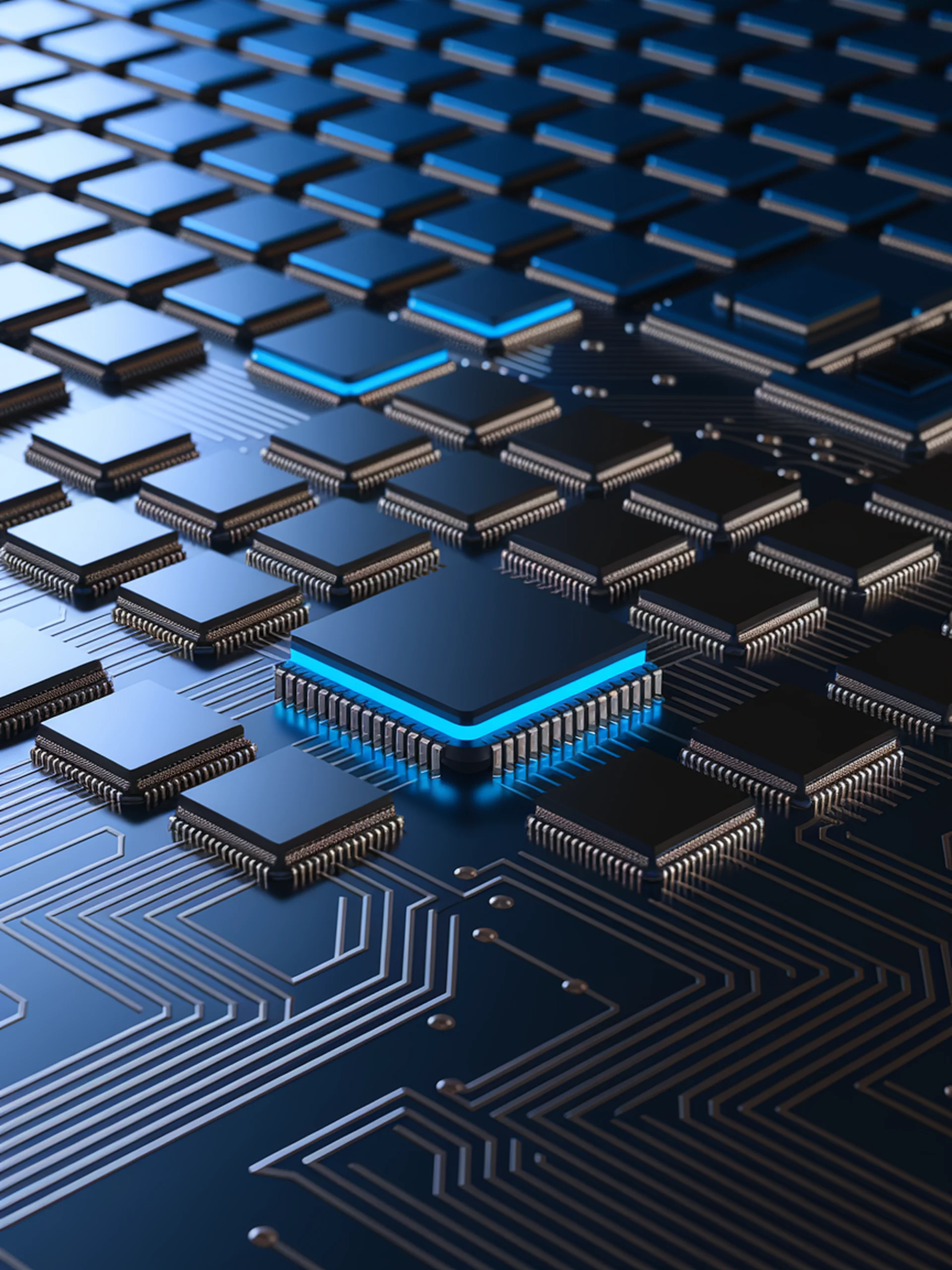
Optimizing MoE Models with Smart Quantization
Structure-aware compression for large language models
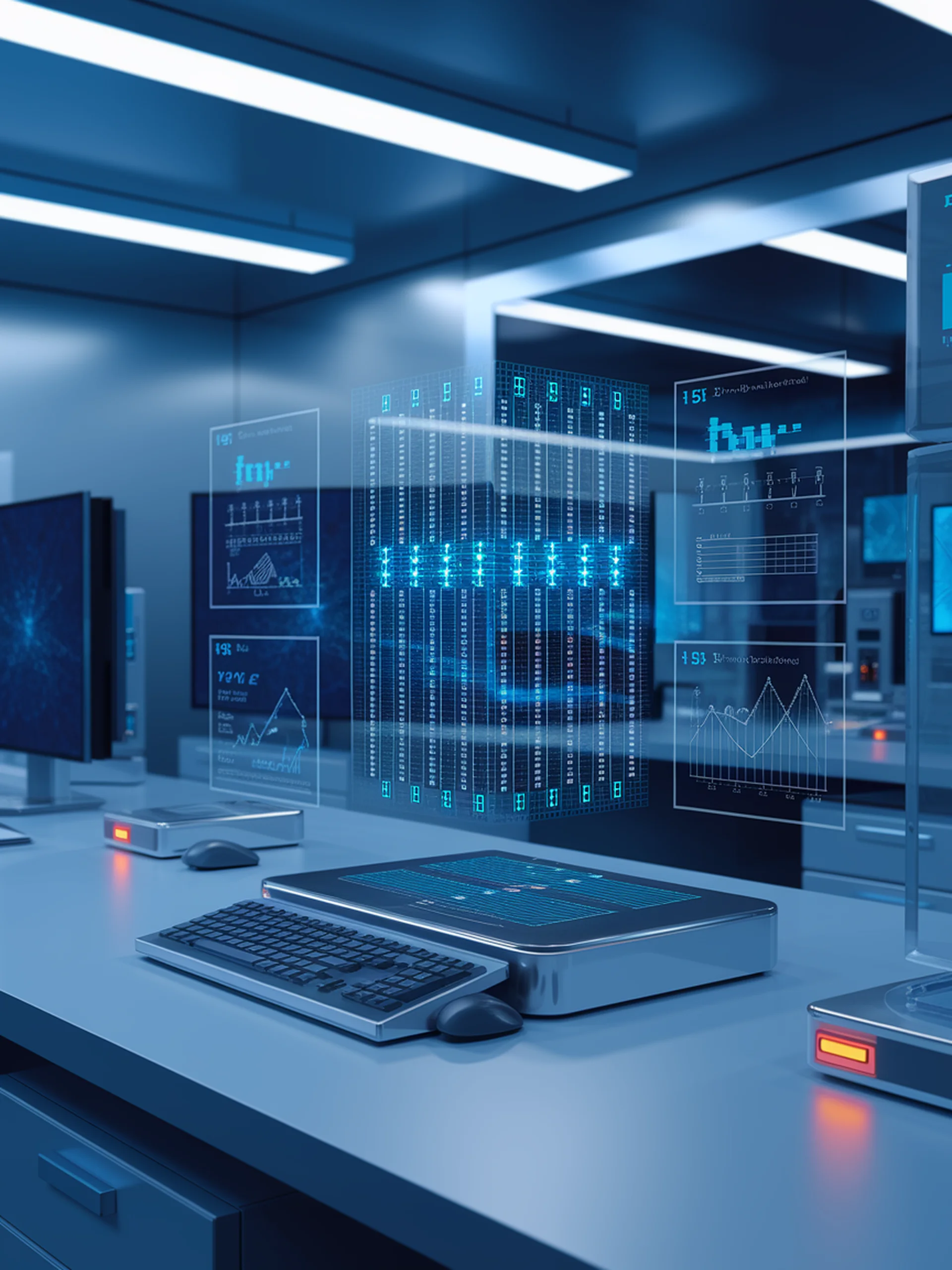
MiLoRA: Smarter Fine-Tuning for LLMs
Using SVD Analytics for More Efficient Model Adaptation
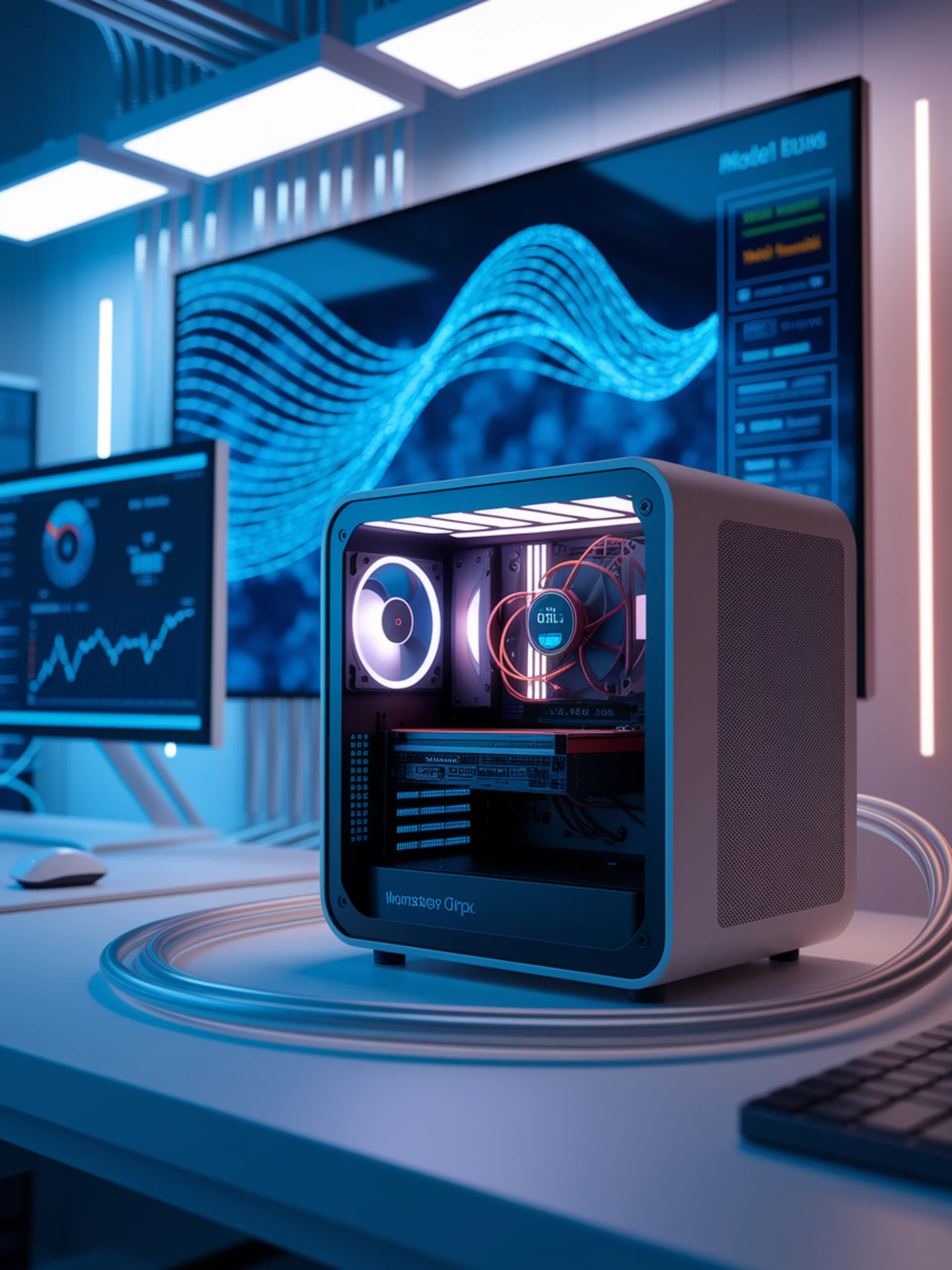
Optimizing LLM Fine-Tuning for Limited Hardware
Breaking GPU memory barriers through learned sparse projectors
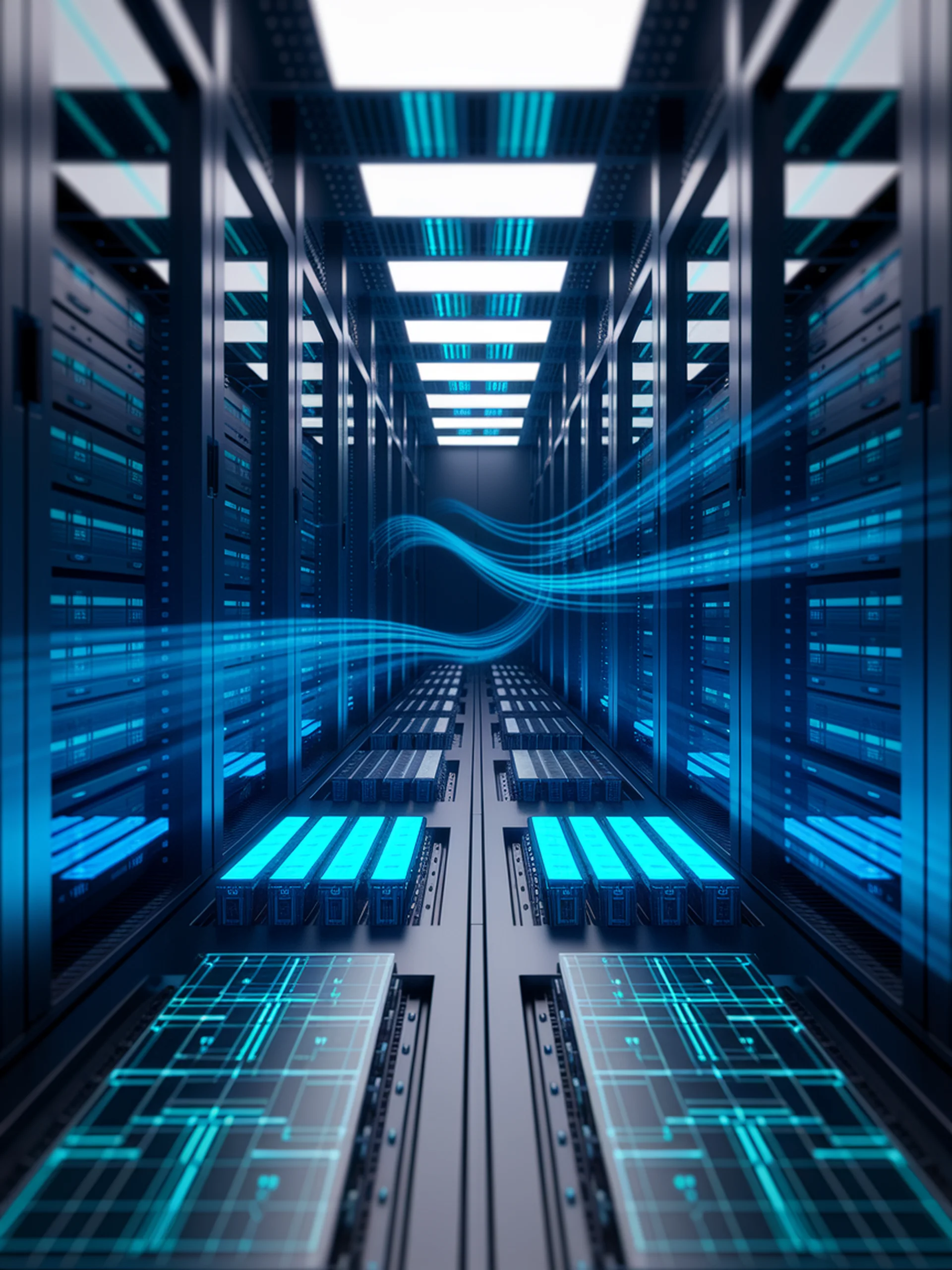
Smarter Memory Management for LLMs
D2O: A Dynamic Approach to Handling Long Contexts
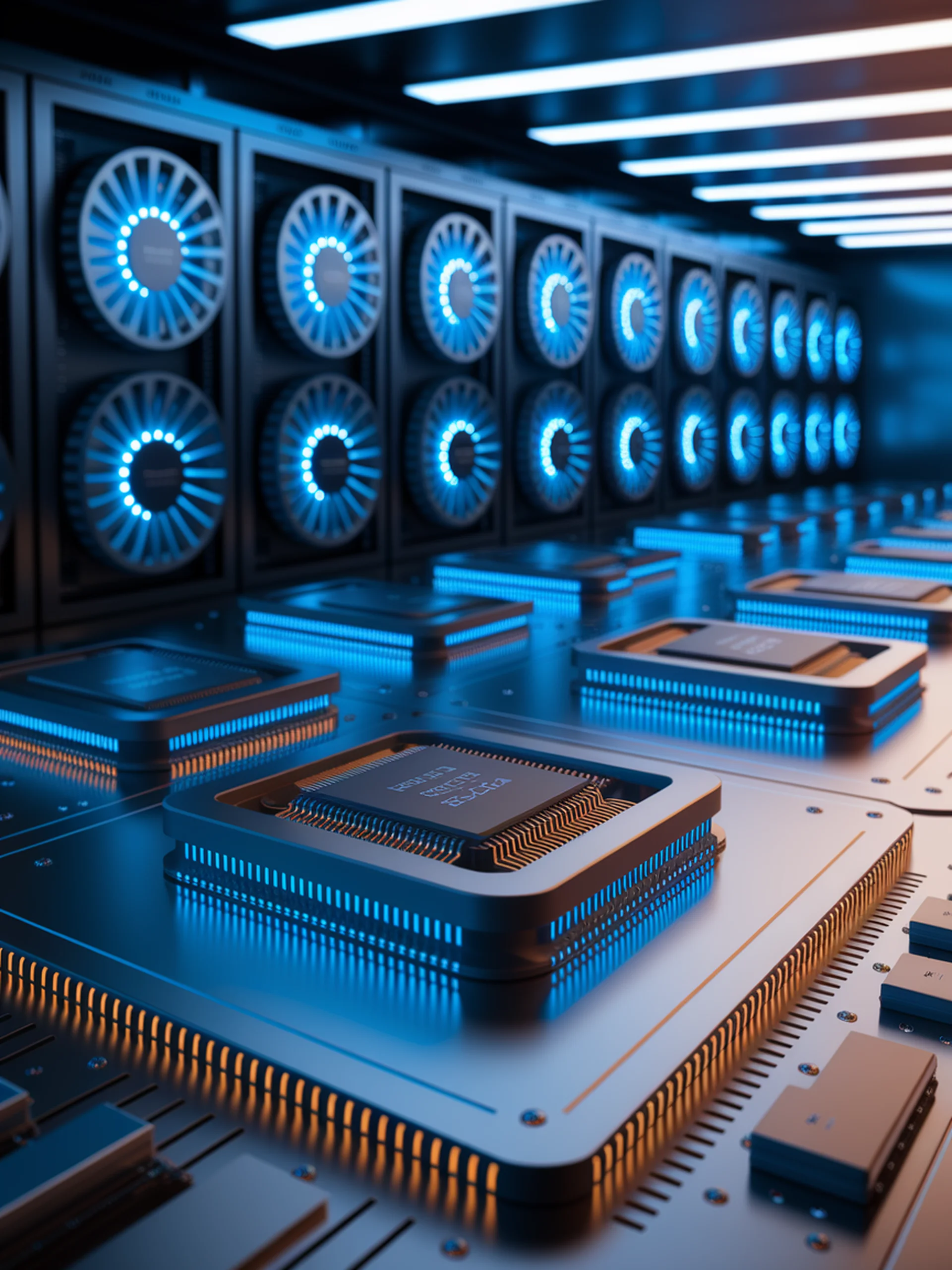
Smarter AI Model Compression
Optimizing LLMs for Efficiency without Performance Loss
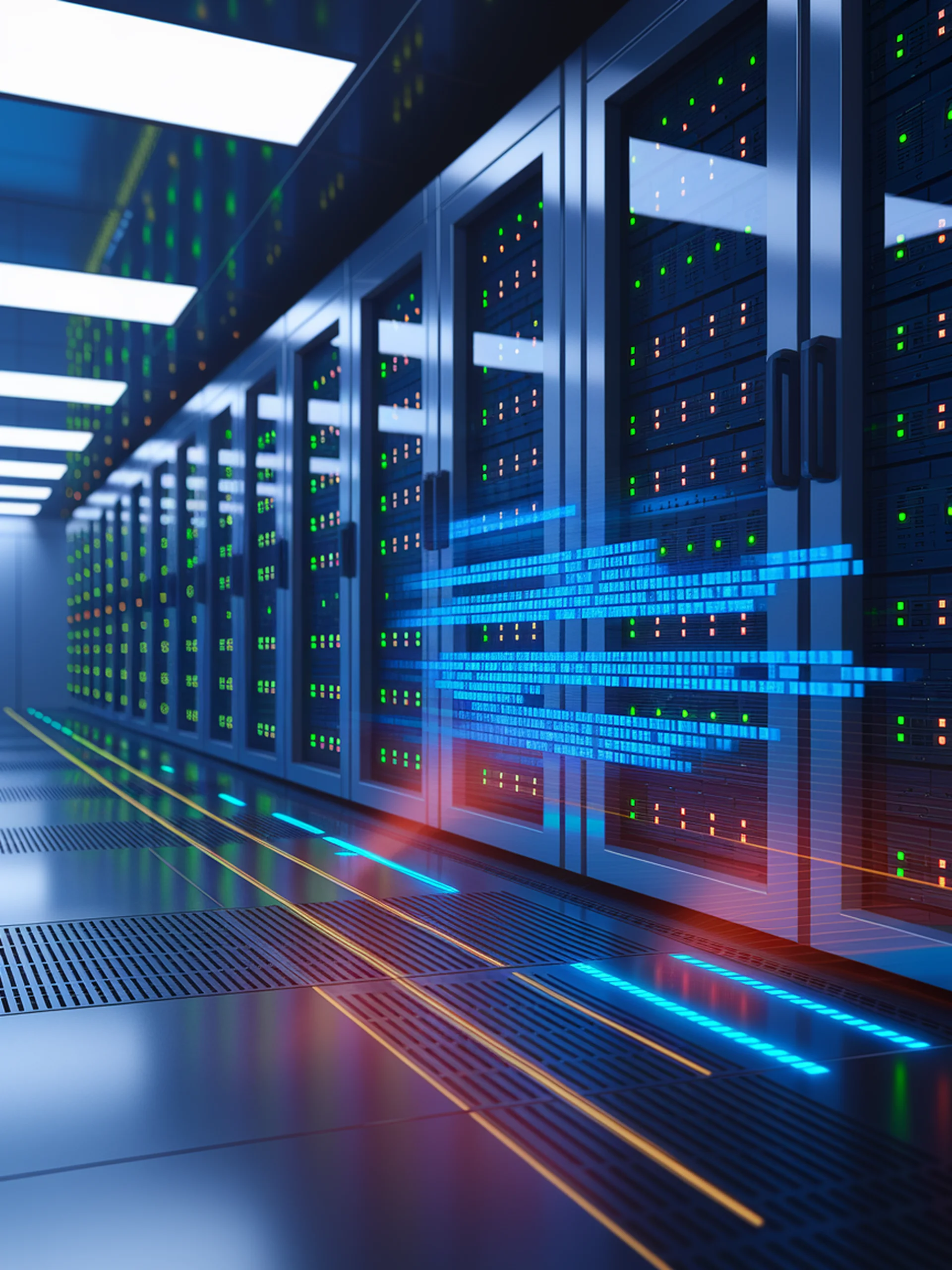
Optimizing LLM Serving with Slice-Level Scheduling
Achieving Higher Throughput and Better Load Balancing for AI Applications
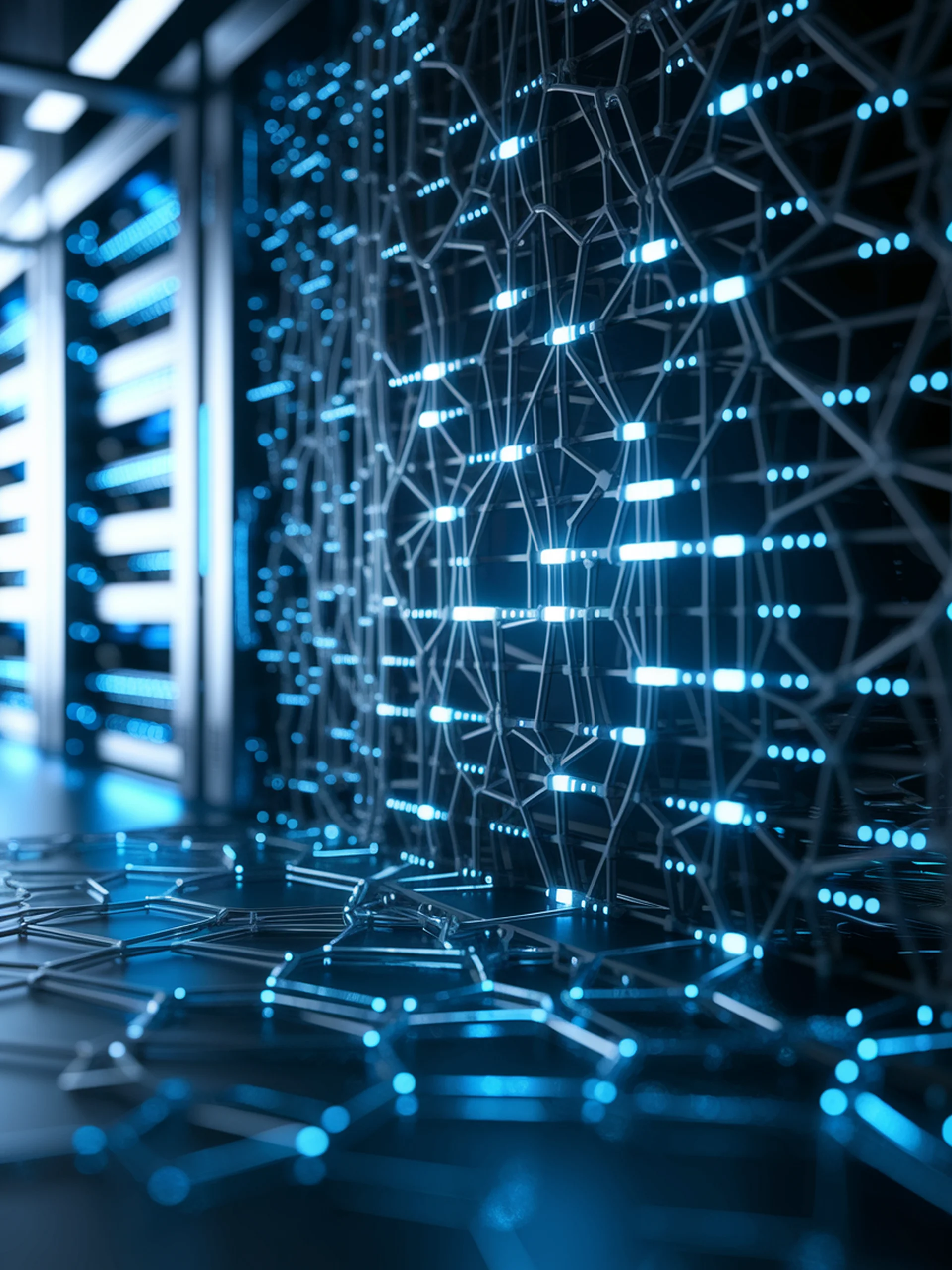
Smarter, More Efficient LLM Fine-Tuning
A gradient-based approach to selective parameter updates
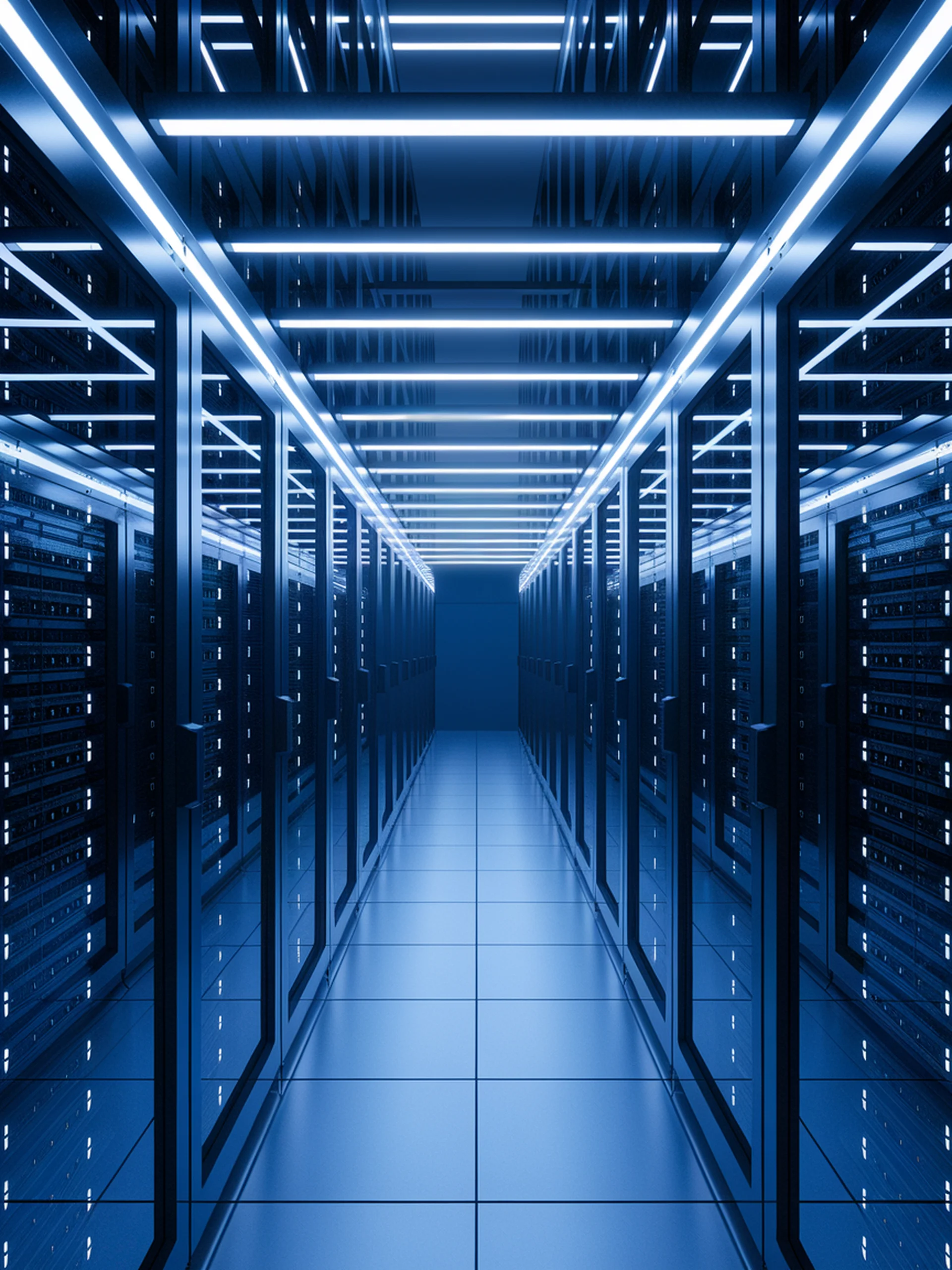
Extending LLM Context Windows Exponentially
A Training-Free Solution for Long-Sequence Processing
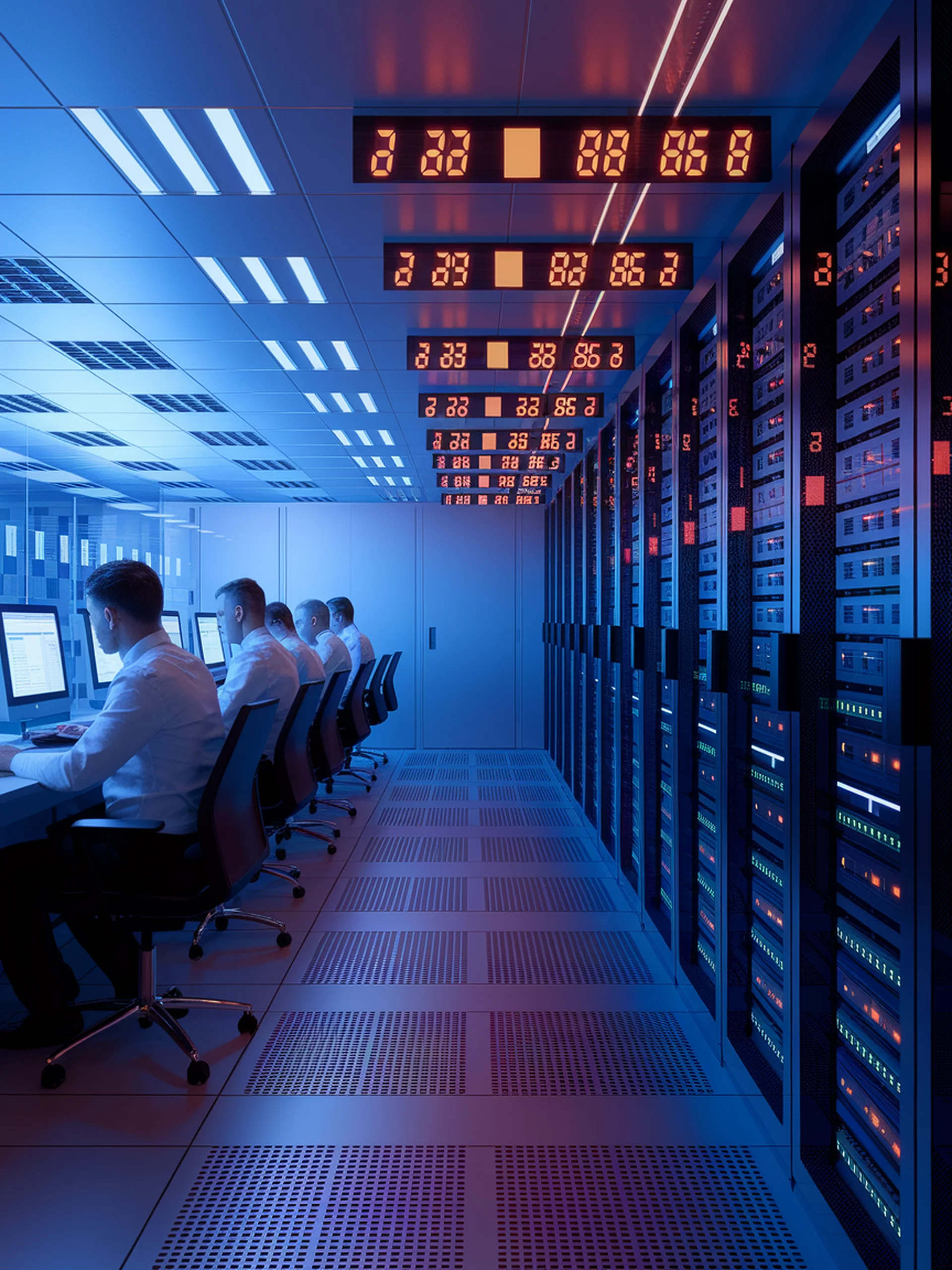
Optimizing LLM Serving with Smart Queue Management
Balancing Interactive and Batch Requests for Improved Resource Utilization
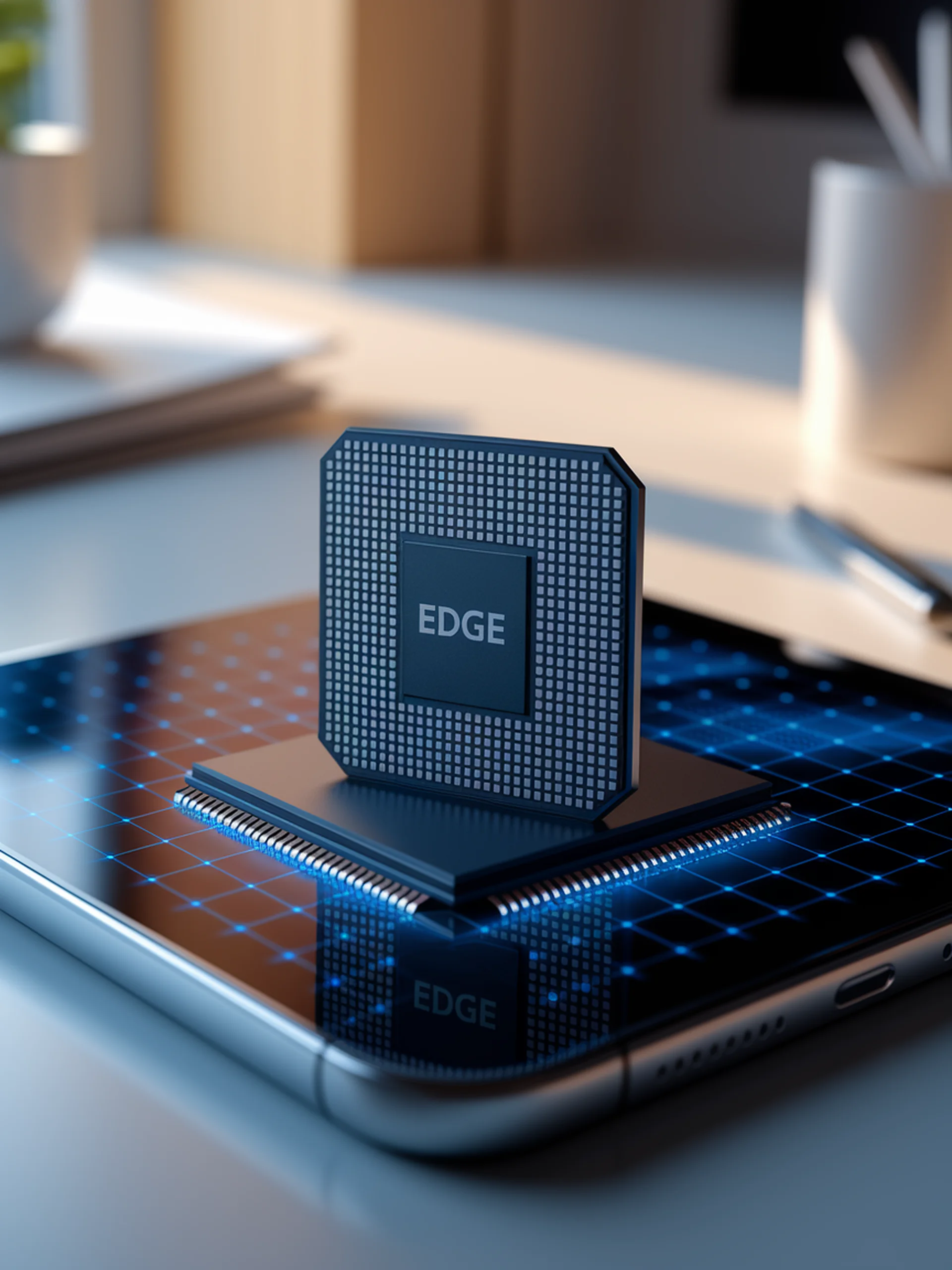
Reviving CPU Performance for Edge AI
T-MAC: A Table-Based Approach to Run Low-Bit LLMs on Edge Devices
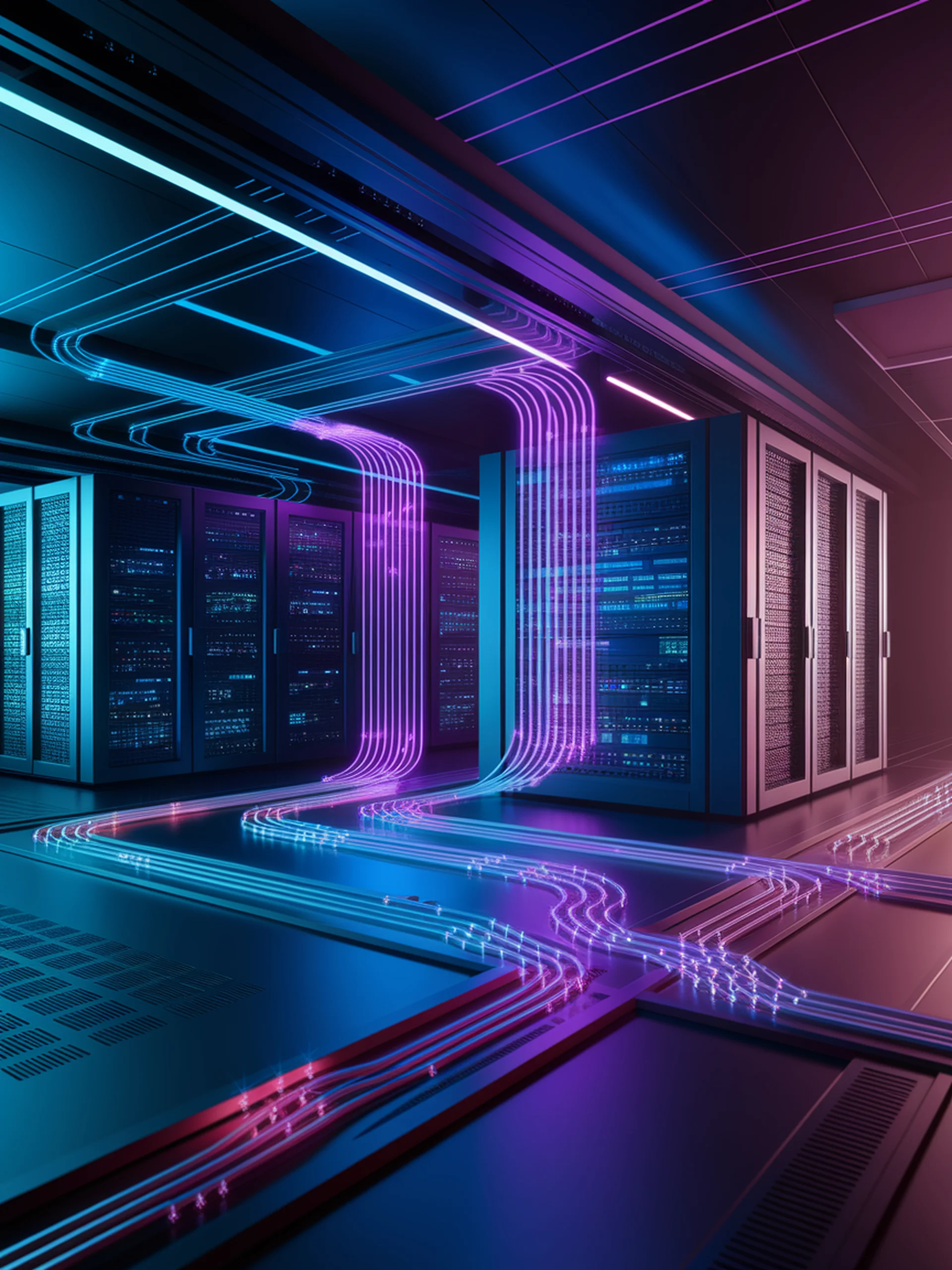
Reinventing LLM Workflow Optimization
Breaking the Module Barrier for End-to-End Performance
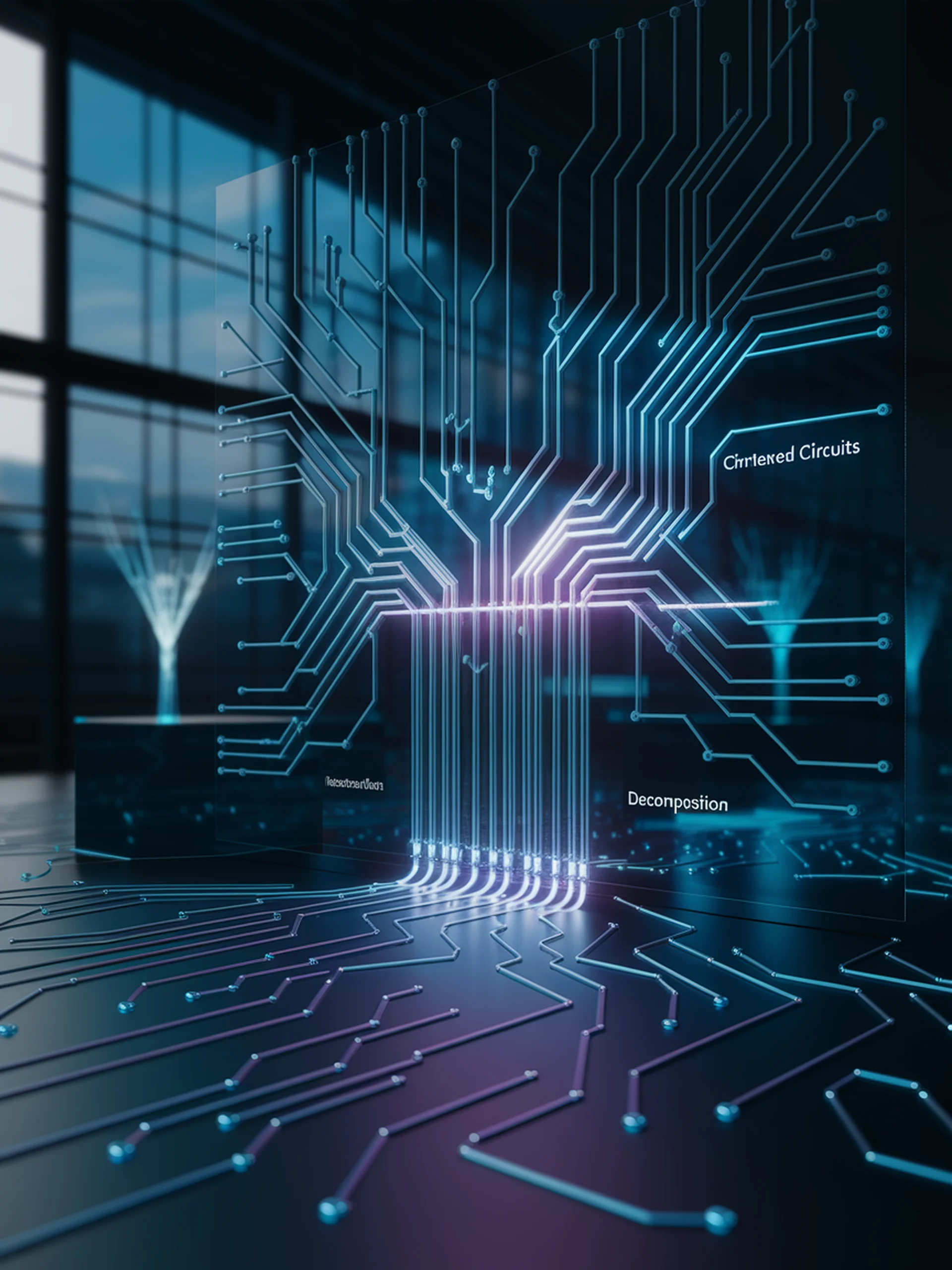
Faster Circuit Discovery in Transformers
Accelerating Mechanistic Interpretability with Contextual Decomposition
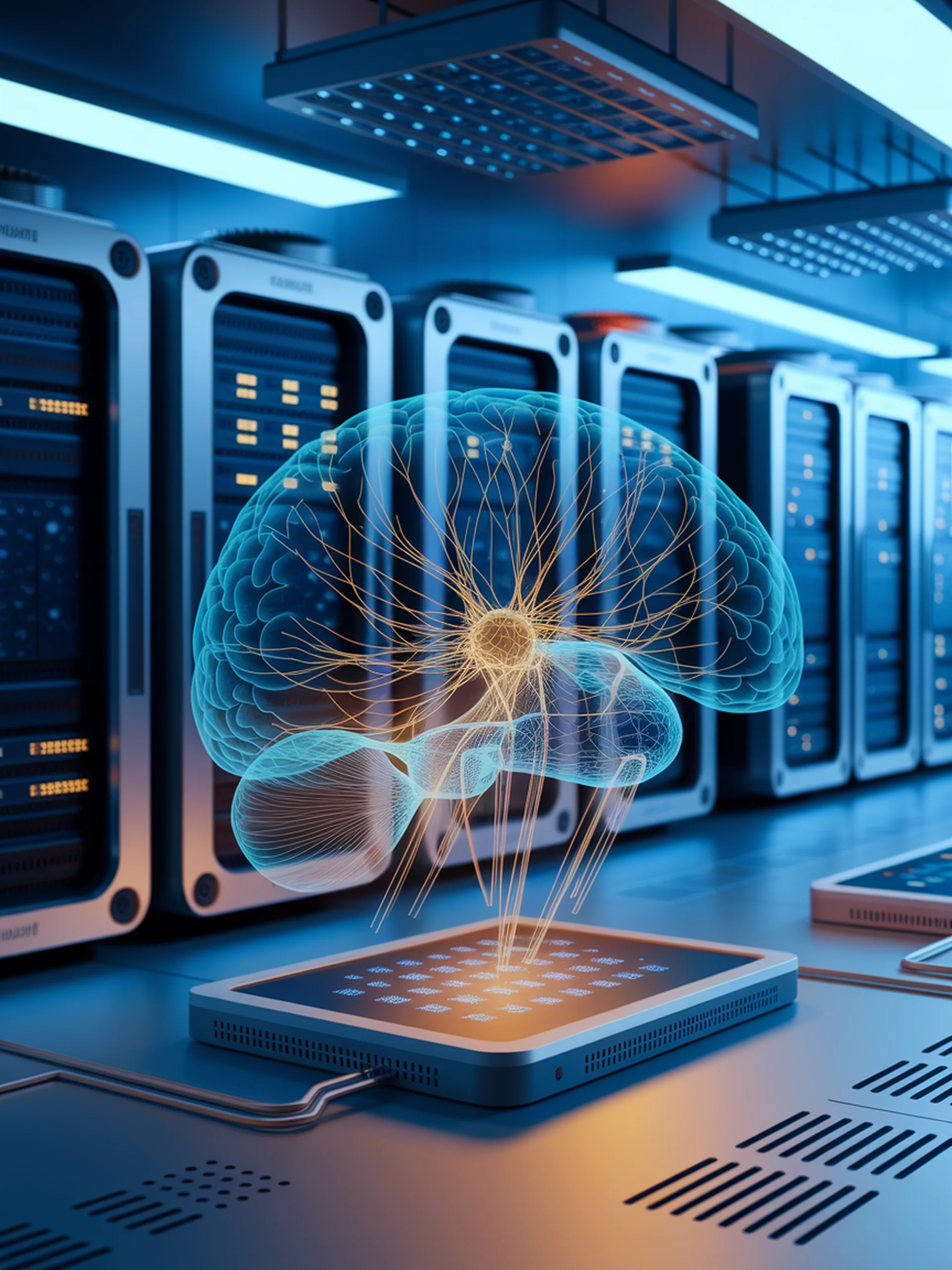
Brain-Inspired Efficiency for LLMs
Scaling Spiking Neural Networks to Billion-Parameter Models
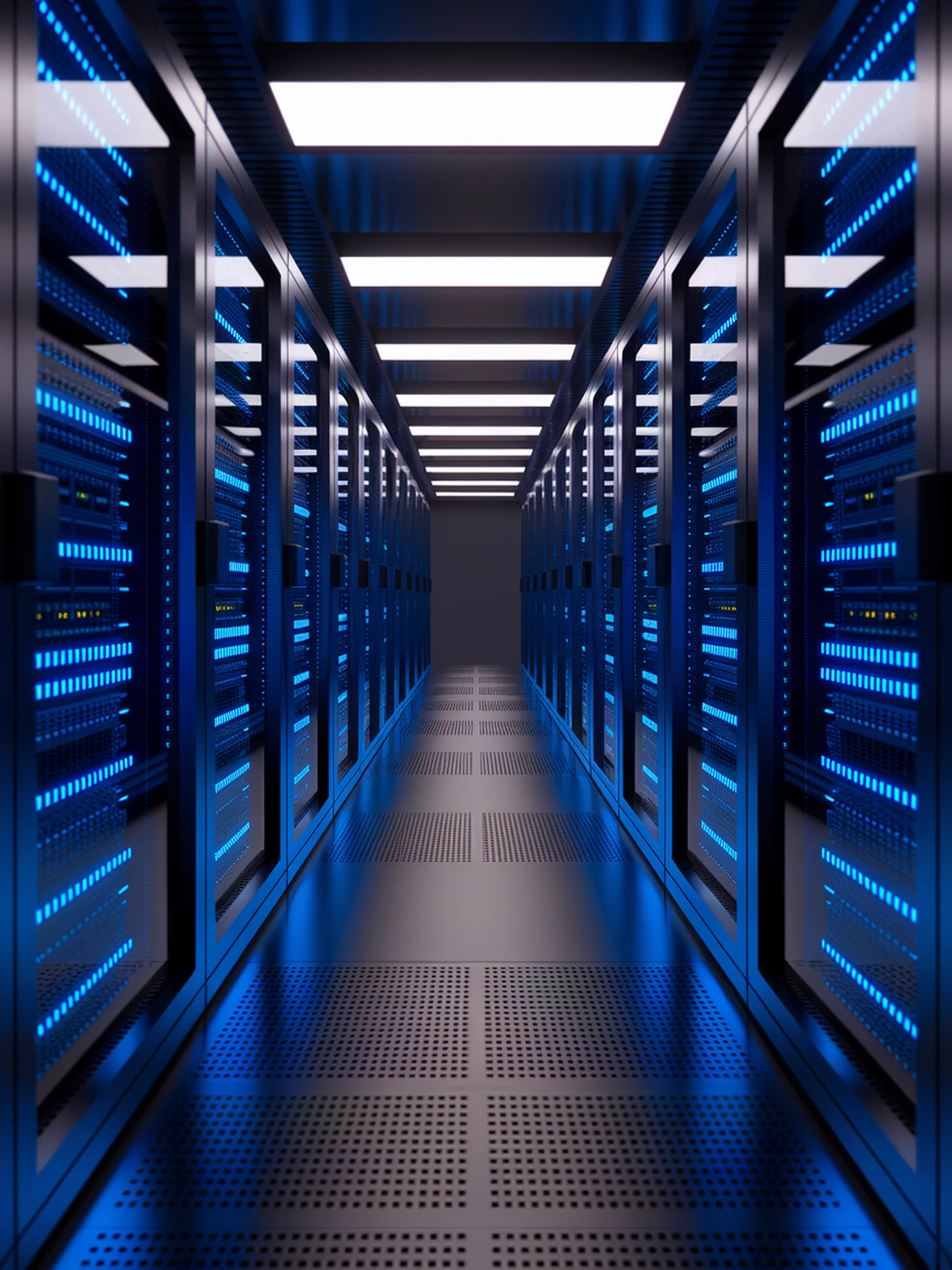
Accelerating LLM Inference
Optimizing multi-token decoding for faster, better responses
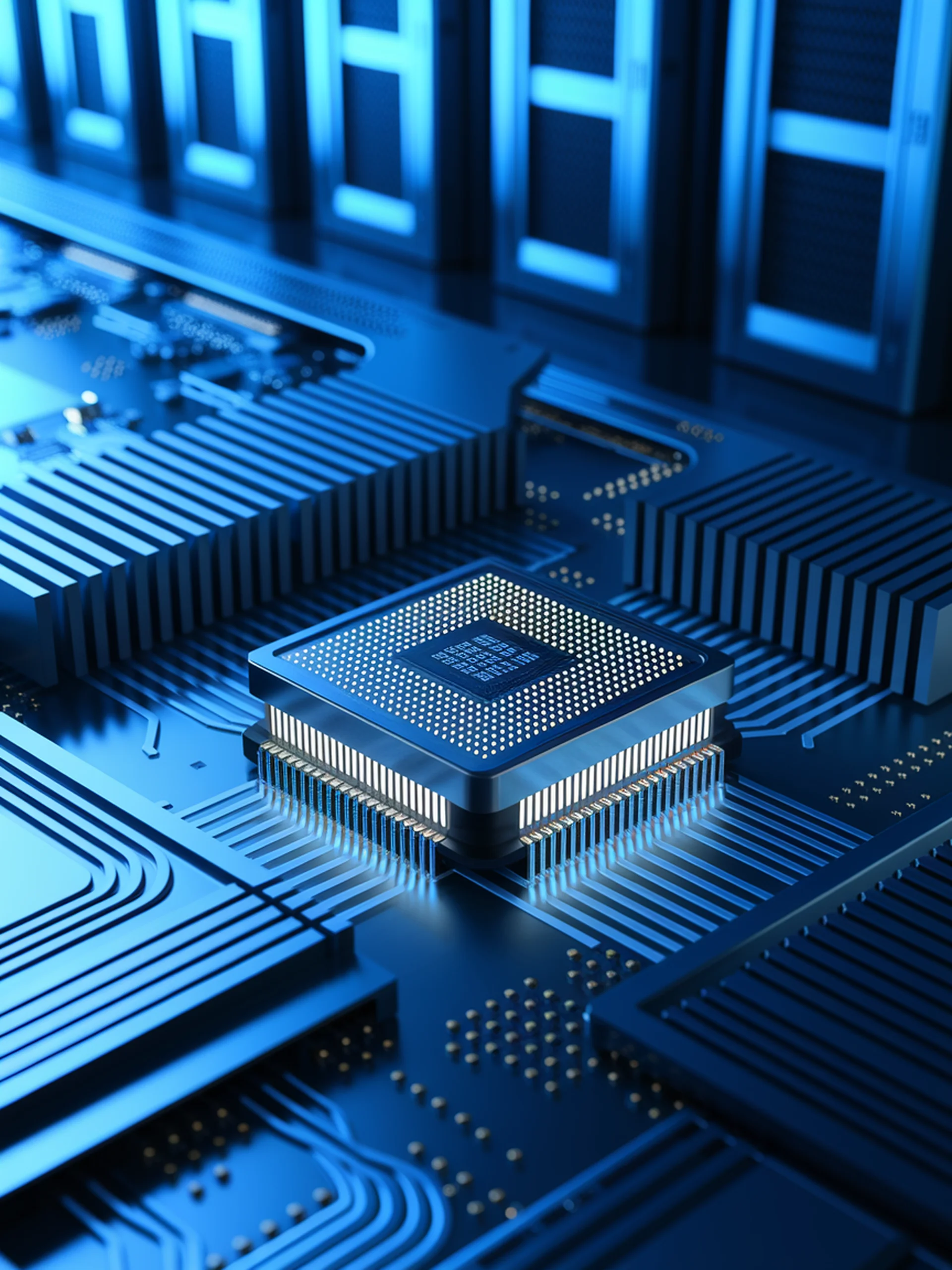
Smarter LLM Compression
Novel quantization technique maintains accuracy while reducing model size
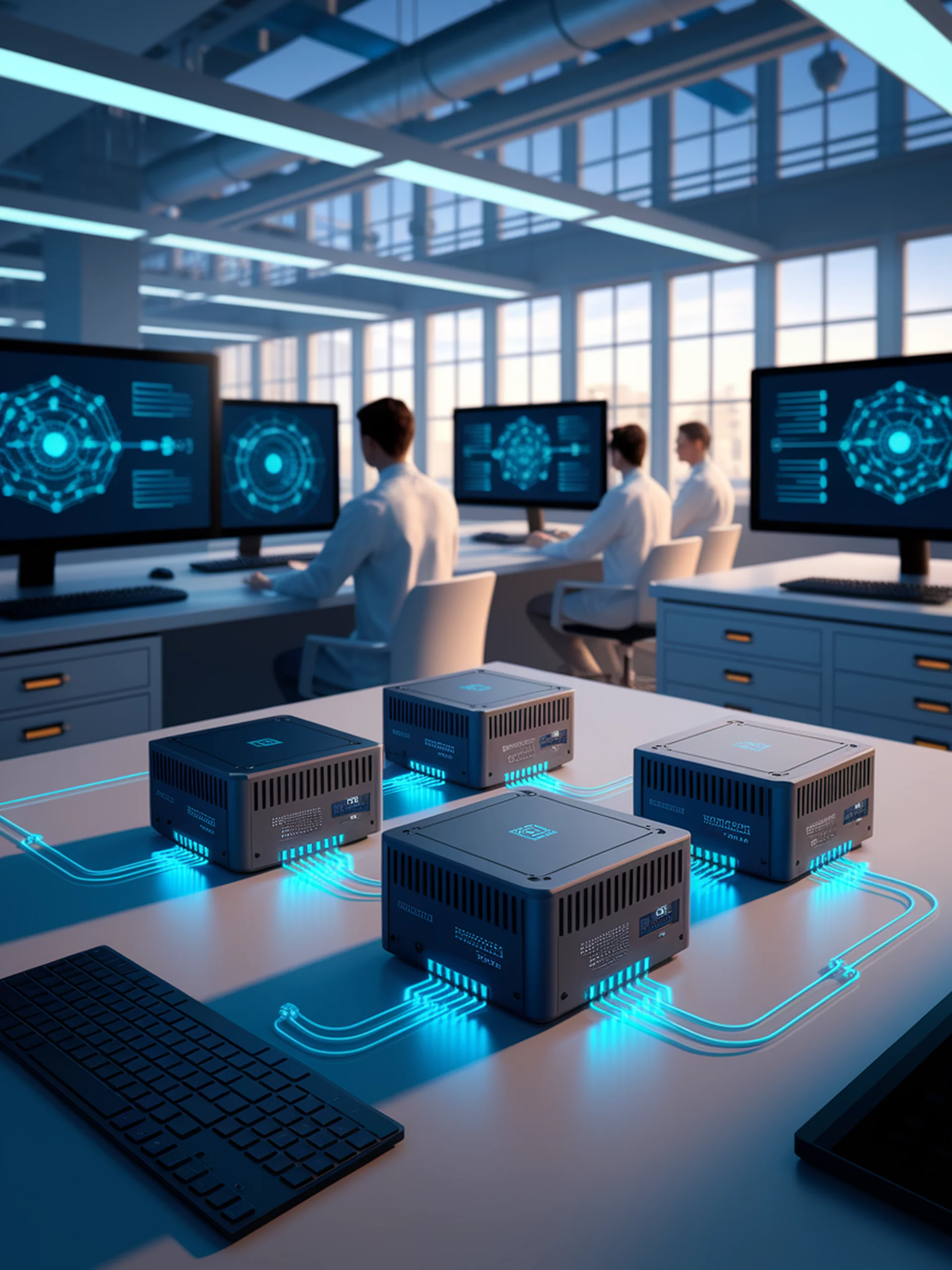
Efficient Multi-Expert LLMs for Limited Resources
How CCoE enables powerful language models on constrained hardware
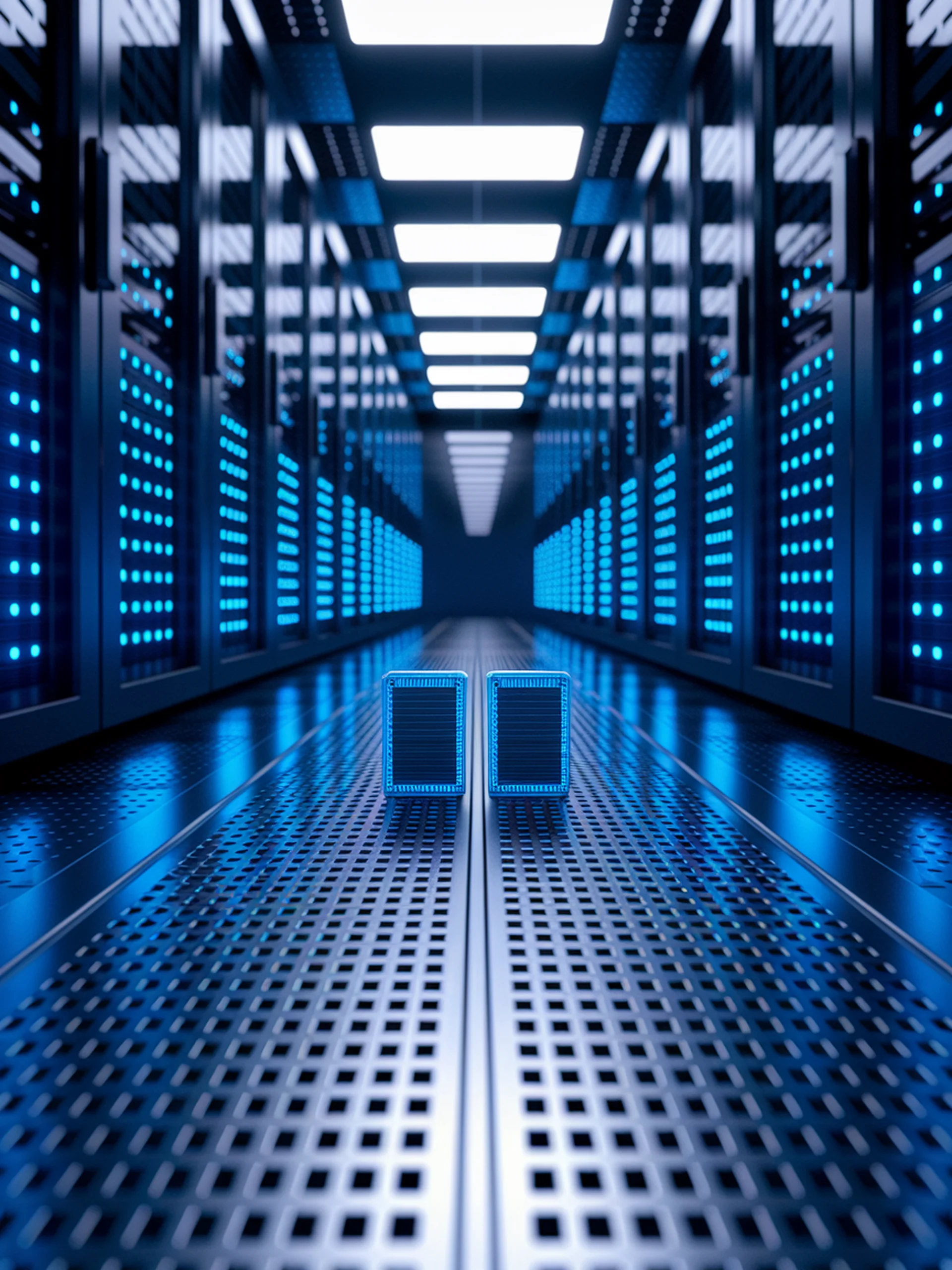
Optimizing LLM Memory for Longer Contexts
Product Quantization Reduces KVCache Memory by 75% with Minimal Quality Loss
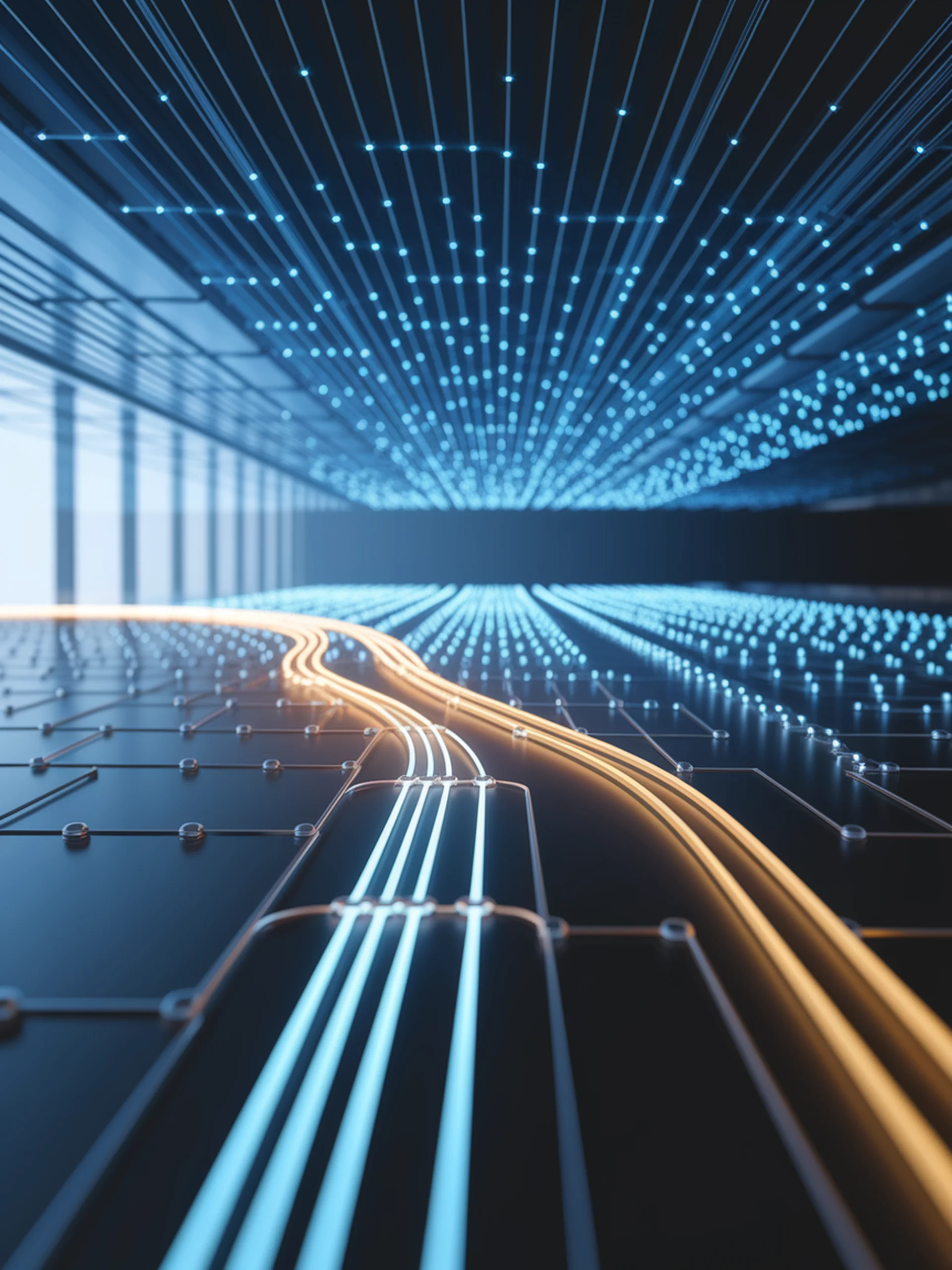
Infinite Context from Finite Attention
Breaking LLM context length barriers without retraining
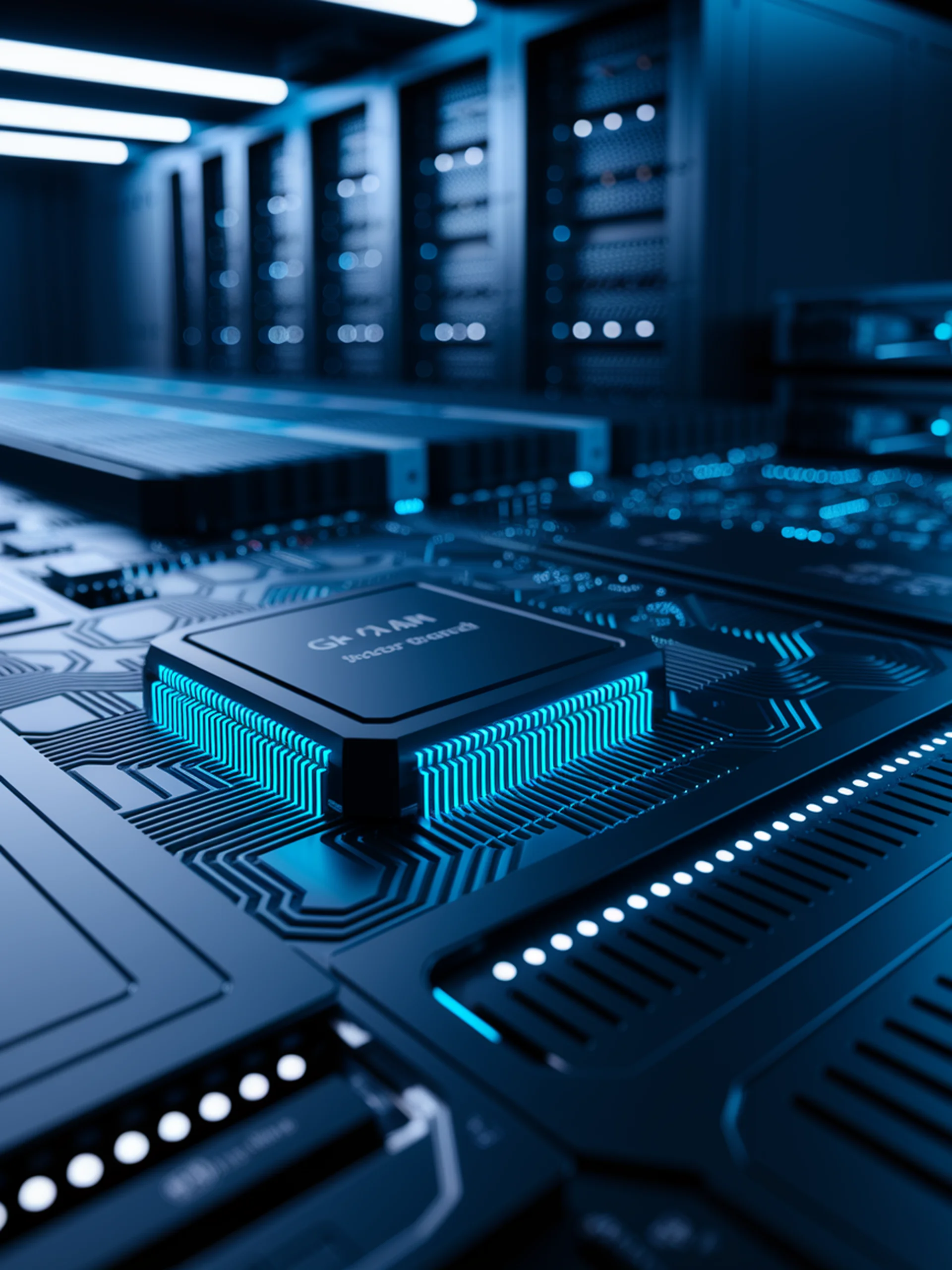
Accelerating LLMs with Smarter Attention
Hardware-Aware Sparse Attention That Actually Delivers Speed Gains
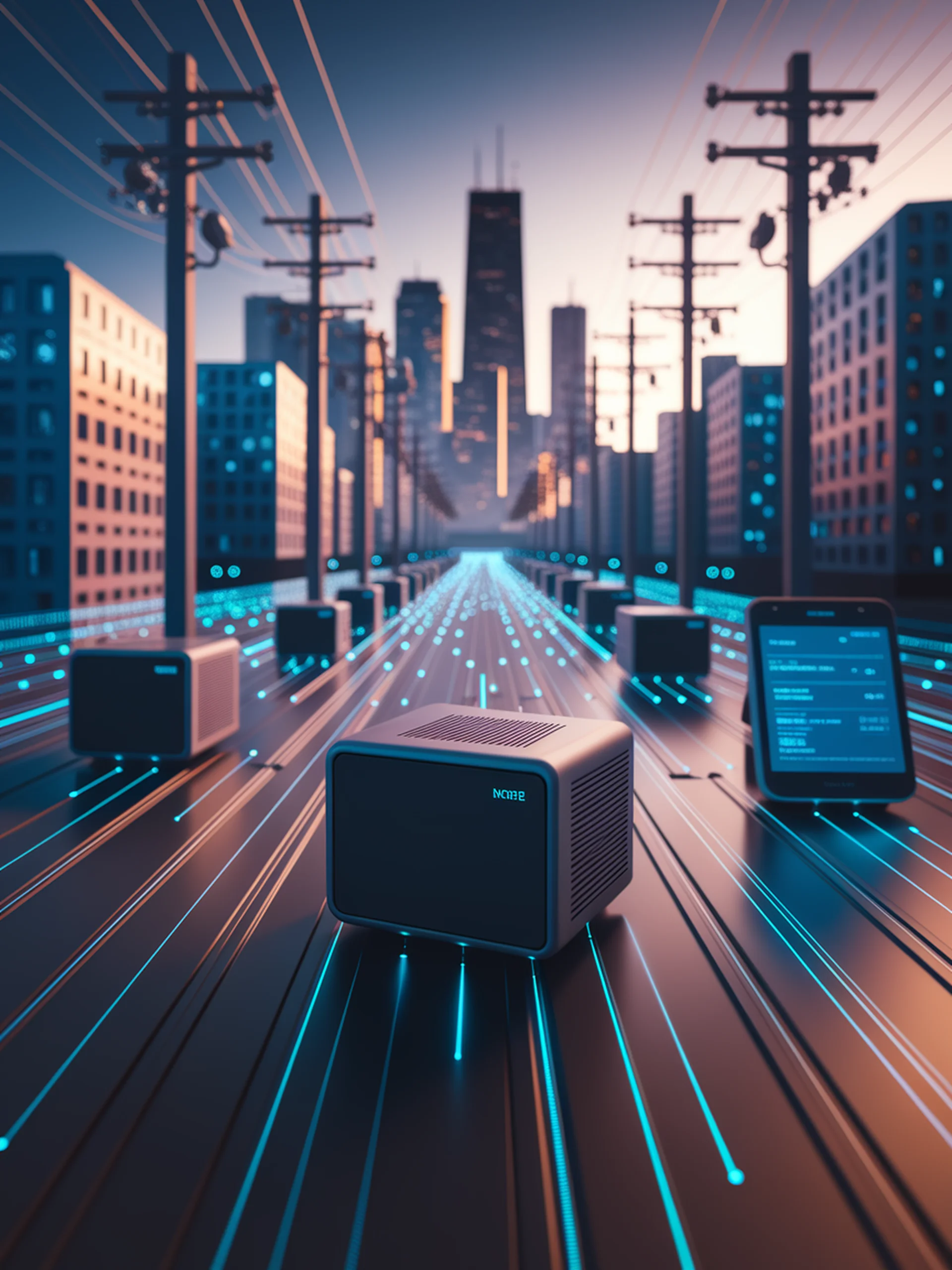
Edge Intelligence for LLMs
Bridging the Gap Between Cloud and On-Device AI
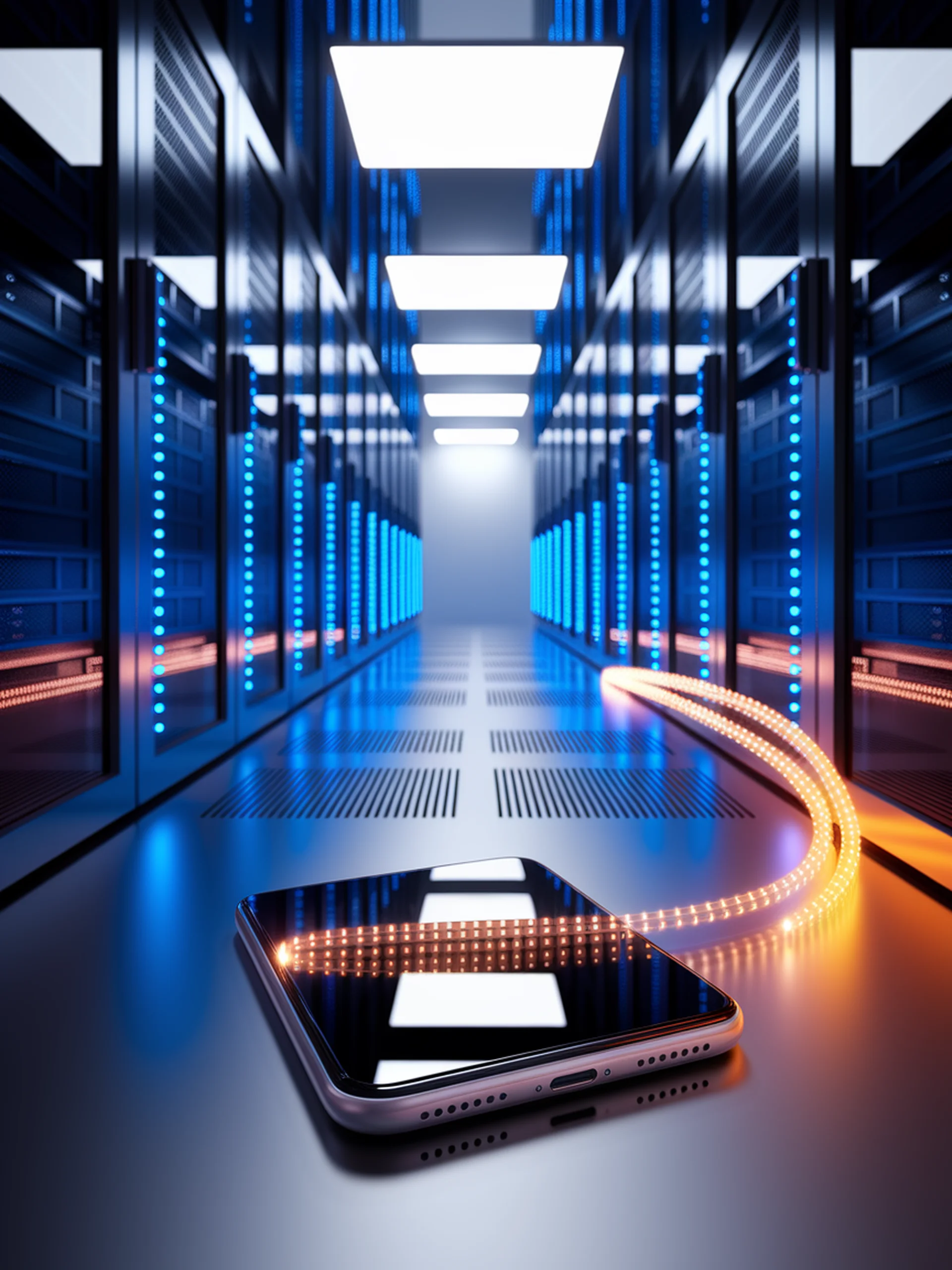
Optimizing Image Transfer for Cloud-Based MLLMs
A novel framework for efficient compressed image latents
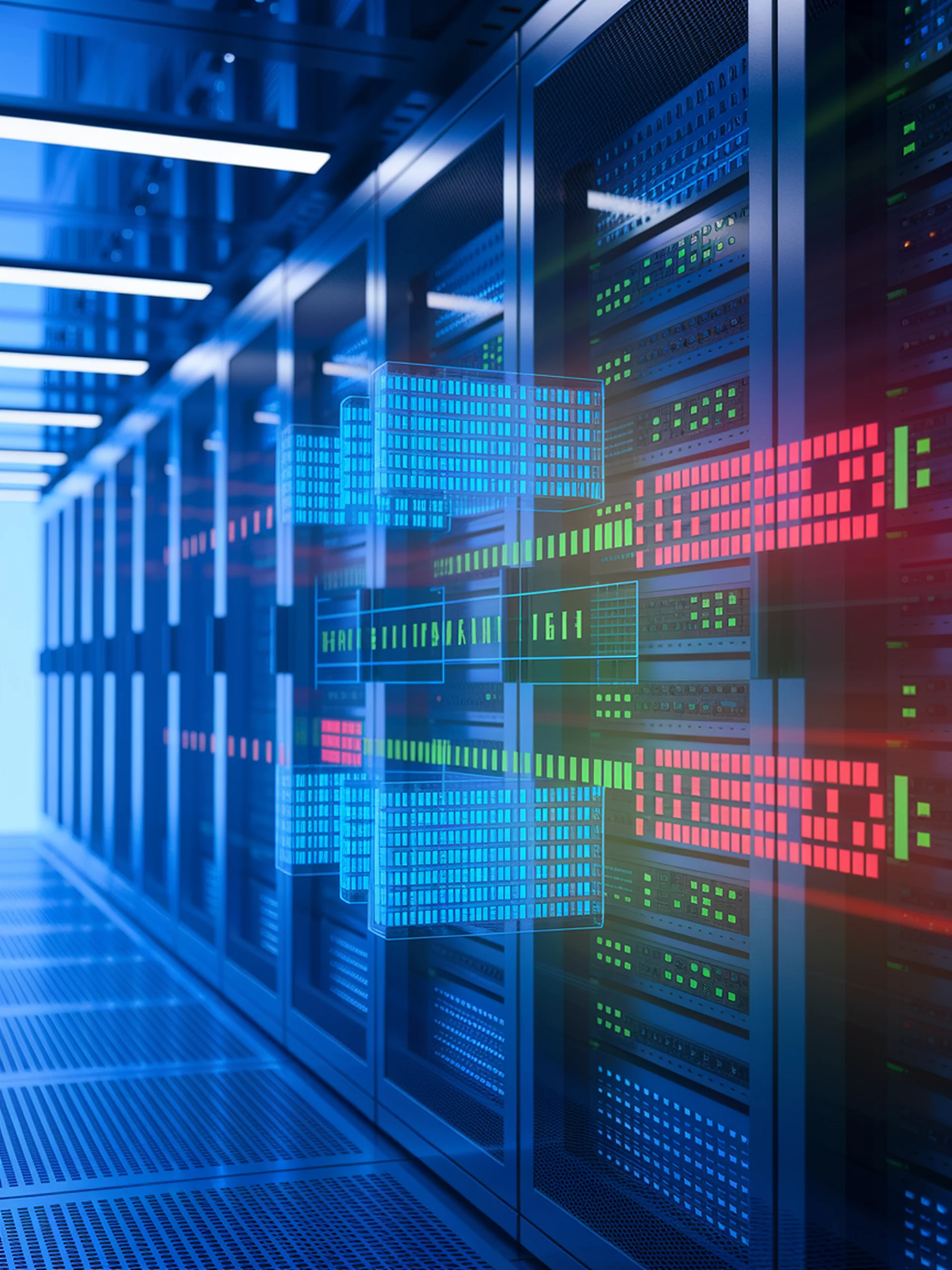
Streamlining LLM Memory Consumption
Query-driven pruning for efficient KV cache management
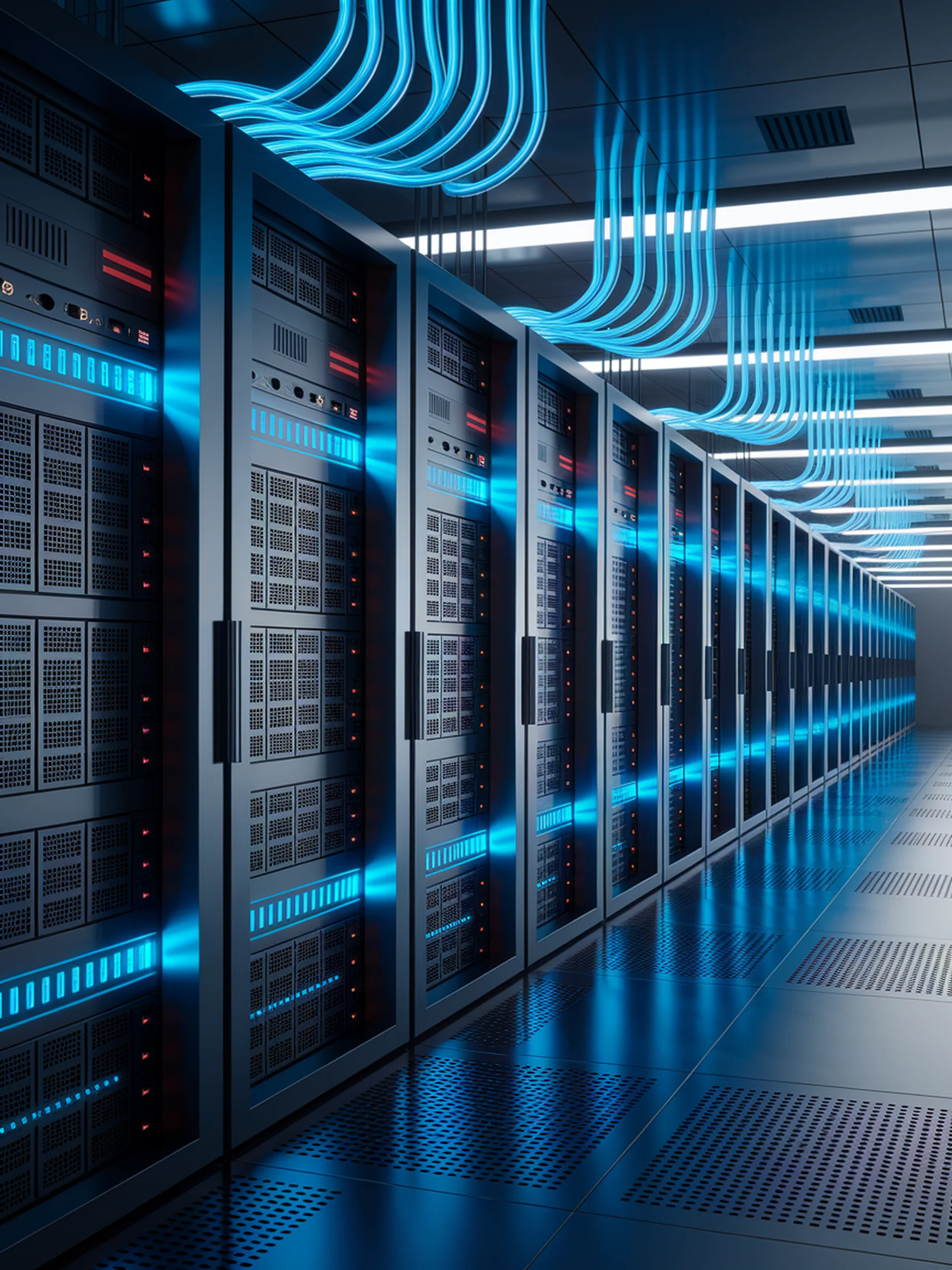
Memory Optimization for LLM Inference
Network-Accelerated Memory Offloading for Scalable GPU Deployments
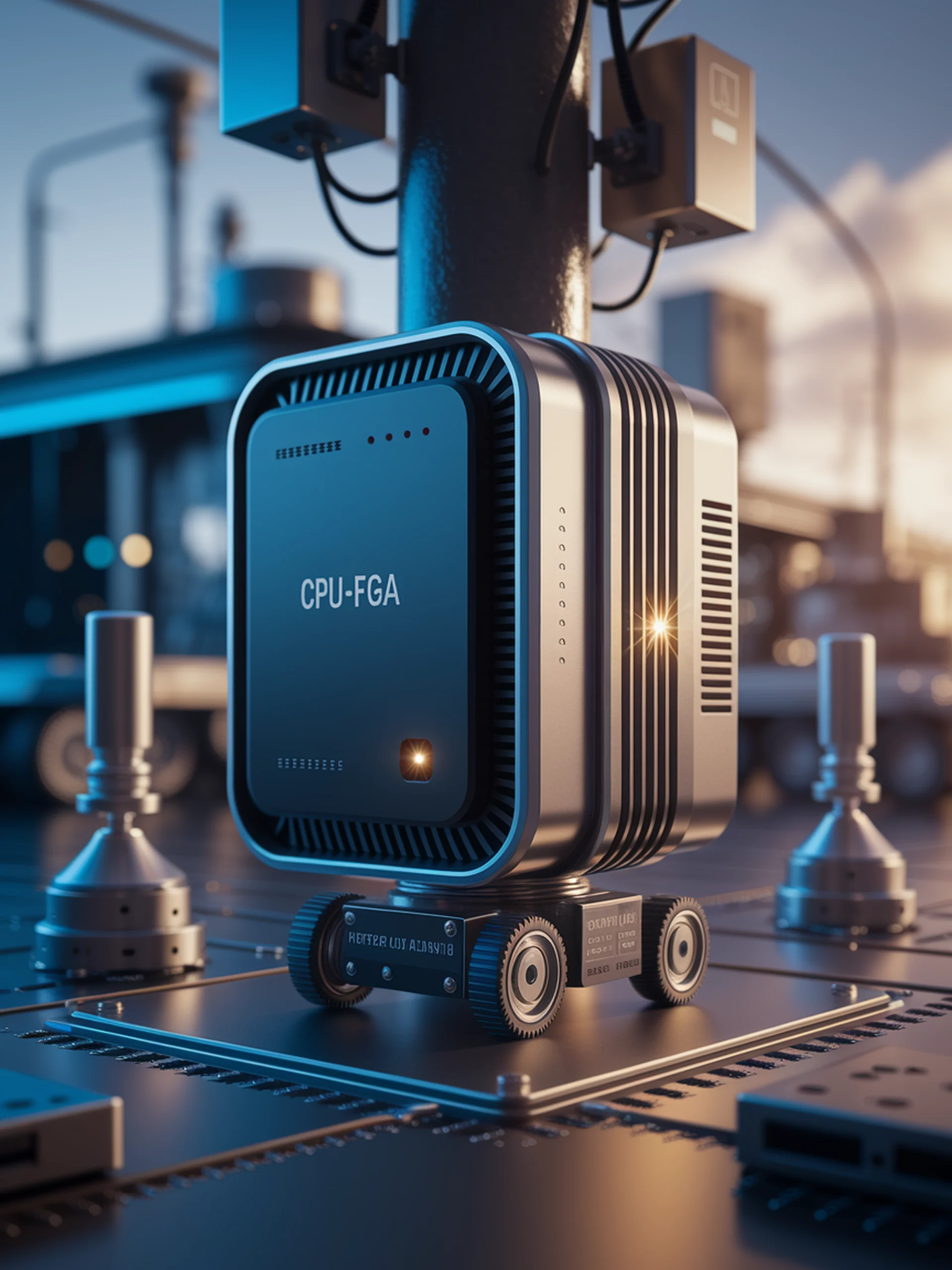
EdgeLLM: Bringing LLMs to Resource-Constrained Devices
A CPU-FPGA hybrid solution enabling efficient edge deployment
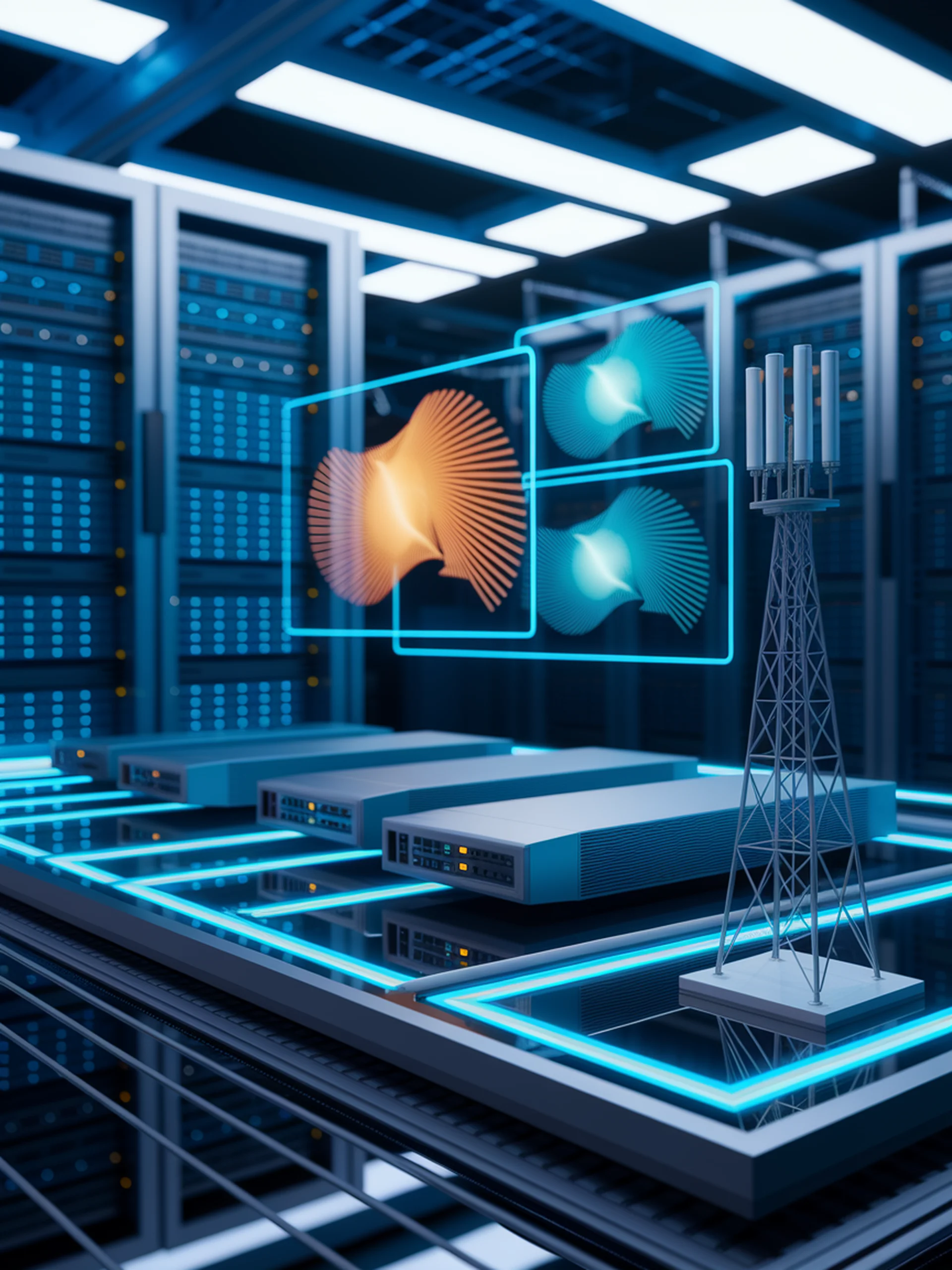
Optimizing GAI for 6G Networks
Task Offloading Framework via In-context Learning
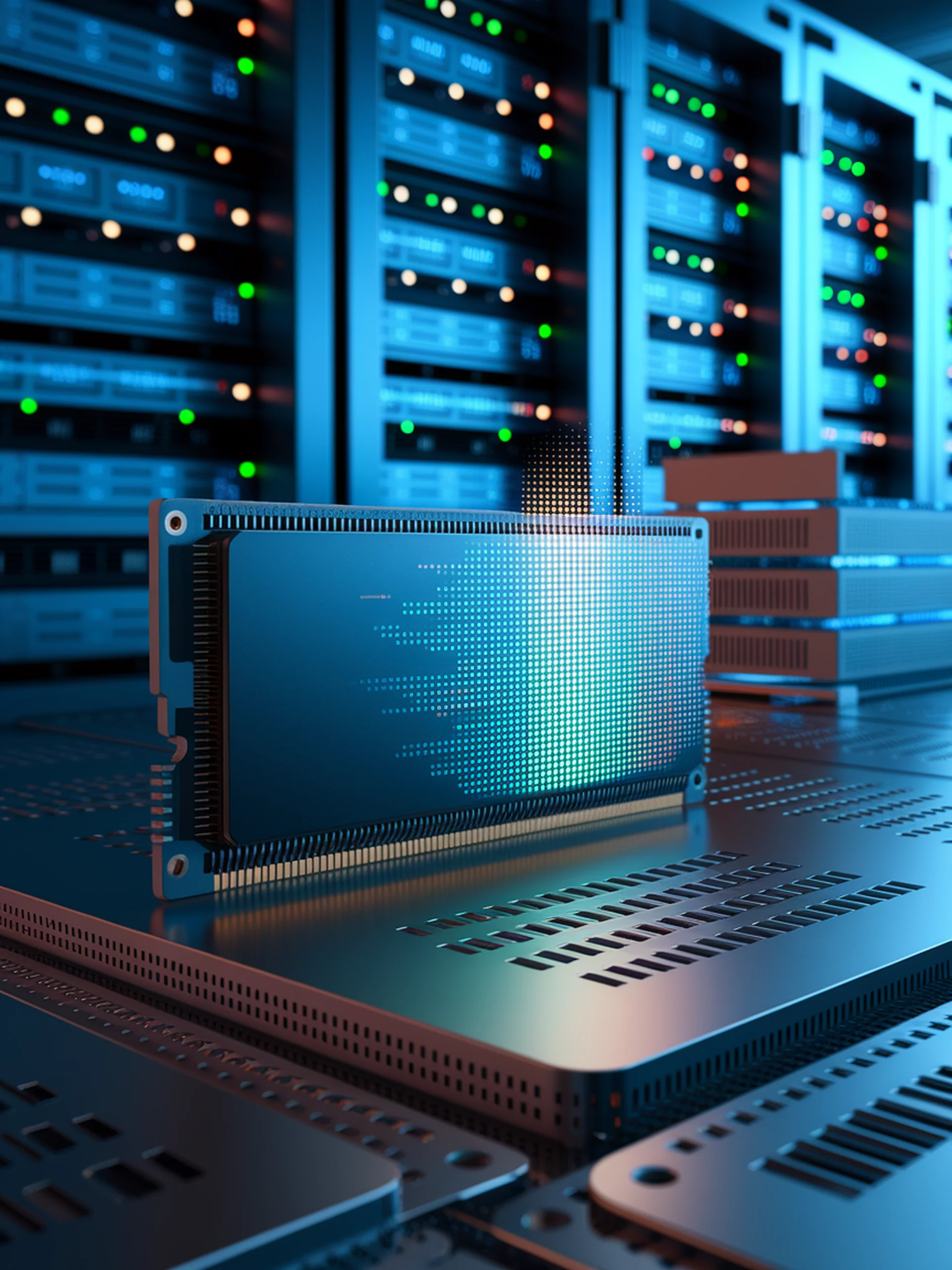
Accelerating LLMs with Zero-Overhead Memory Compression
Solving the Key-Value Cache bottleneck for faster inference
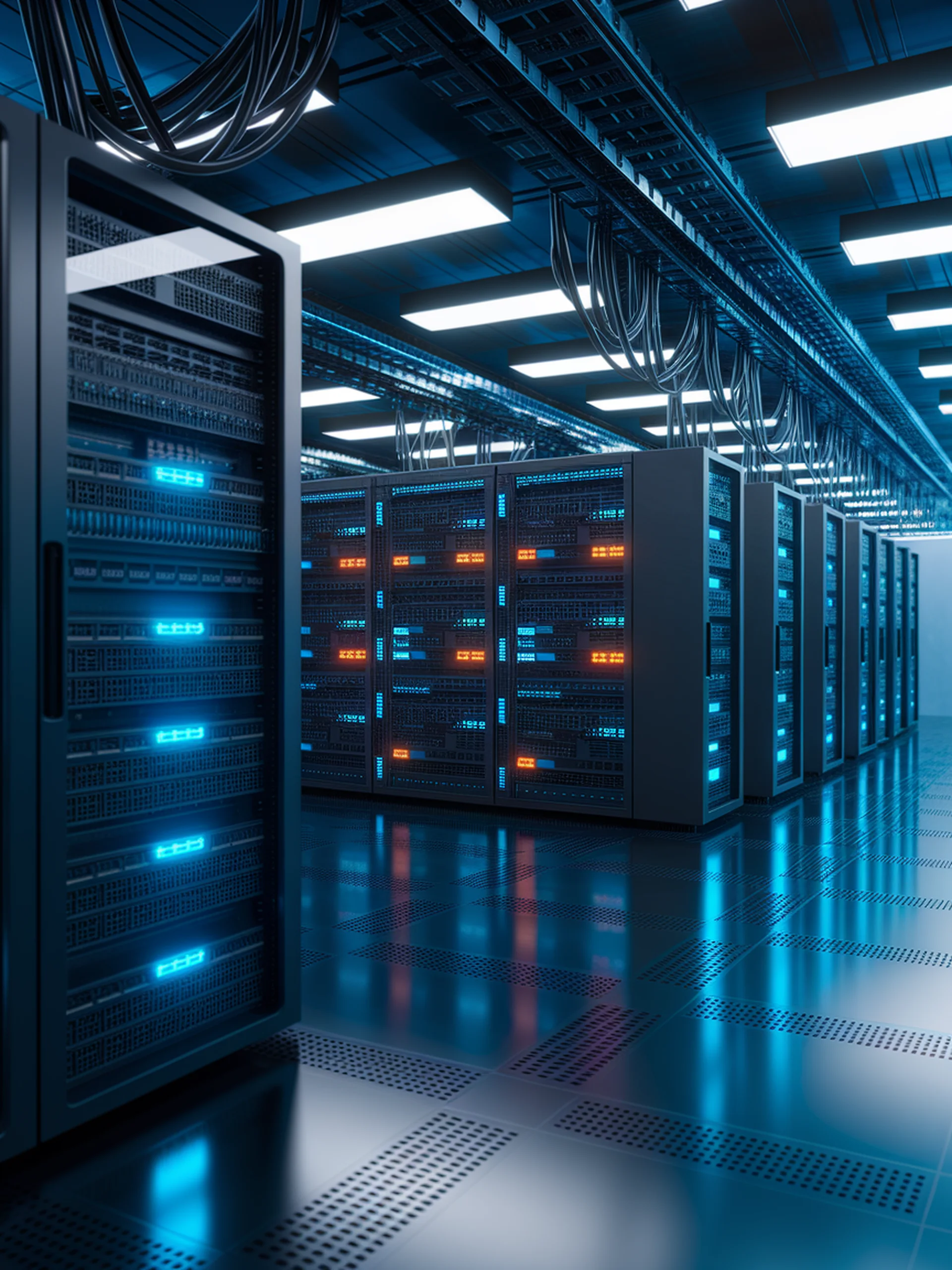
Building Resilient AI Infrastructure
Efficient Fault Tolerance for Sparse MoE Model Training
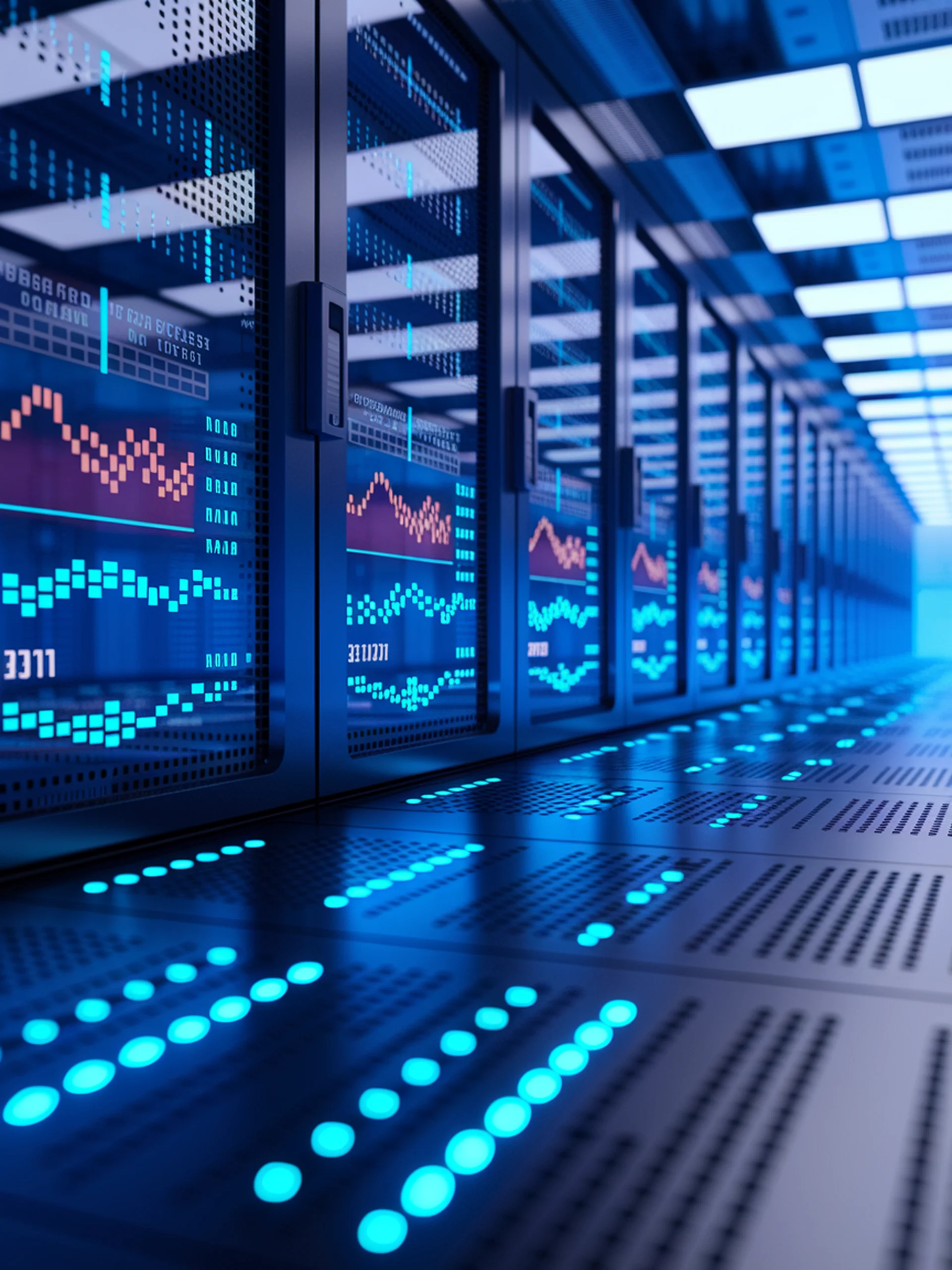
Optimizing LLM Performance for Business Applications
Intelligent Performance Tuning for Large Language Model Services
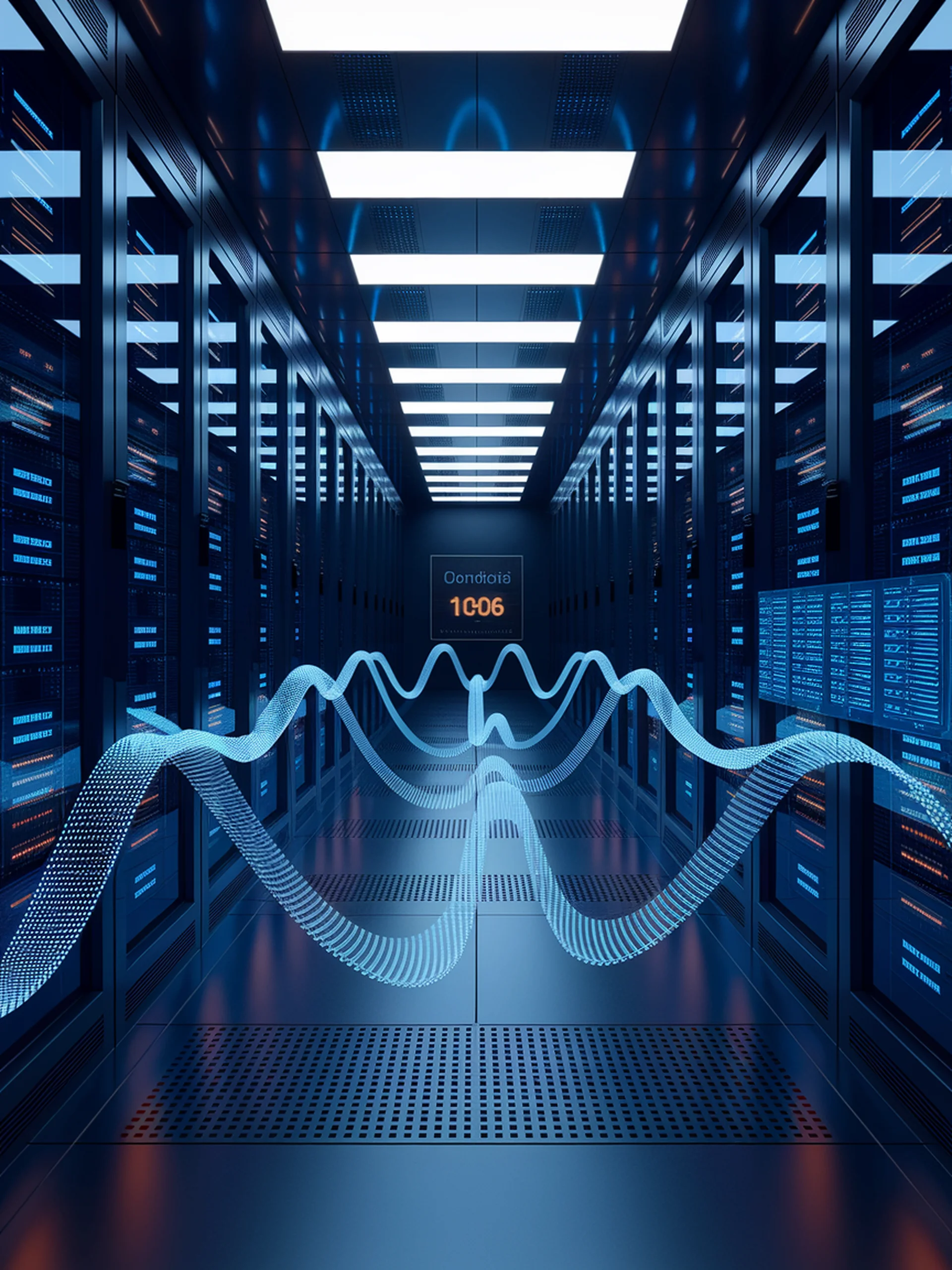
Accelerating LLM Generation with Diffusion
Overcoming the bottlenecks of traditional speculative decoding
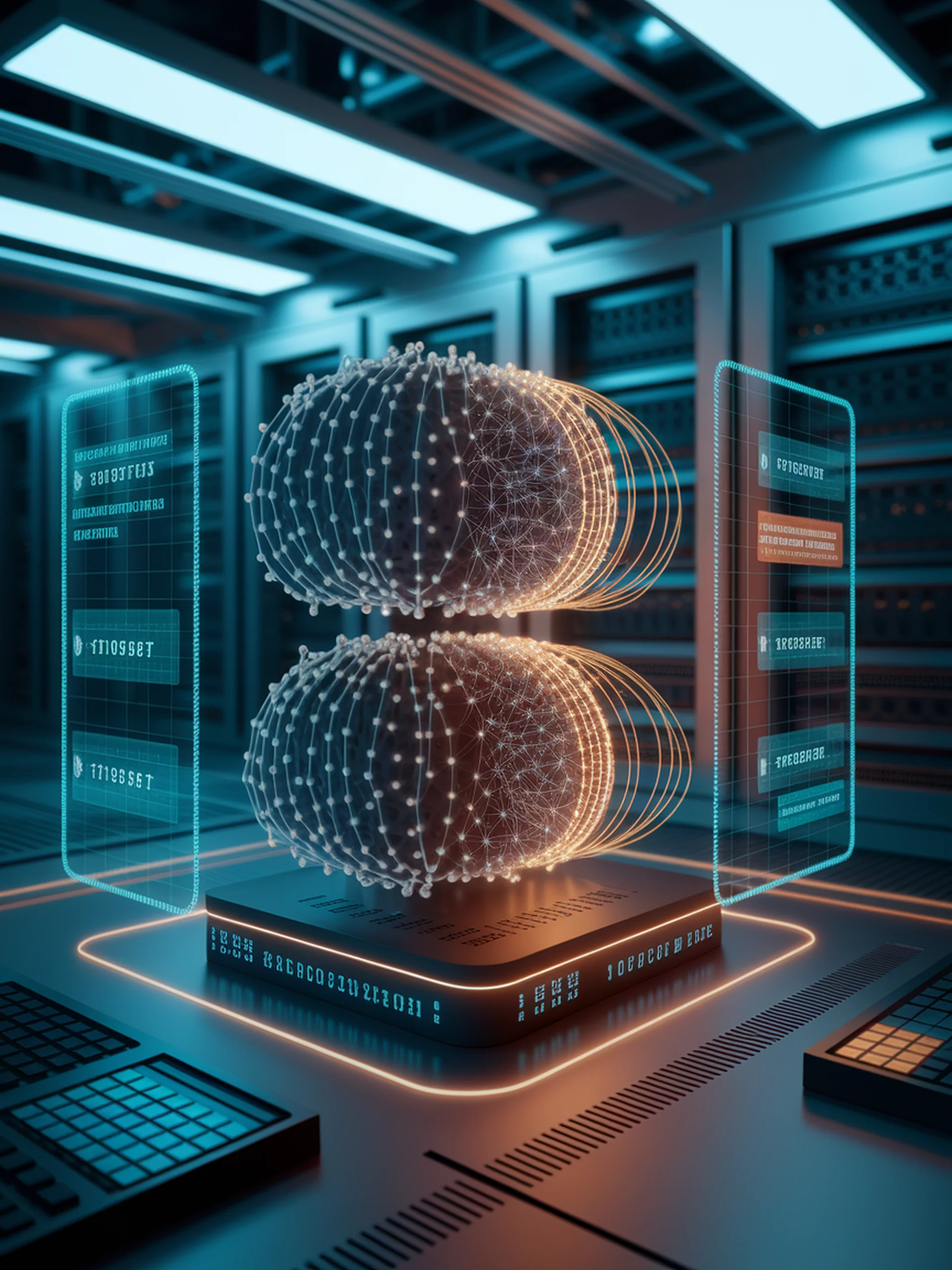
Shrinking Giants: Efficient LLM Compression
A modular approach that reduces model size without sacrificing performance
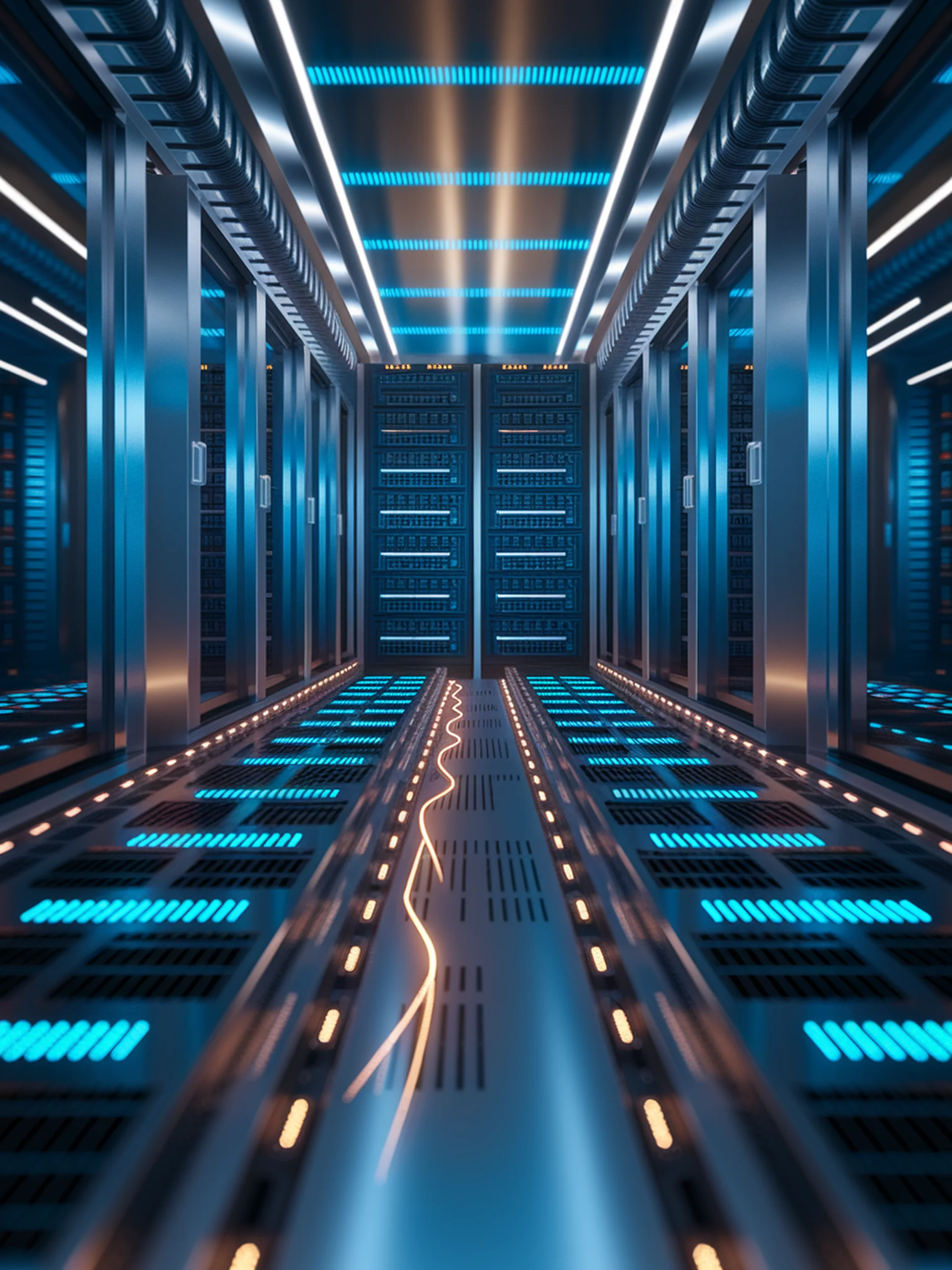
Breaking Memory Barriers in LLM Training
Offloading activations to SSDs for faster, more efficient model training
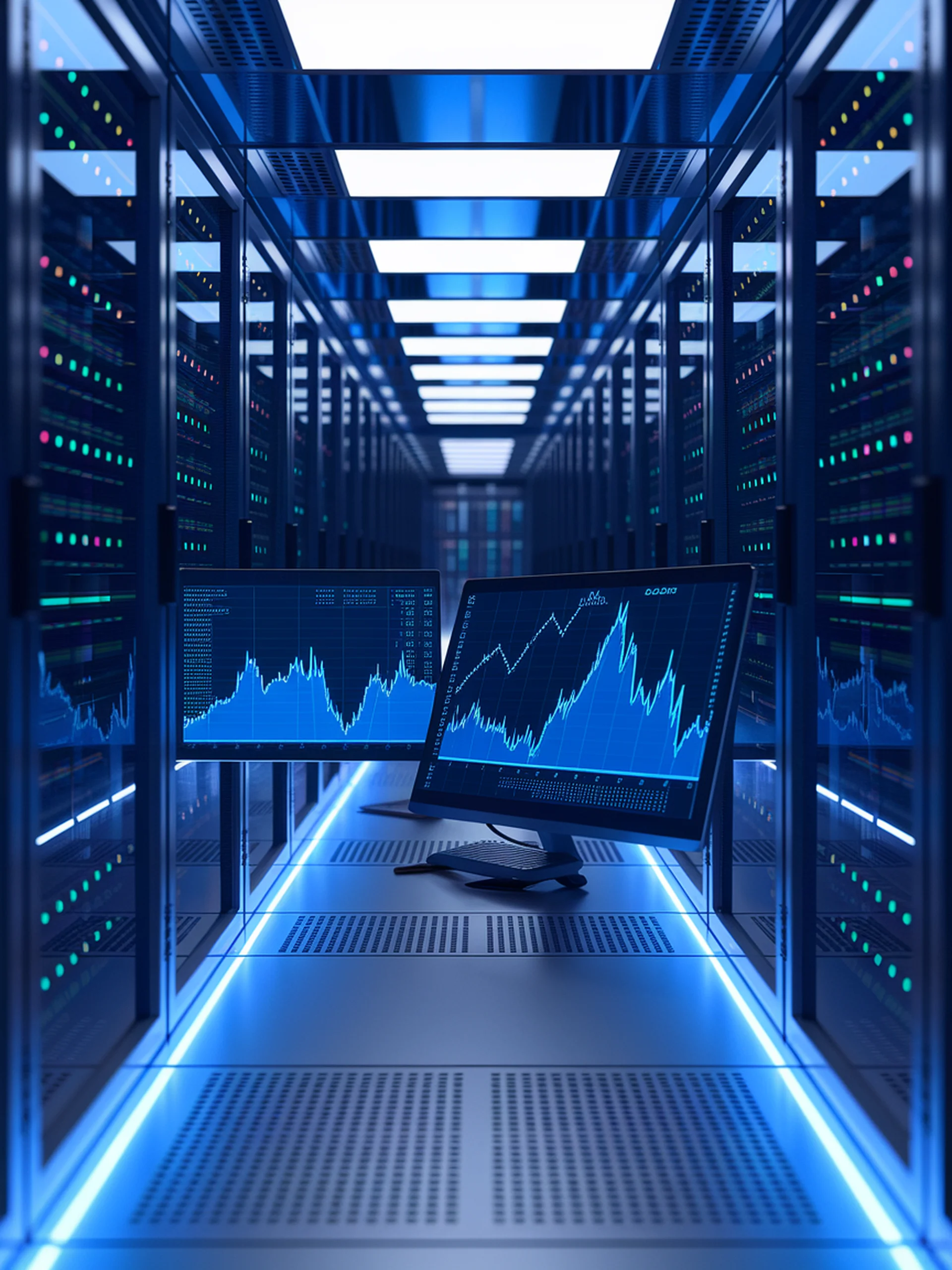
Breaking the Latency-Throughput Barrier
Optimizing Long-Context LLM Performance with MagicDec
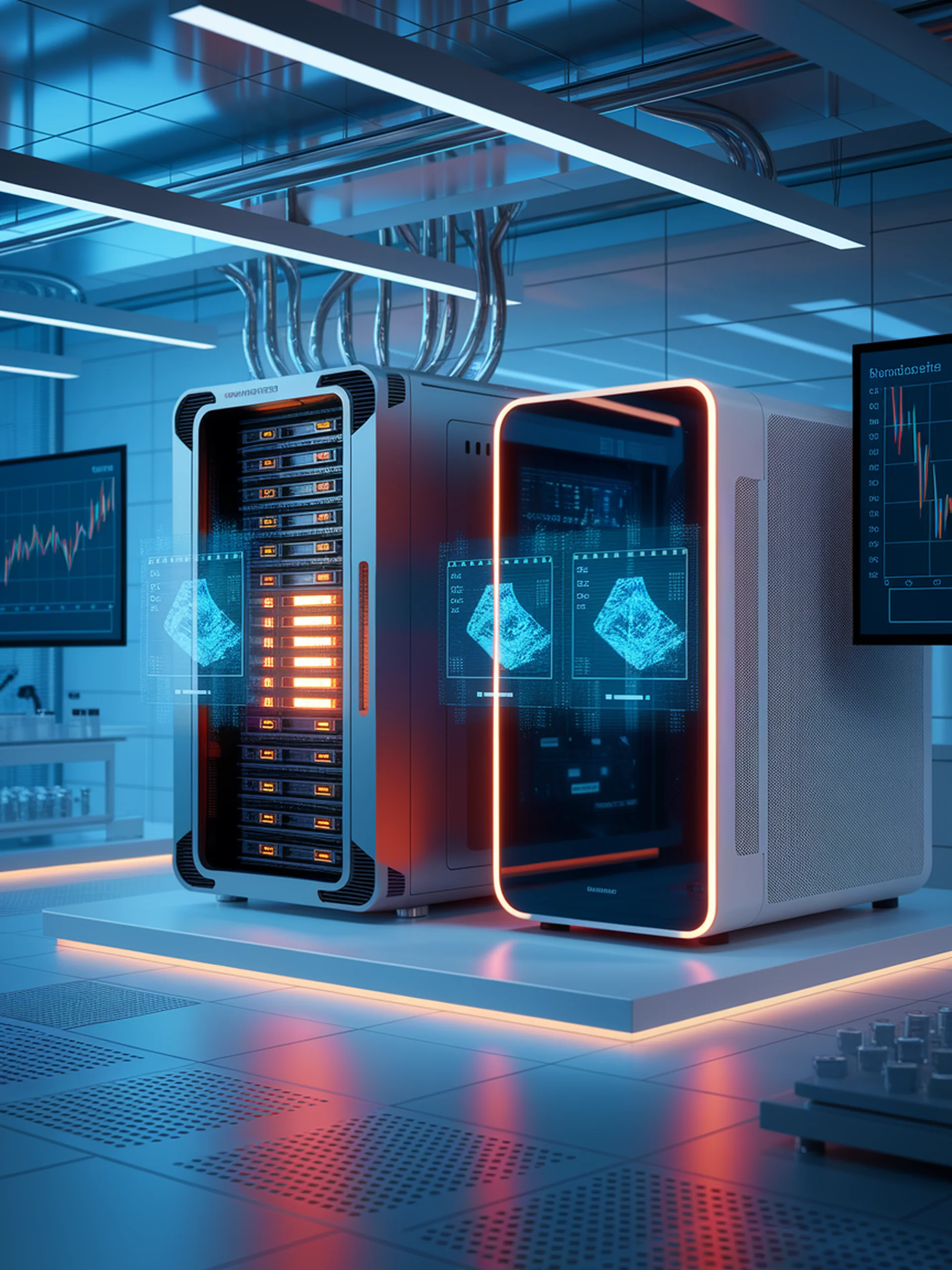
Balancing Efficiency in Multimodal LLMs
A novel approach that optimizes both data and computational resources
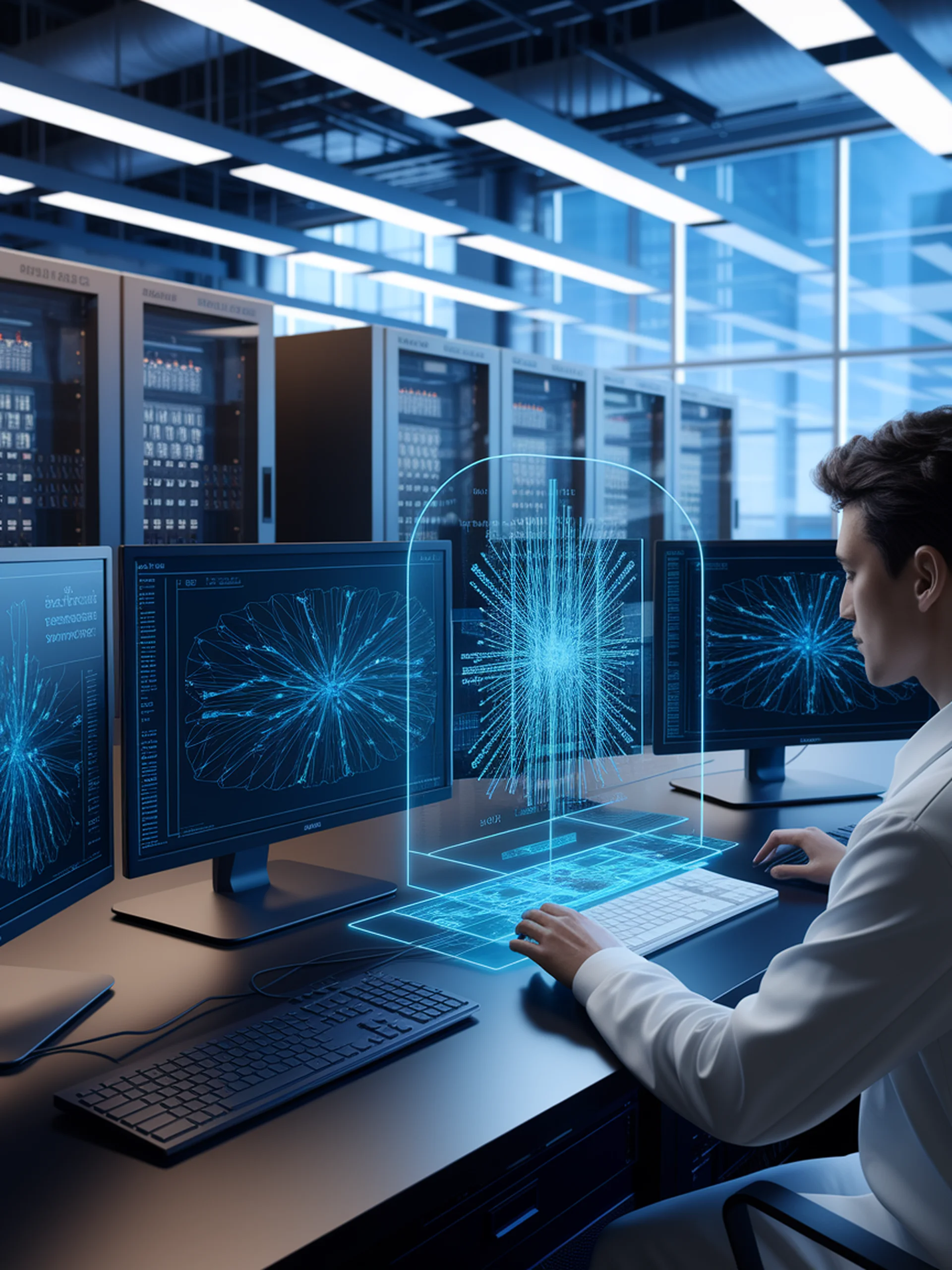
Speeding Up LLMs Without Retraining
A training-free approach to activation sparsity for faster inference
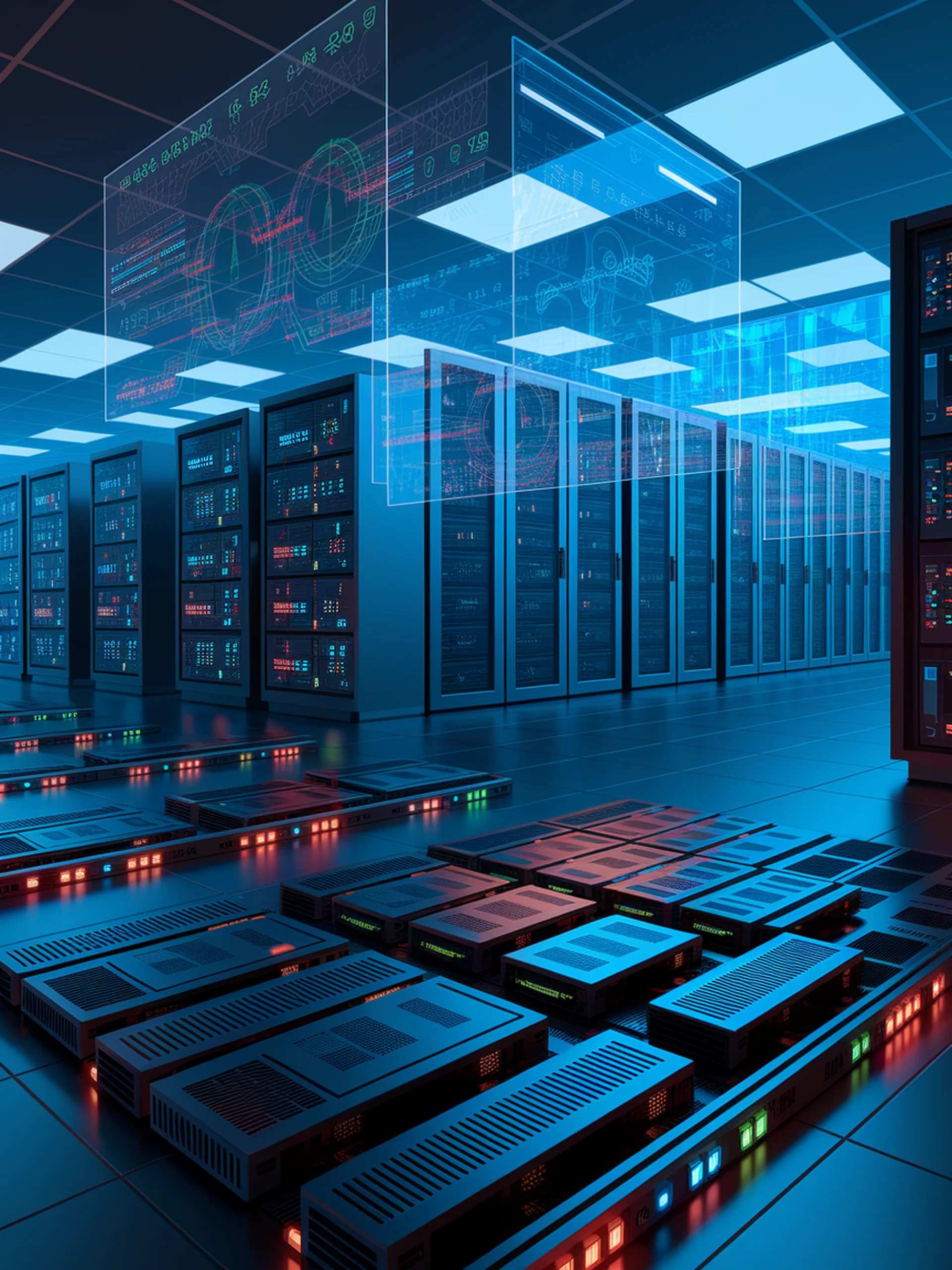
Flexible LLM Training on Mixed Hardware
Unlocking cost-effective LLM development with heterogeneous GPU environments
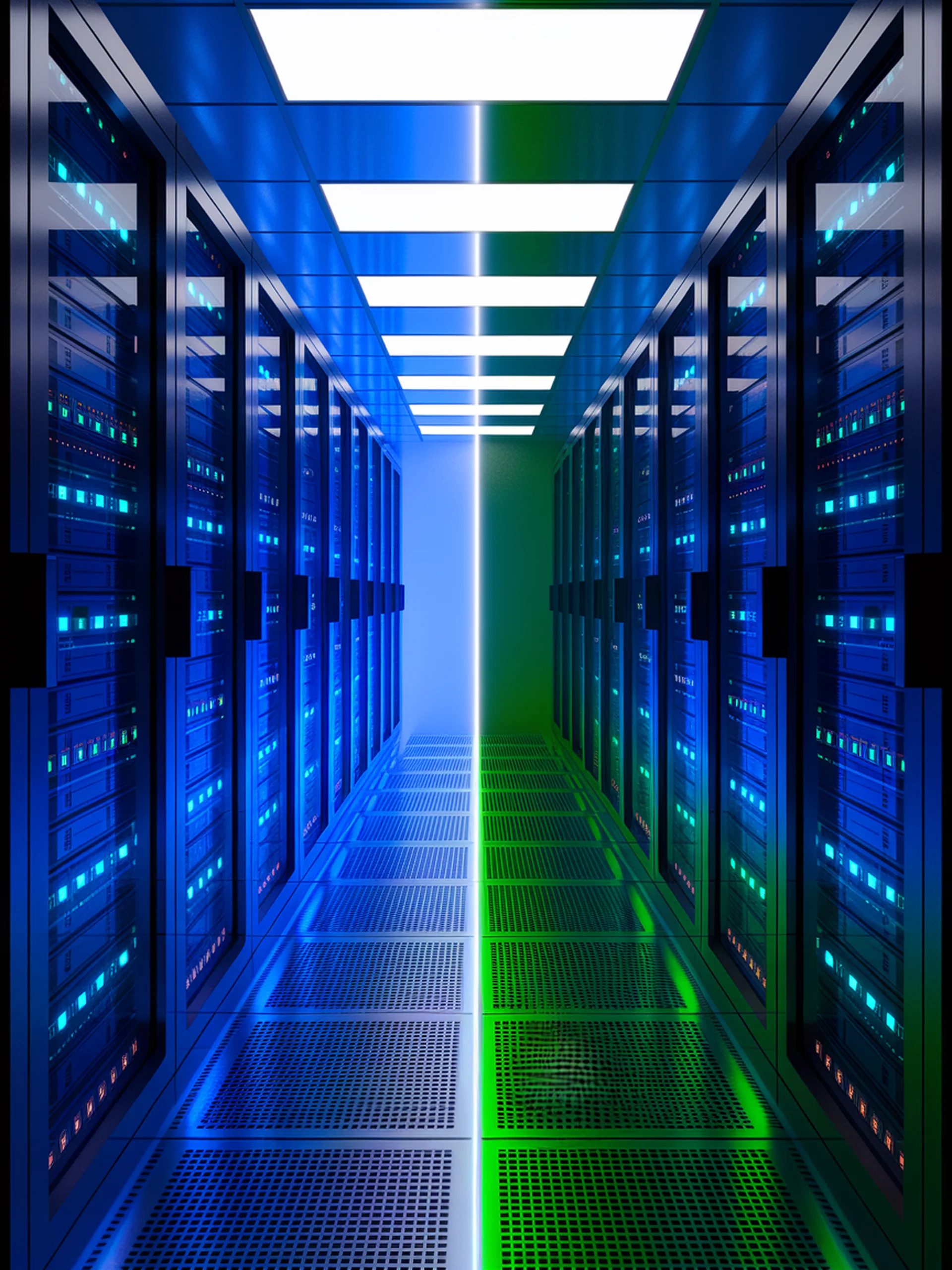
Boosting LLM Efficiency with Path-Consistency
A novel approach to reduce computational costs while maintaining reasoning quality
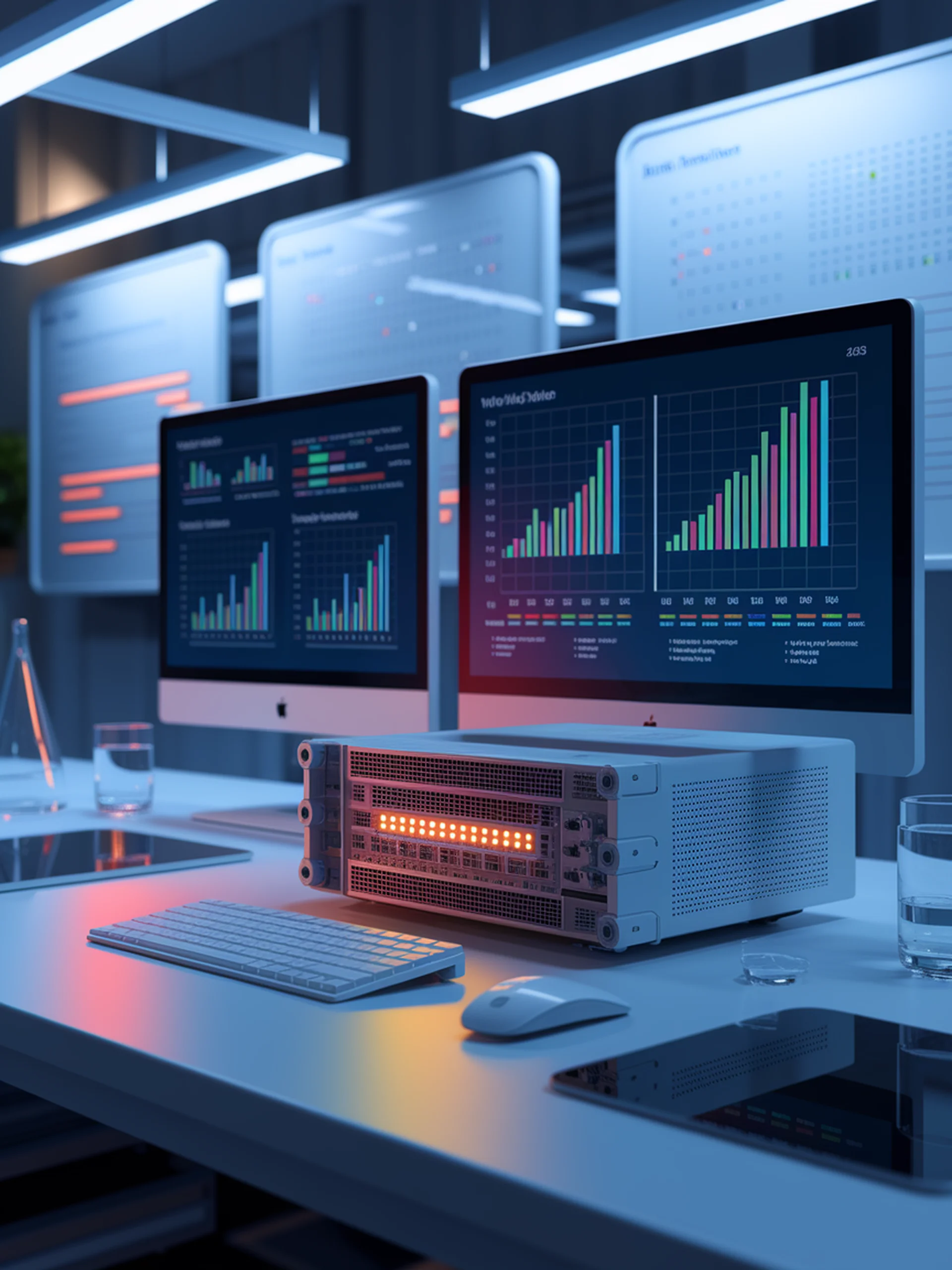
Quantization Trade-Offs in LLMs
A comprehensive analysis across model sizes, tasks, and methods
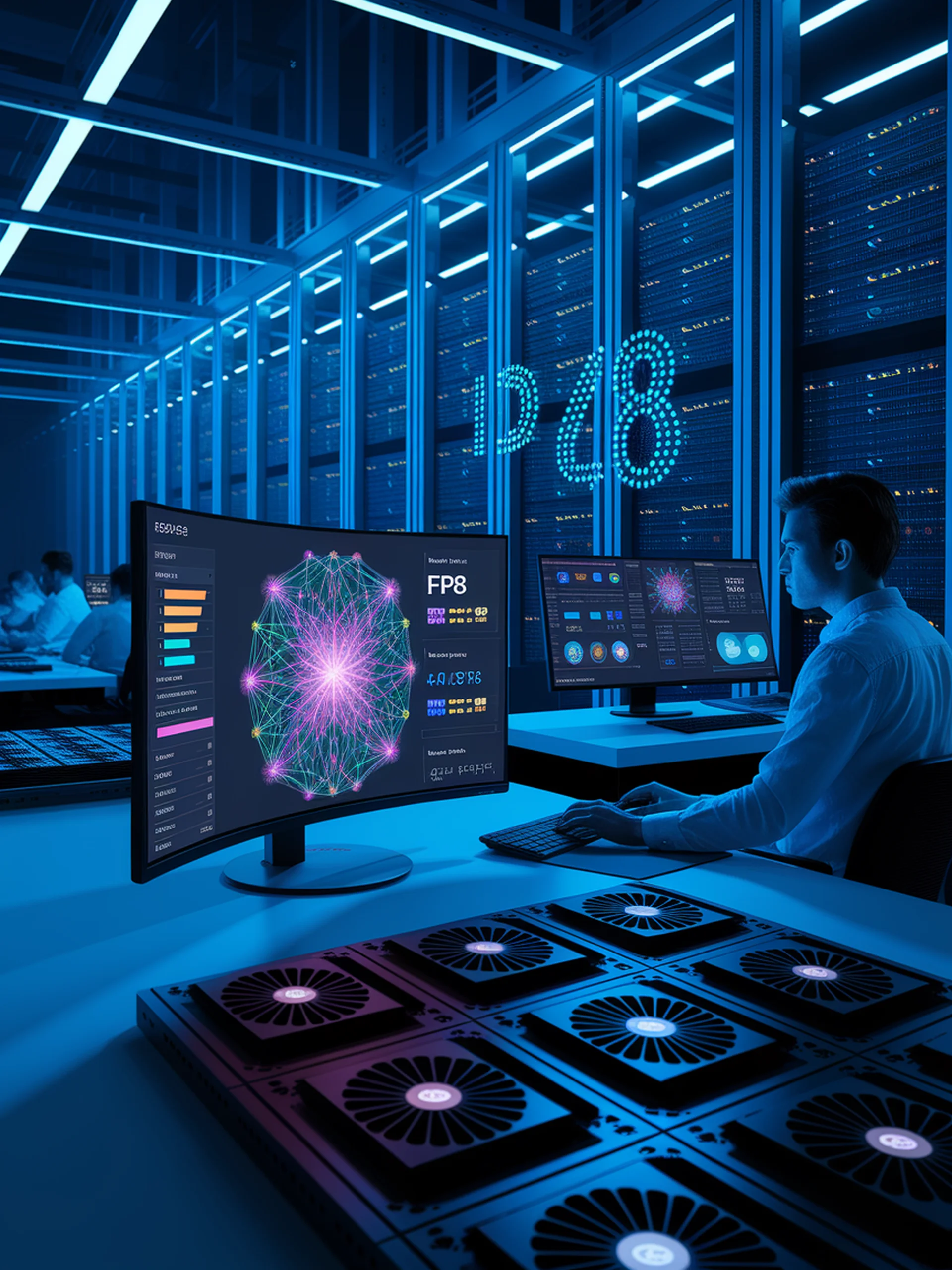
Breaking Barriers: FP8 Training at Trillion-Token Scale
Solving critical instabilities for unprecedented LLM training efficiency
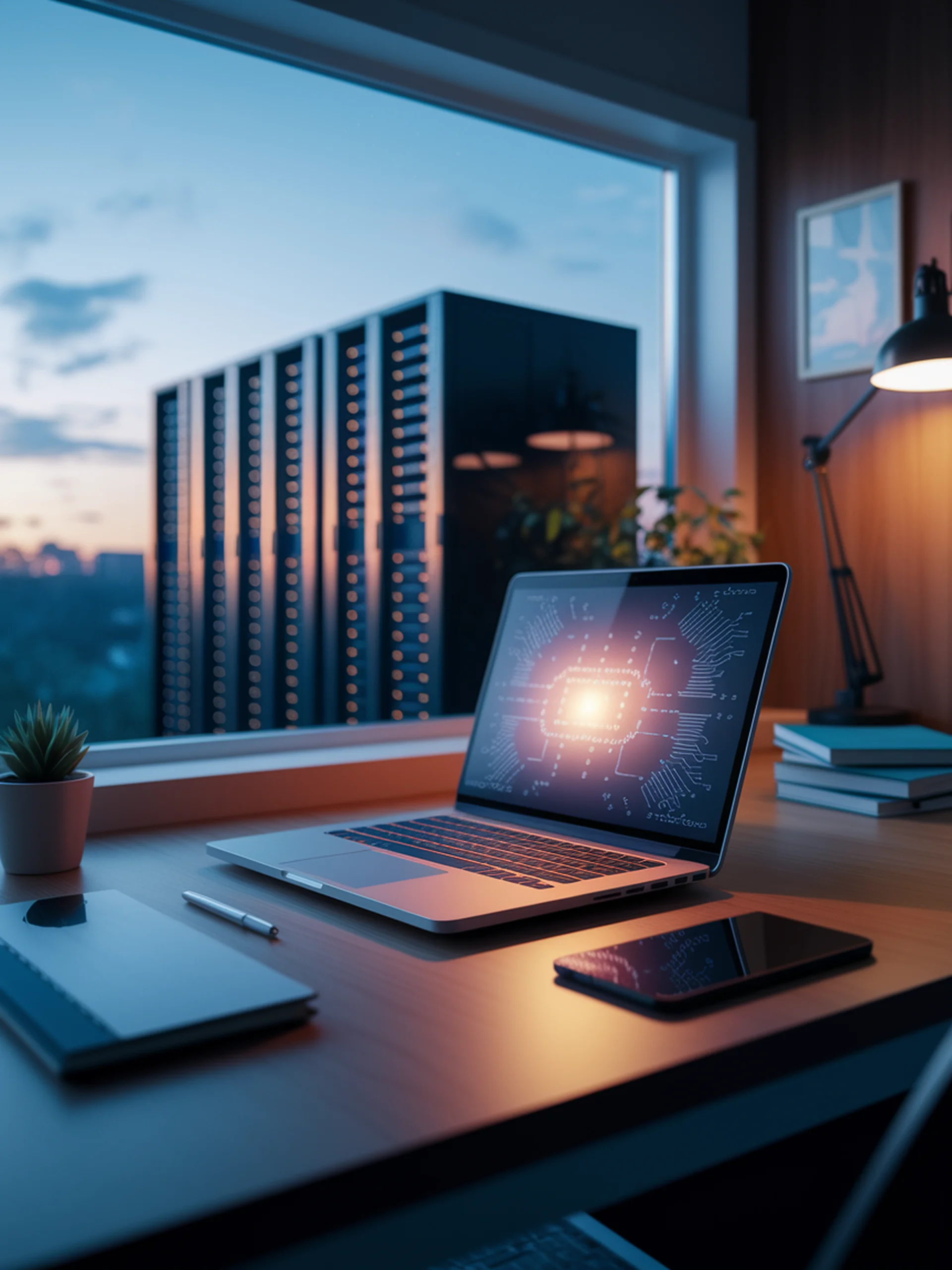
Right-Sizing AI: The Power of Small Language Models
Making AI accessible beyond data centers
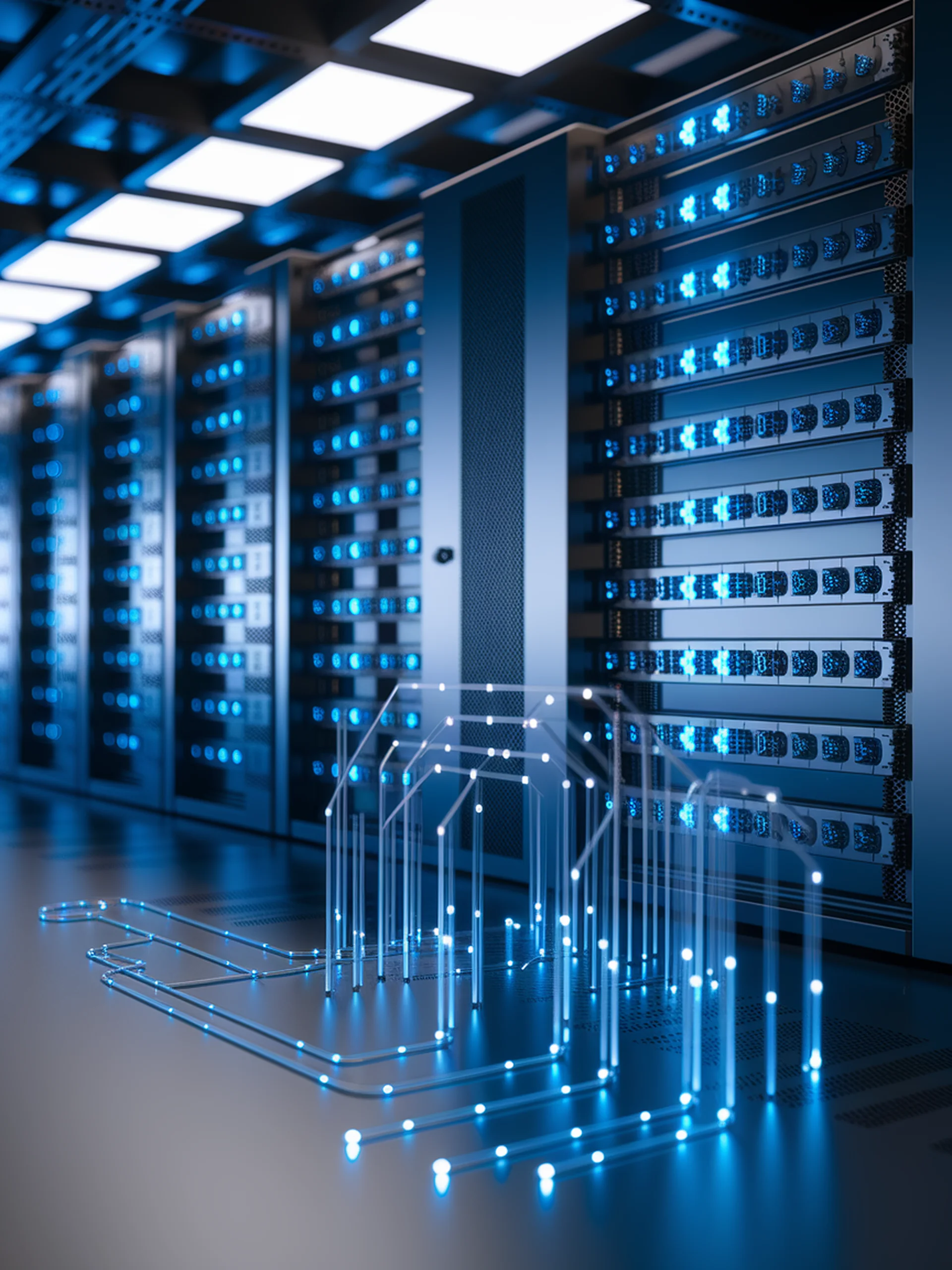
Speeding Up LLM Inference
A novel dynamic-width beam search approach for faster AI text generation
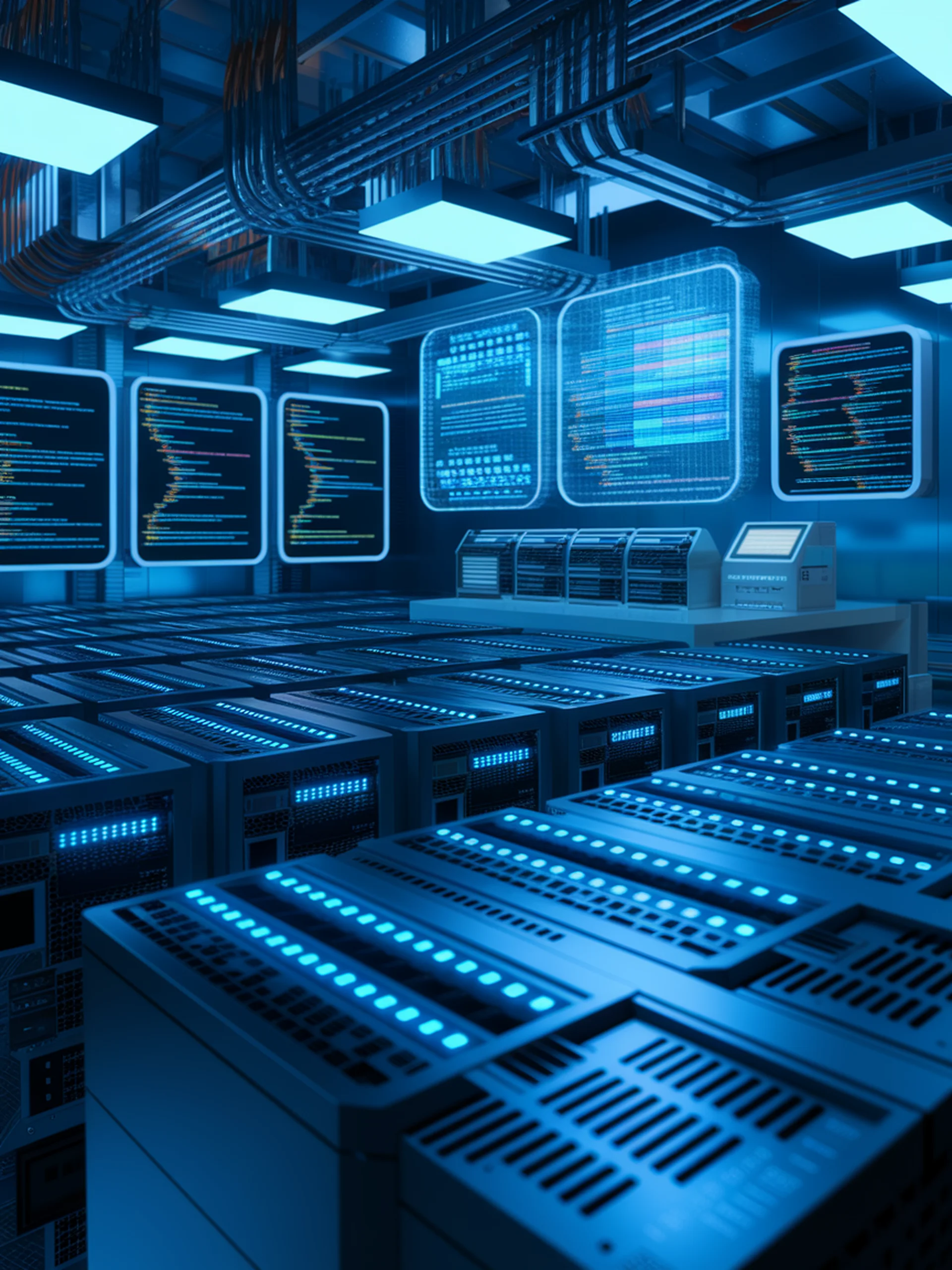
Breaking the Context Length Barrier for LLMs
Efficient Inference for Multi-Million Token Contexts Without Approximations
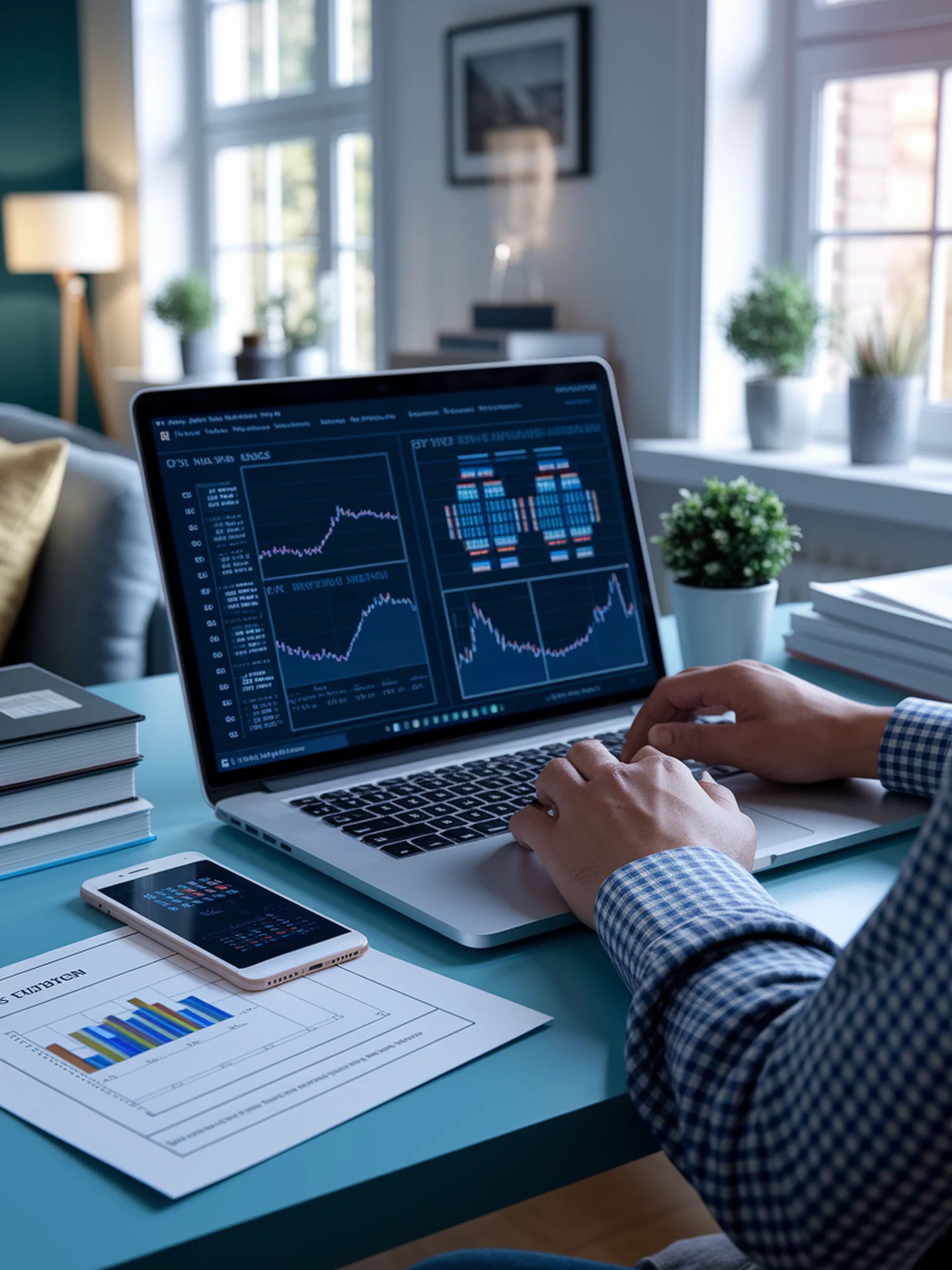
Unlocking Long-Context LLMs on Consumer Devices
Efficient memory management with trained retaining heads
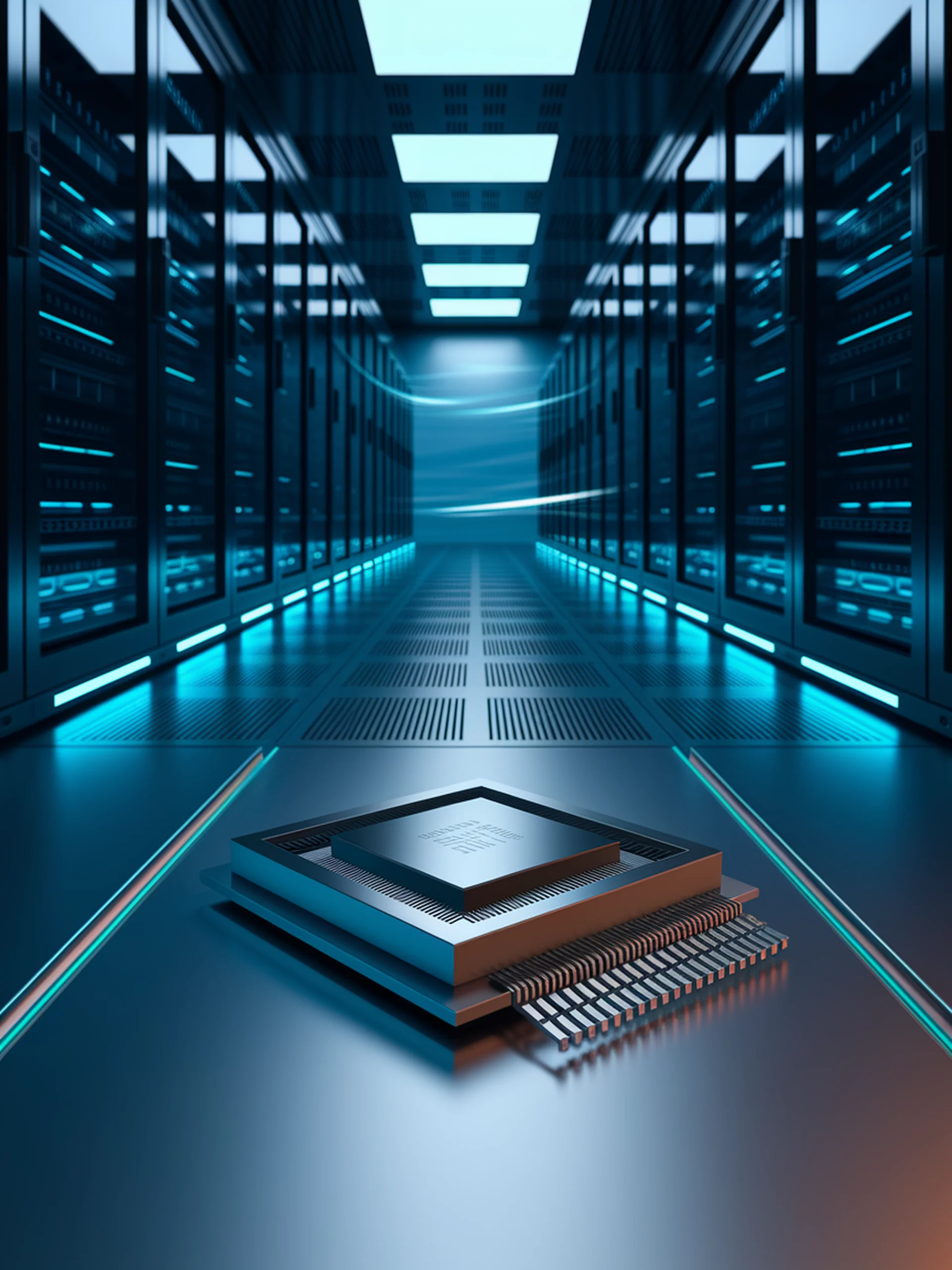
8-Bit Precision: The Future of LLM Acceleration
Transforming attention mechanisms for faster, efficient inference
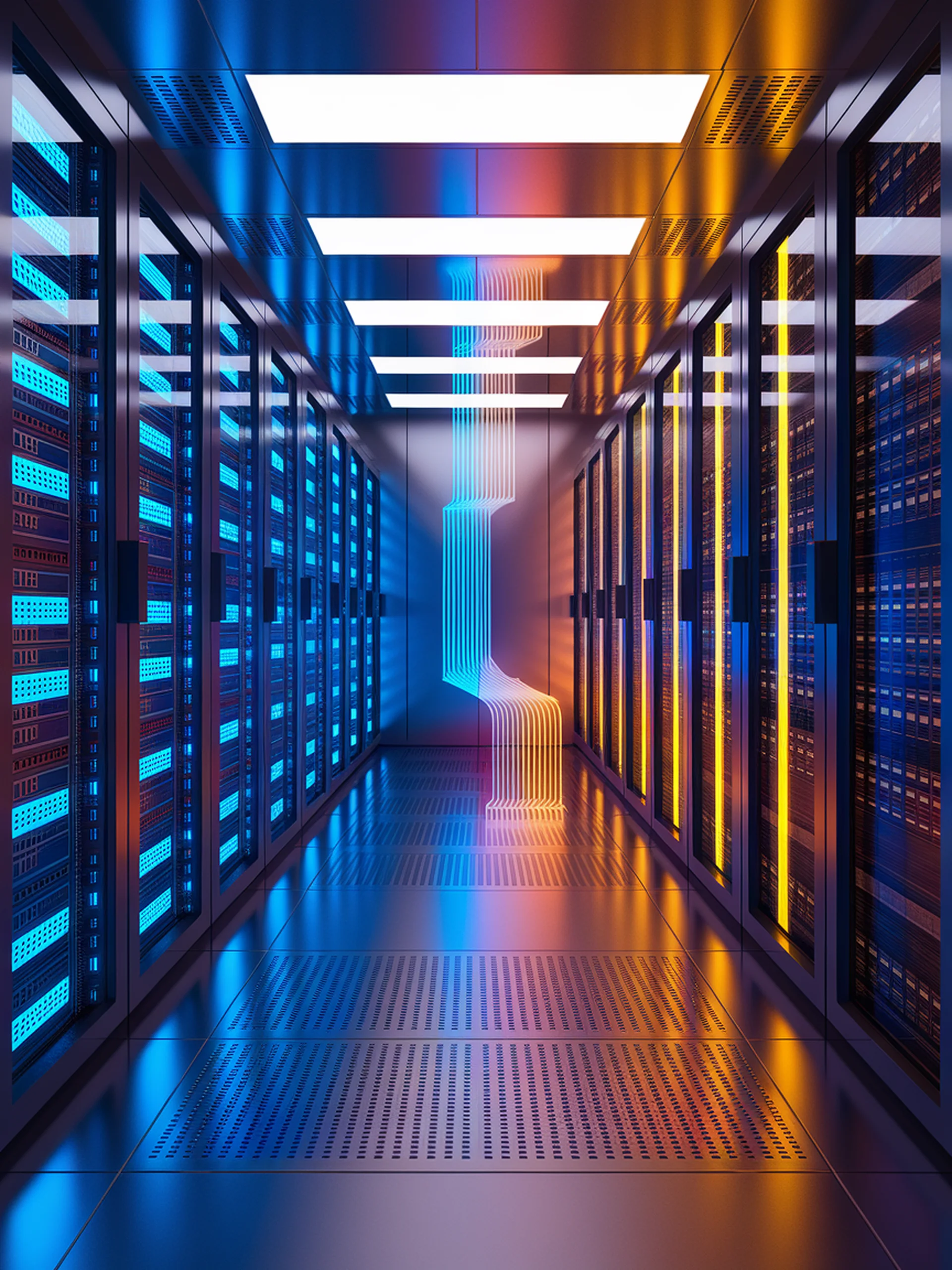
Optimizing LLM Inference with Hybrid KV Cache Management
A Dynamic Approach to Balance Computing and Loading for Better Performance
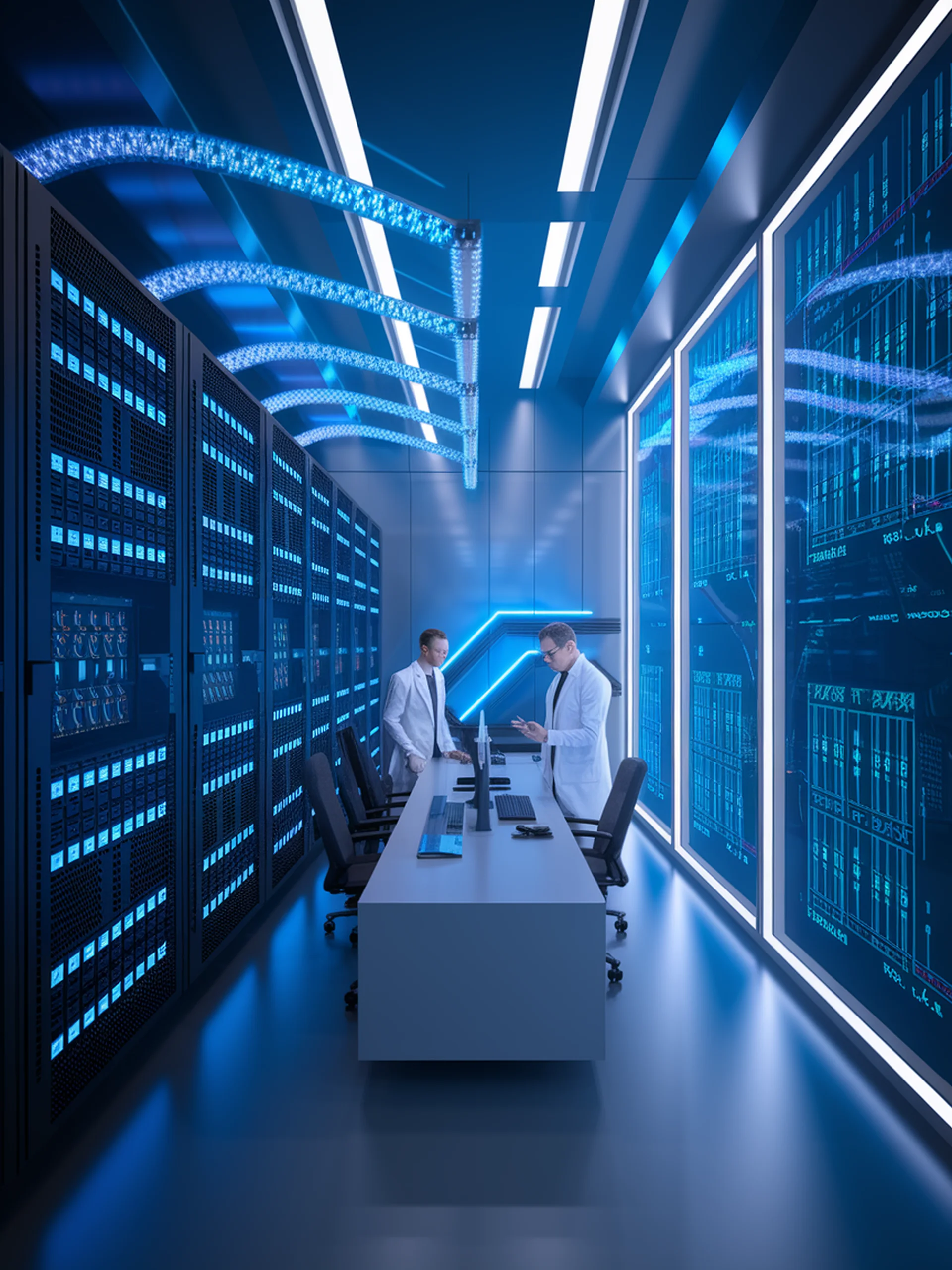
Accelerating LLMs with Smart Decoding
Mixture of Attentions approach dramatically speeds up language model inference
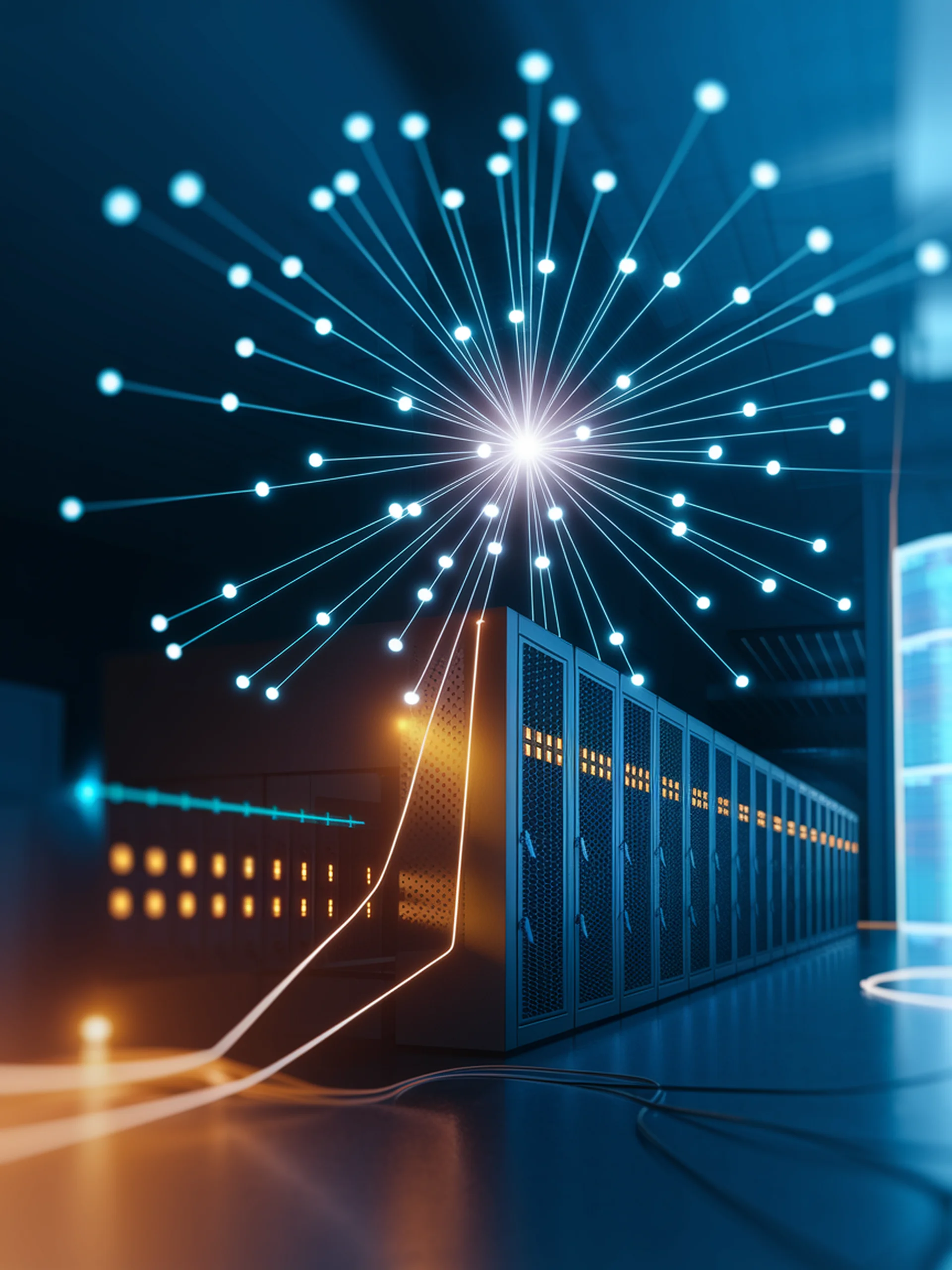
GraphRouter: Intelligent LLM Selection
A graph-based approach to match queries with optimal language models
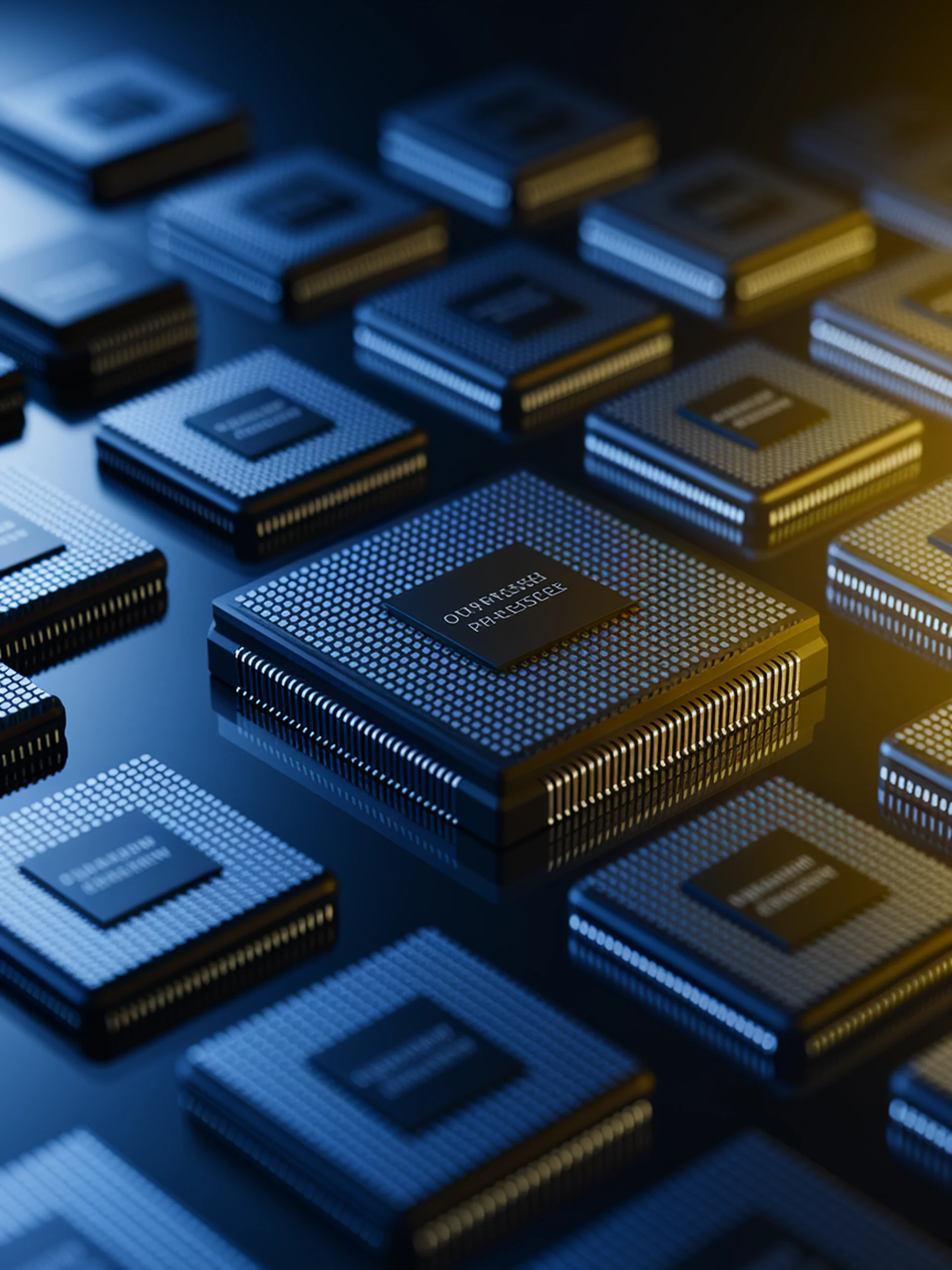
Smarter Model Compression with PrefixQuant
Eliminating token-wise outliers for superior LLM quantization
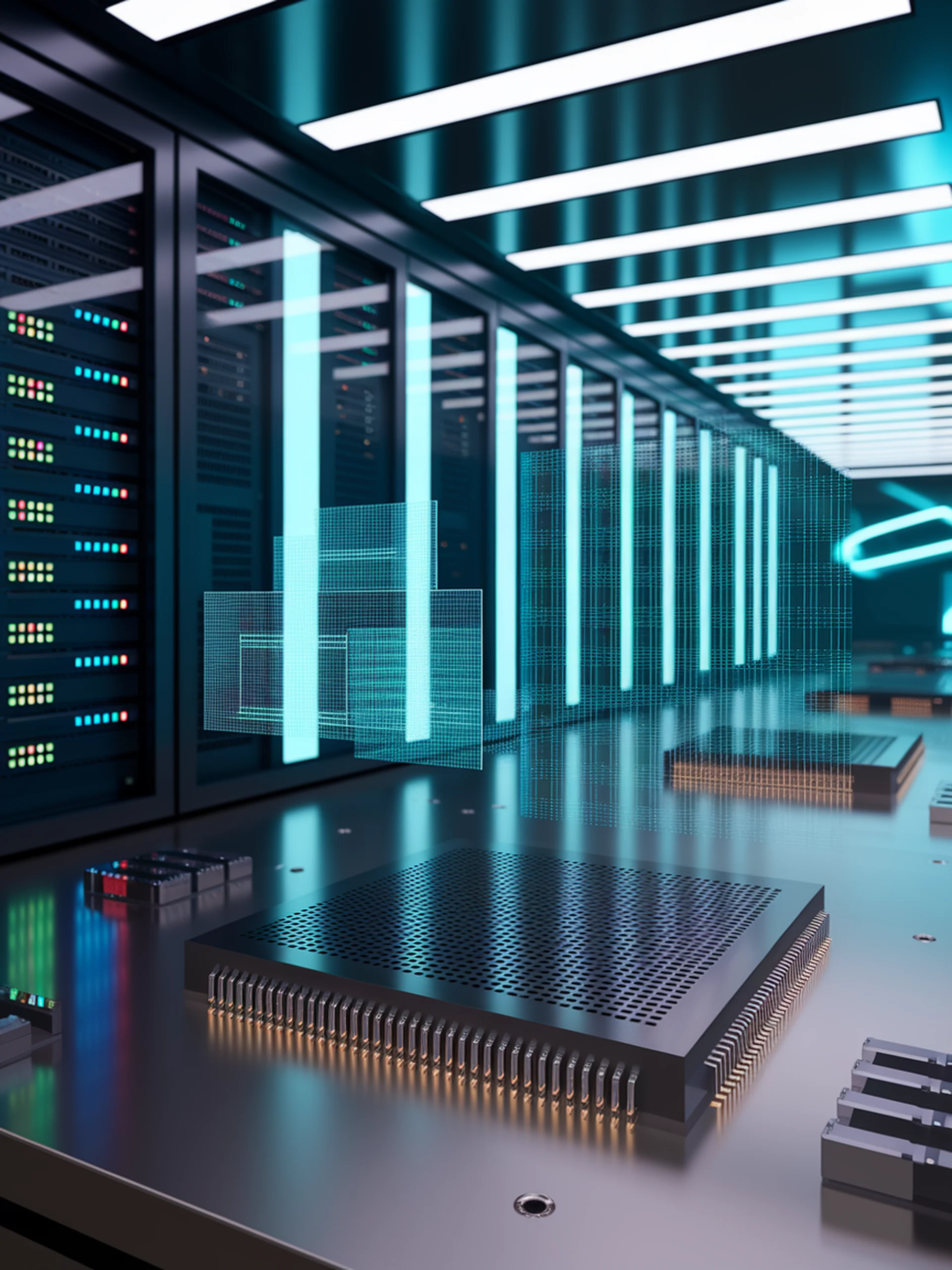
Efficient LLM Deployment Through Precision Engineering
A Novel Framework for Balancing Low Precision and Accuracy
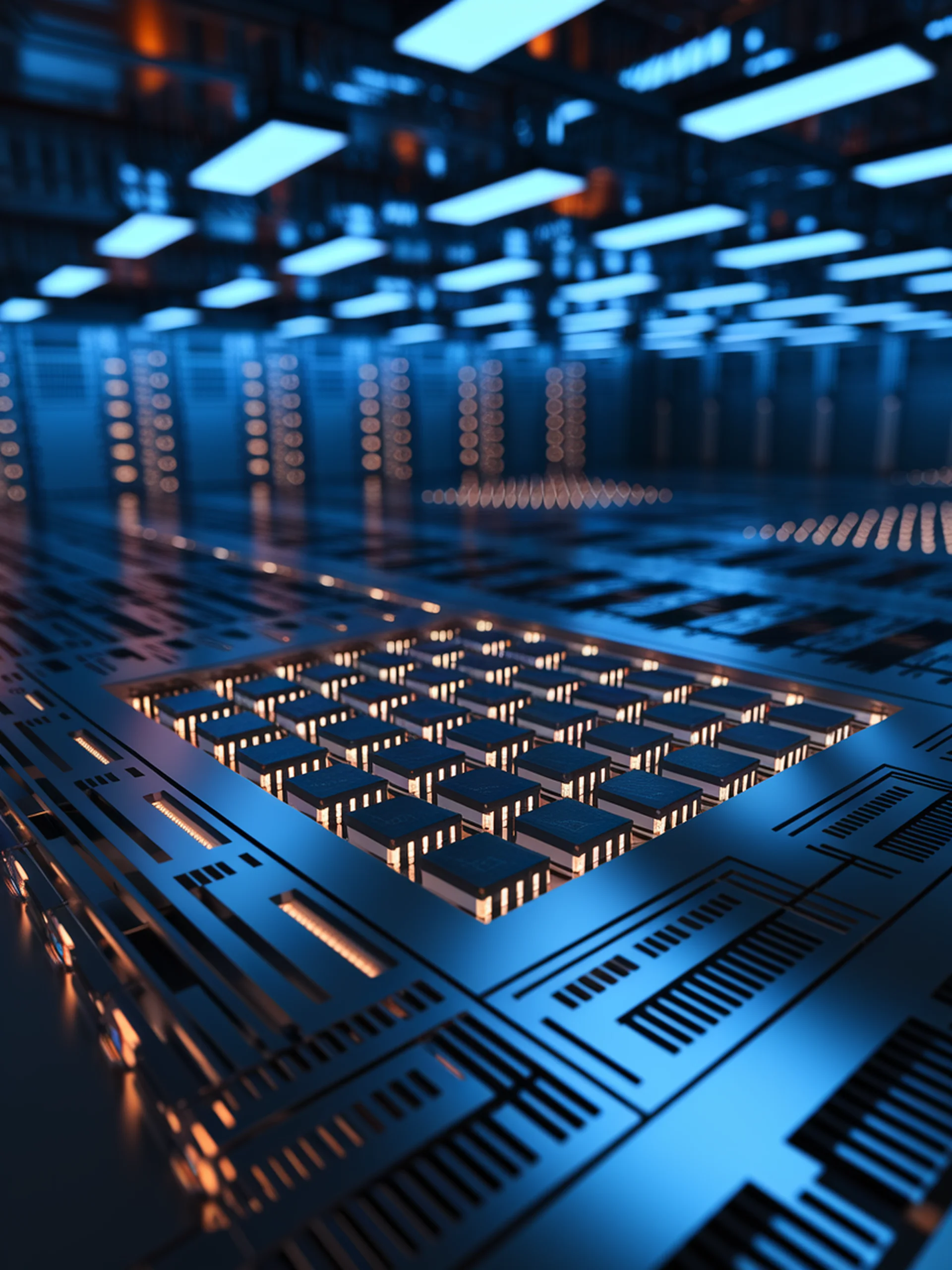
Streamlining MoE Language Models
Reducing redundancy and memory footprint in Mixture-of-Experts LLMs
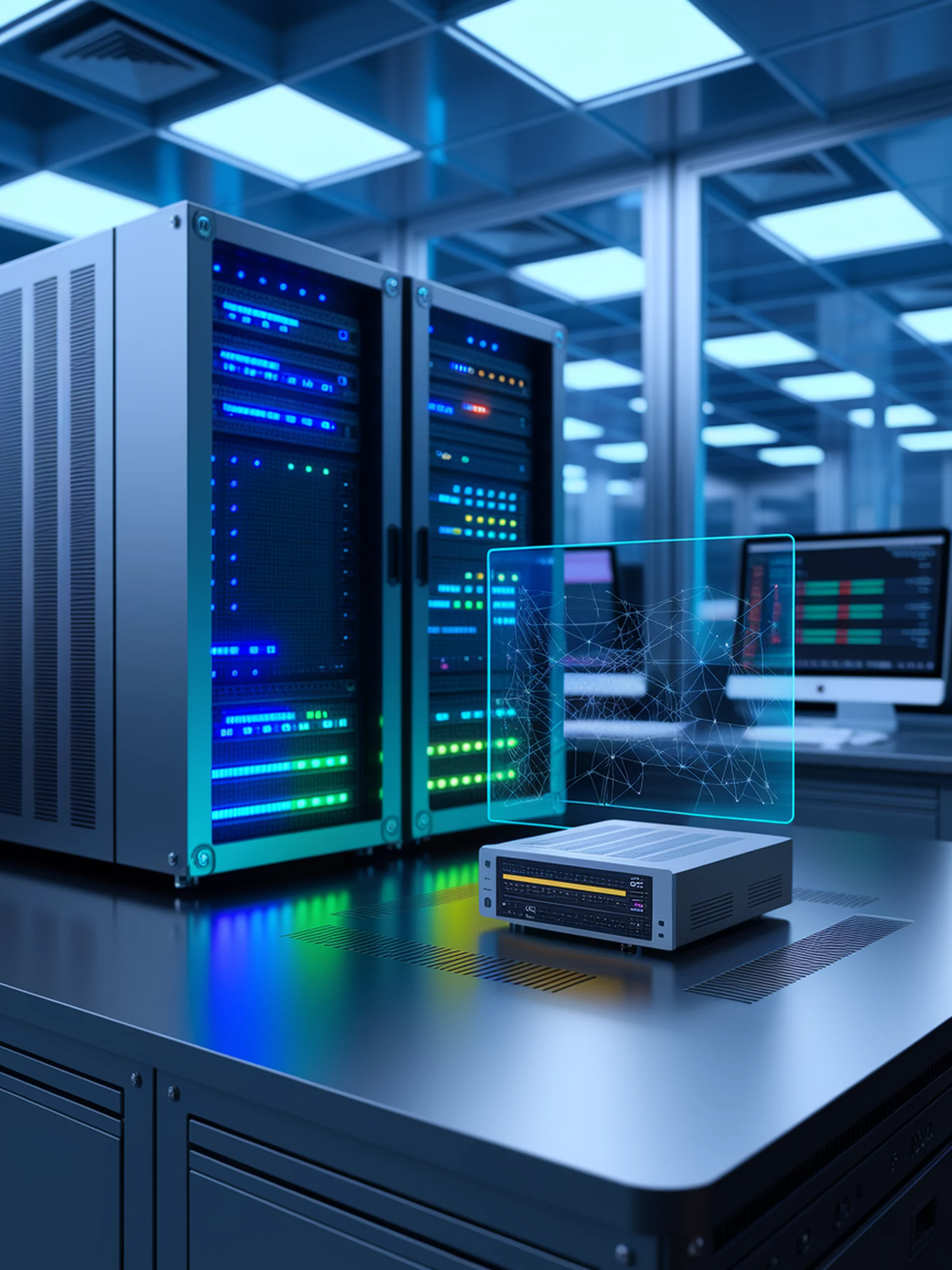
Shrinking LLMs without Sacrificing Performance
Automated techniques to make large language models smaller and faster
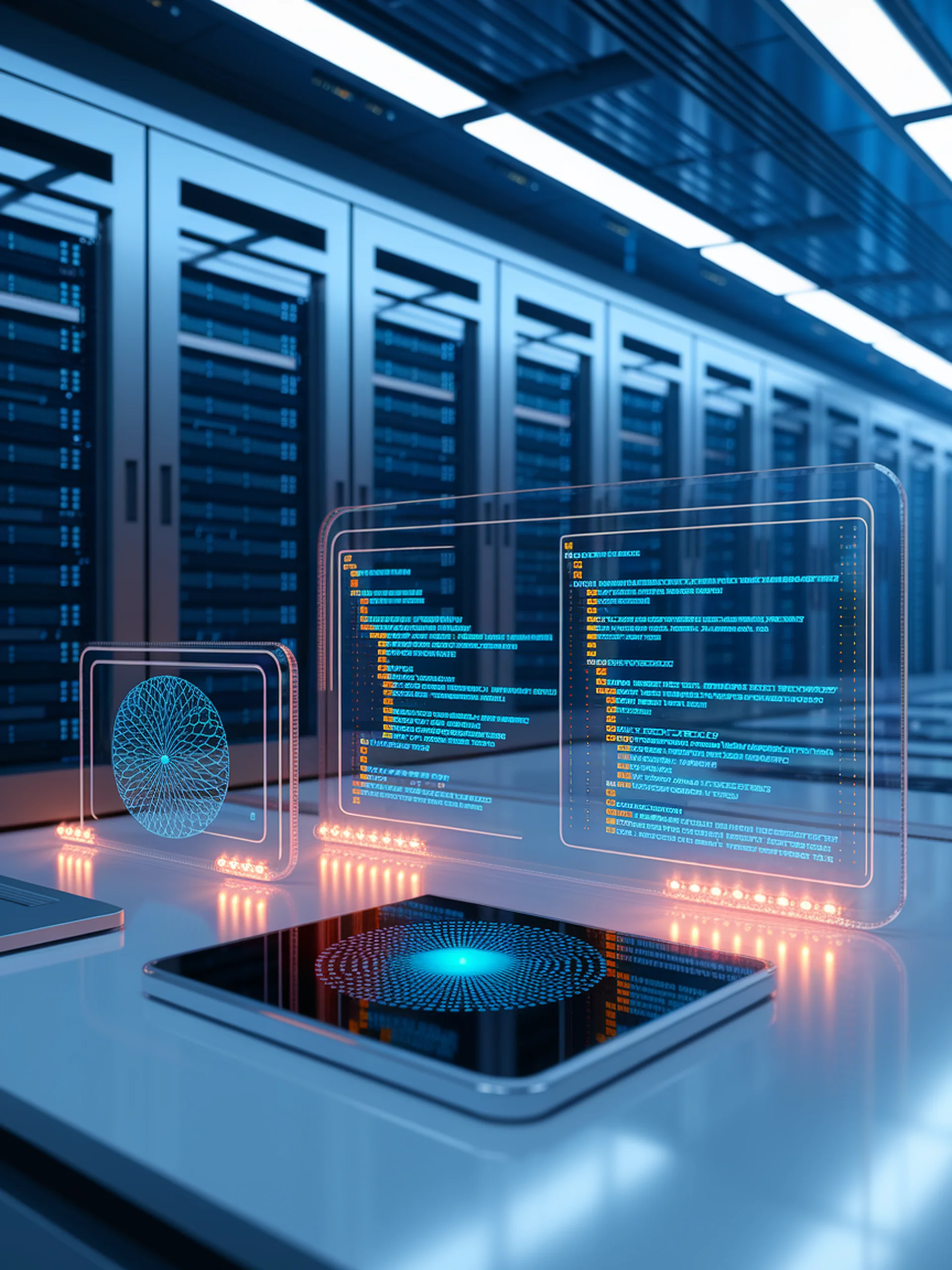
Smarter Compression for Vision-Language Models
Optimizing multi-modal AI with advanced quantization
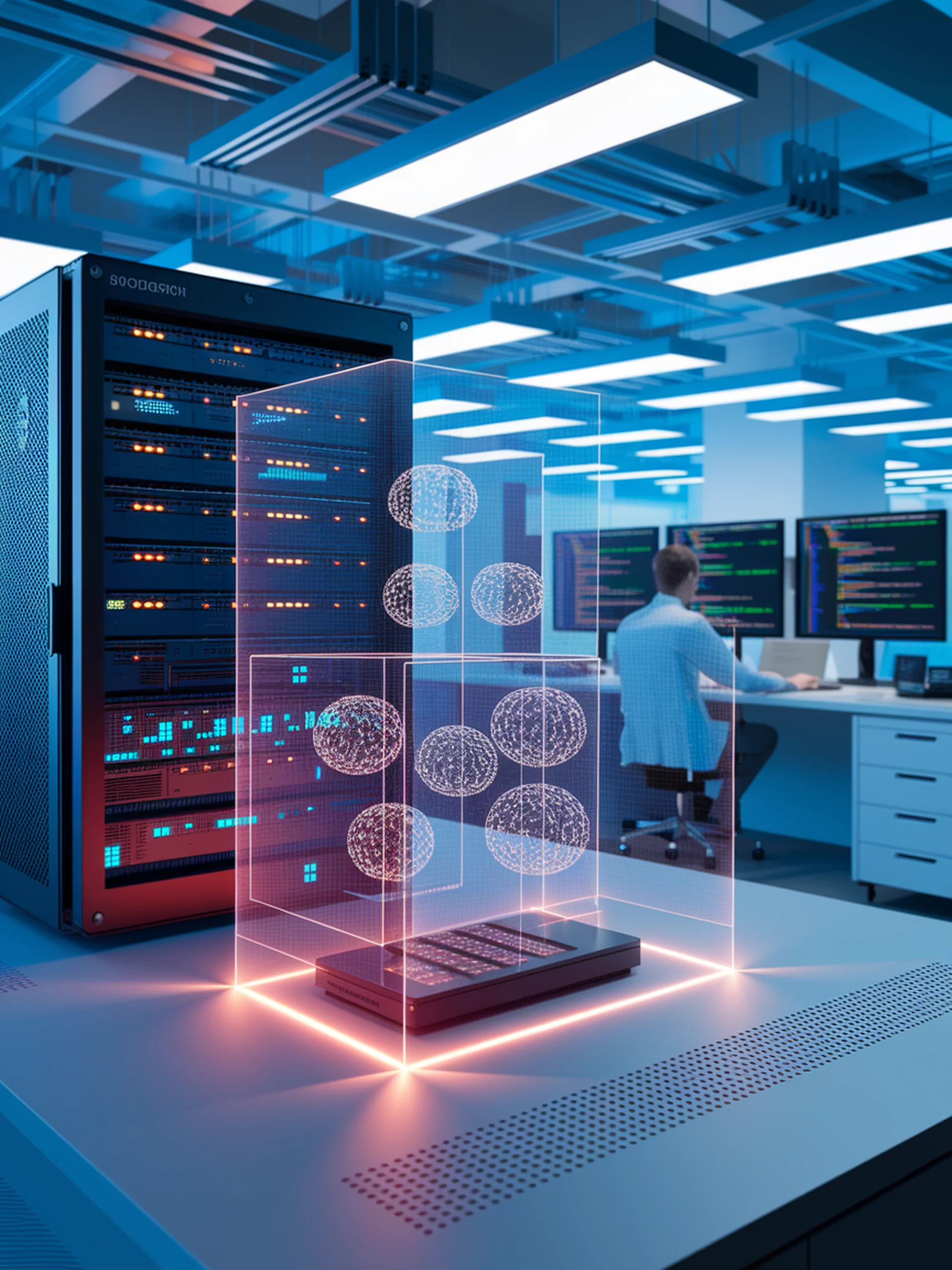
Optimizing Sparse MoE Models
Merging experts through hierarchical clustering without retraining
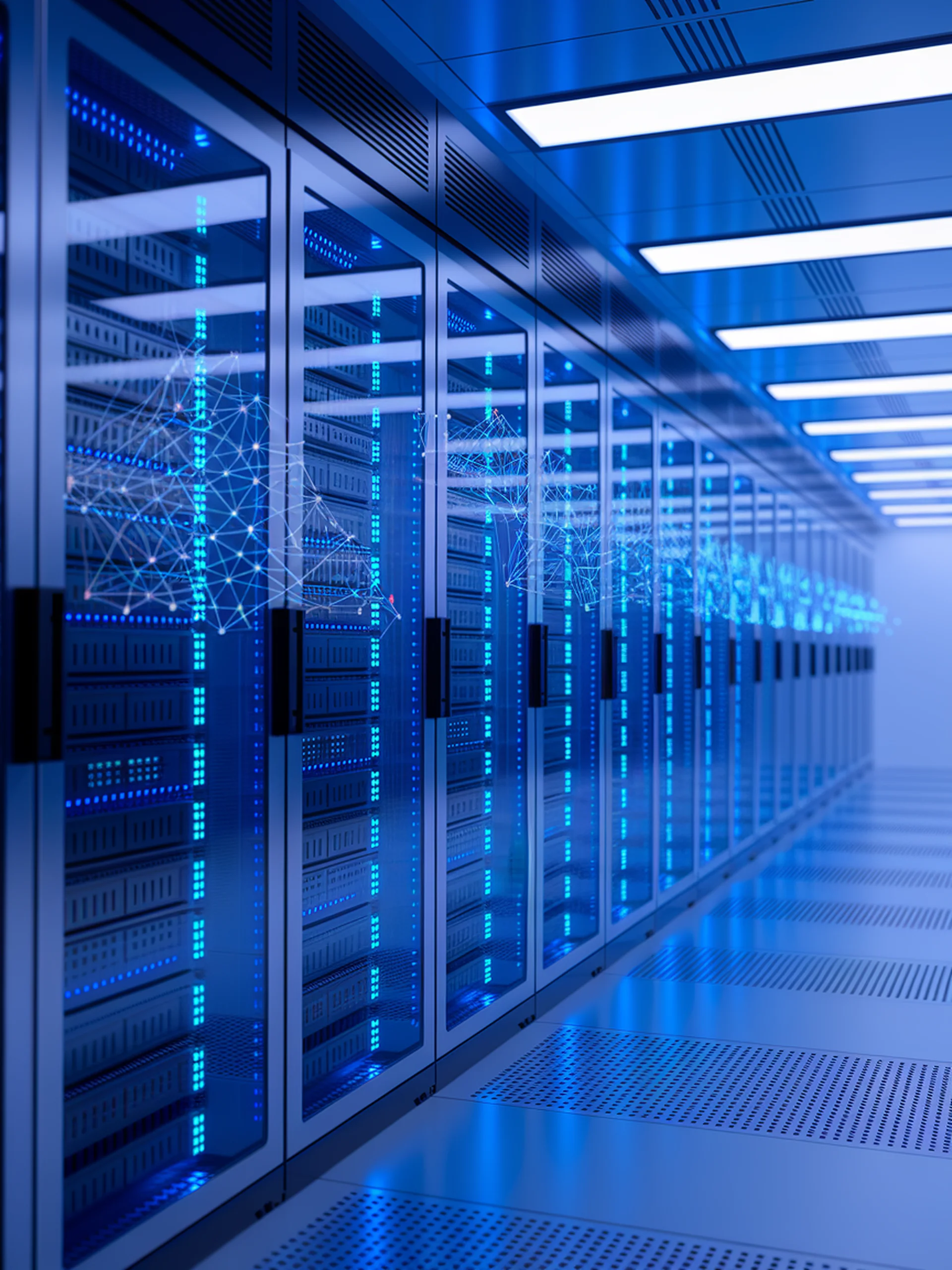
Accelerating LLMs for Long-Context Tasks
A Novel Sparse Attention Approach Using HSR Enhancement
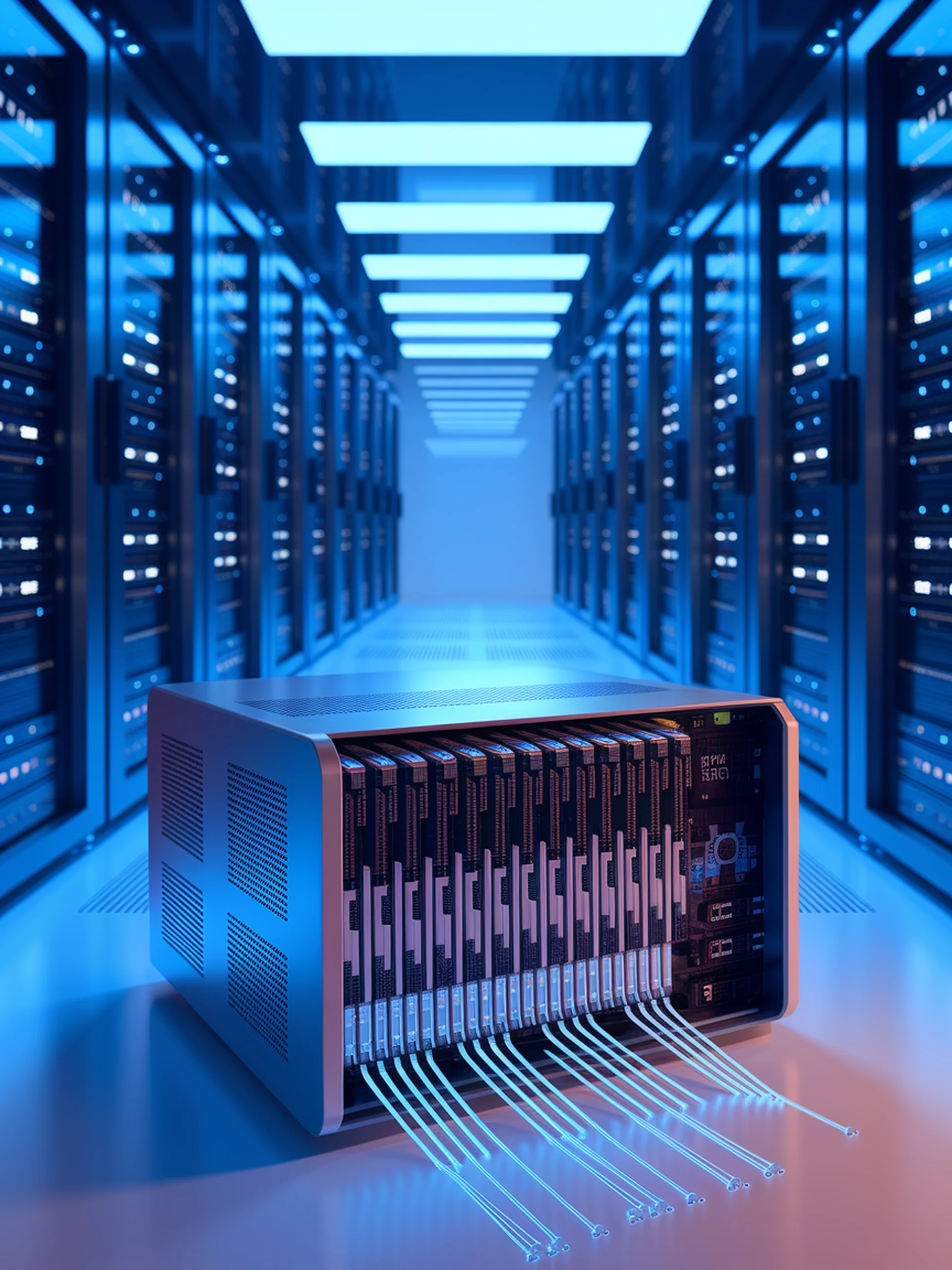
Making LLMs Faster & More Efficient
A novel approach to linearize large language models without performance loss
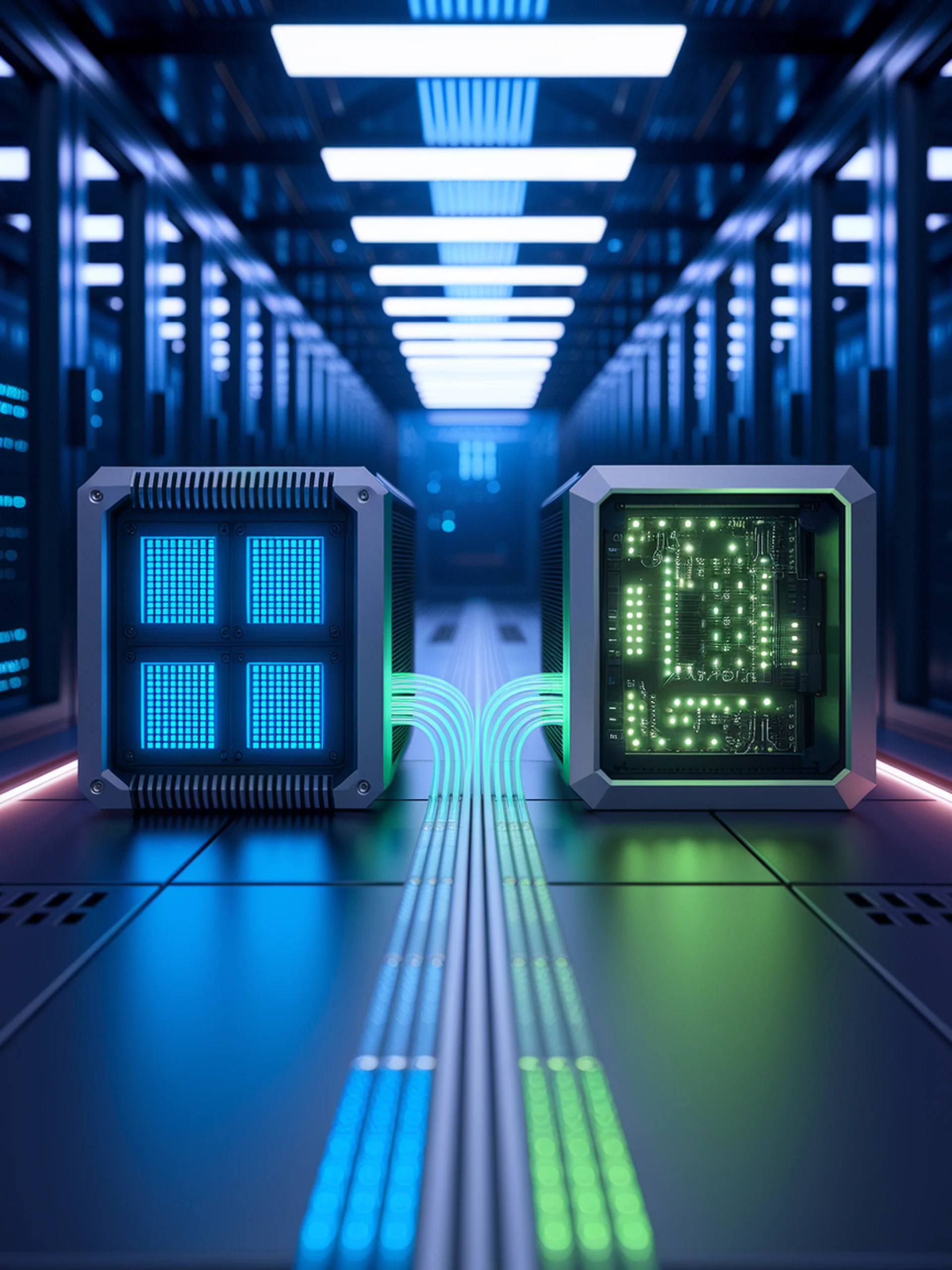
Speeding Up LLMs with Dual-Quantization
Combining quantization schemes for faster, more accurate inference
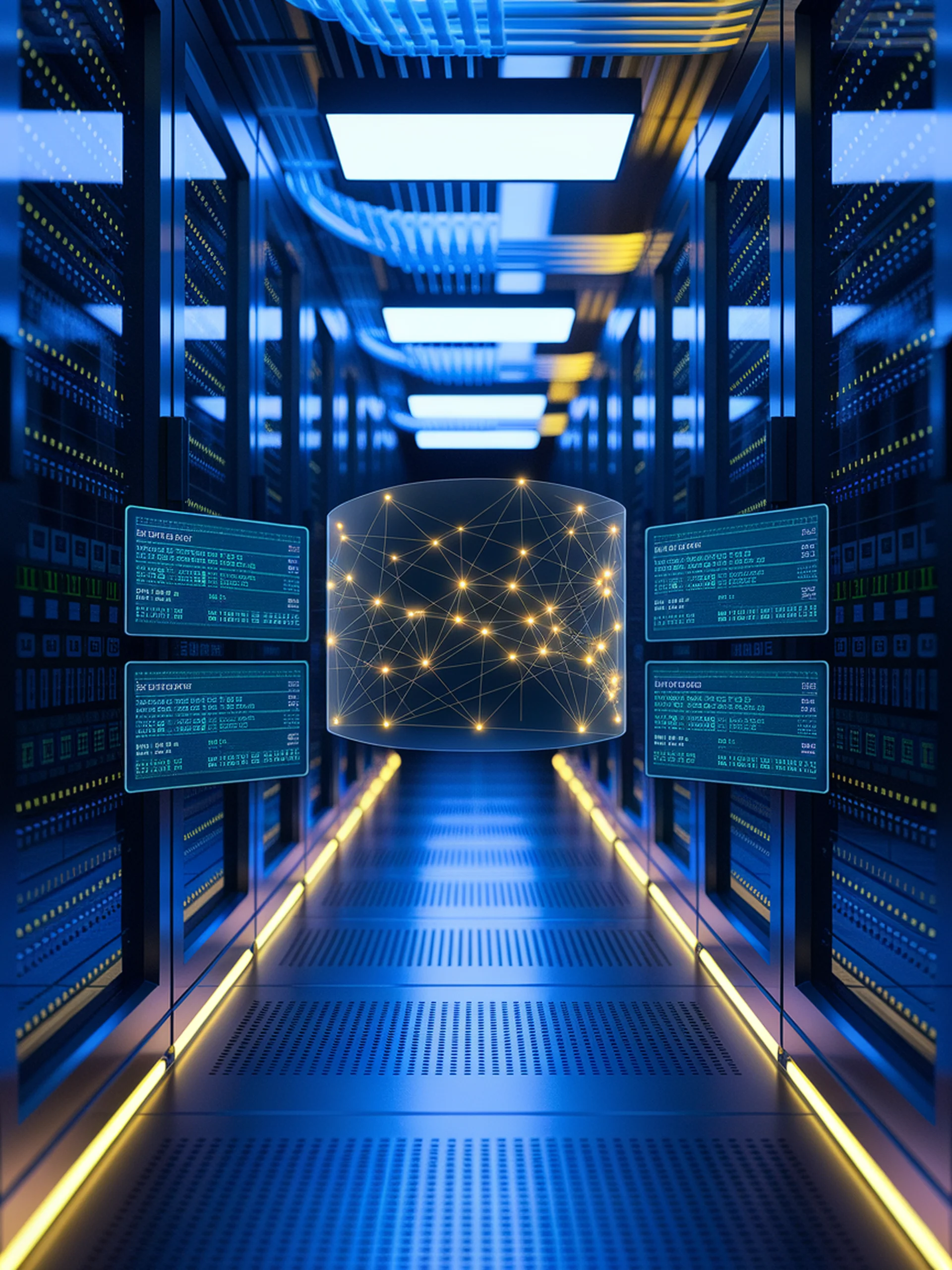
Fault-Proofing LLM Training
A highly-optimized approach to prevent attention mechanism failures
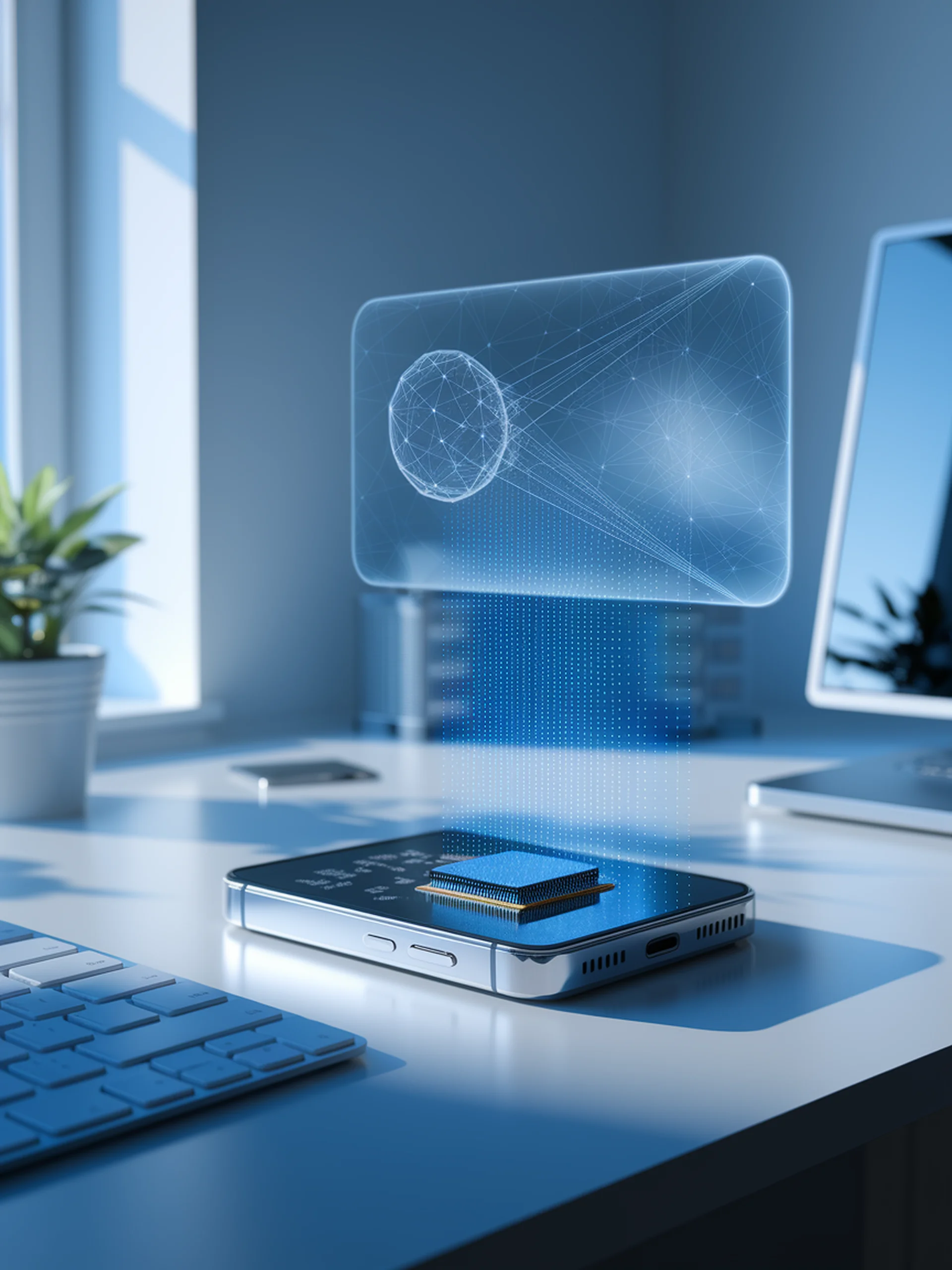
Optimizing LLMs for Edge Computing
Addressing the challenges of deploying powerful AI on resource-constrained devices

Making LLMs Faster & Smarter with Sparse Attention
A novel approach to reduce computational complexity in large language models
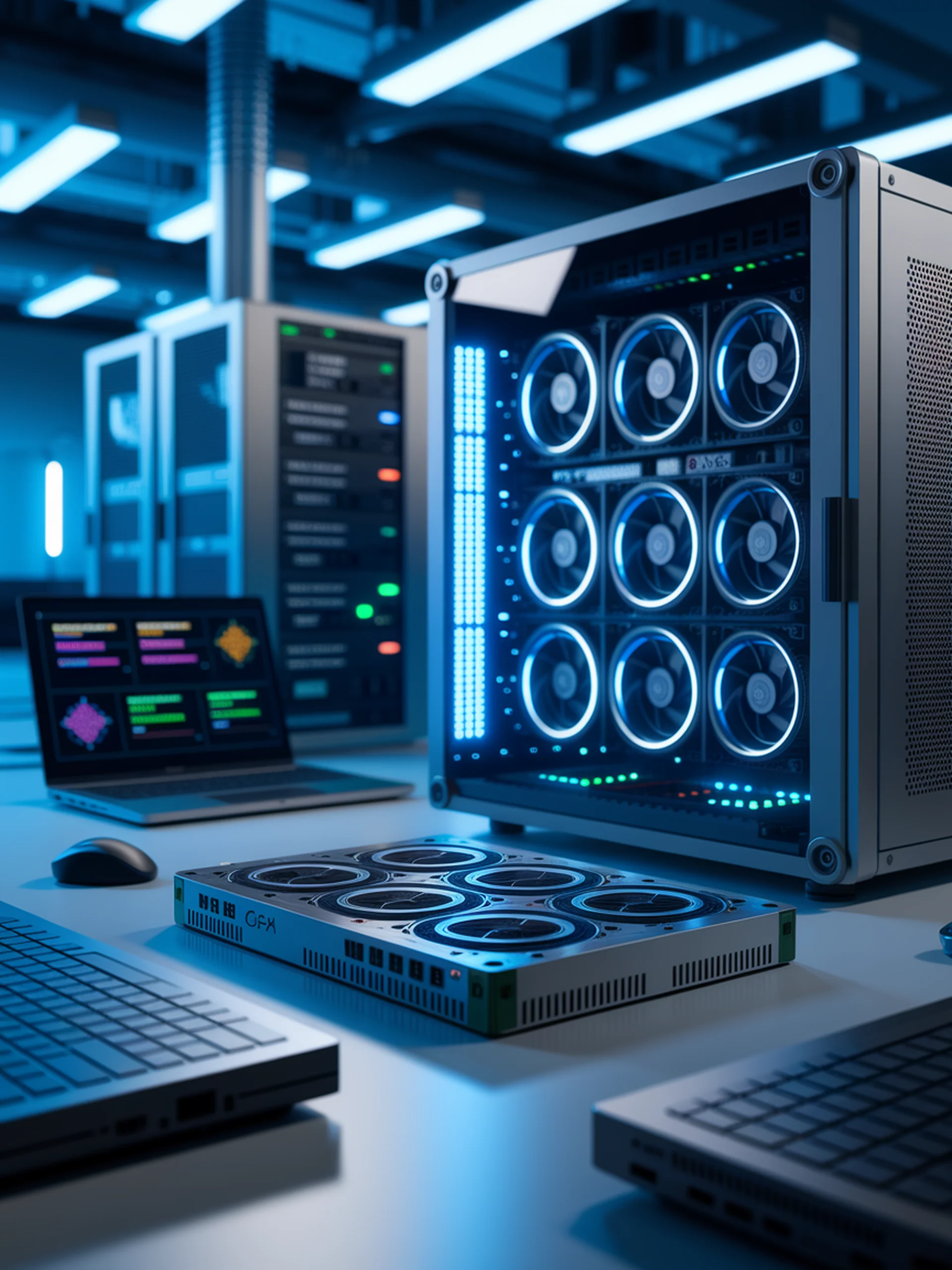
Optimizing LLM Efficiency Without Sacrificing Accuracy
Progressive Mixed-Precision Decoding for Resource-Constrained Environments
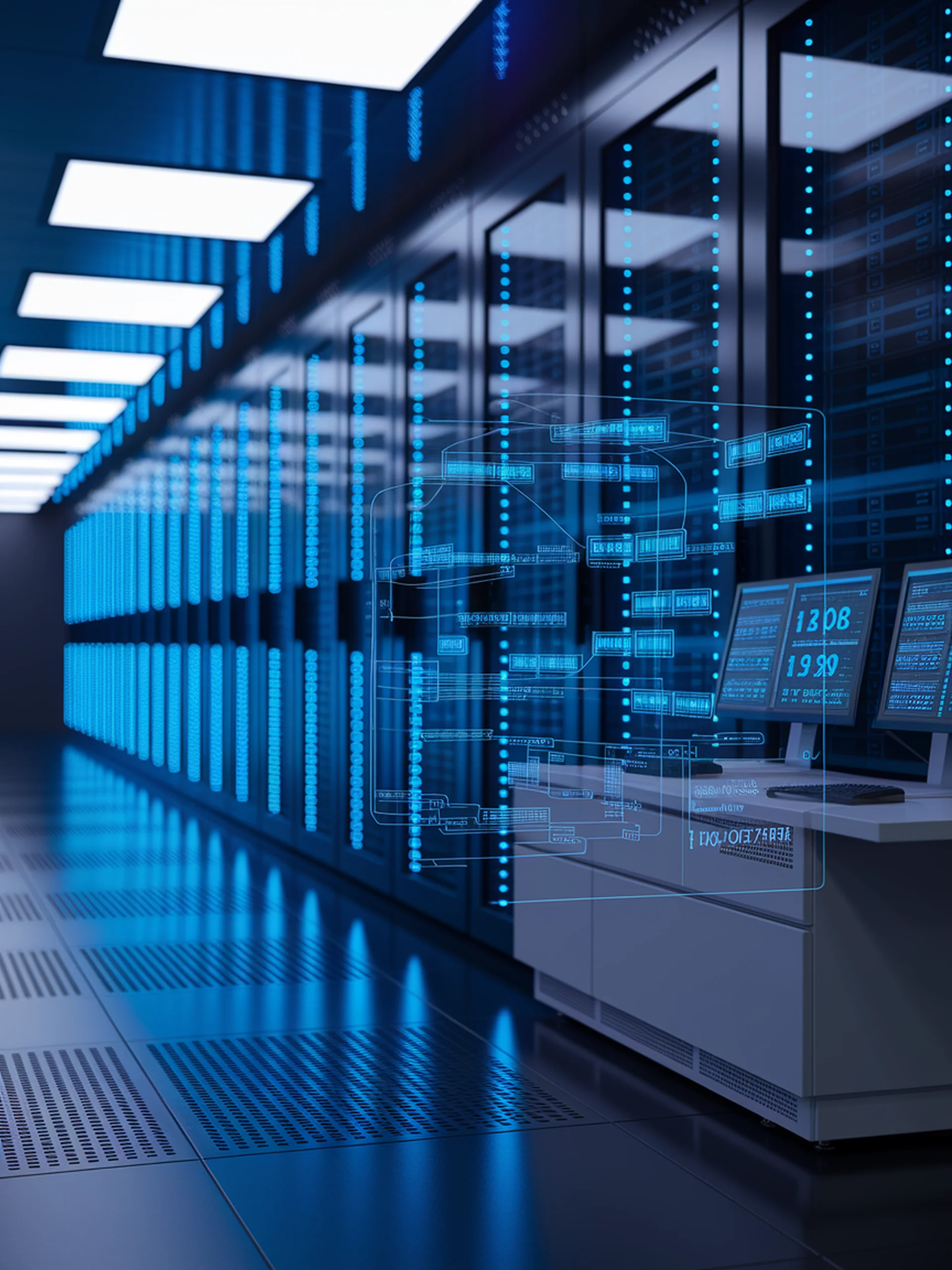
Optimizing LLM Inference with Cross-Layer KV Sharing
A systematic approach to reducing computational costs while maintaining performance
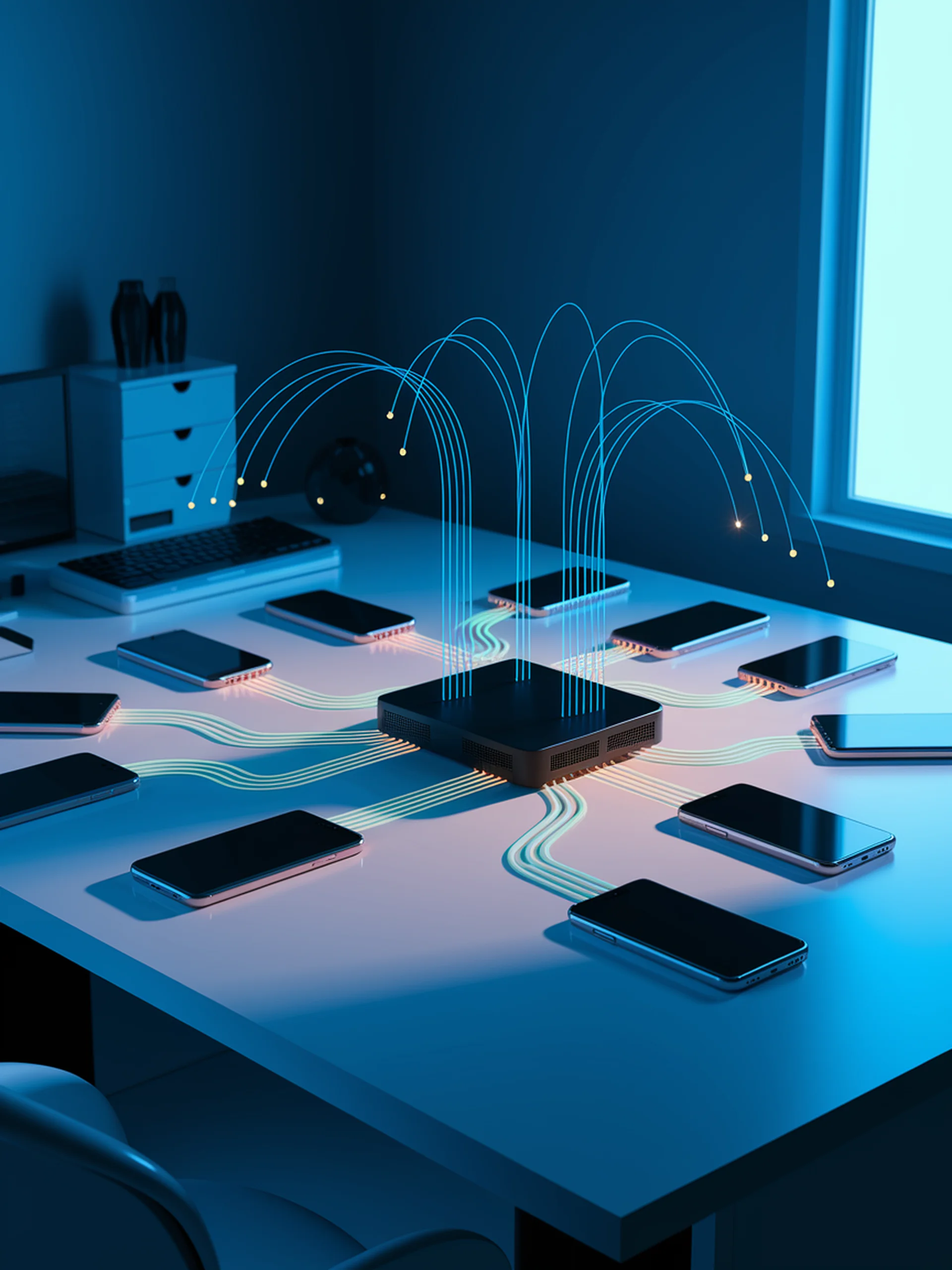
DistRL: Scaling Control Agents for Mobile Devices
Distributed Reinforcement Learning Framework for On-Device Intelligence
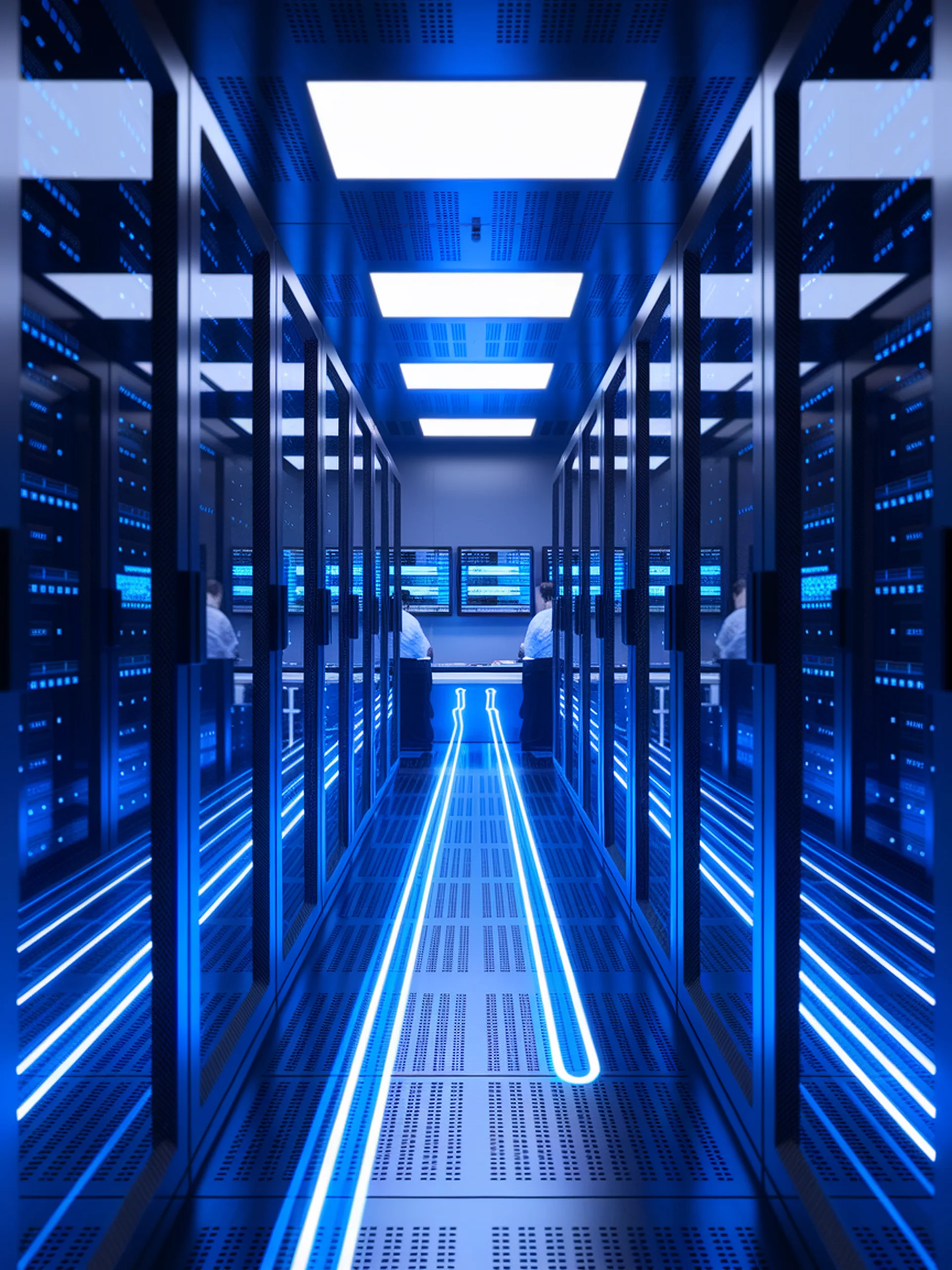
Reimagining LLM Serving Efficiency
Position-Independent Context Caching for Faster Response Times

Self-Improving AI Systems
LLMs designing their own improvement algorithms
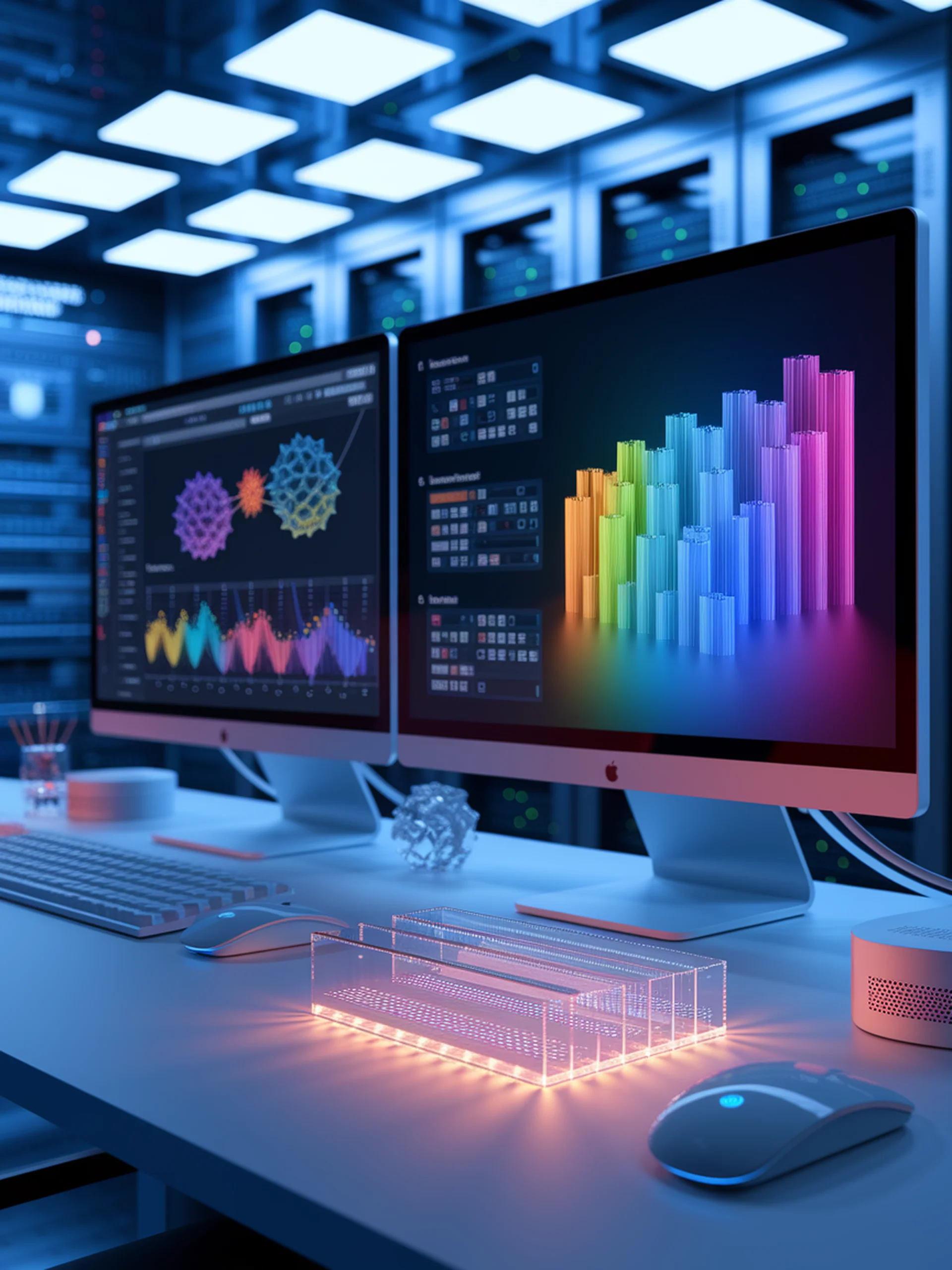
LDAdam: Training Large Models with Less Memory
Memory-efficient optimization through low-dimensional subspaces
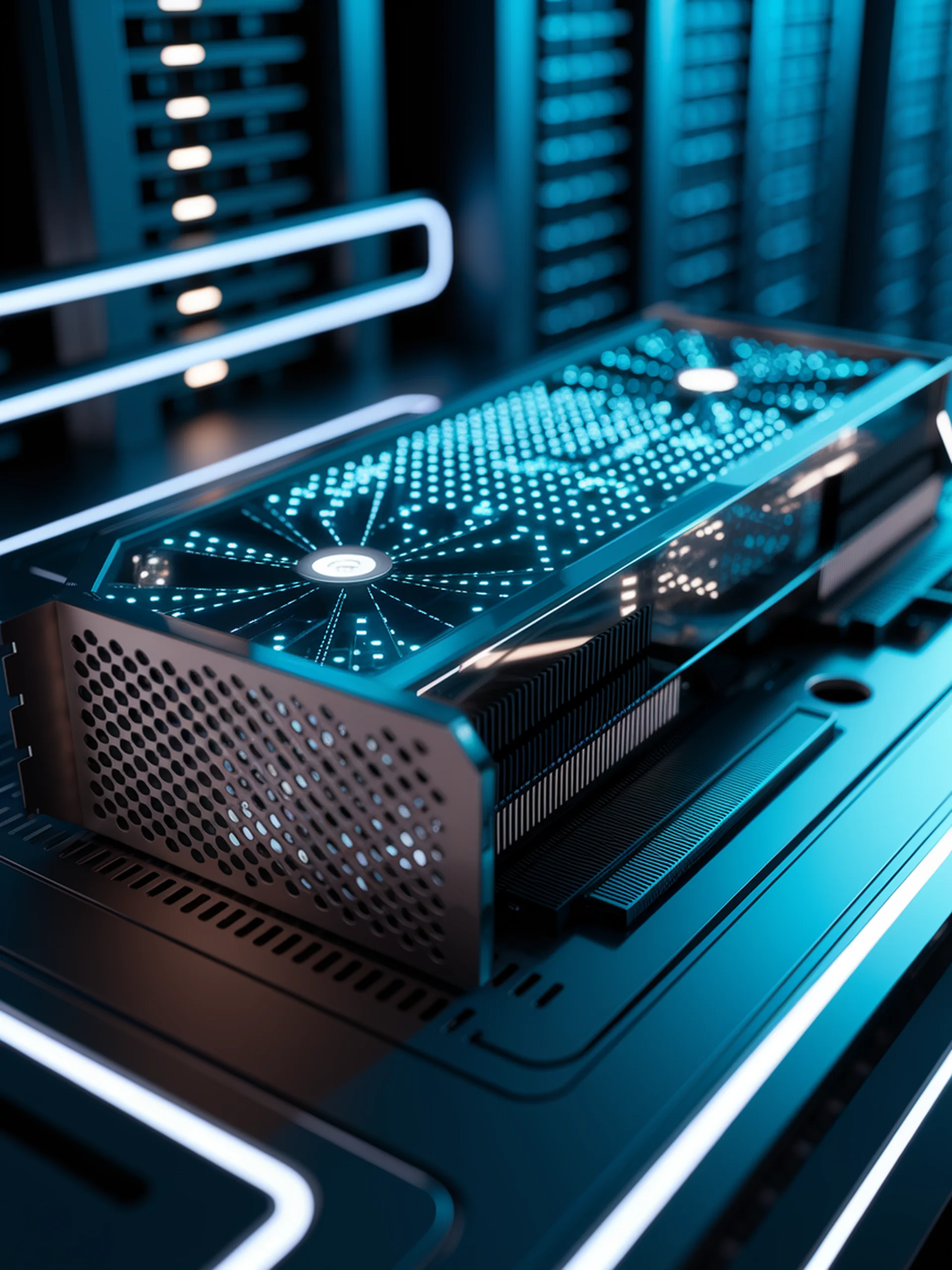
Breaking the 2:4 Barrier in GPU Sparsity
Unlocking V:N:M sparse patterns for faster transformer inference
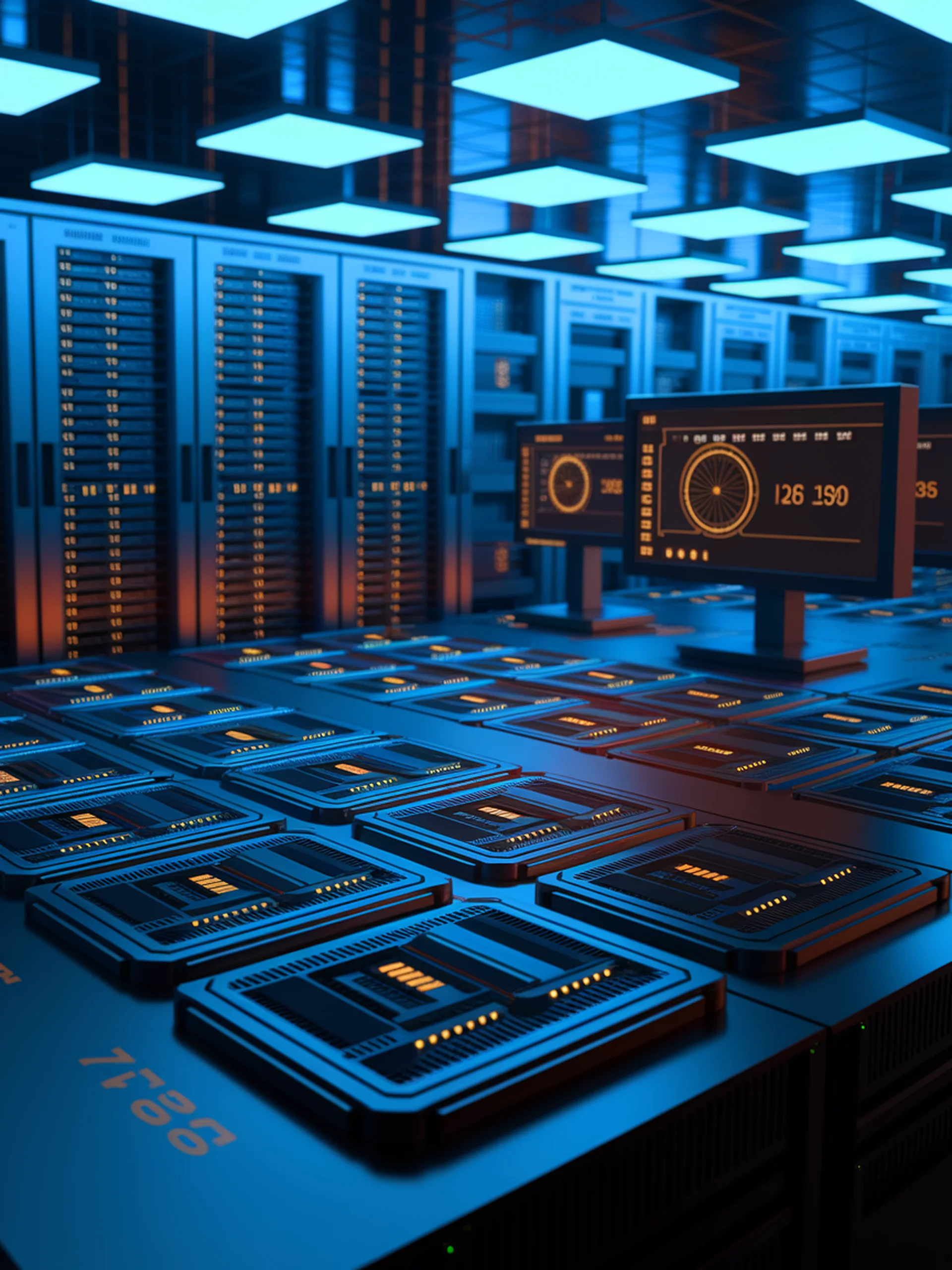
Optimizing LLM Performance Through Smart Scheduling
Practical techniques to maximize GPU utilization and throughput
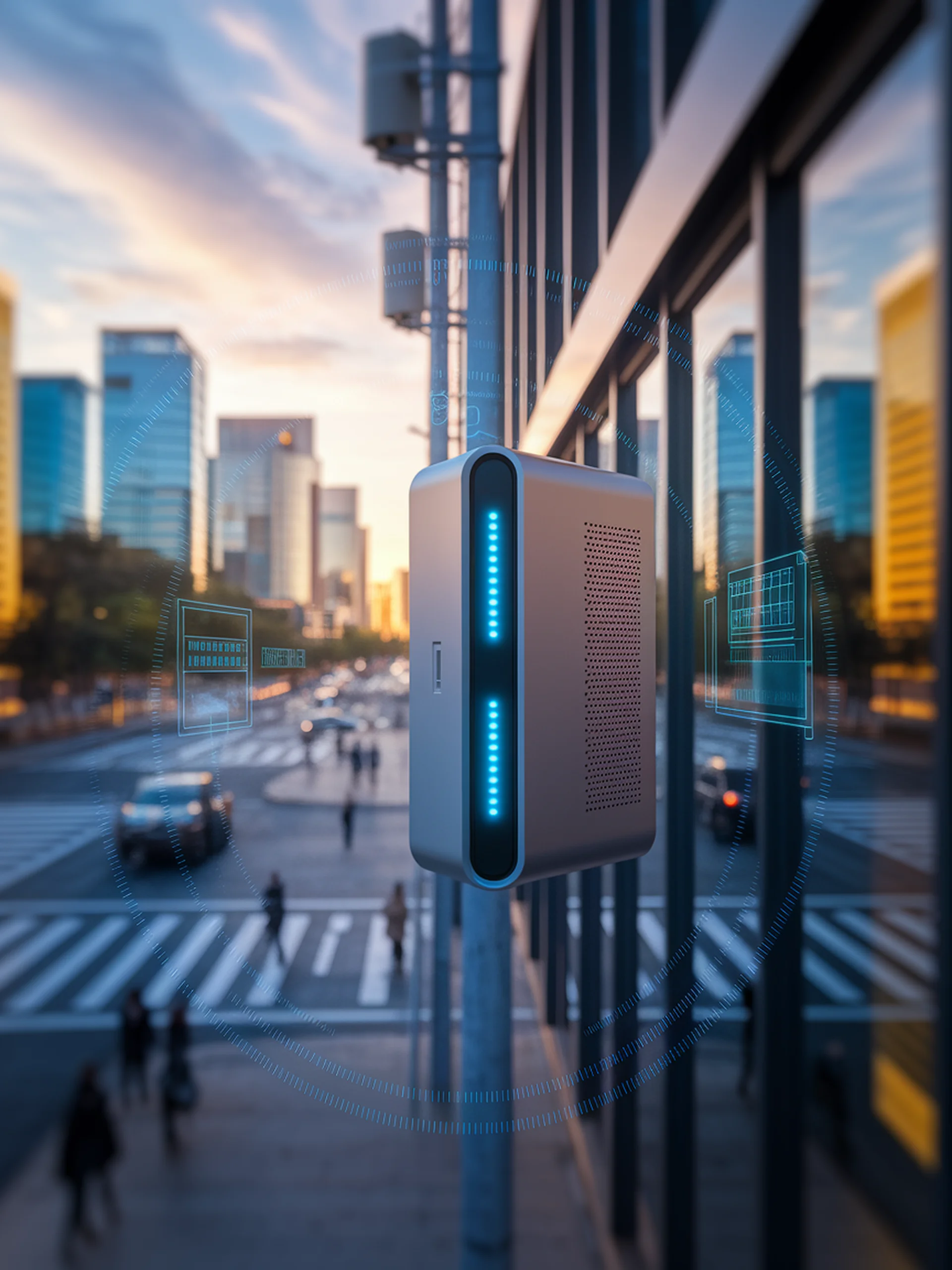
Edge General Intelligence: The Next Frontier
How Large Language Models Are Transforming Edge Computing

Memory-Efficient LLM Training
Revolutionizing FP8 Training with COAT Framework
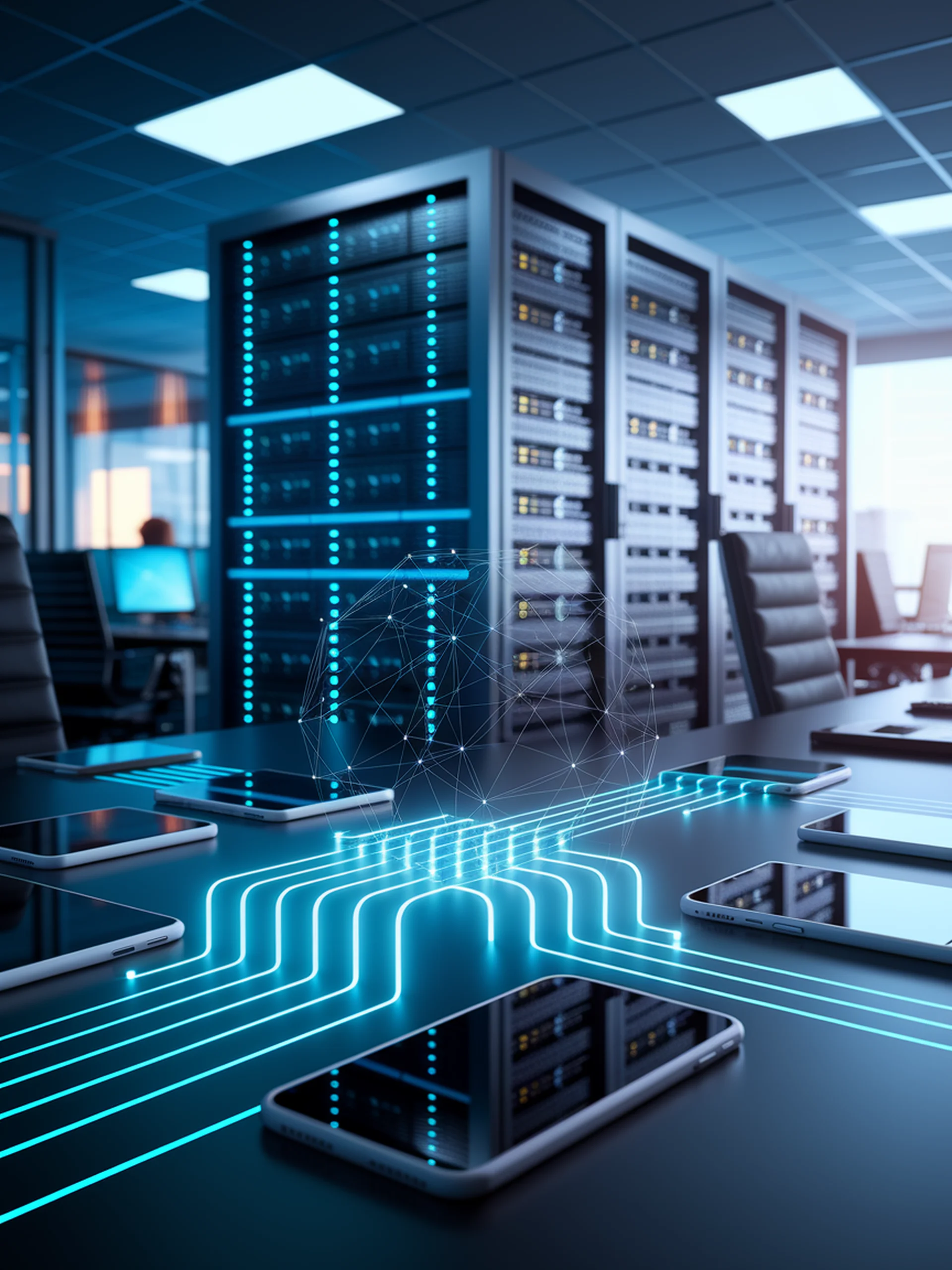
Edge-Cloud LLM Collaboration
Optimizing language models by combining edge devices with cloud resources
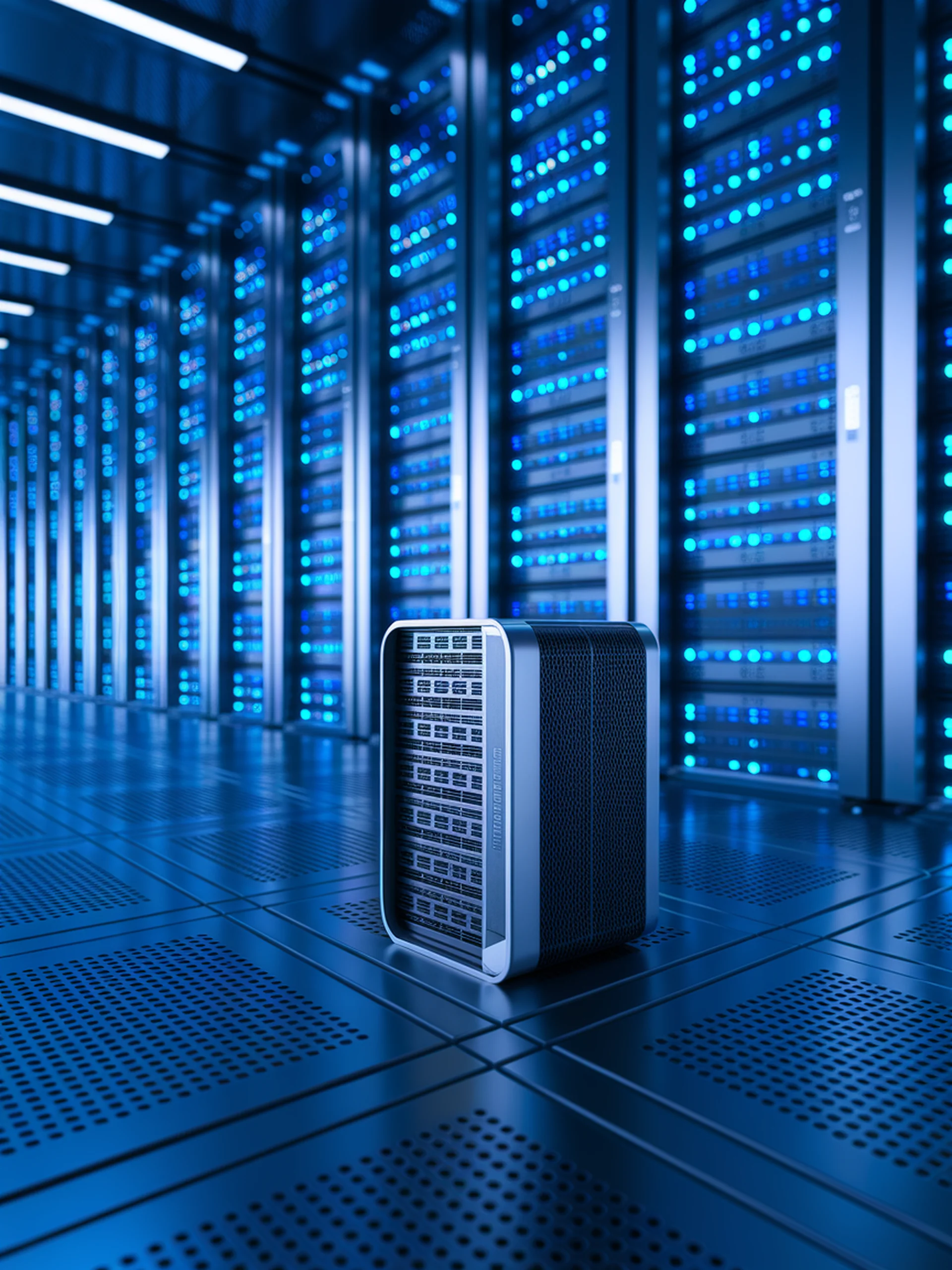
Shrinking LLMs Through Smart Recycling
Making language models smaller and cheaper with innovative parameter sharing
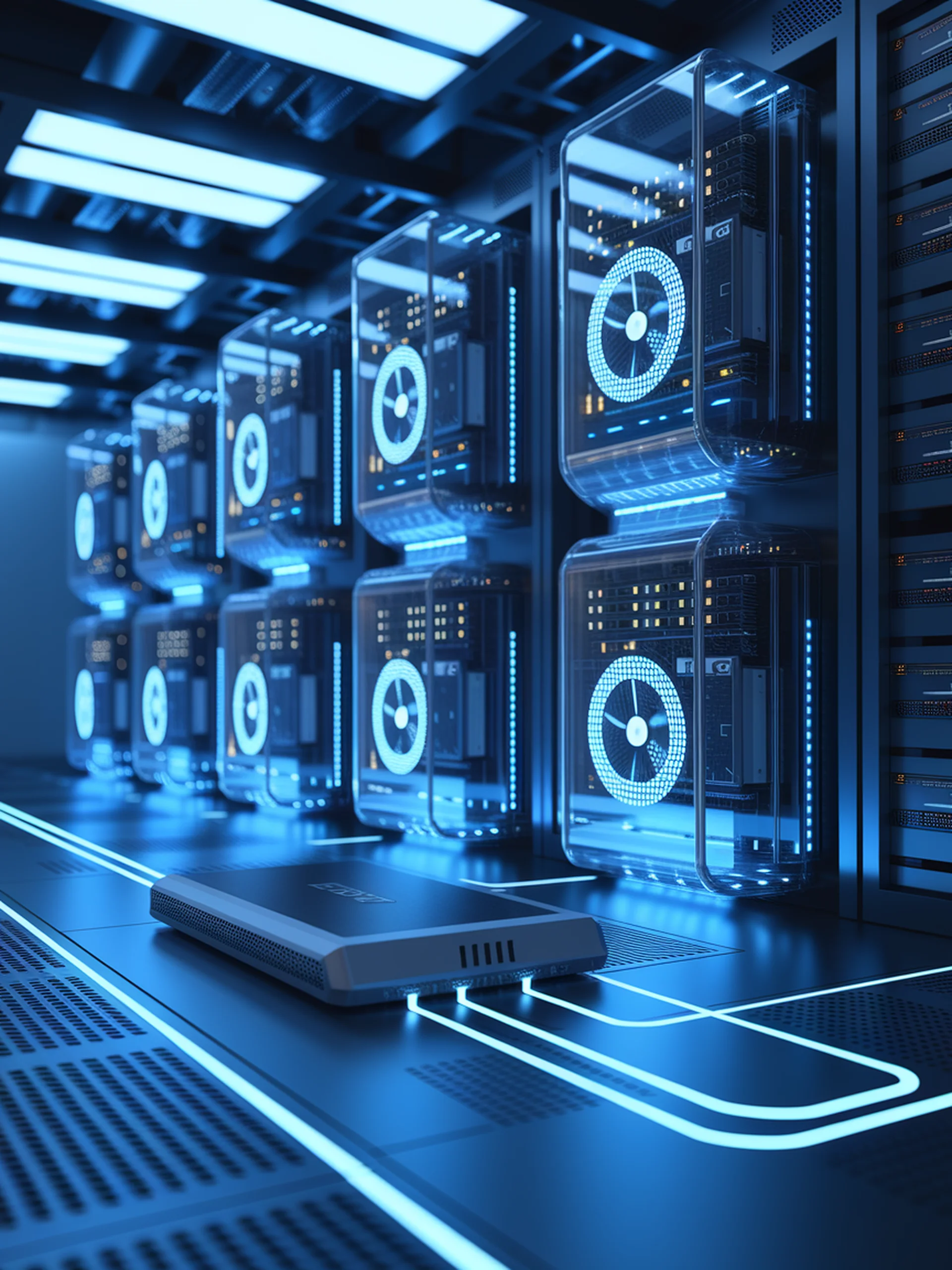
Accelerating LLM Inference with ProMoE
Optimizing MoE models through proactive expert caching
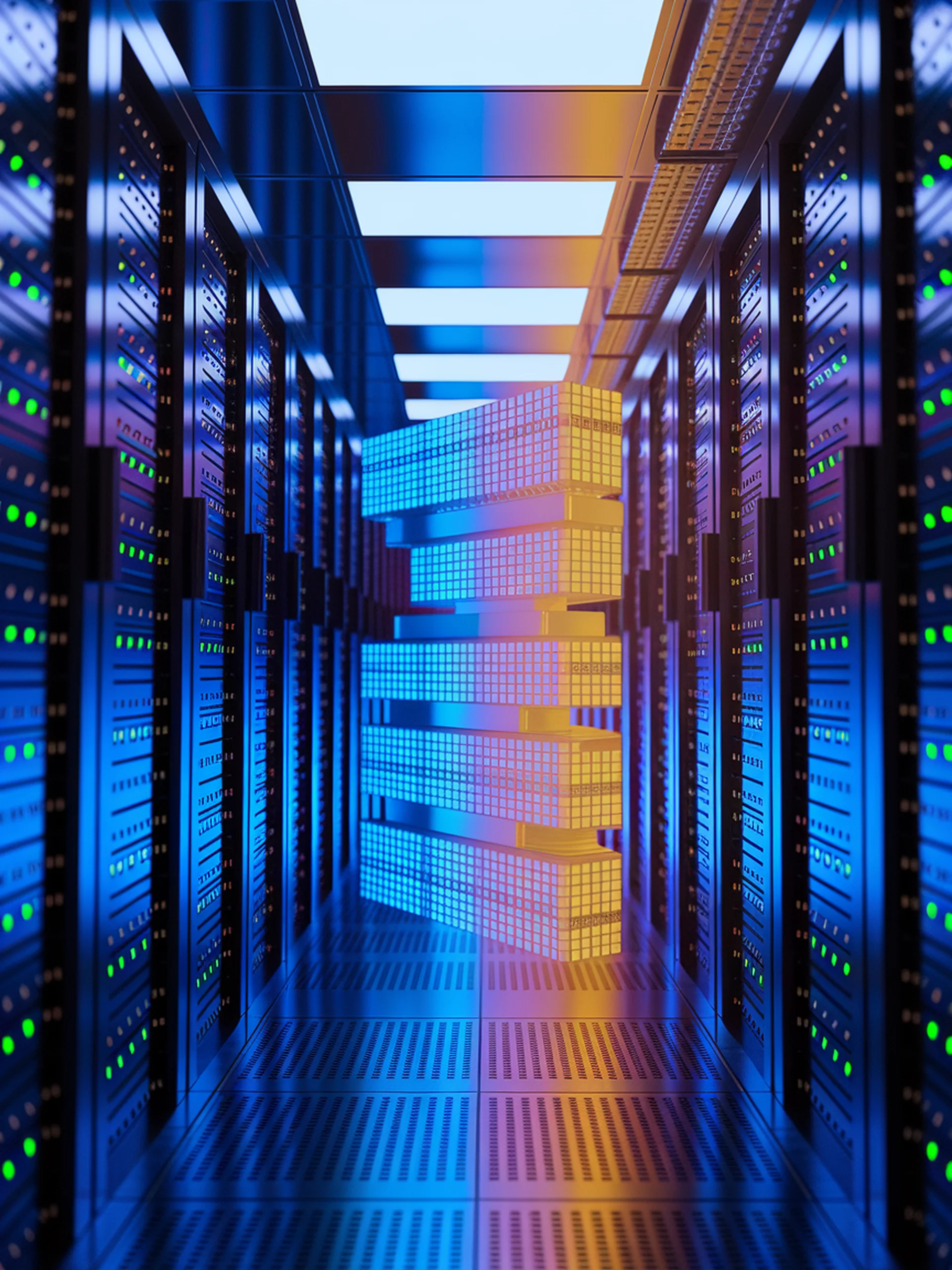
BitStack: Flexible LLM Compression
Adaptive compression for variable memory environments
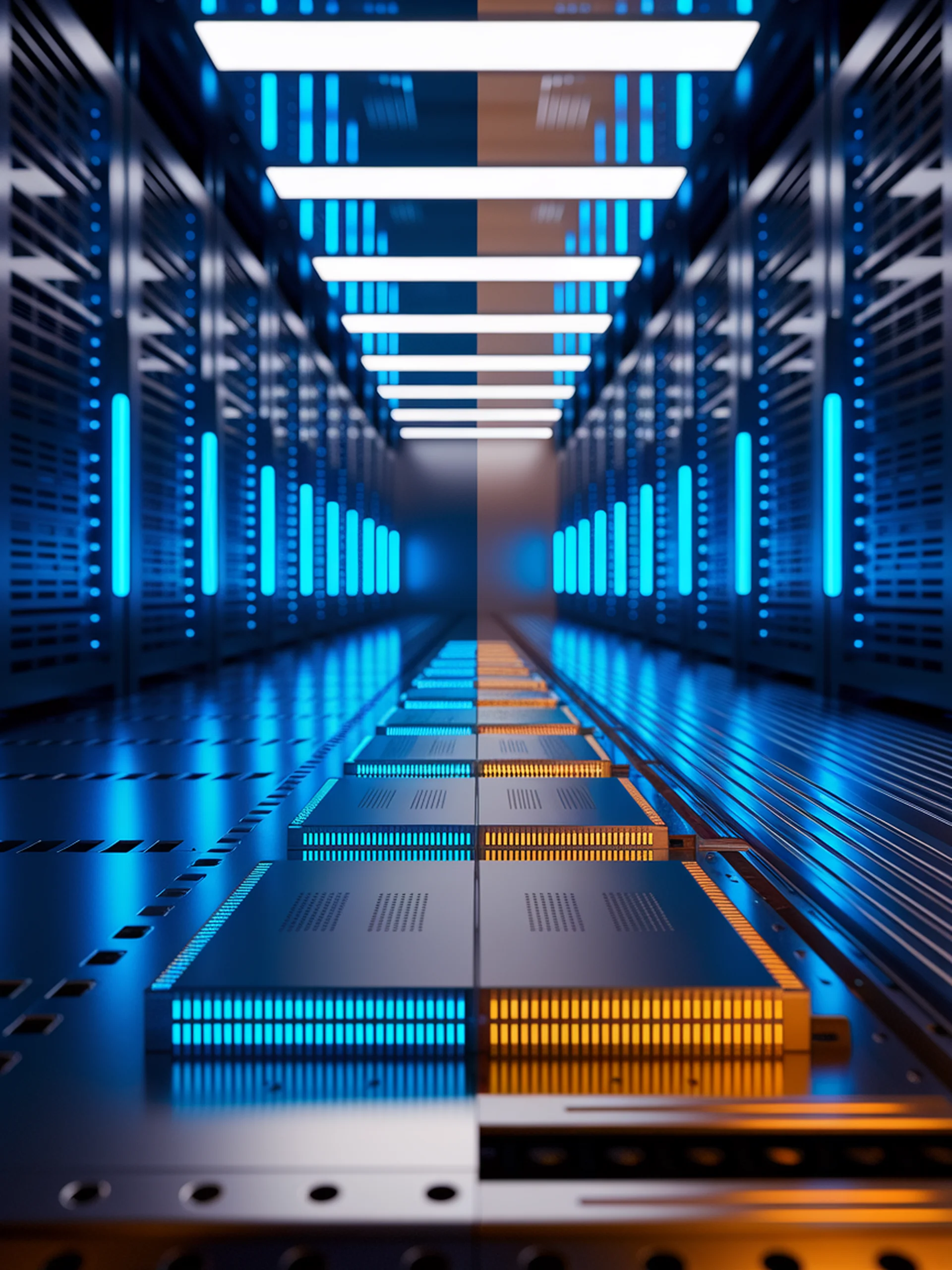
Quantization Precision vs. Performance
The definitive guide to LLM efficiency trade-offs
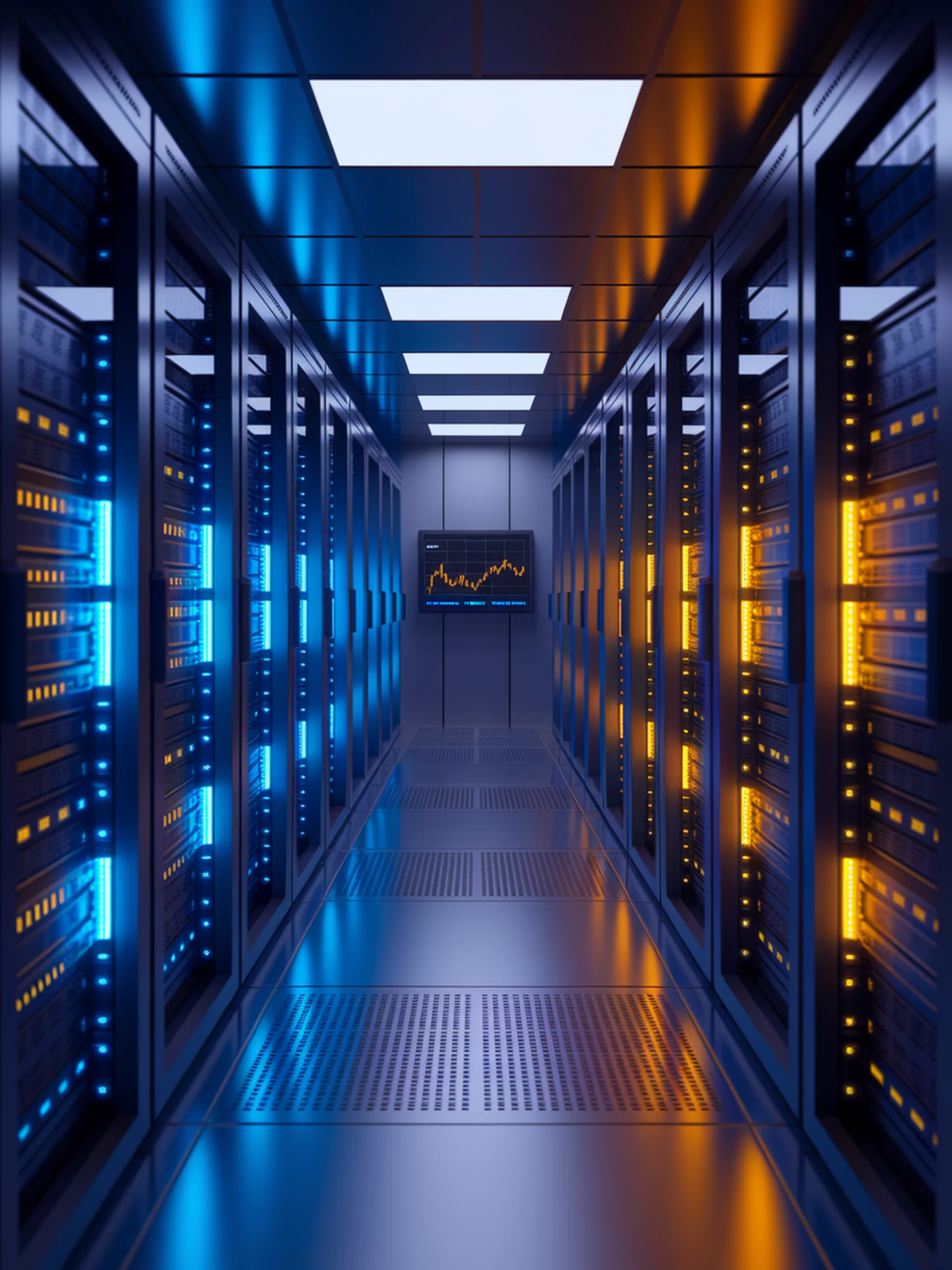
Optimizing LLM Serving Resources
Balancing GPU Compute and Key-Value Cache for Efficient LLM Deployment
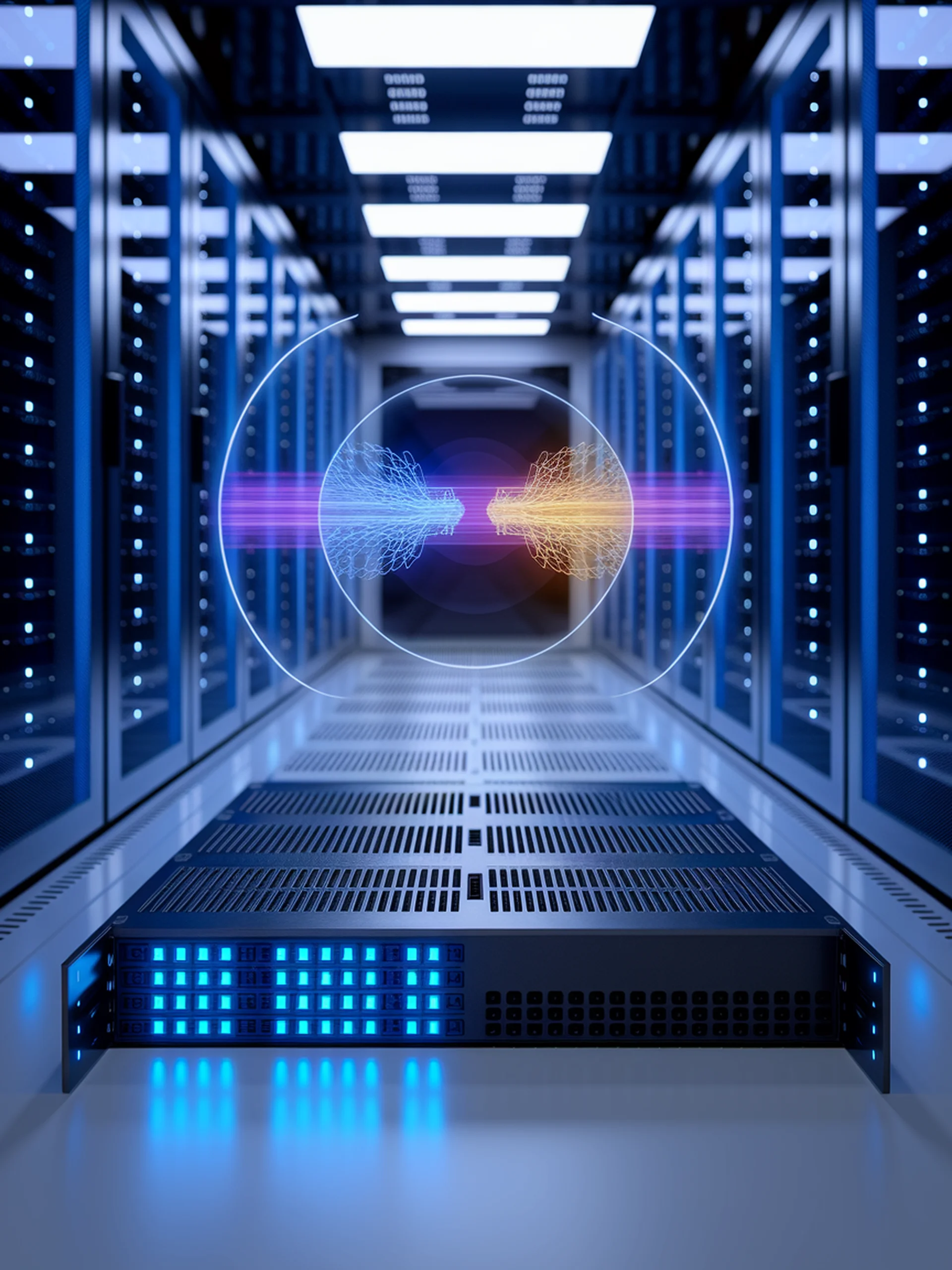
Efficient LLM Compression
Combining LoRA and Knowledge Distillation for Smarter AI Compression
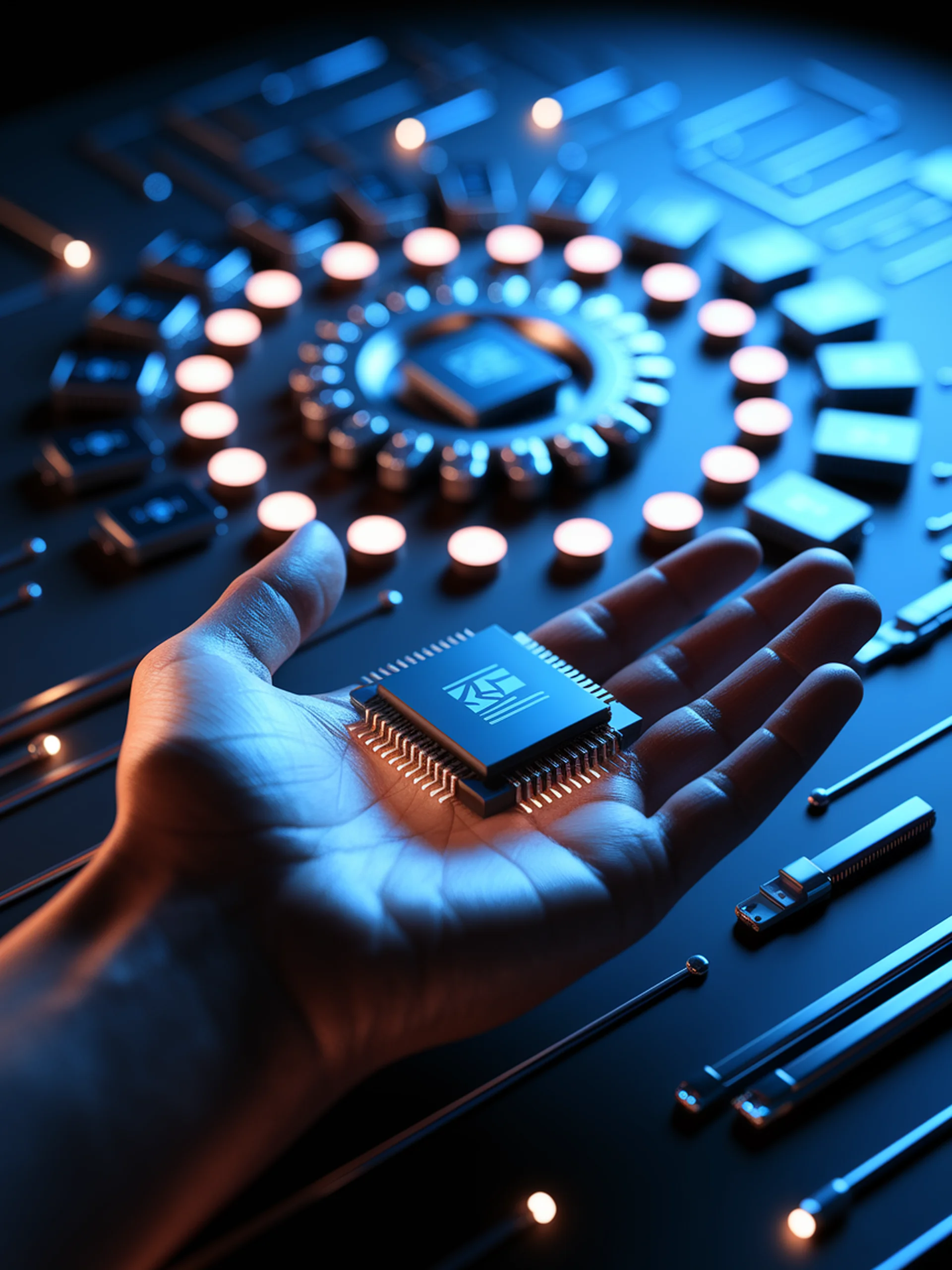
Bringing LLMs to Resource-Constrained Devices
Efficient federation learning techniques for tiny transformers
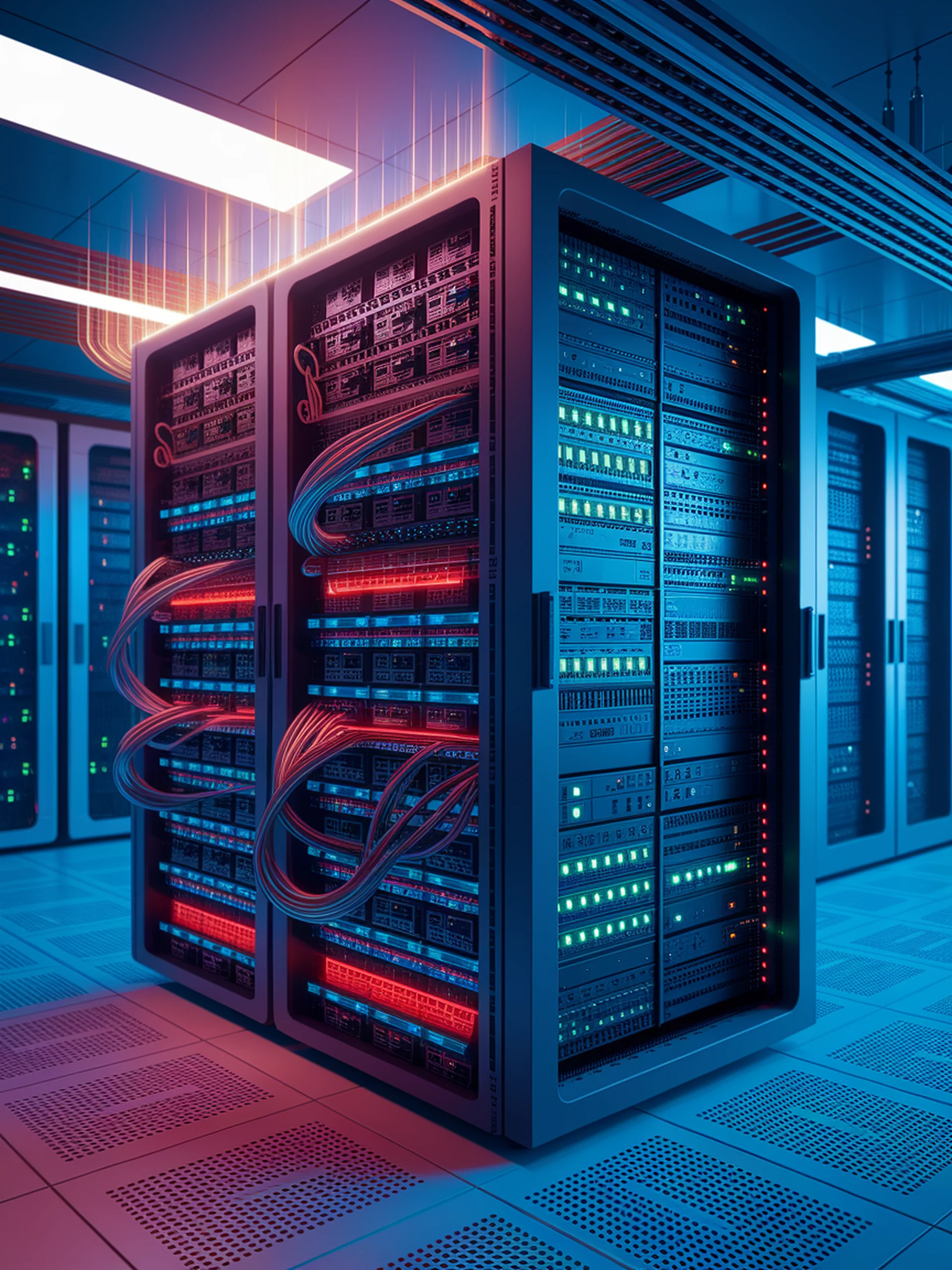
Slashing Memory Costs in LLM Training
How Cut Cross-Entropy dramatically reduces memory footprint without sacrificing performance
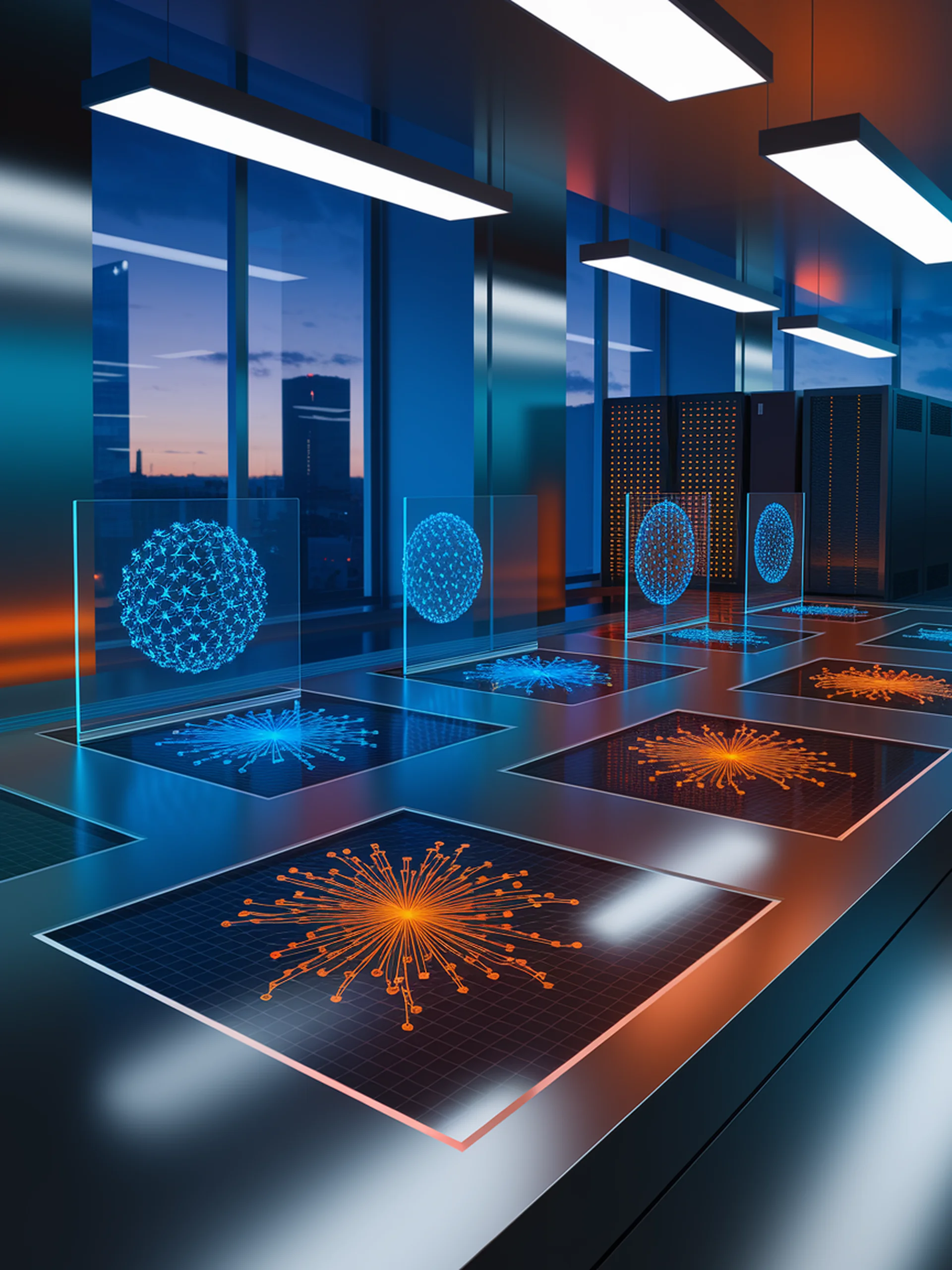
Optimizing LLM Reasoning with Sparse Attention
Reducing computational costs while maintaining reasoning quality
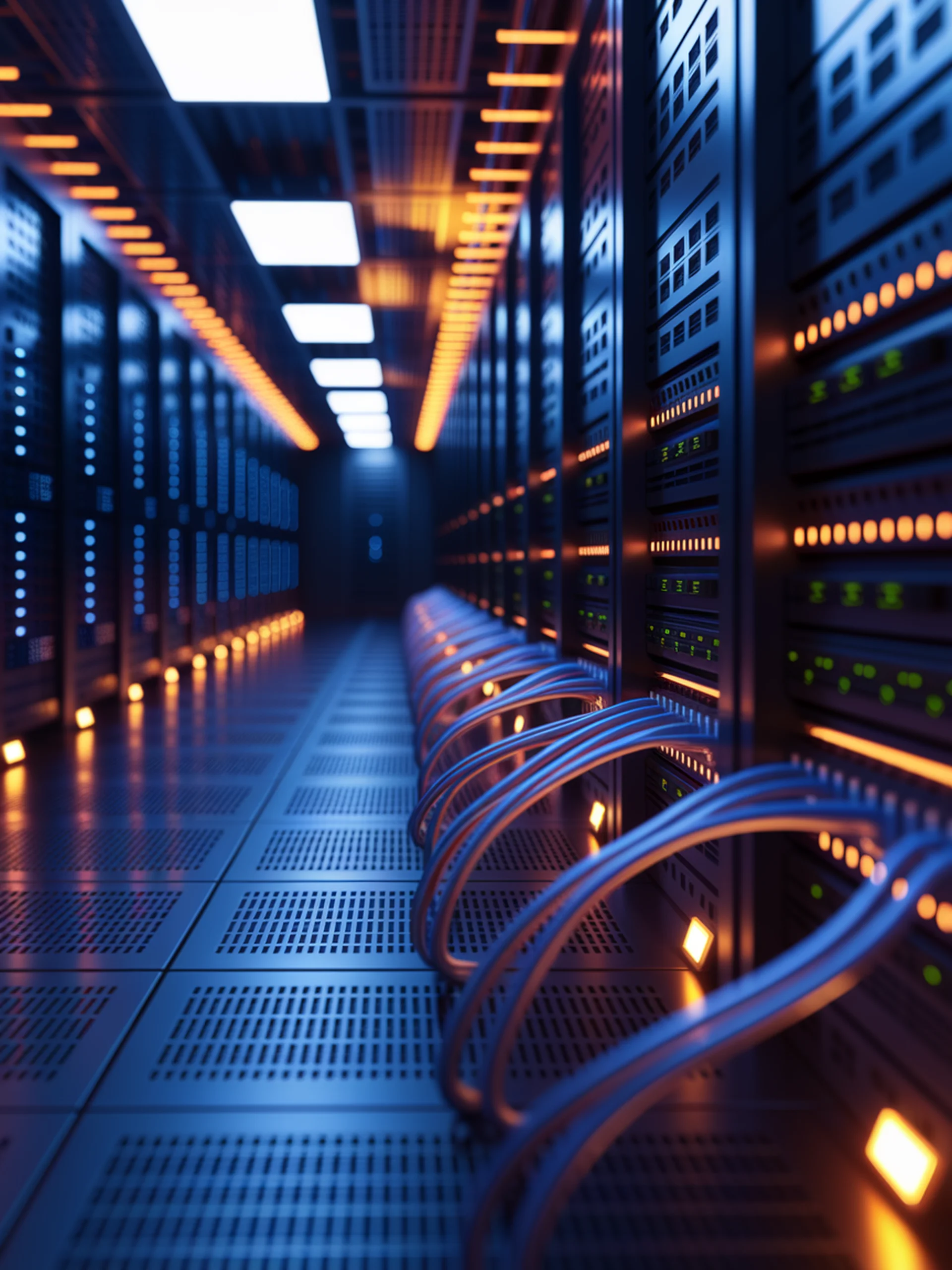
The Scaling Plateau in LLM Training
Diminishing returns challenge hardware efficiency in distributed AI systems
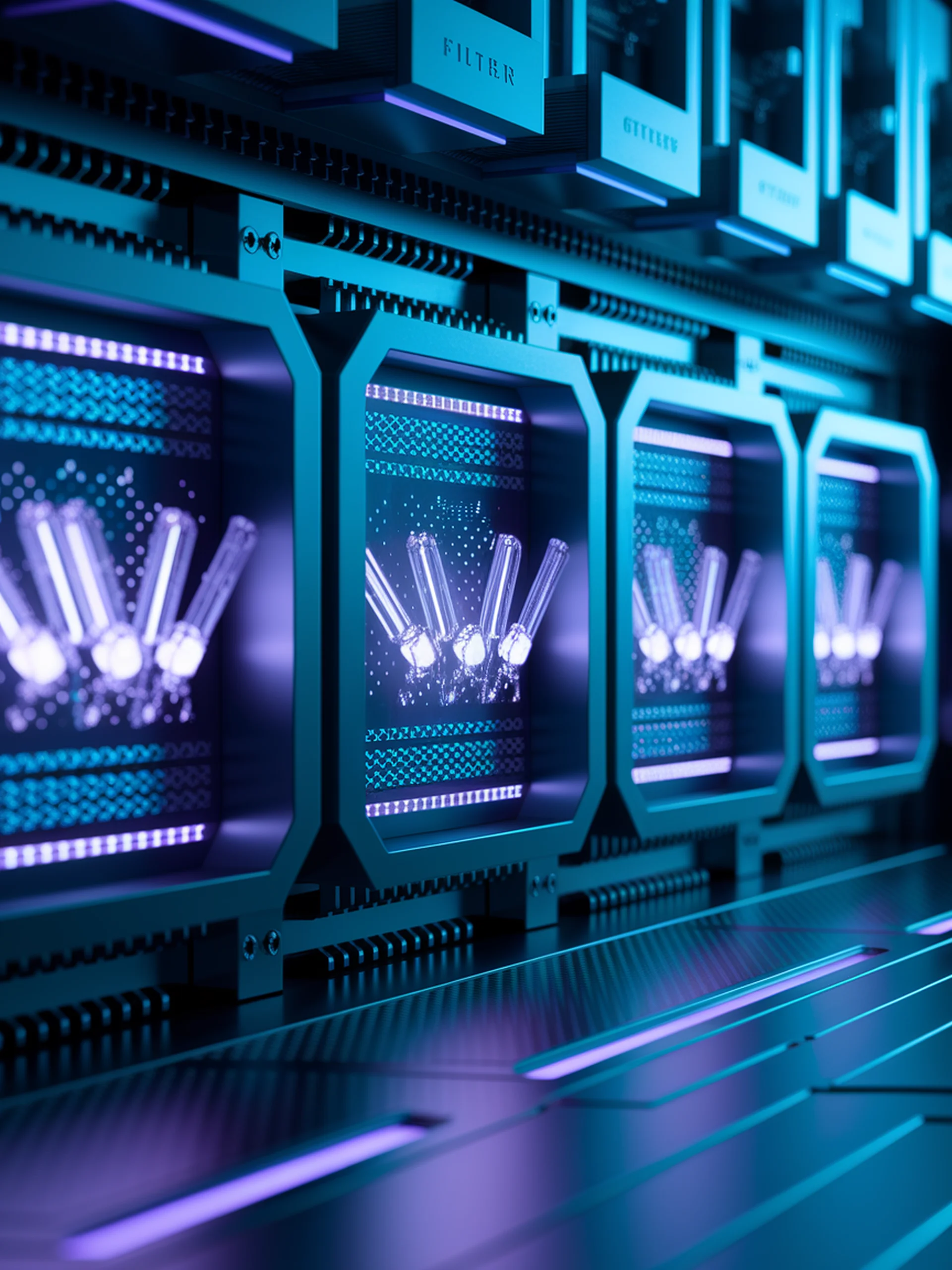
Accelerating Multimodal LLMs
Training-Free Token Reduction for Efficient MLLM Deployment
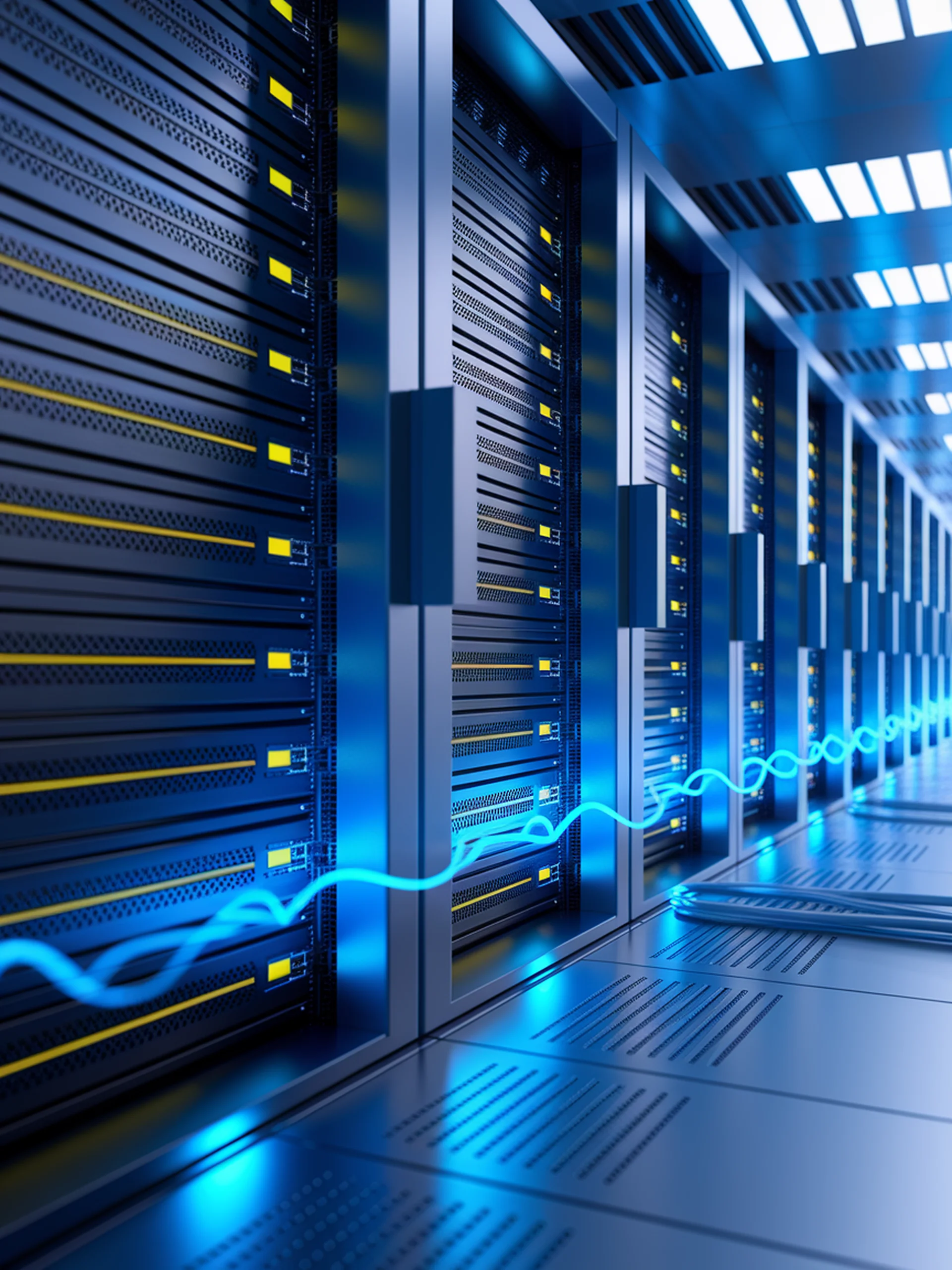
Accelerating LLM Services in Wireless Networks
Optimizing prompts and power for faster, more efficient LLM deployment
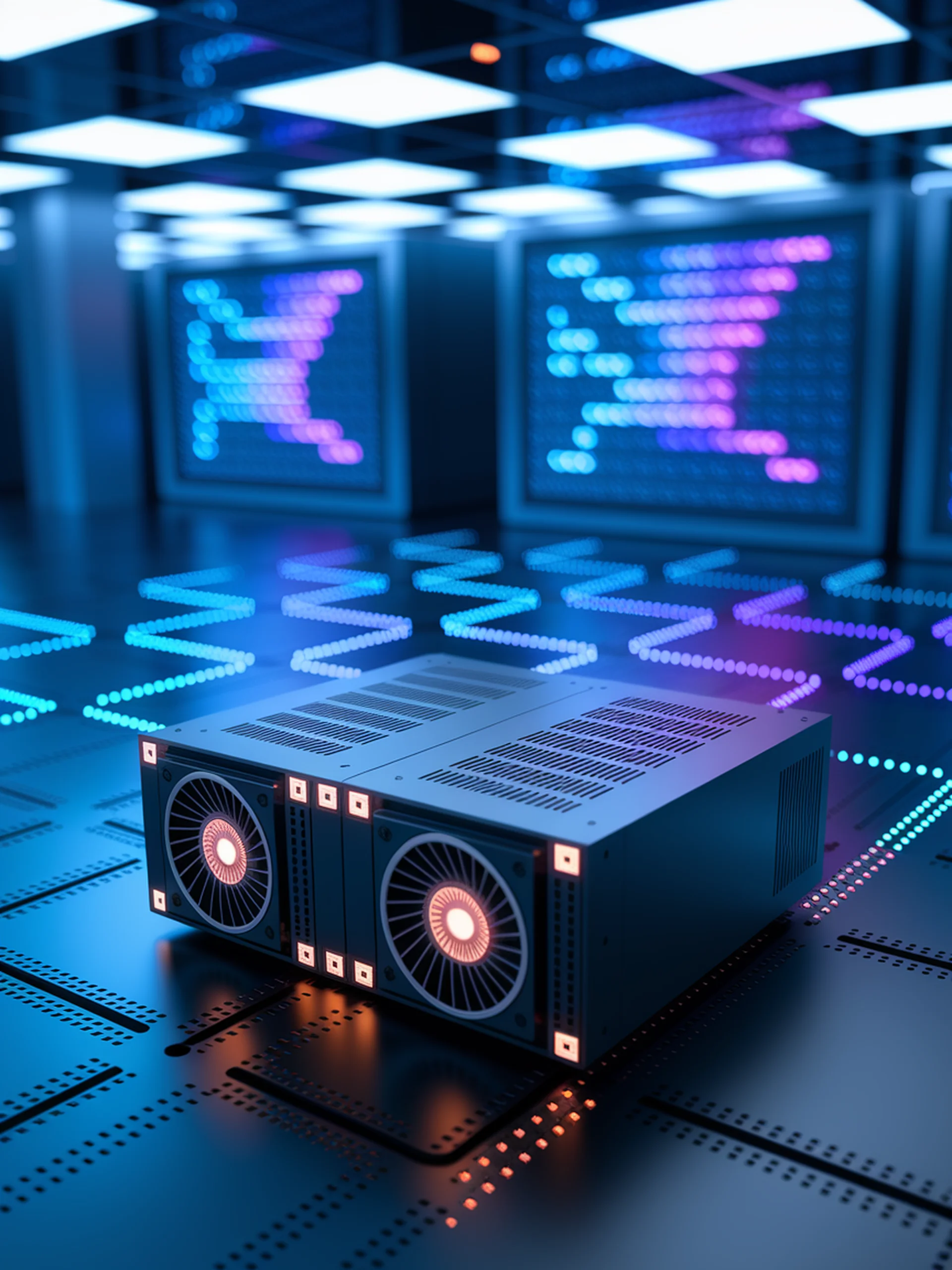
Accelerating LLM Inference with Puzzle
Hardware-aware optimization that preserves model capabilities
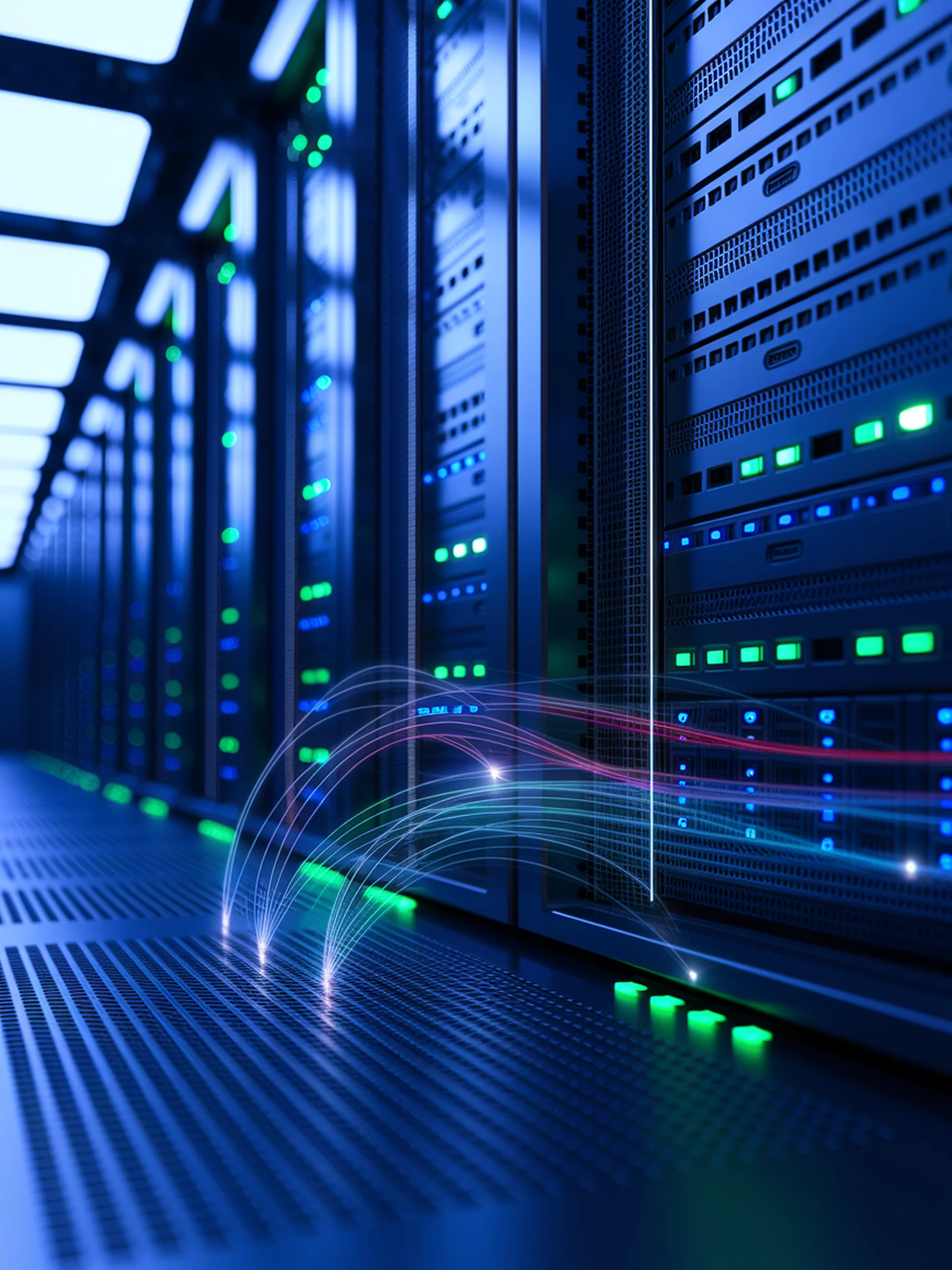
Prefix Caching for Hybrid LLMs
Optimizing Performance in Modern Language Models
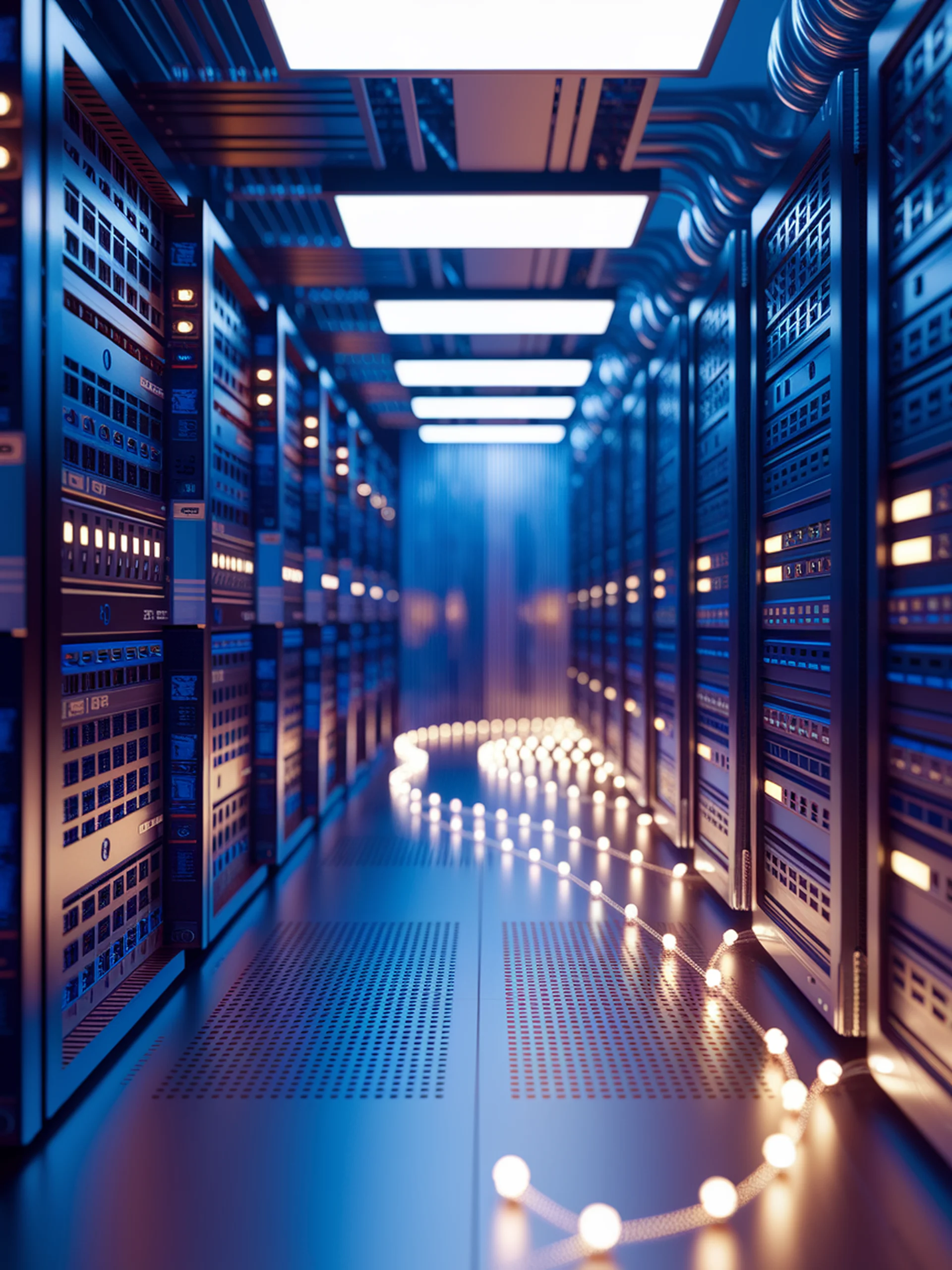
Smarter Model Compression for LLMs
Going beyond pruning with ConDense-MoE architecture
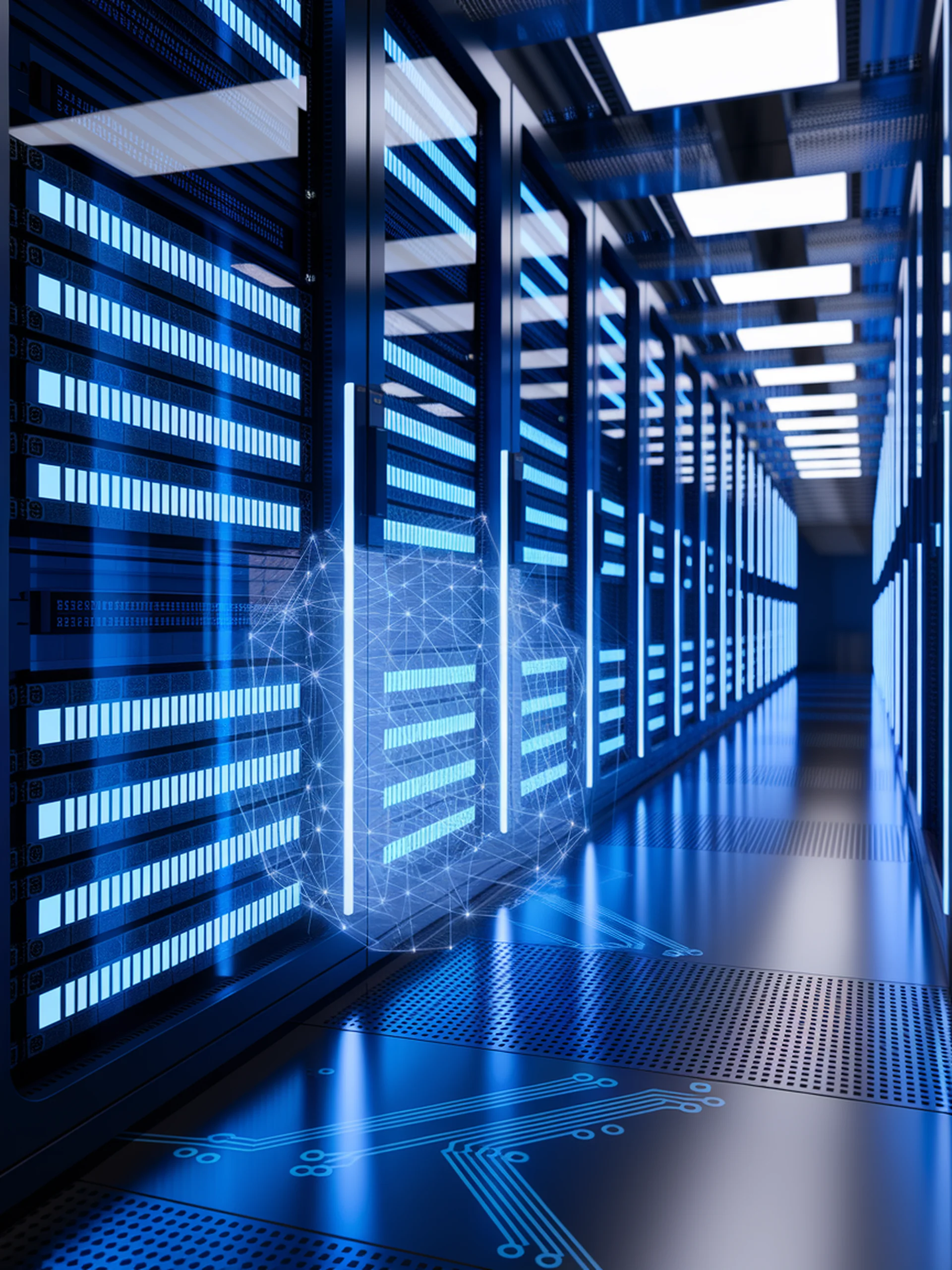
Smarter Memory Usage for AI Training
Correlation-Aware Projection Reduces Memory Needs While Preserving Performance
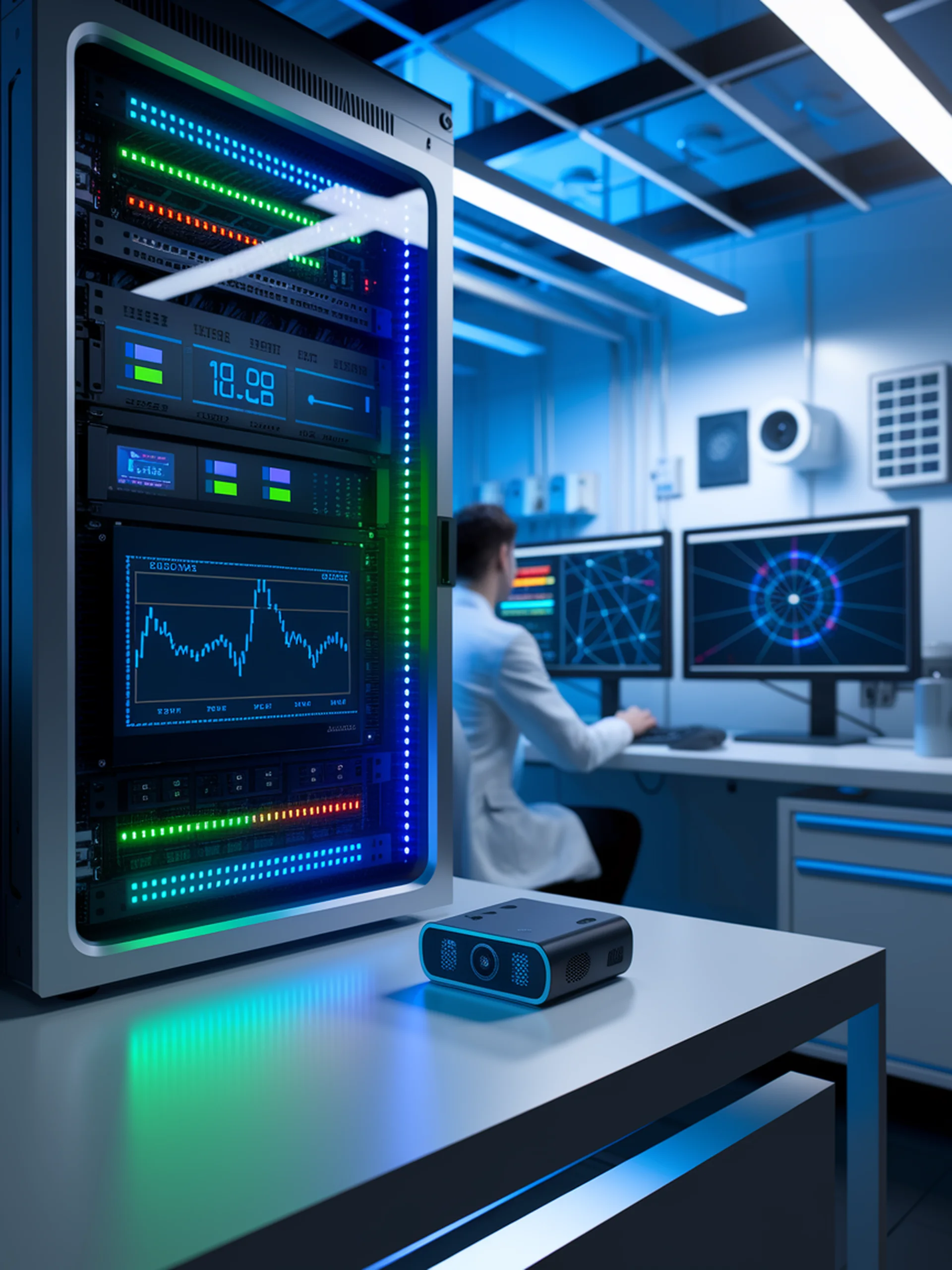
Dynamic Context Sparsification for MLLMs
Accelerating multimodal models for real-time applications
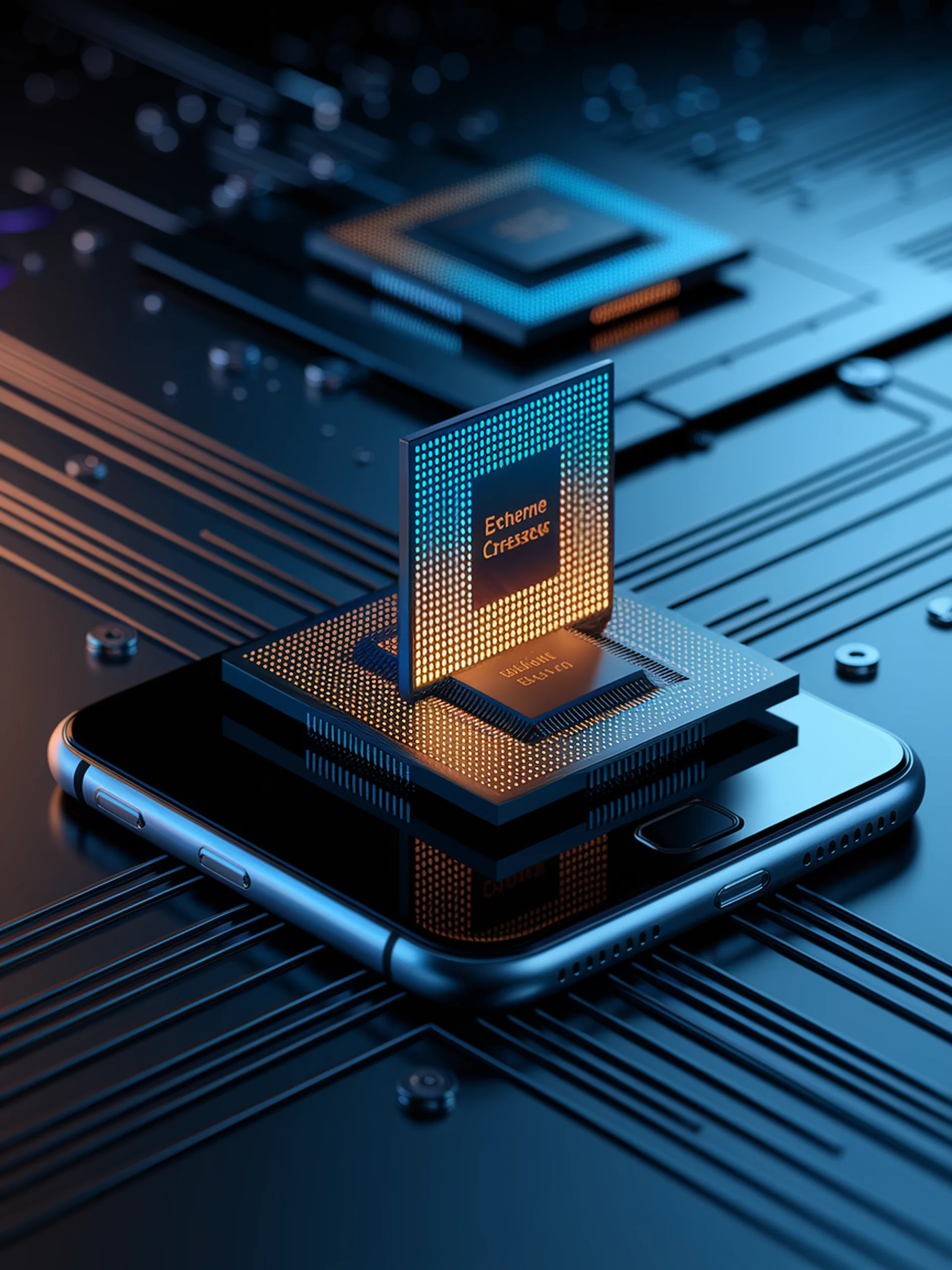
Boosting Ultra-Low-Bit LLMs
A breakthrough in 2-bit model performance with RILQ
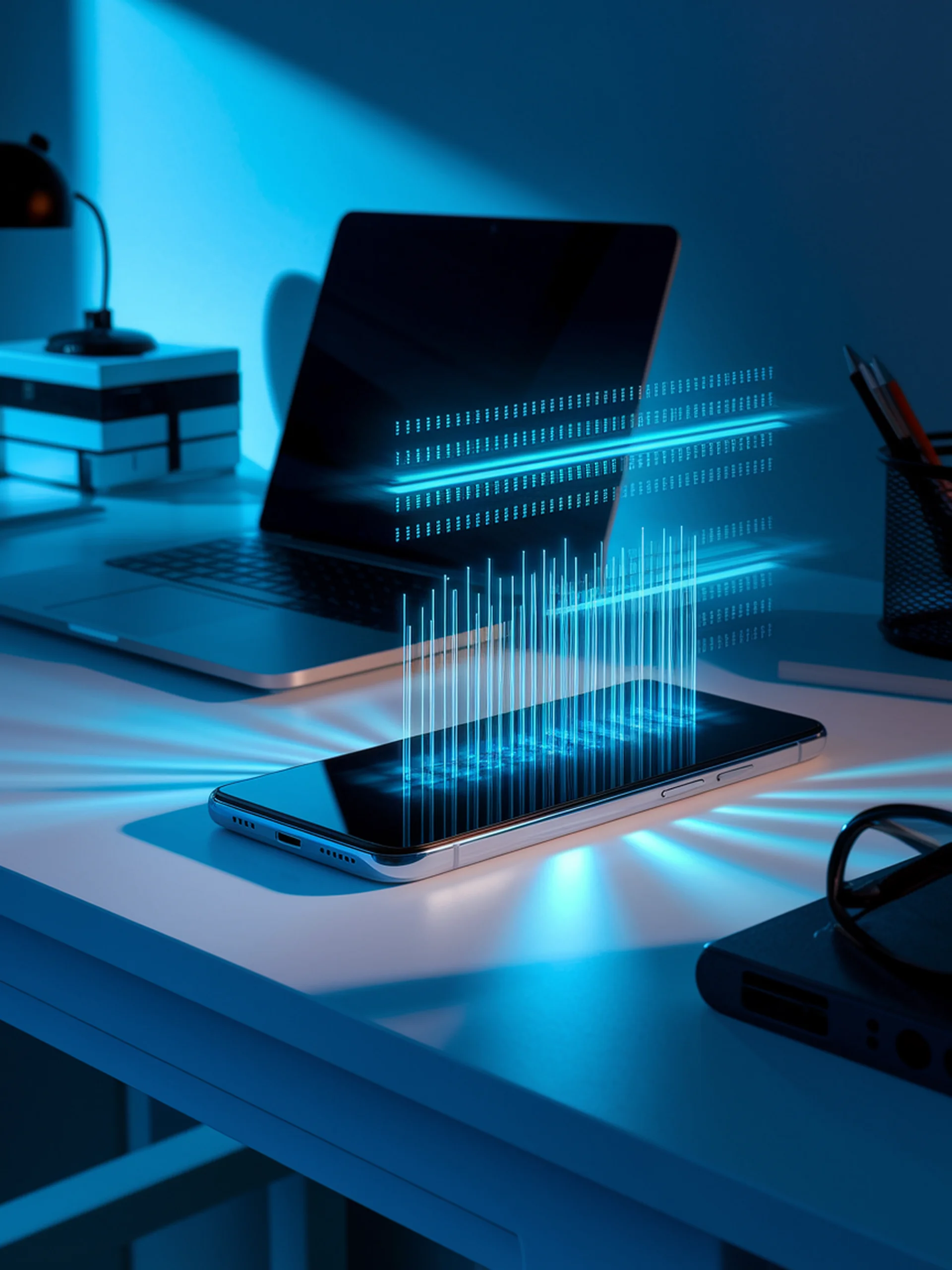
Boosting LLM Speed on Mobile Devices
Overcoming Memory Bottlenecks with Smart Pruning Techniques
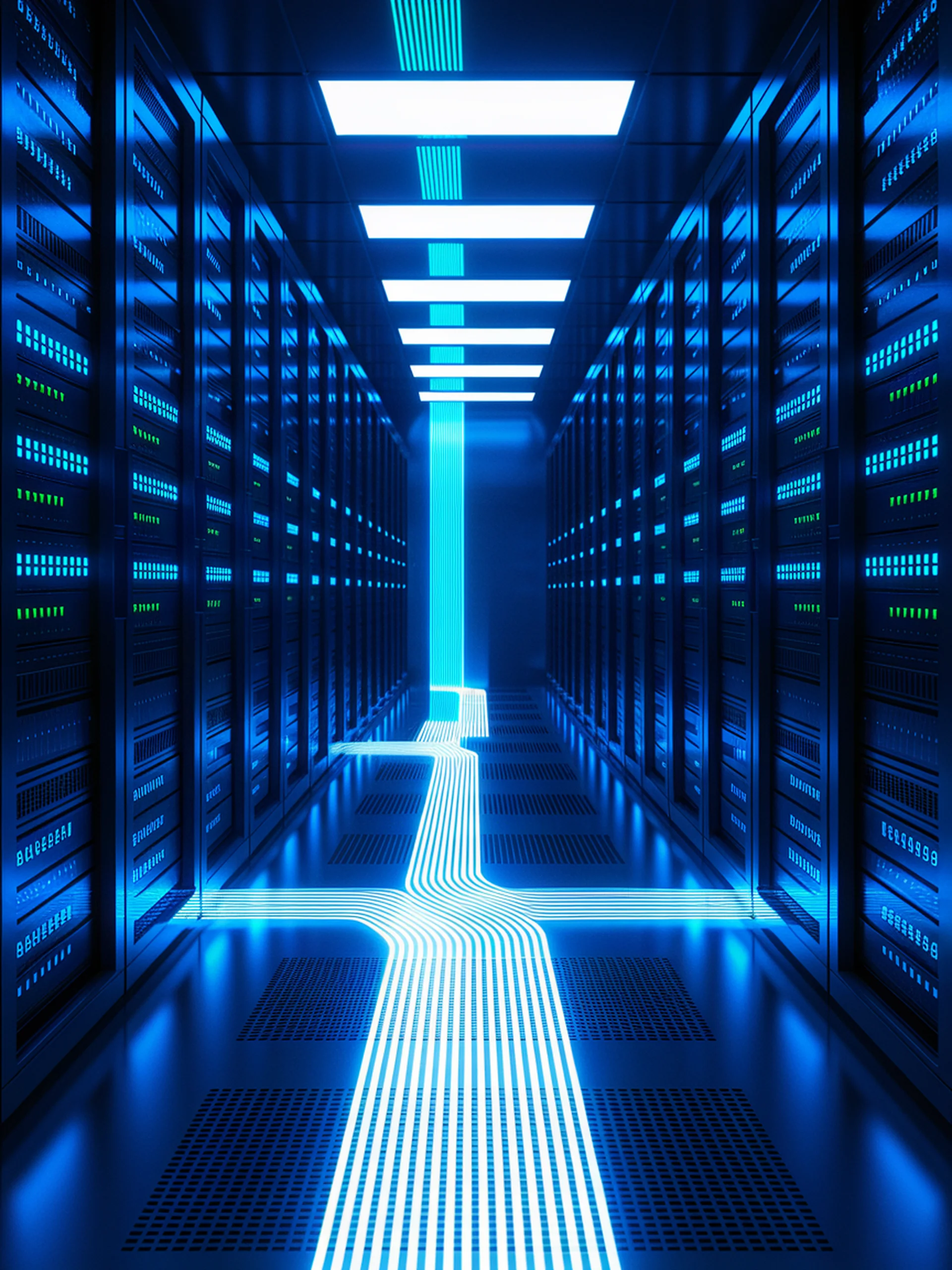
Accelerating Long-Context LLM Training
Flexible Sequence Parallelism for Heterogeneous Inputs
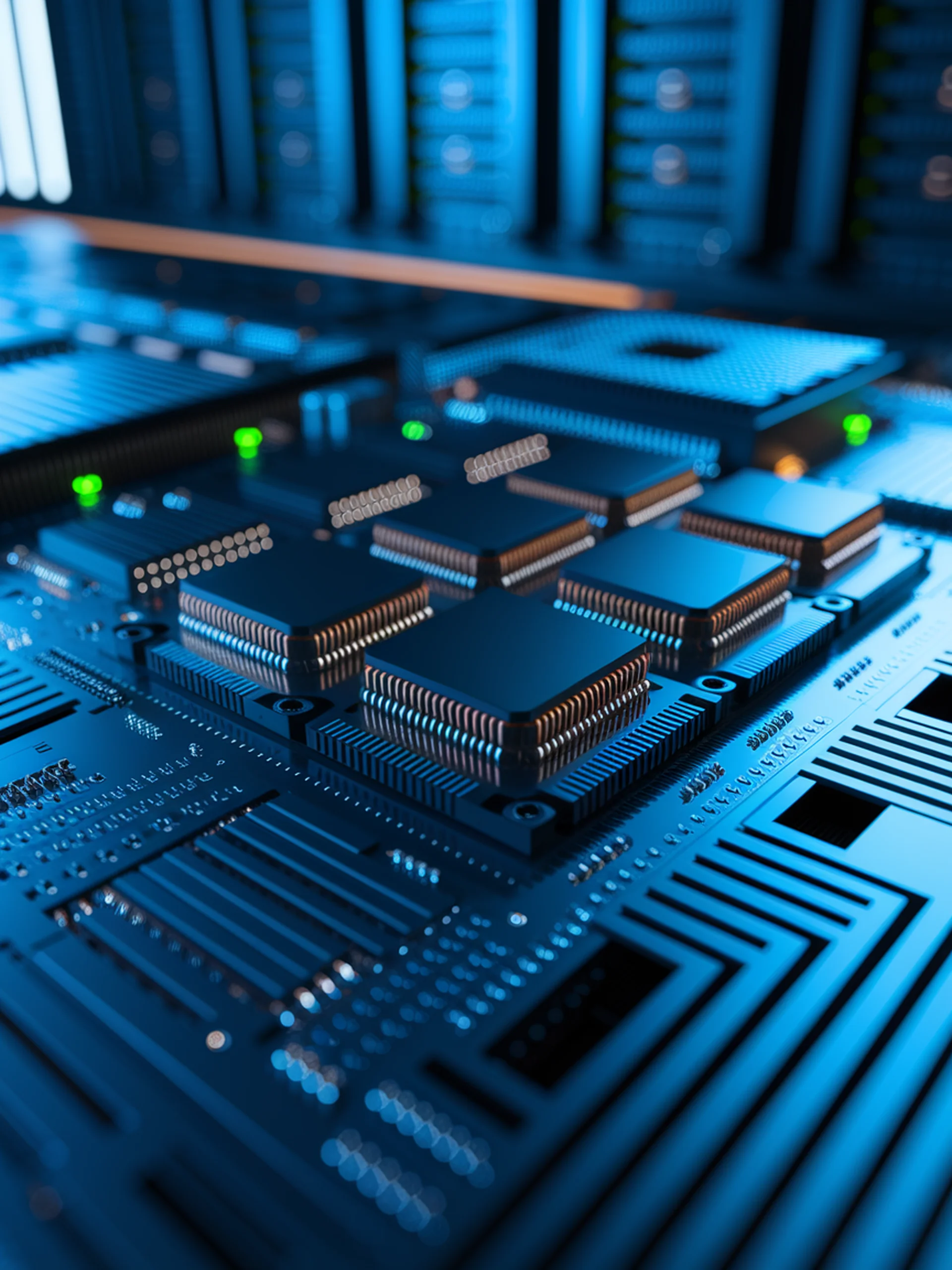
Smarter Memory for Smarter AI
A novel approach to reducing LLM memory bottlenecks
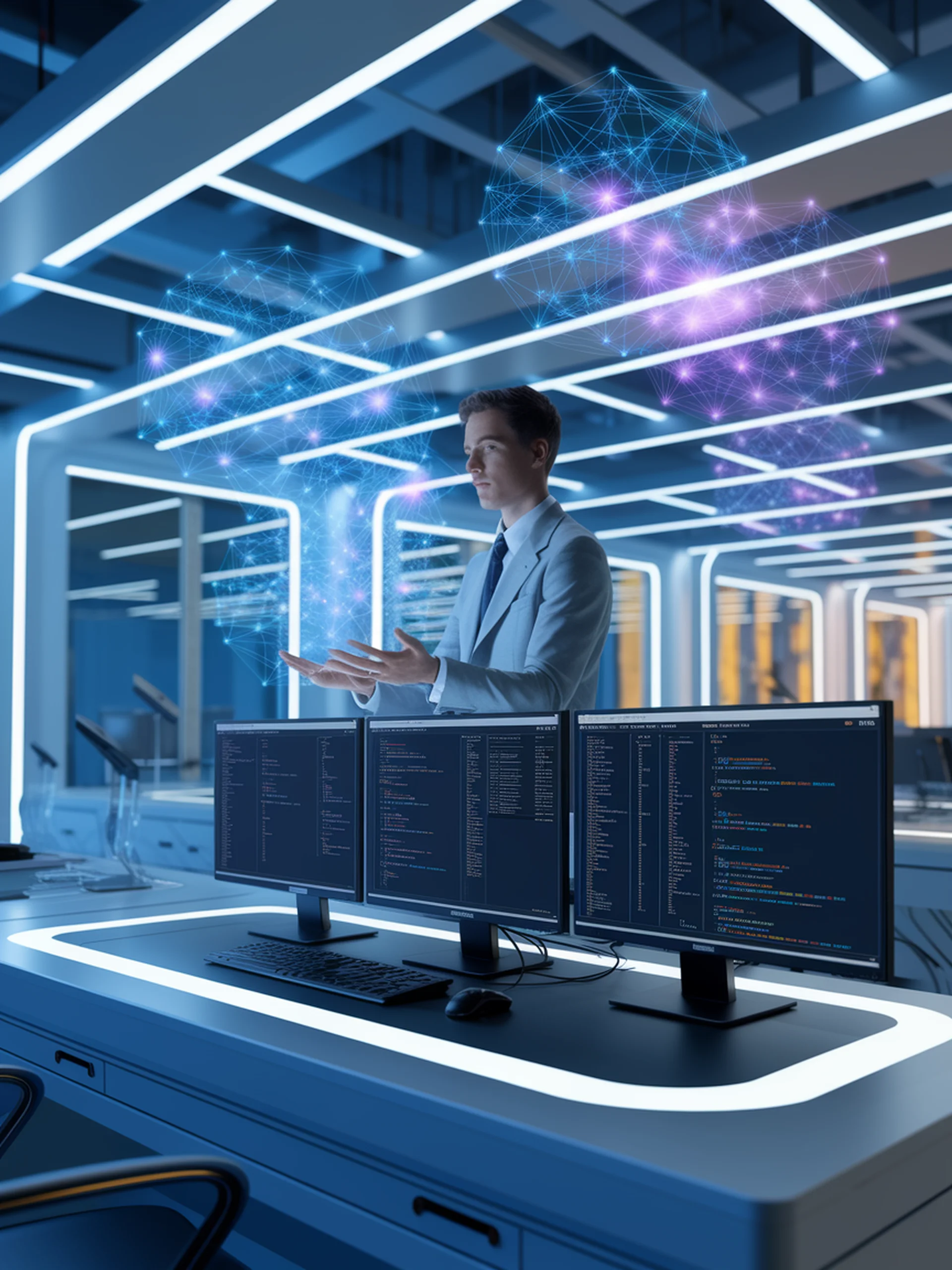
Efficient LLM Adaptation with KaSA
Knowledge-aware parameter-efficient fine-tuning for LLMs
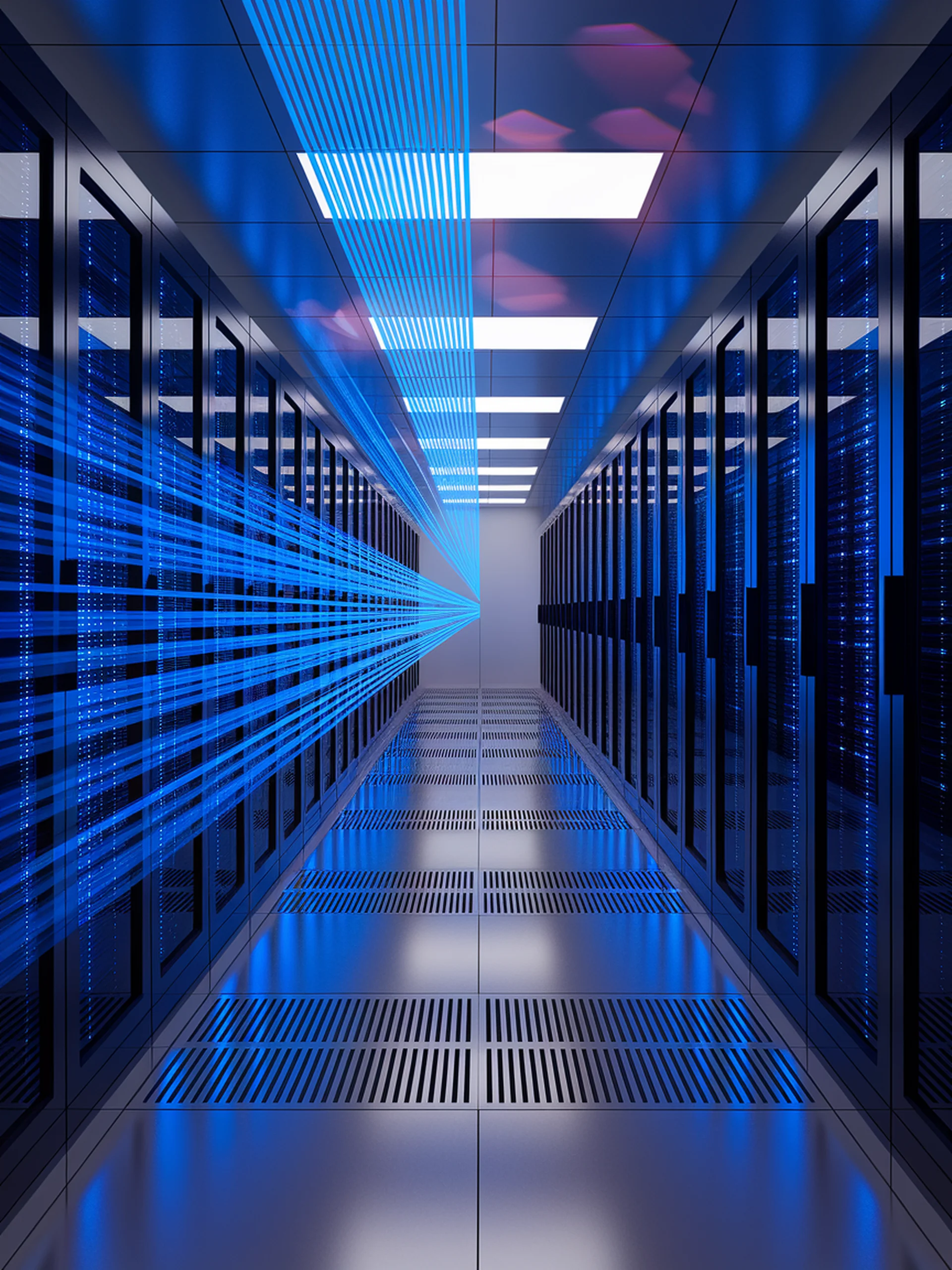
Breaking the Communication Bottleneck in LLM Training
EDiT: A More Efficient Approach to Distributed LLM Training
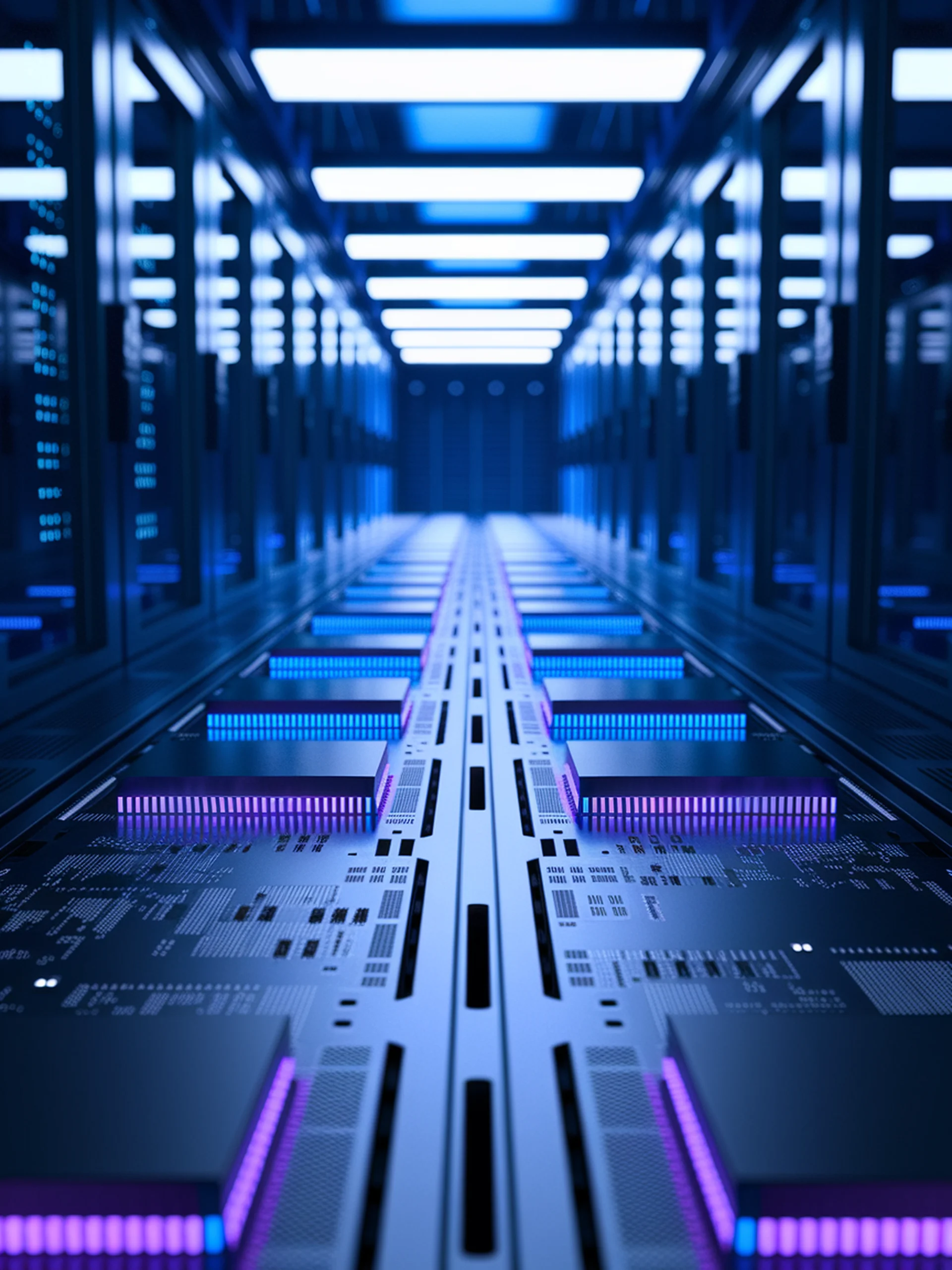
Optimizing LLM Memory with EMS
A novel adaptive approach to KV cache compression
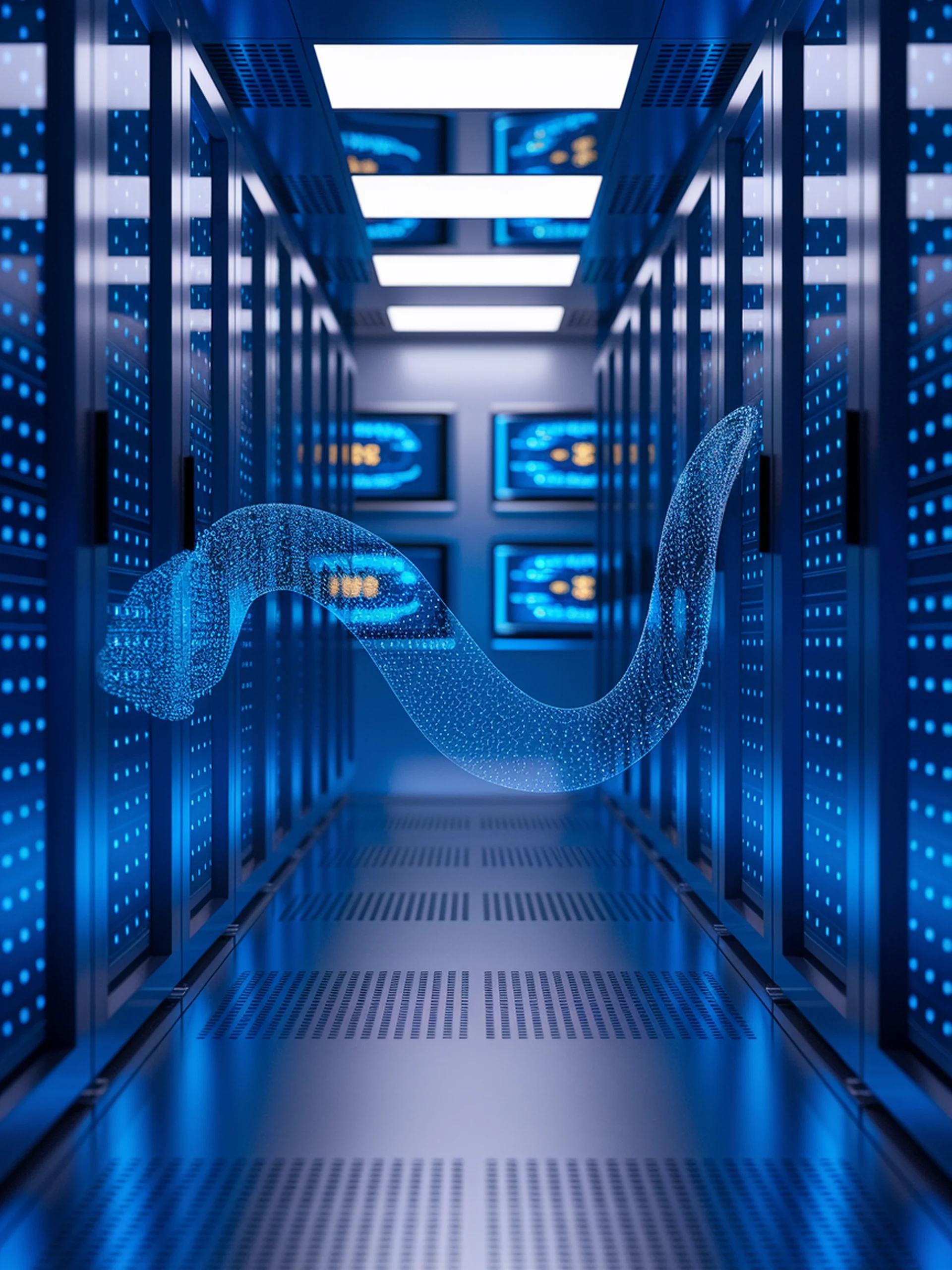
Accelerating LLMs with Separator Compression
Reducing computational demands by transforming segments into separators
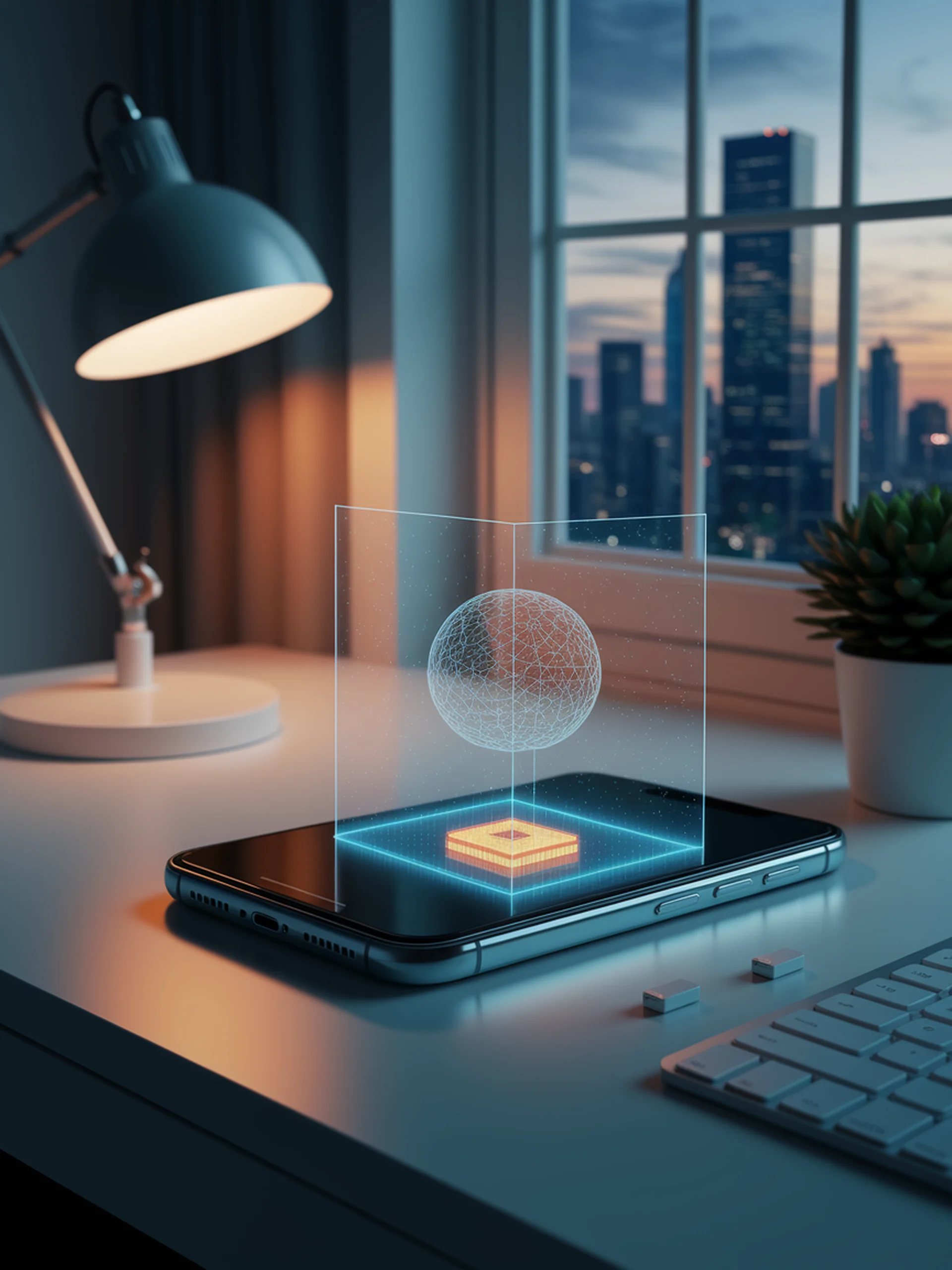
Shrinking Giants: LLM Compression Breakthrough
Leveraging Activation Sparsity for Edge Device Deployment
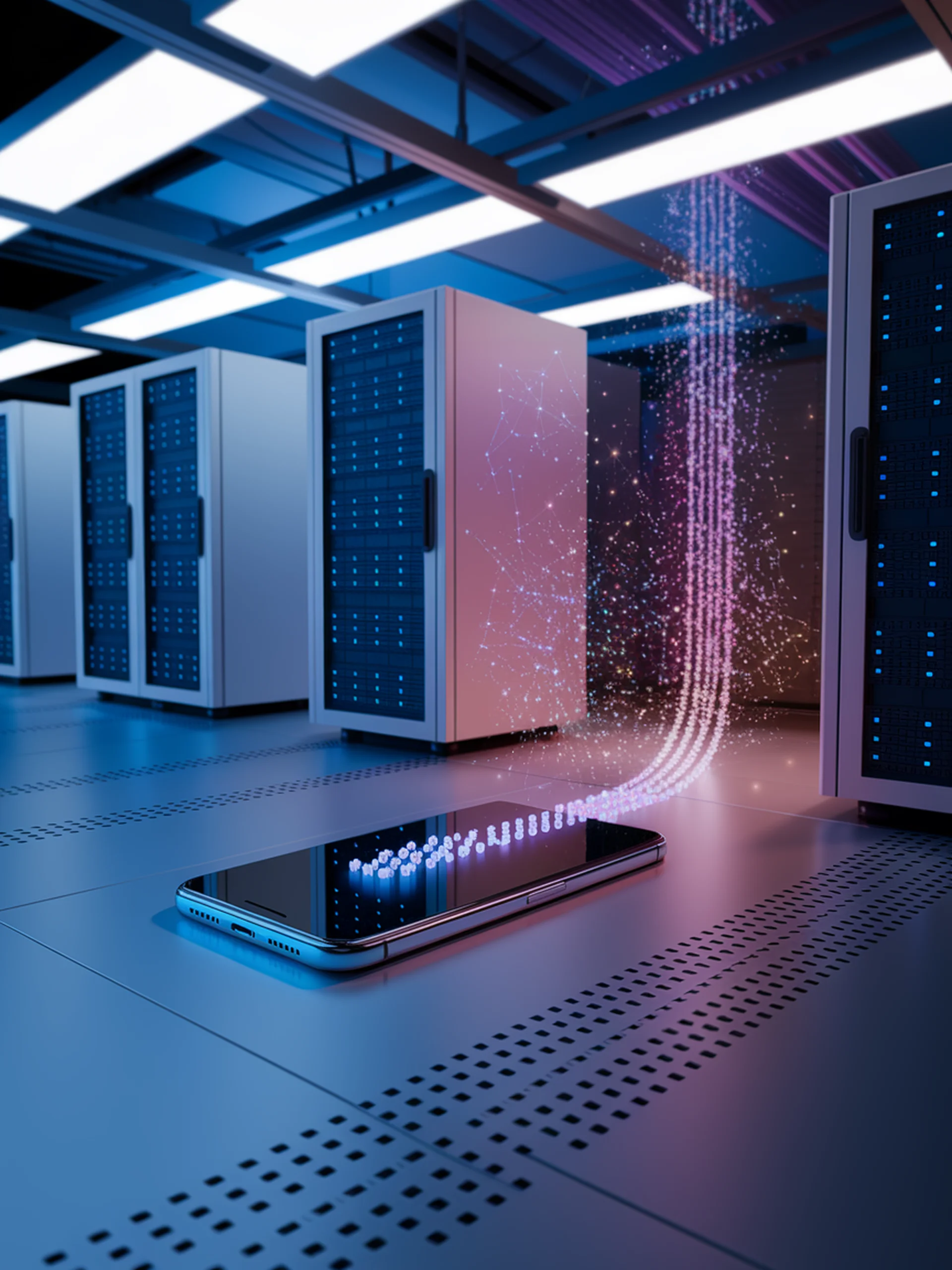
Smart Hybrid Language Models
Optimizing AI Inference Across Device and Cloud
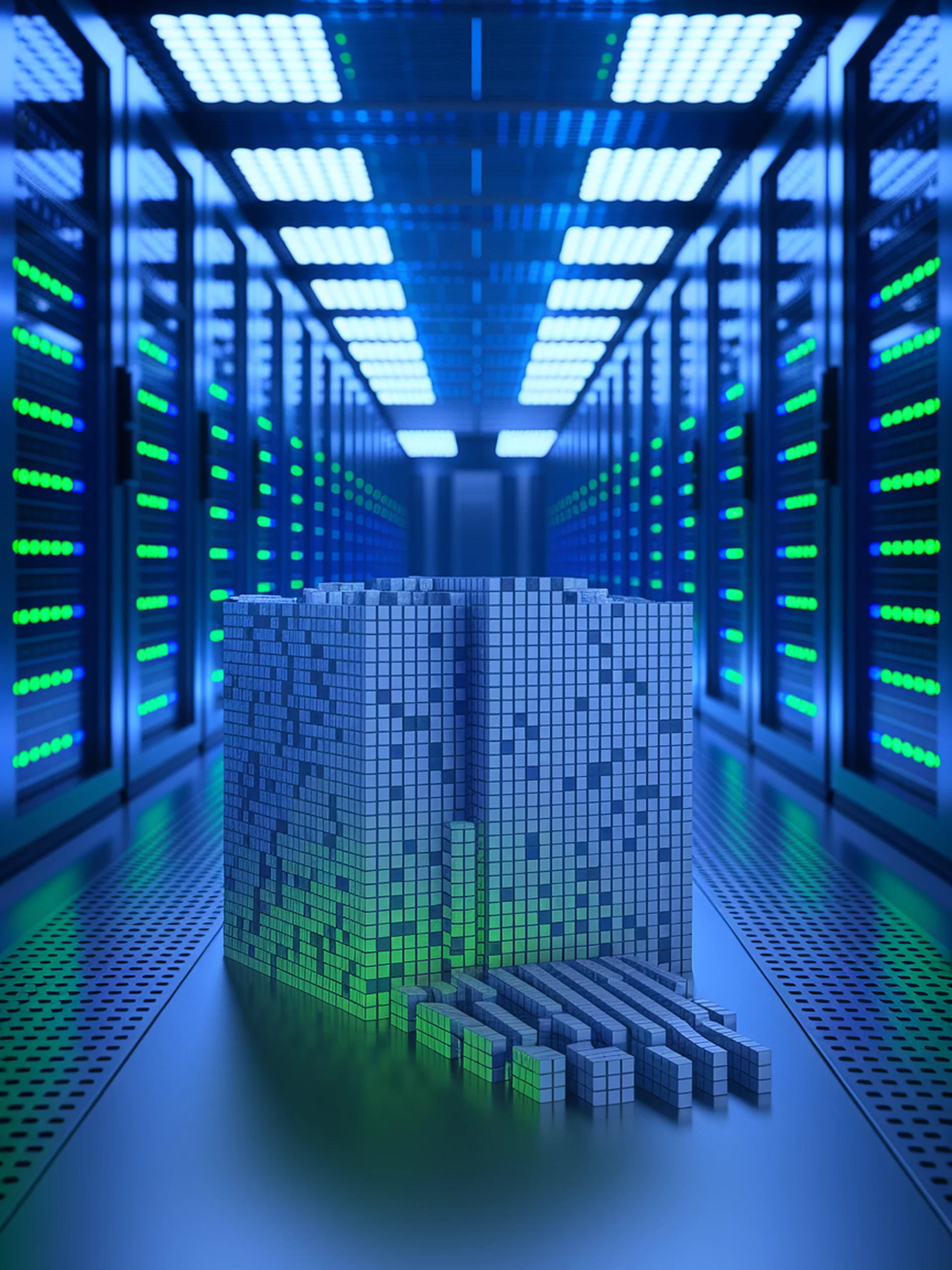
Optimizing KV Cache for Efficient LLMs
Finding the Sweet Spot Between Token Reduction and Precision
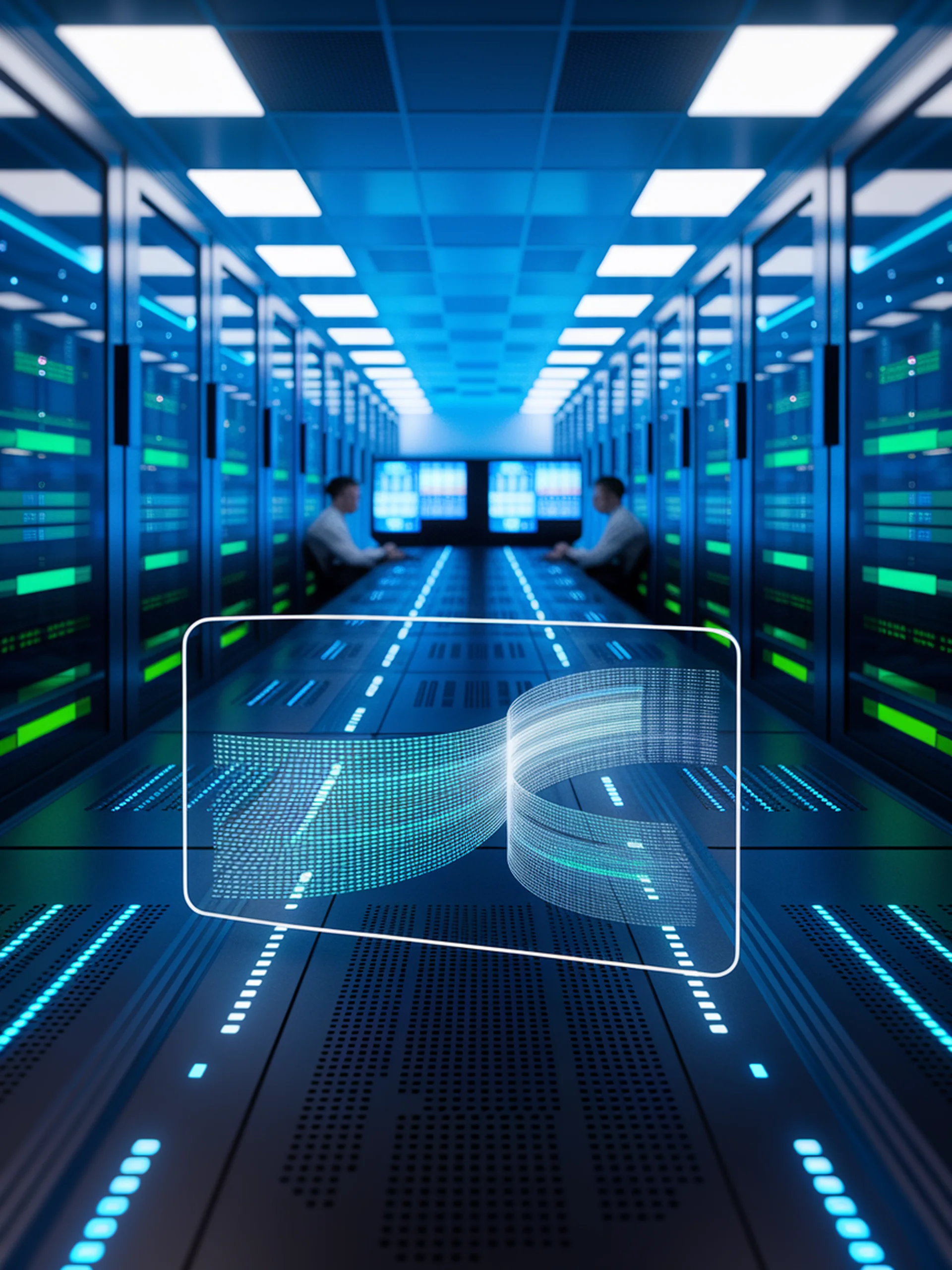
Memory-Efficient LLM Training
A stateless optimizer that reduces memory footprint while maintaining performance
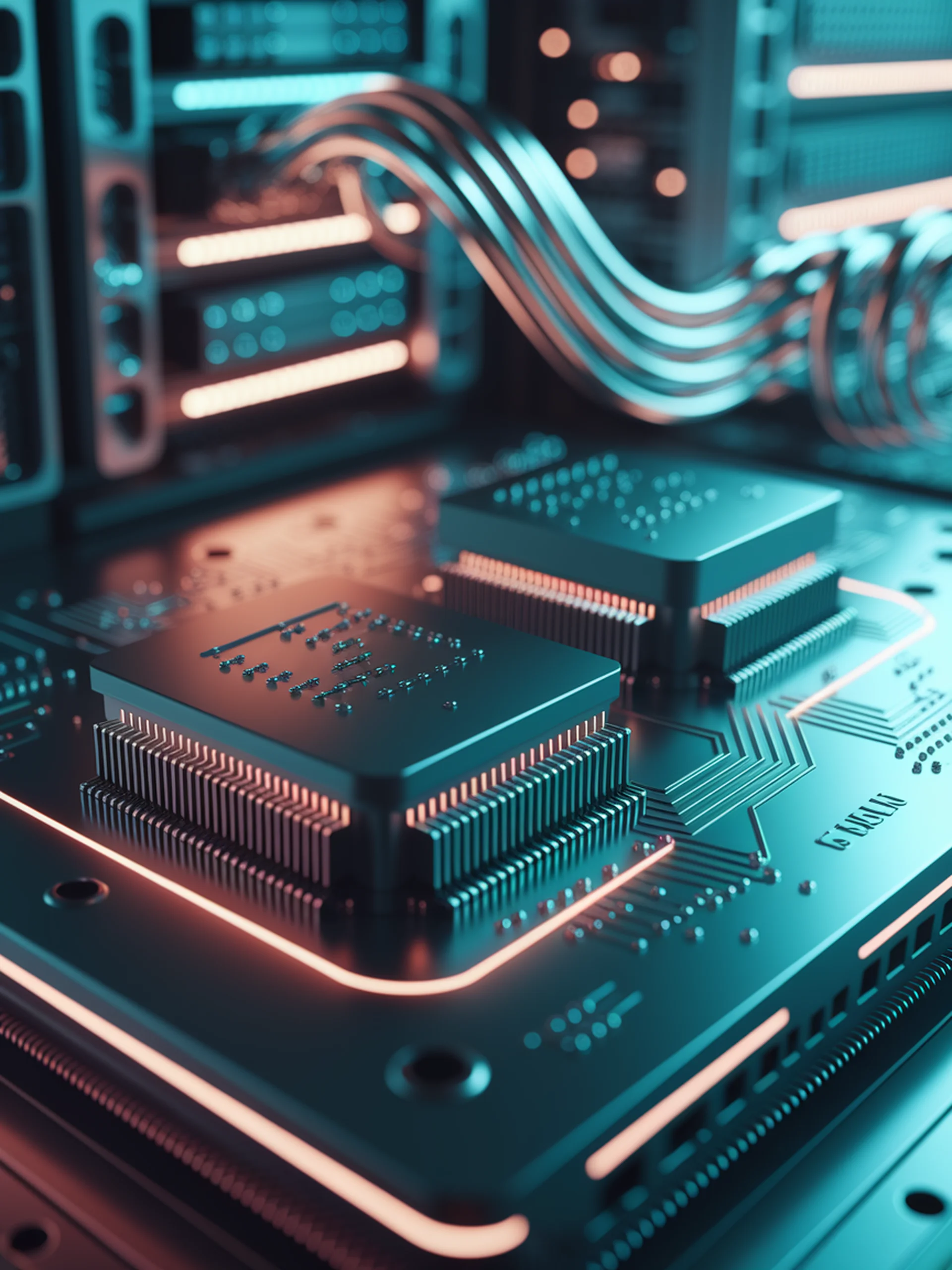
ResQ: Efficient LLM Quantization
Boosting 4-bit Quantization Performance with Low-Rank Residuals
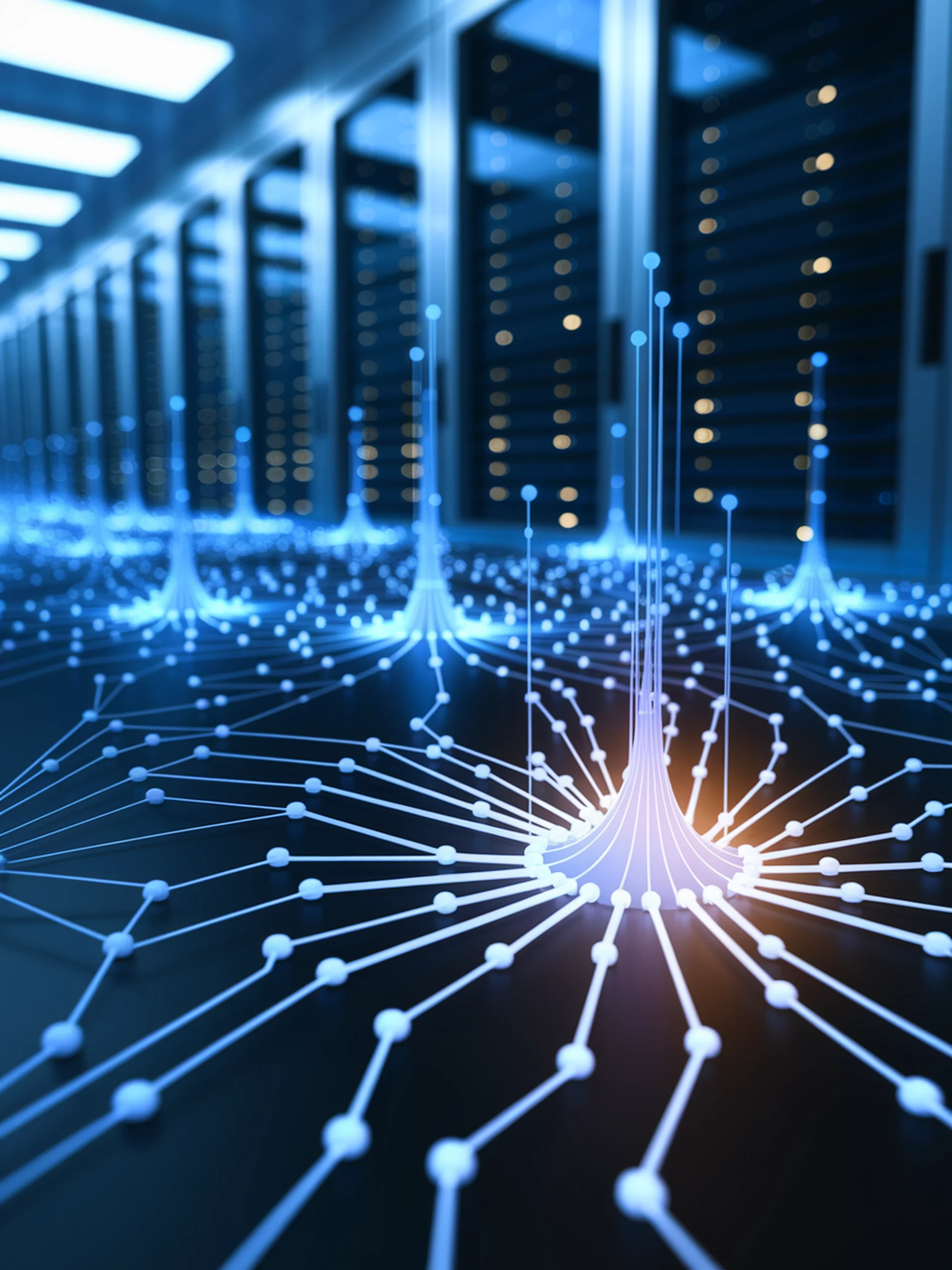
Self-Evolving LLMs
How Language Models Can Engineer Their Own Training Data
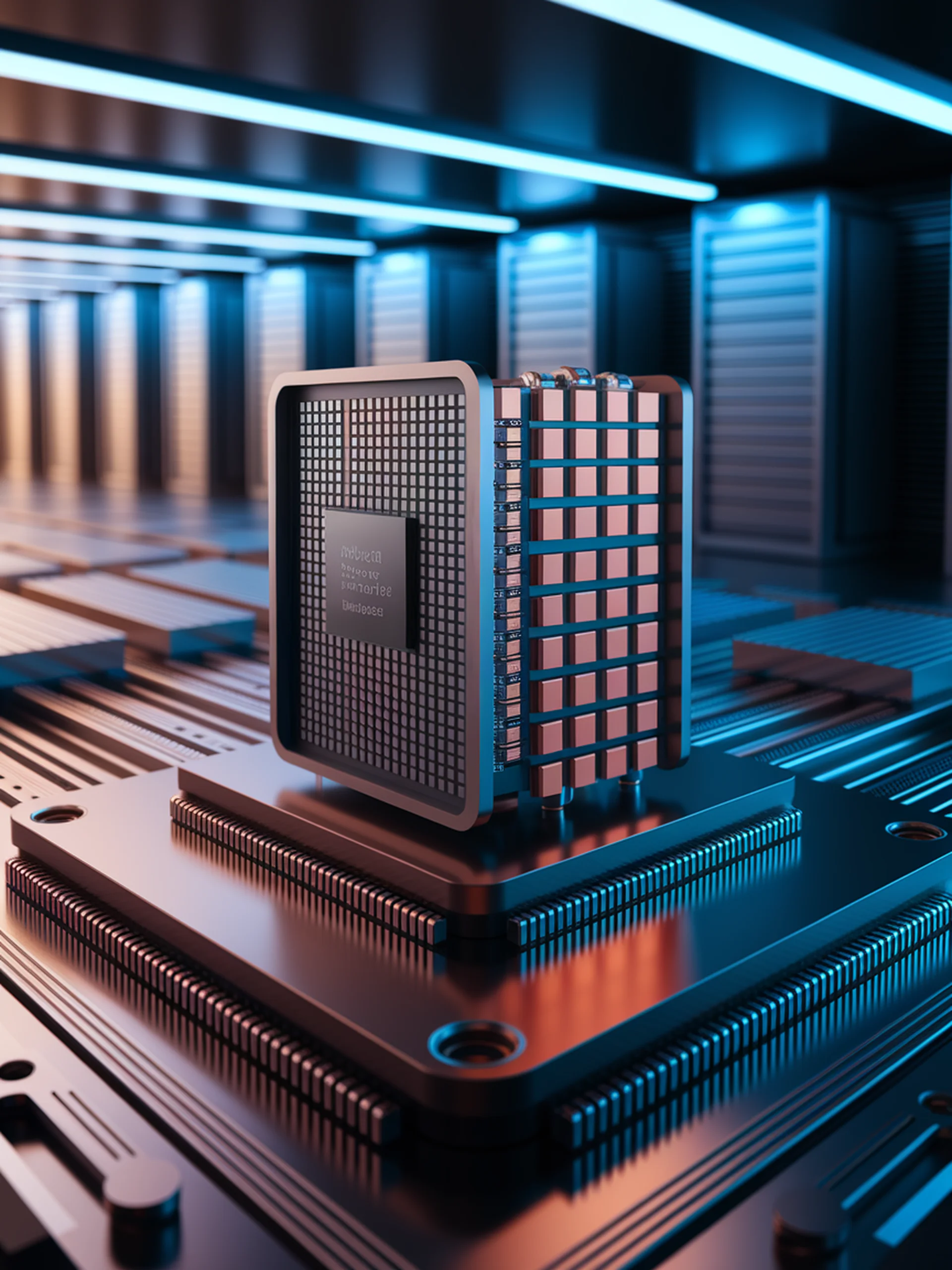
Accelerating LLMs with GQSA
Combining Quantization and Sparsity for Efficient Language Models
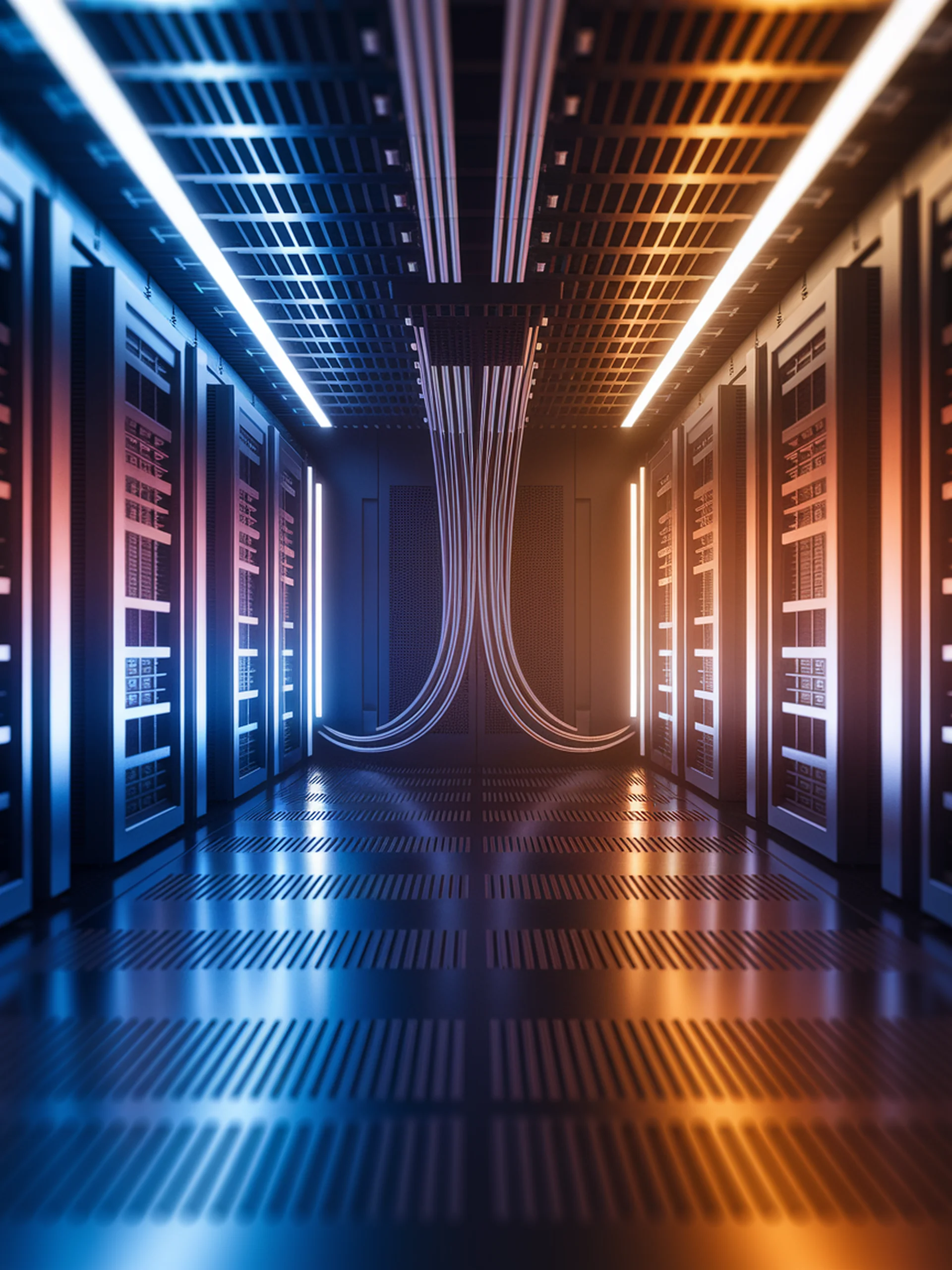
Balancing Efficiency in Vision-Language Models
A smarter approach to model quantization across modalities
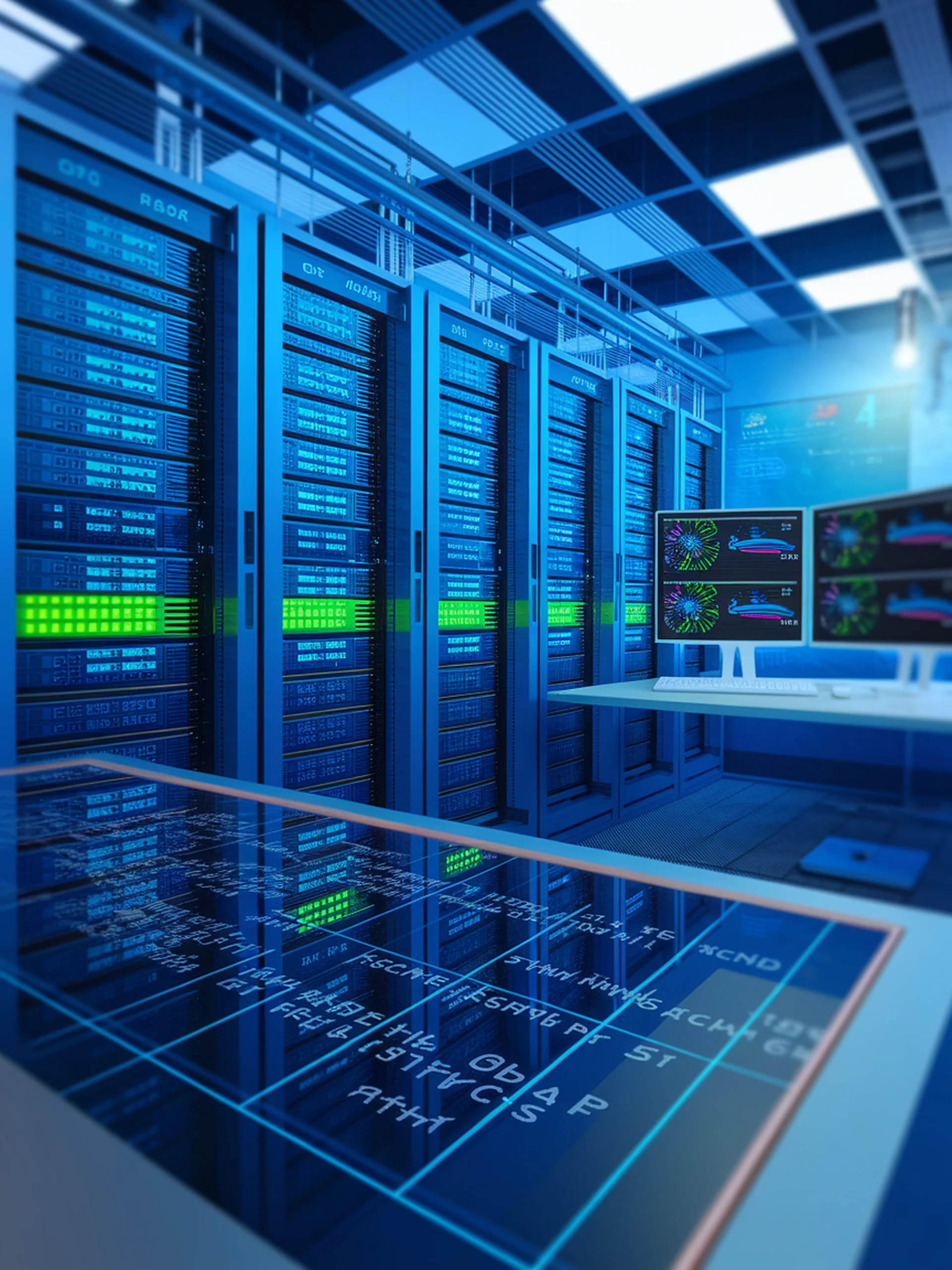
Optimizing LLM Training Efficiency
Adaptive Batch Size Scheduling for Better Performance and Efficiency
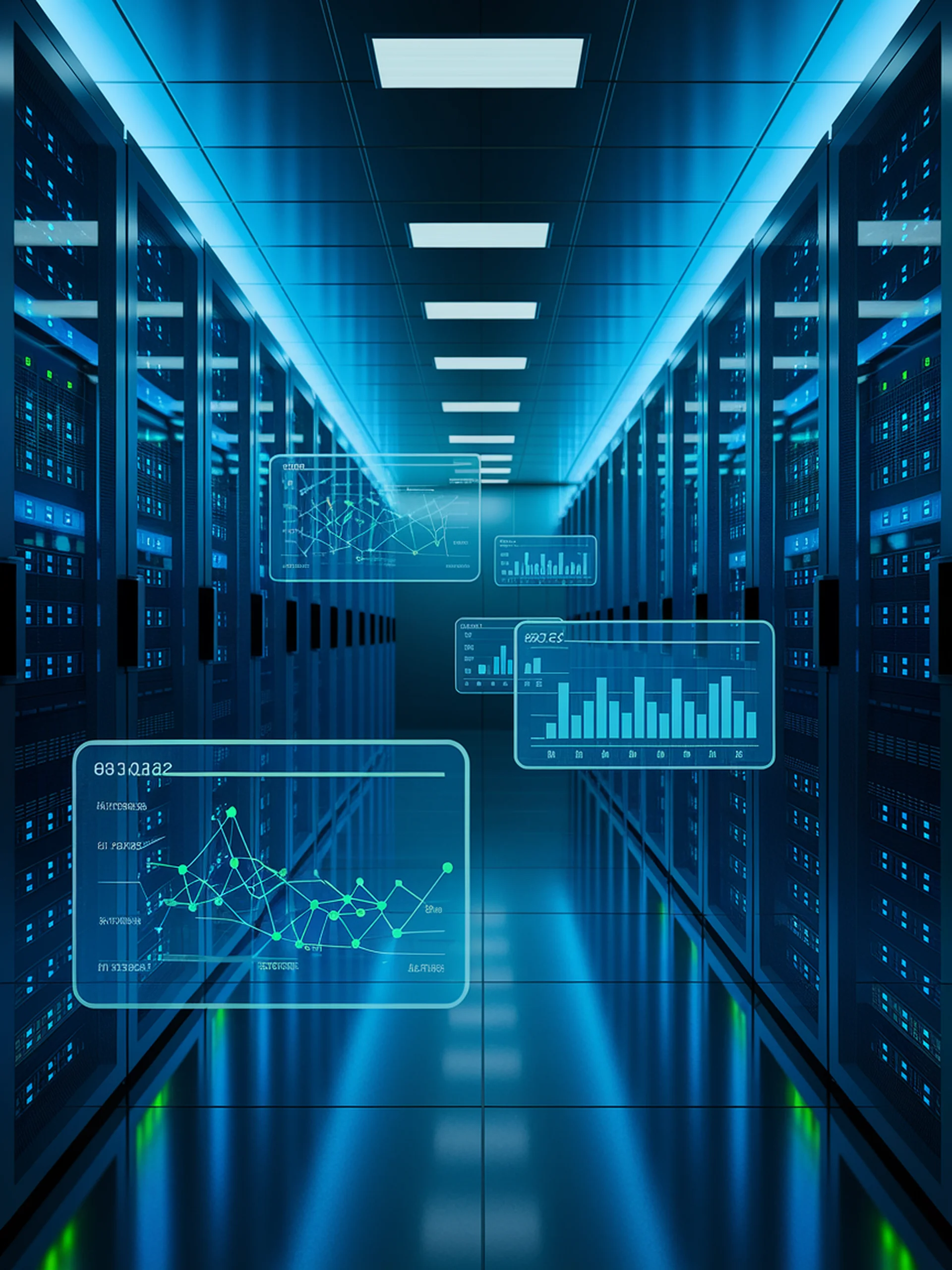
Supercharging Data Deduplication for LLMs
GPU-Accelerated Framework for Faster, More Efficient Dataset Processing
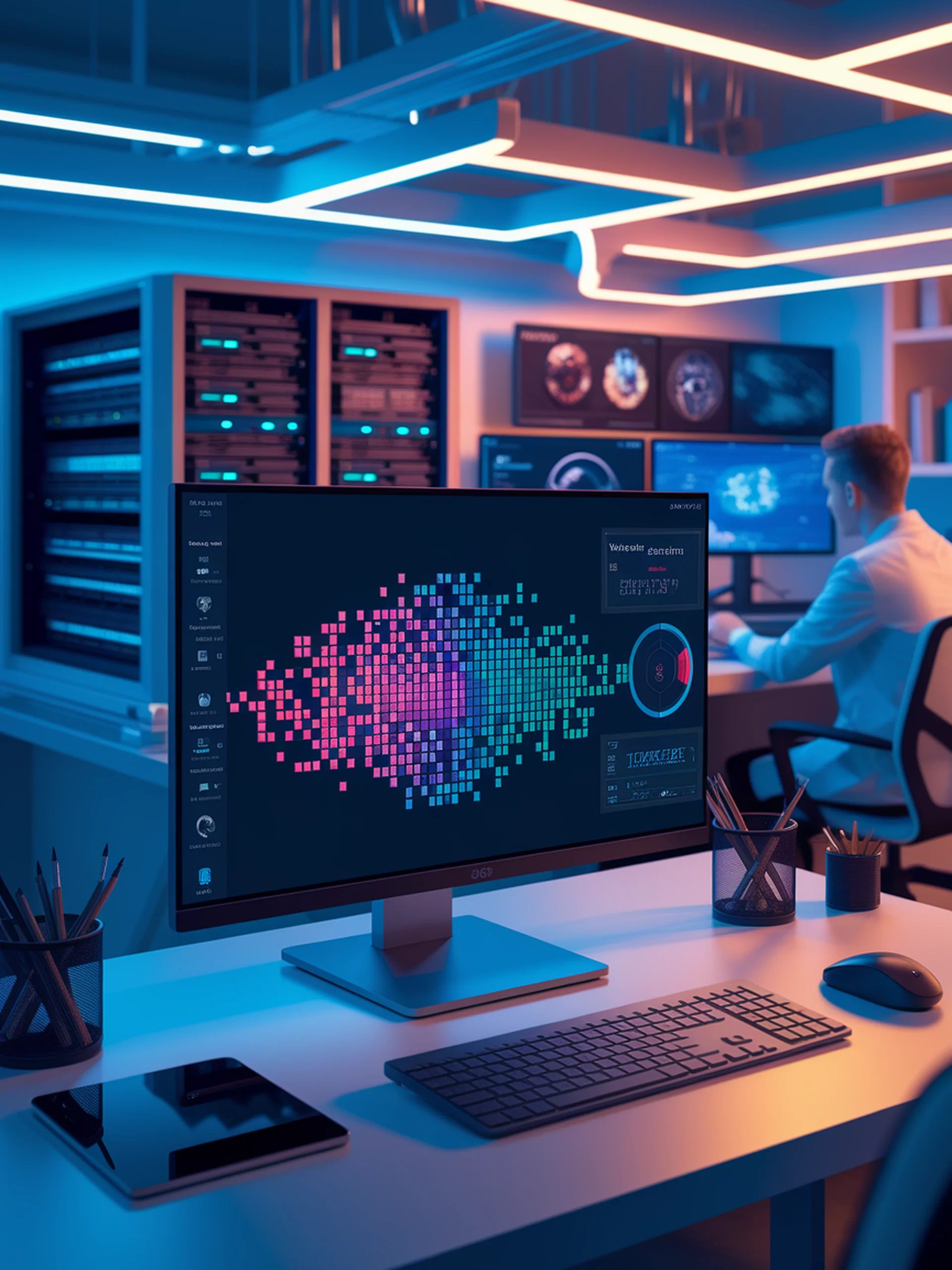
FOLDER: Accelerating Multi-modal LLMs
A plug-and-play solution for faster, more efficient visual processing
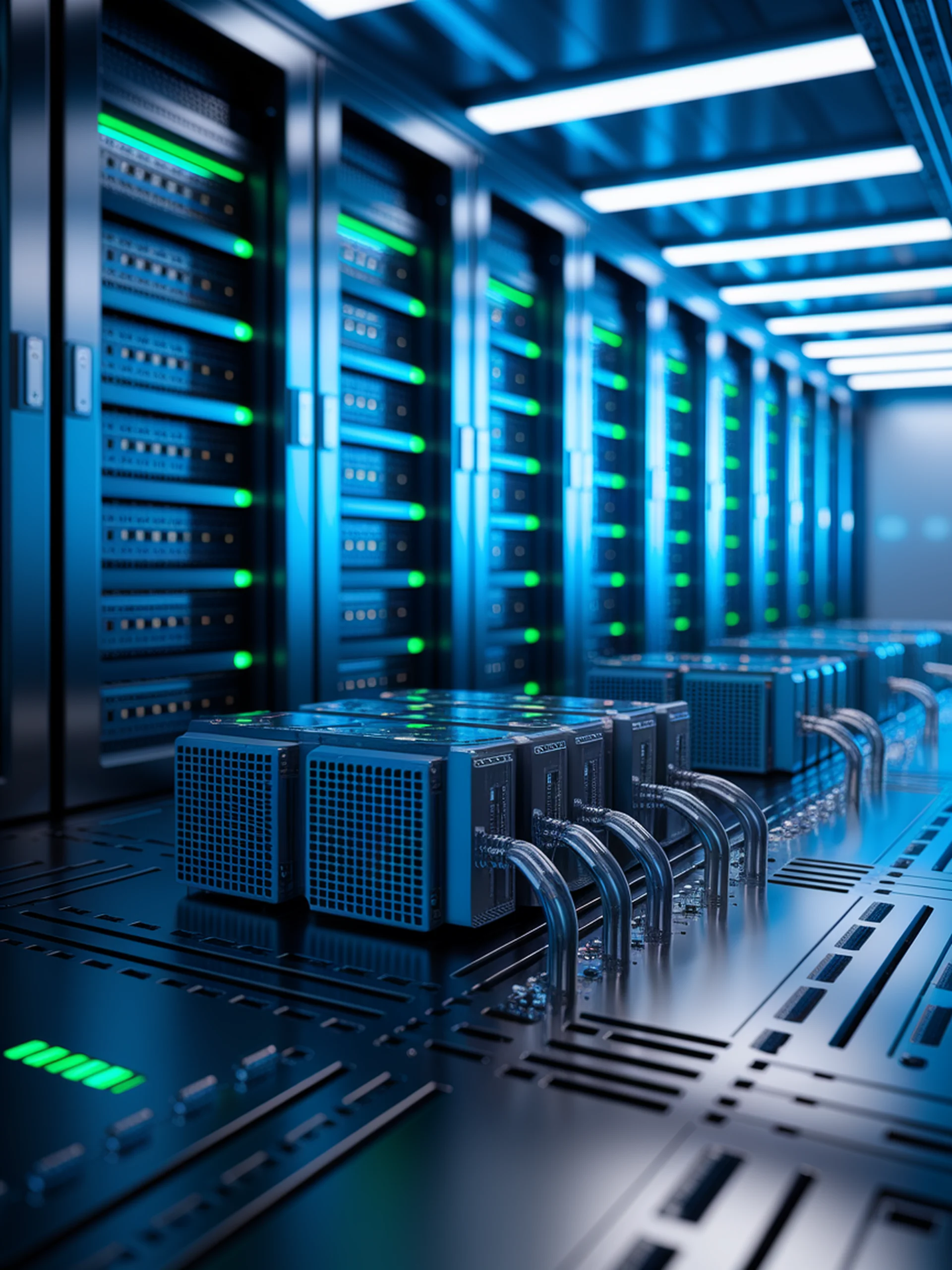
HALO: Efficient Low-Precision LLM Training
Enabling accurate quantized training for large language models
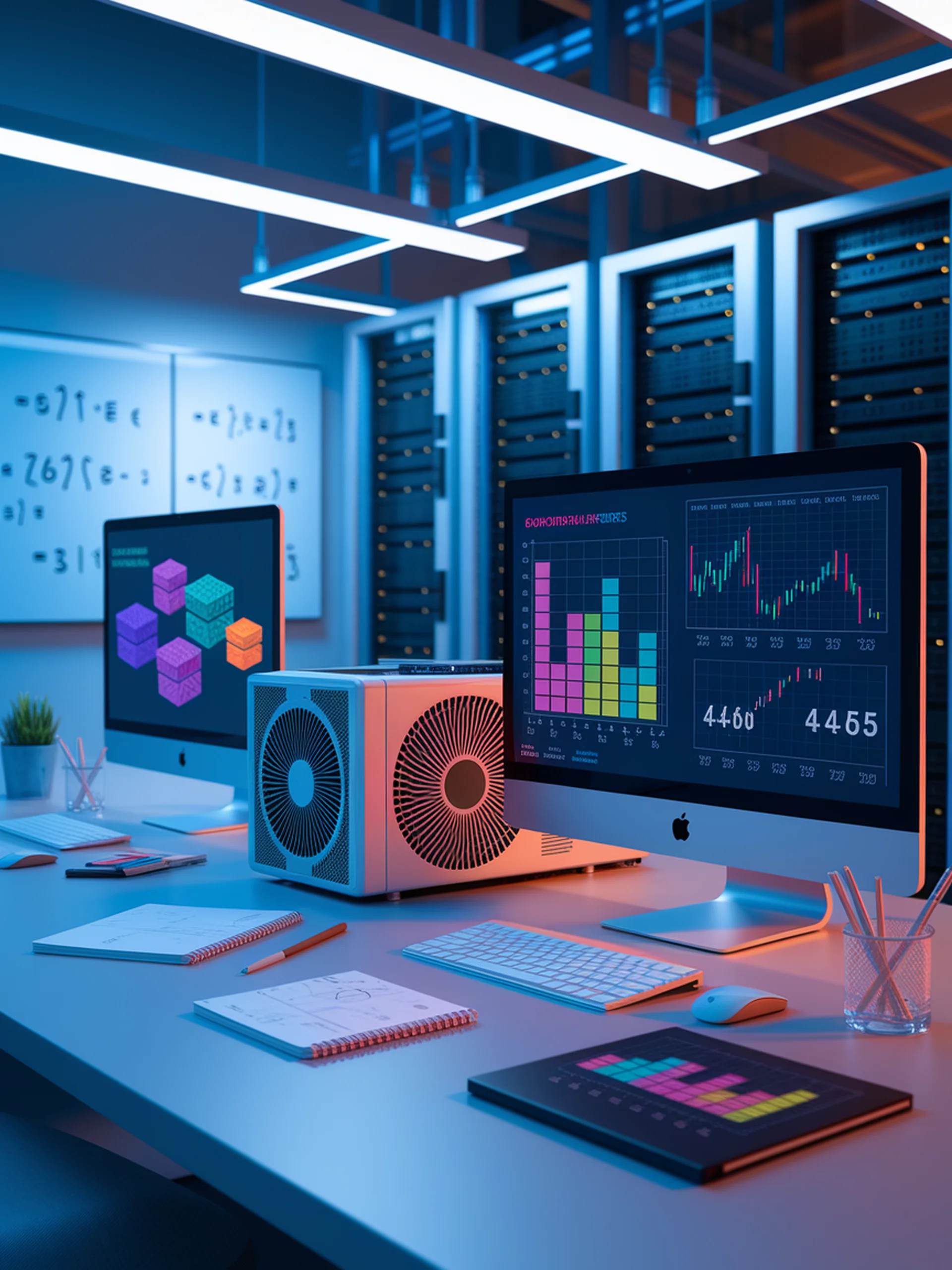
Optimizing LLMs for Mathematical Reasoning
Examining how quantization affects reasoning capabilities
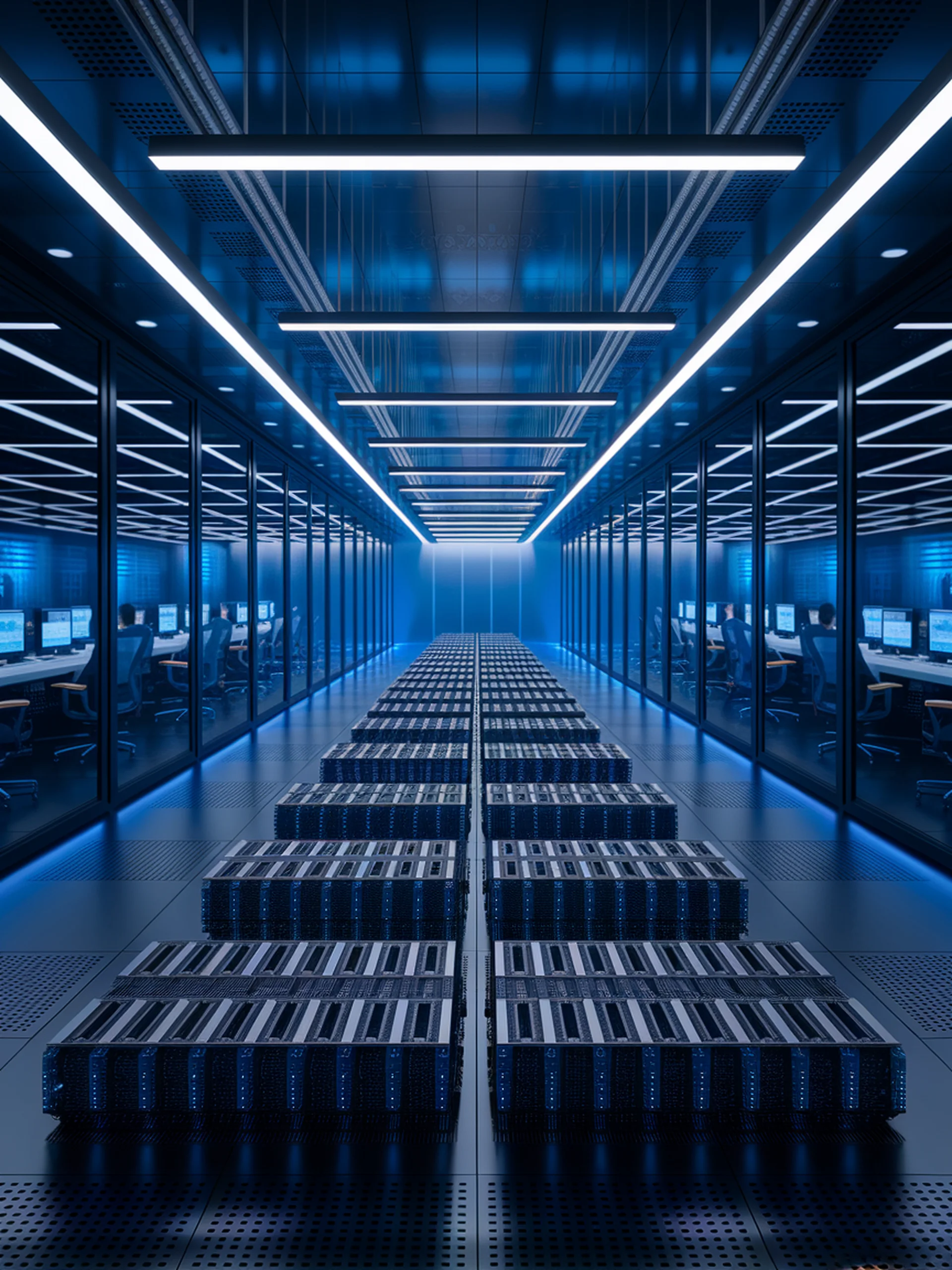
Scaling Large Language Model Training on Frontier with Low-B...
By Lang Xu, Quentin Anthony...
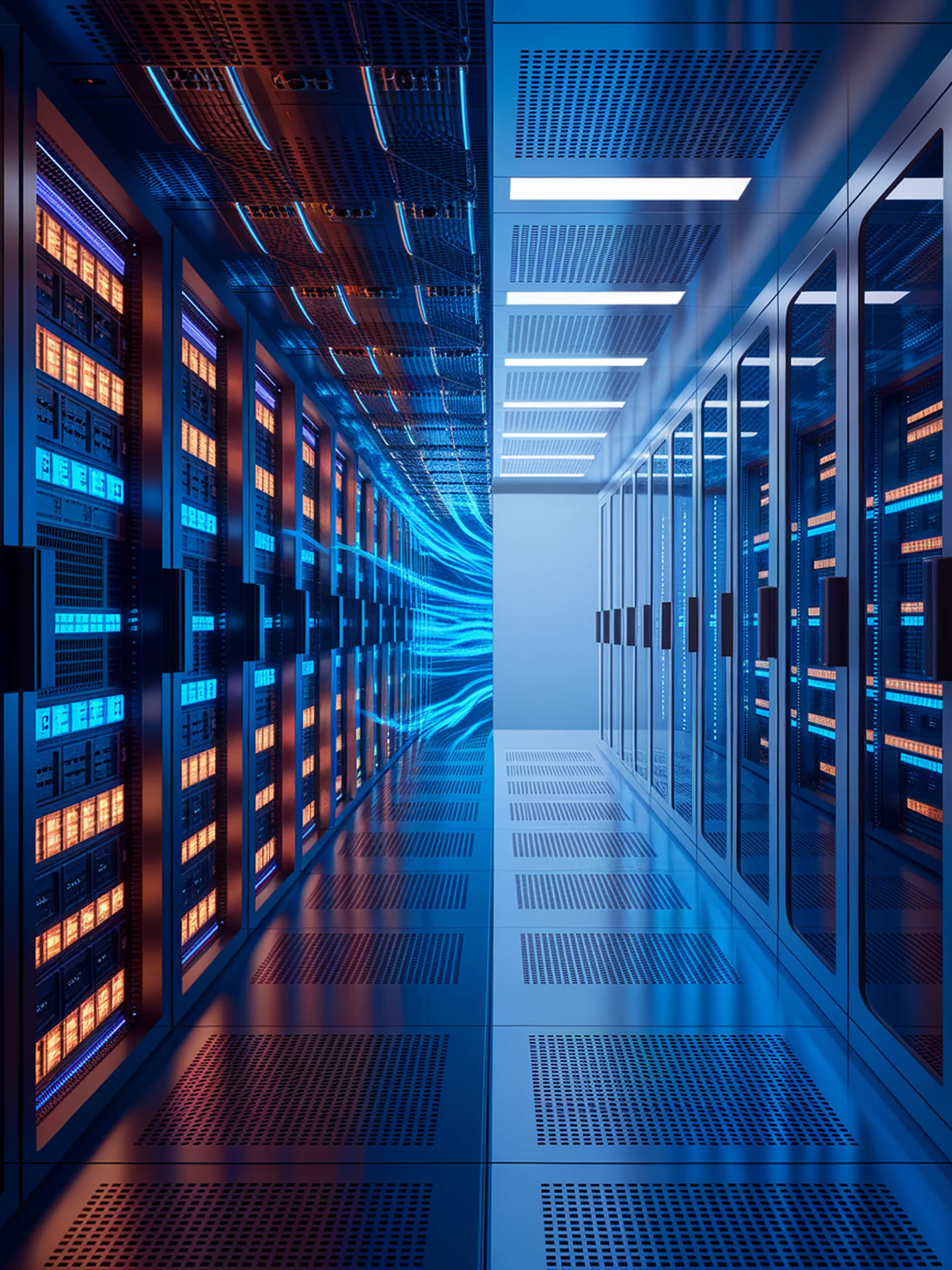
Boosting Multimodal AI Performance
EPD Disaggregation: A Framework for Faster, More Efficient LMM Serving
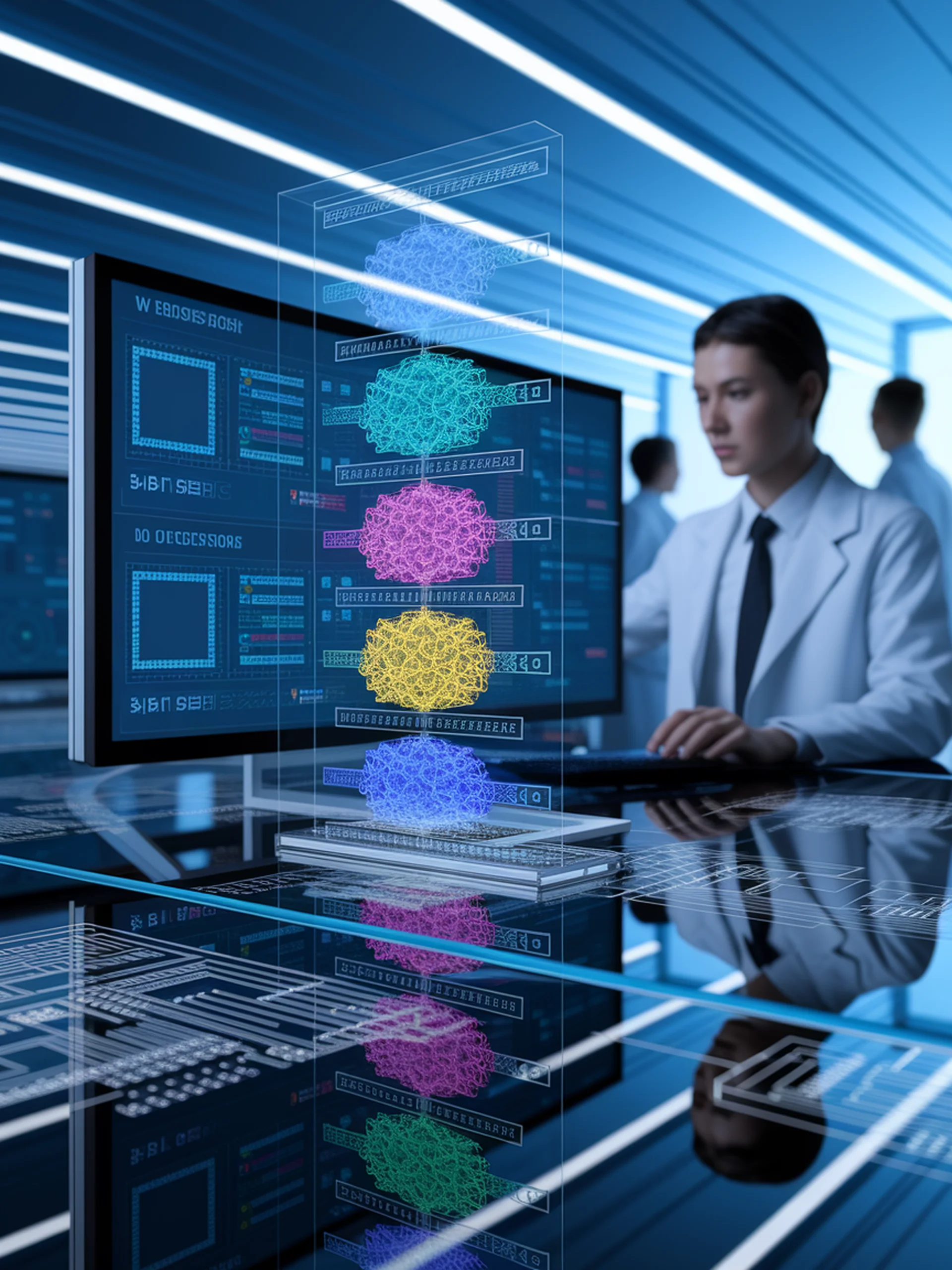
Self-Improving Multimodal LLMs
Enhancing reasoning capabilities through cascaded self-evaluation
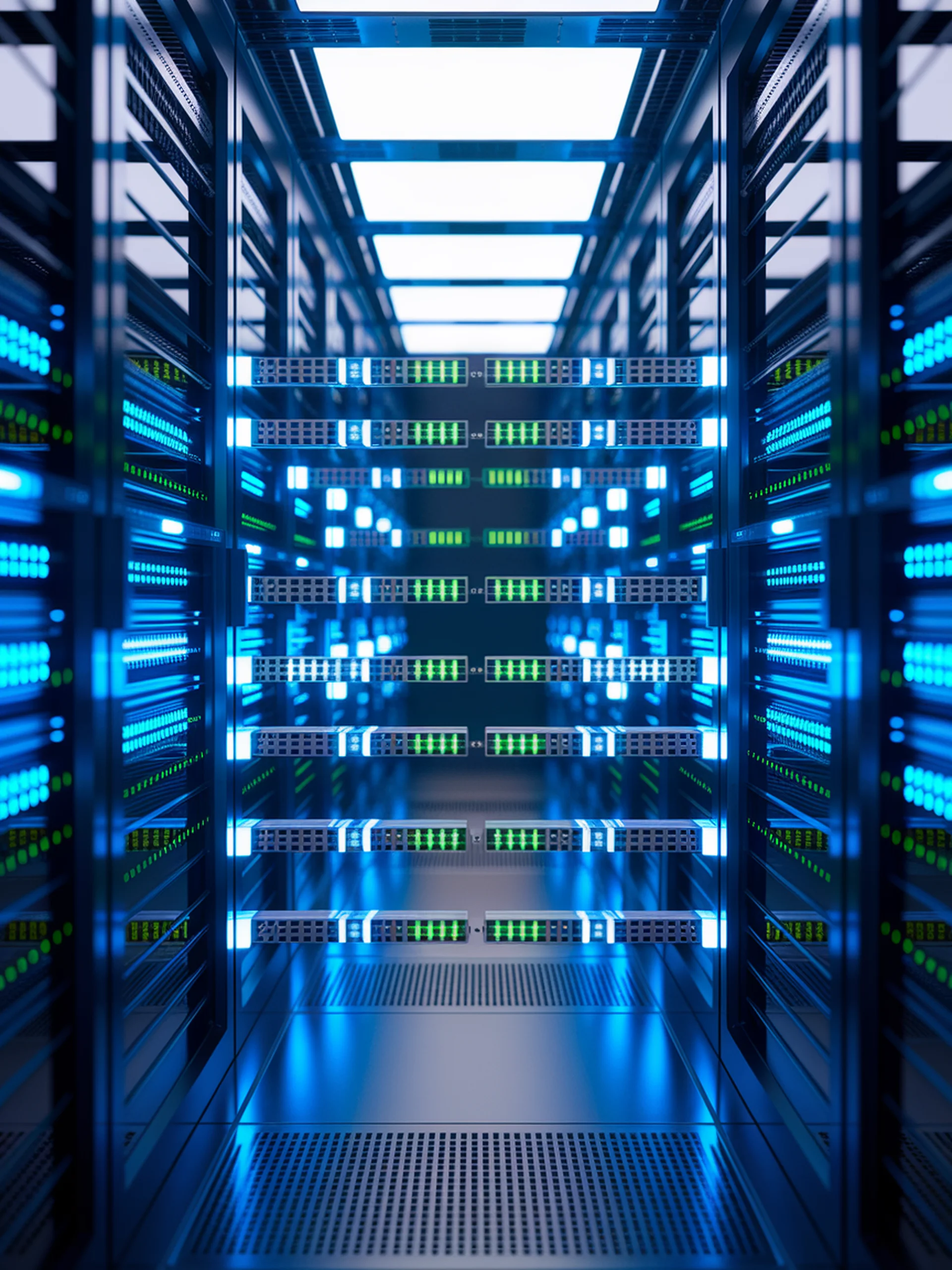
Accelerating LLM Inference With Parallel Processing
Overcoming GPU communication bottlenecks with ladder-residual architecture
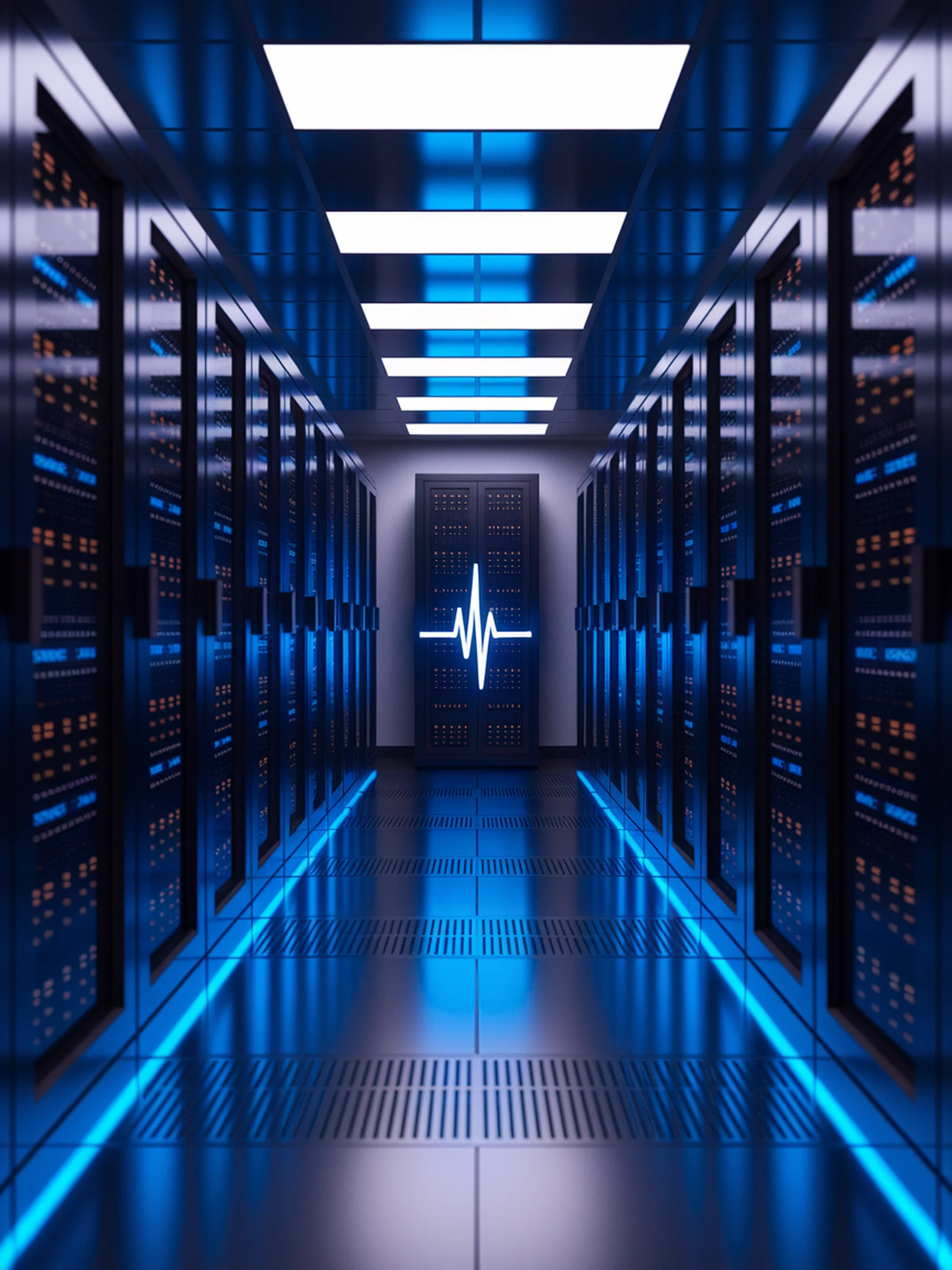
Taming the Spikes in LLM Training
A novel Adam optimizer that dramatically improves training stability
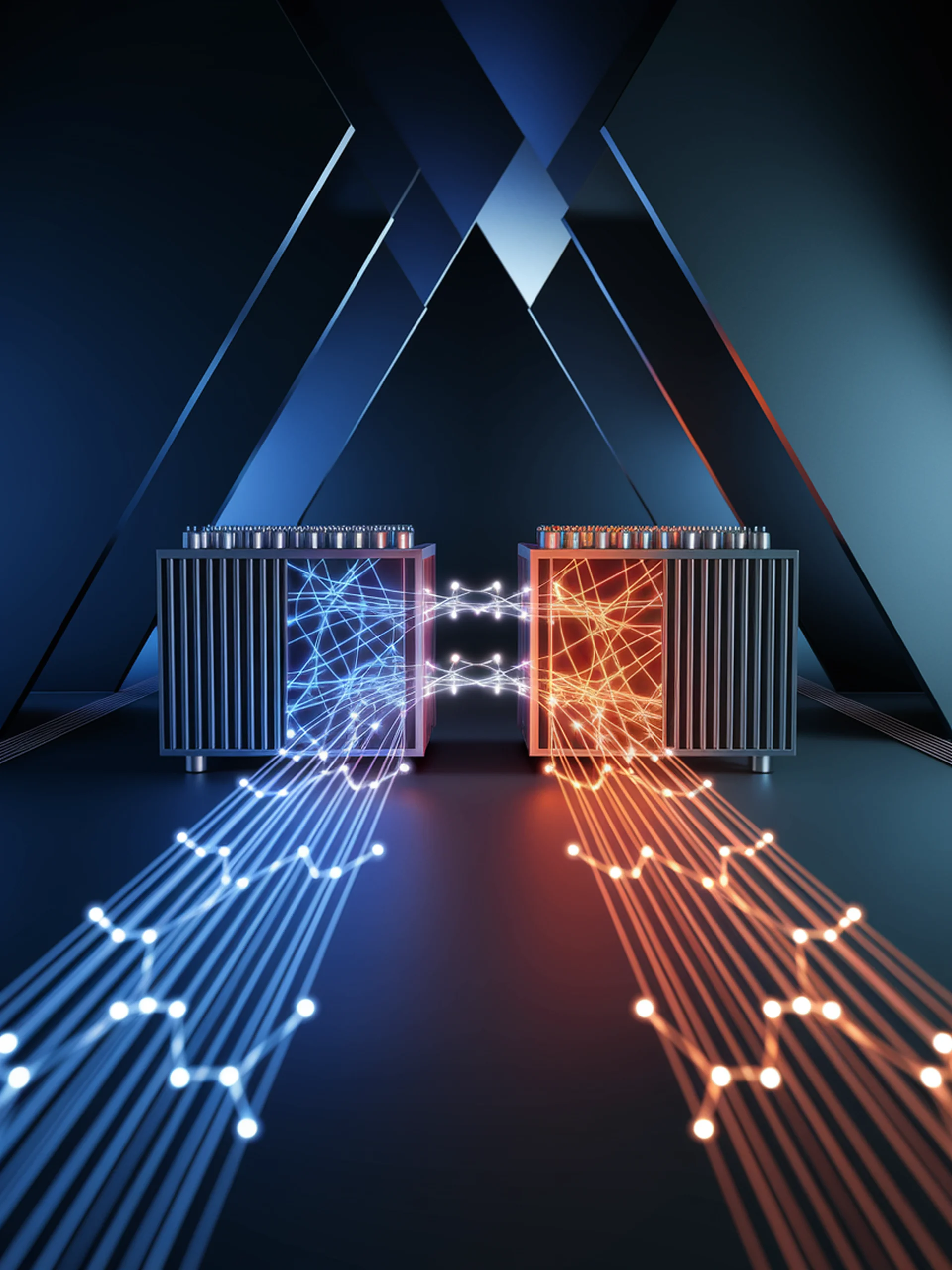
Solving Catastrophic Forgetting in LLMs
A novel architecture for preserving knowledge during model evolution
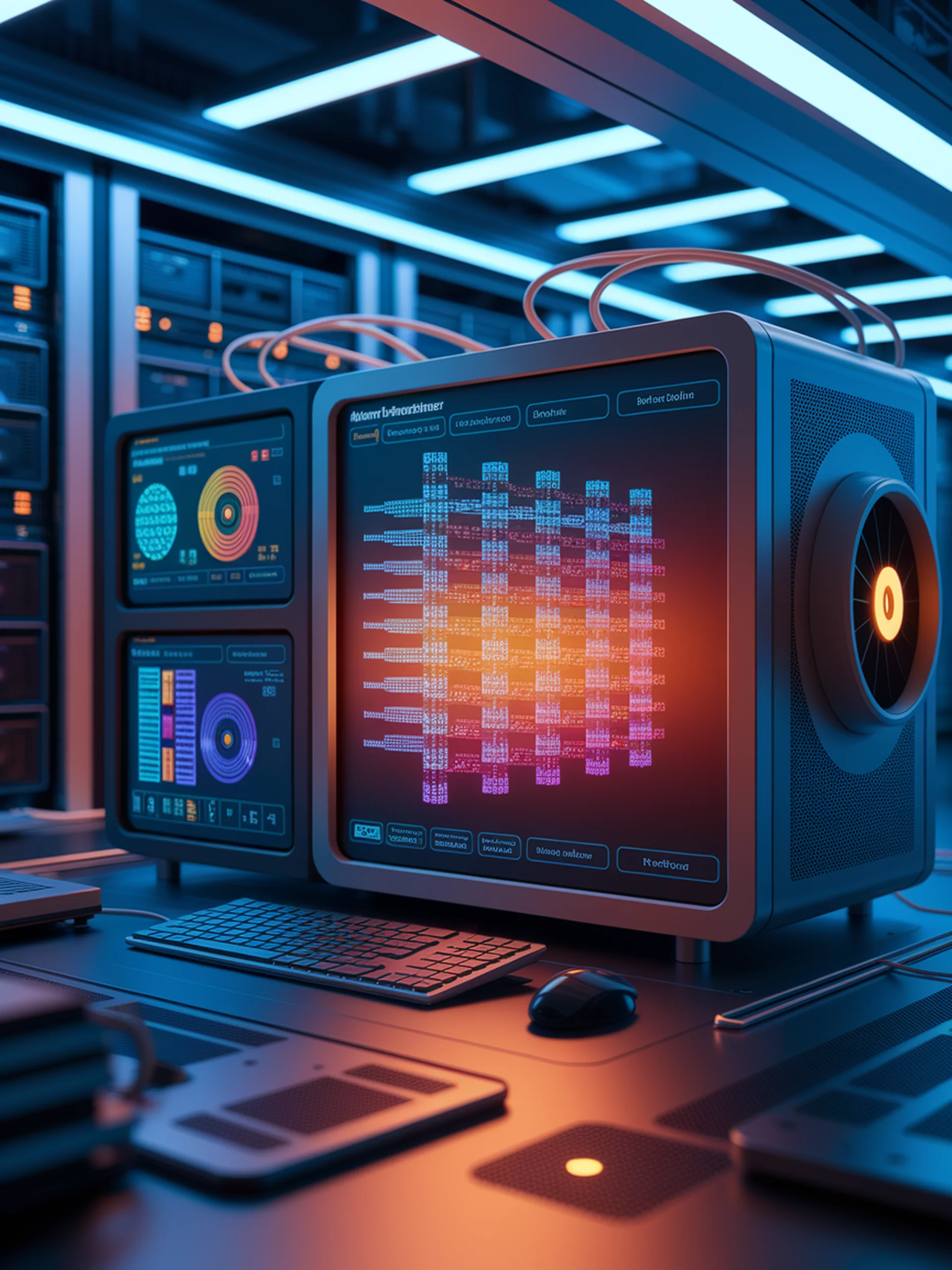
Breaking Size Barriers in AI Parameter Generation
Generating hundreds of millions of parameters on a single GPU
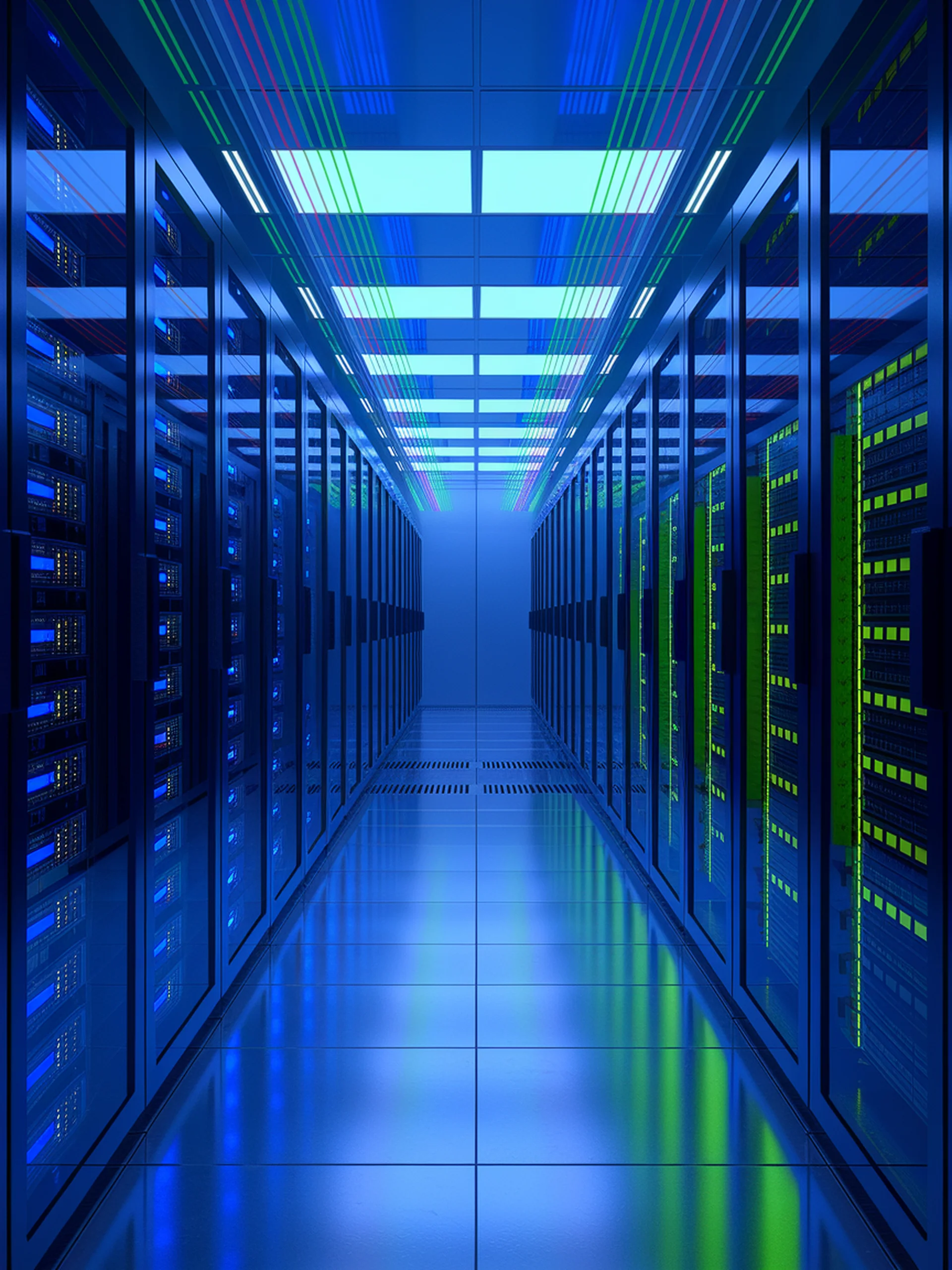
Optimizing LLM Performance with Glinthawk
A two-tiered approach to enhance offline LLM inference efficiency
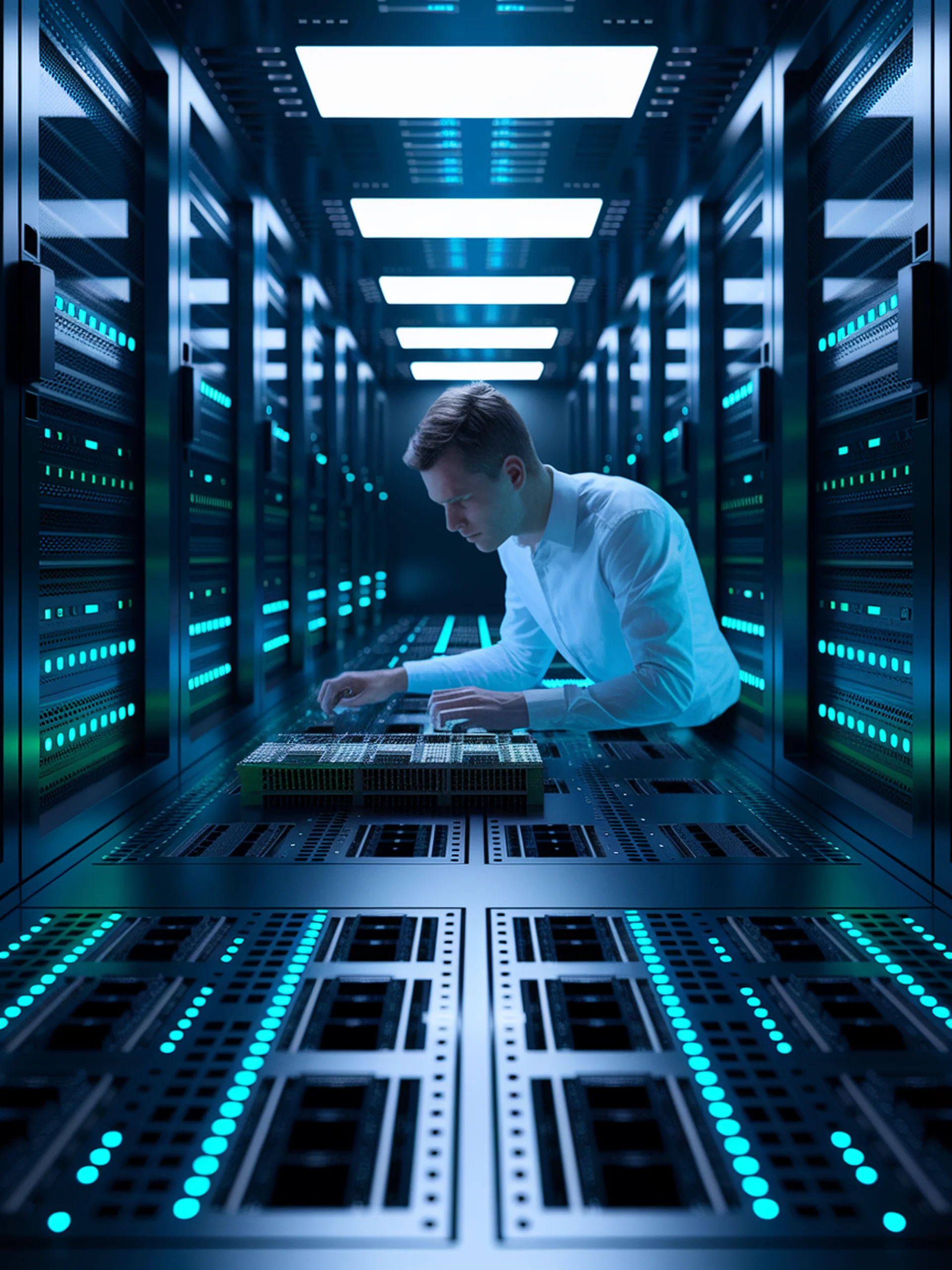
GPU-Adaptive Quantization for LLMs
Enhancing efficiency without sacrificing performance
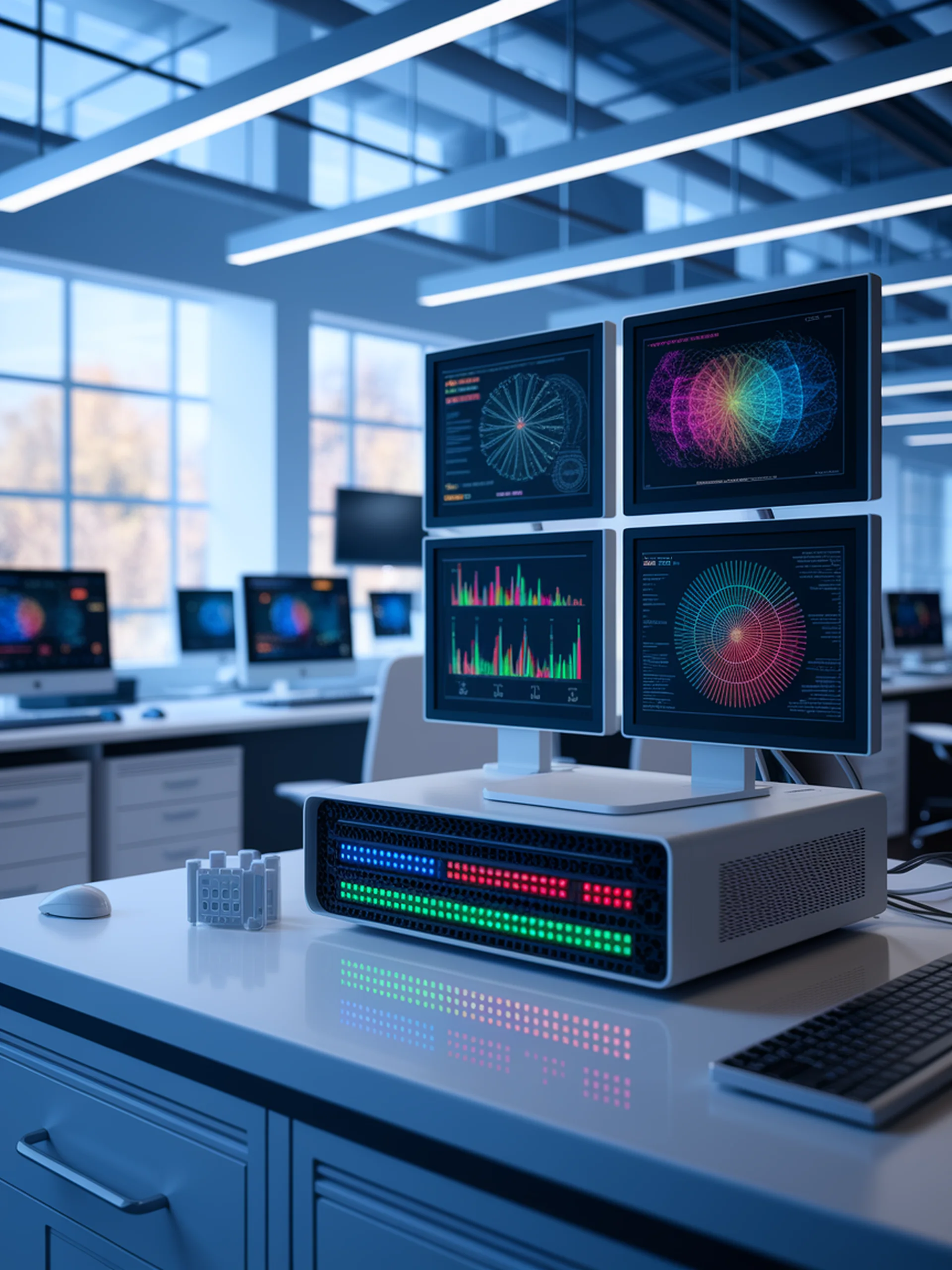
Optimizing LLMs for Long-Context Applications
A Training-Free Approach to Efficient Prompt Compression
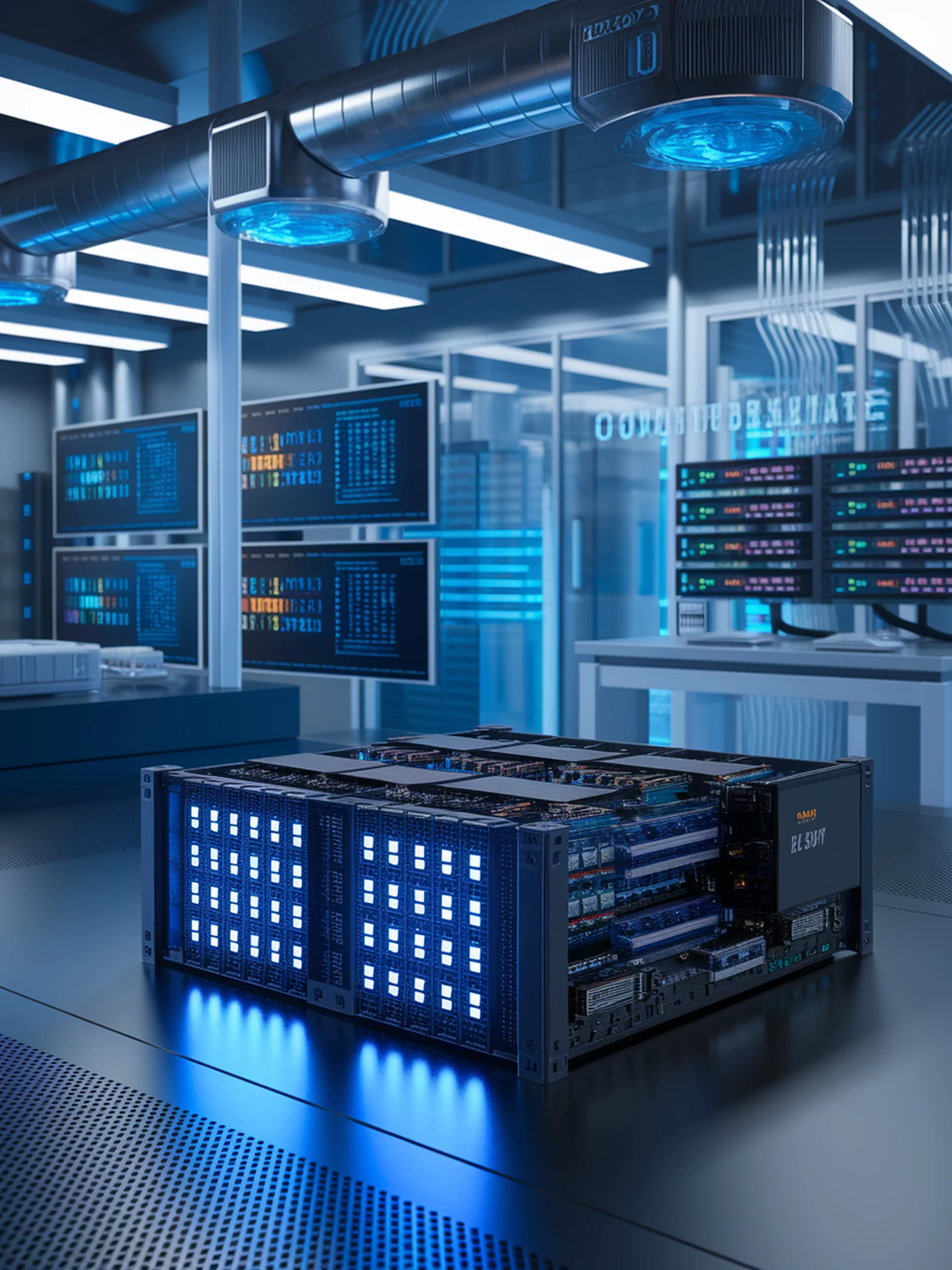
QRazor: Cutting-Edge 4-bit LLM Optimization
Efficient quantization without accuracy loss
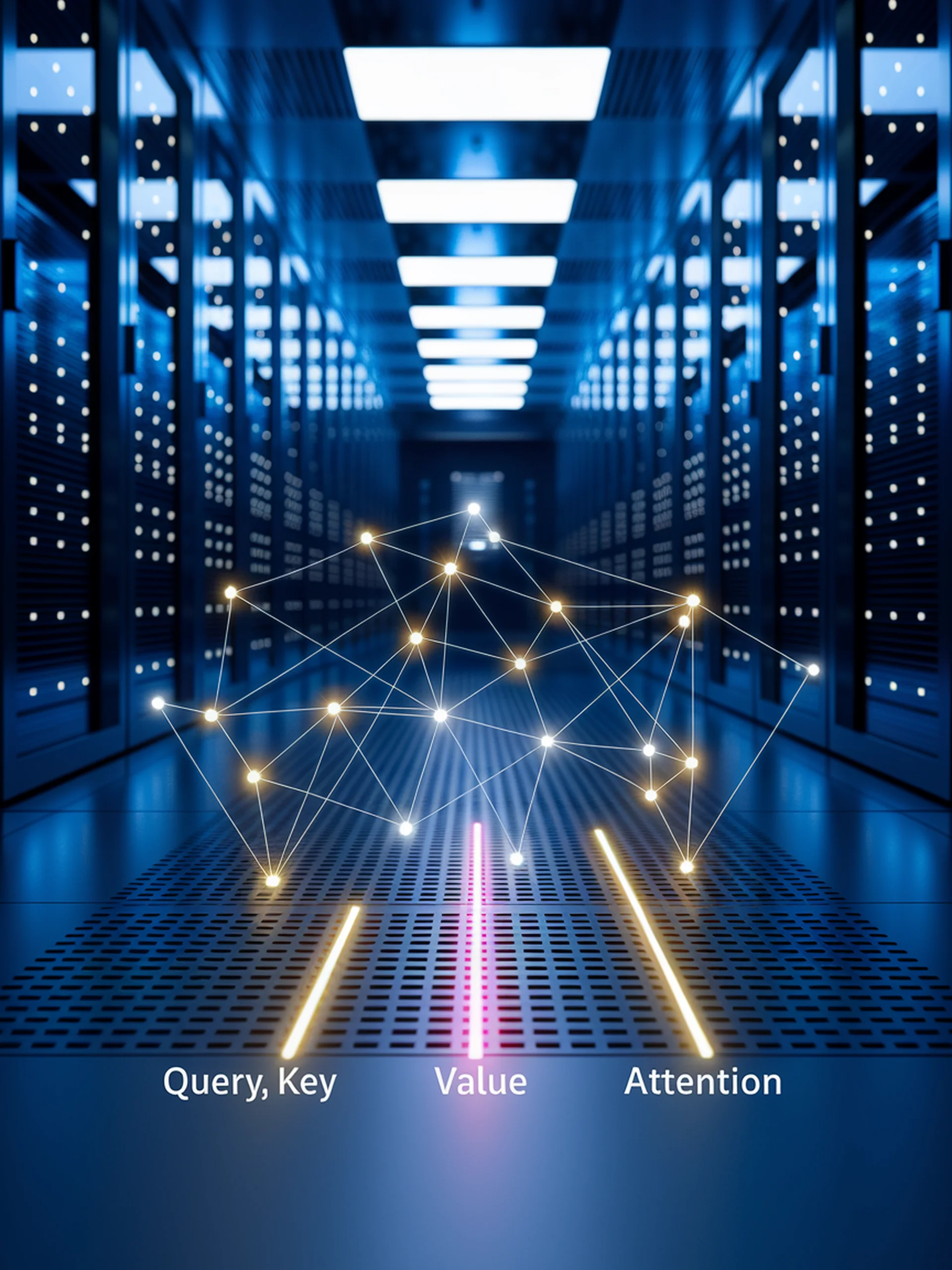
Sigma: Boosting LLM Efficiency
Novel DiffQKV attention mechanism enhances performance while reducing computational costs
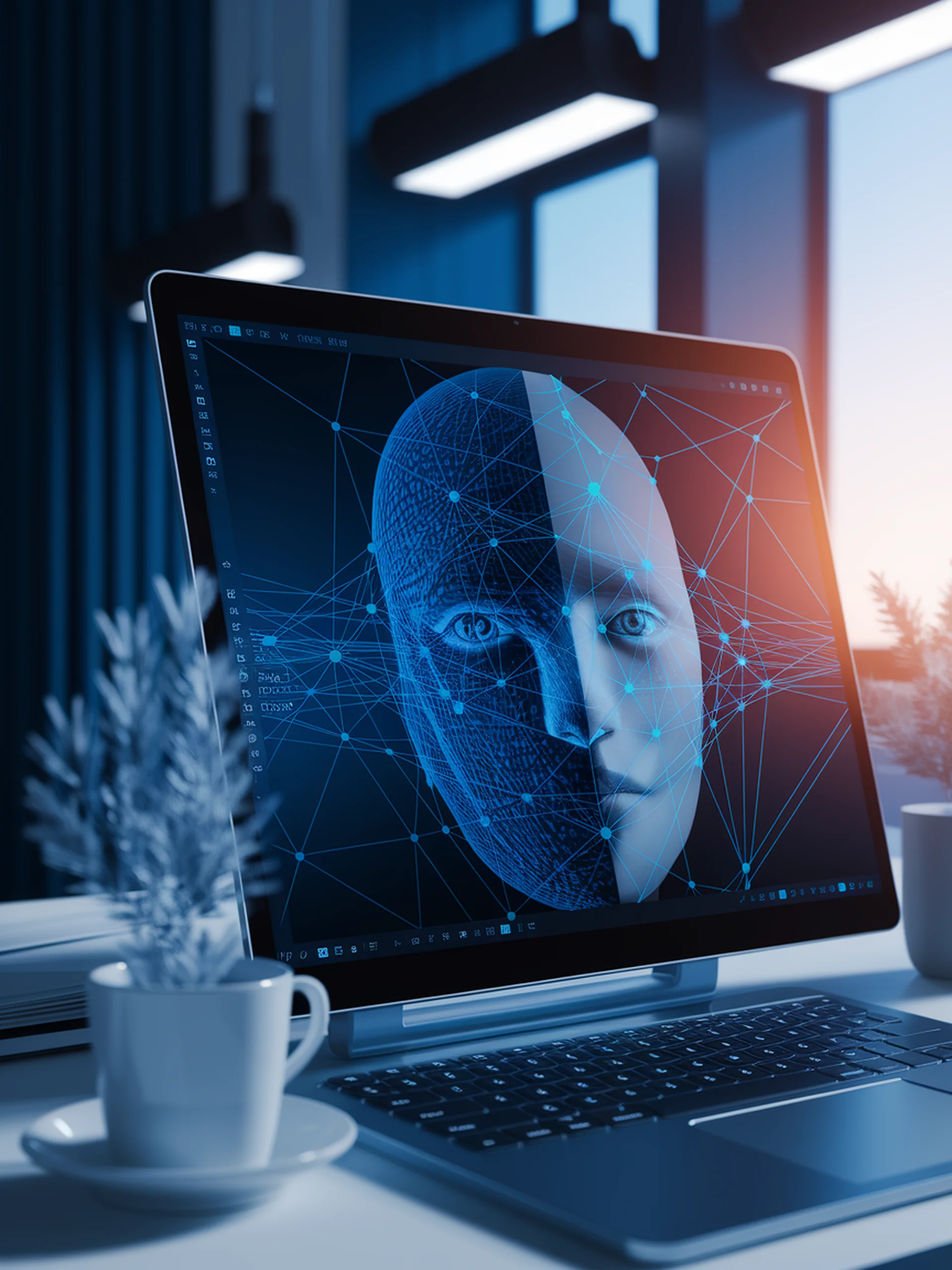
Smart Vision Pruning for Efficient MLLMs
Boosting performance while reducing computational costs
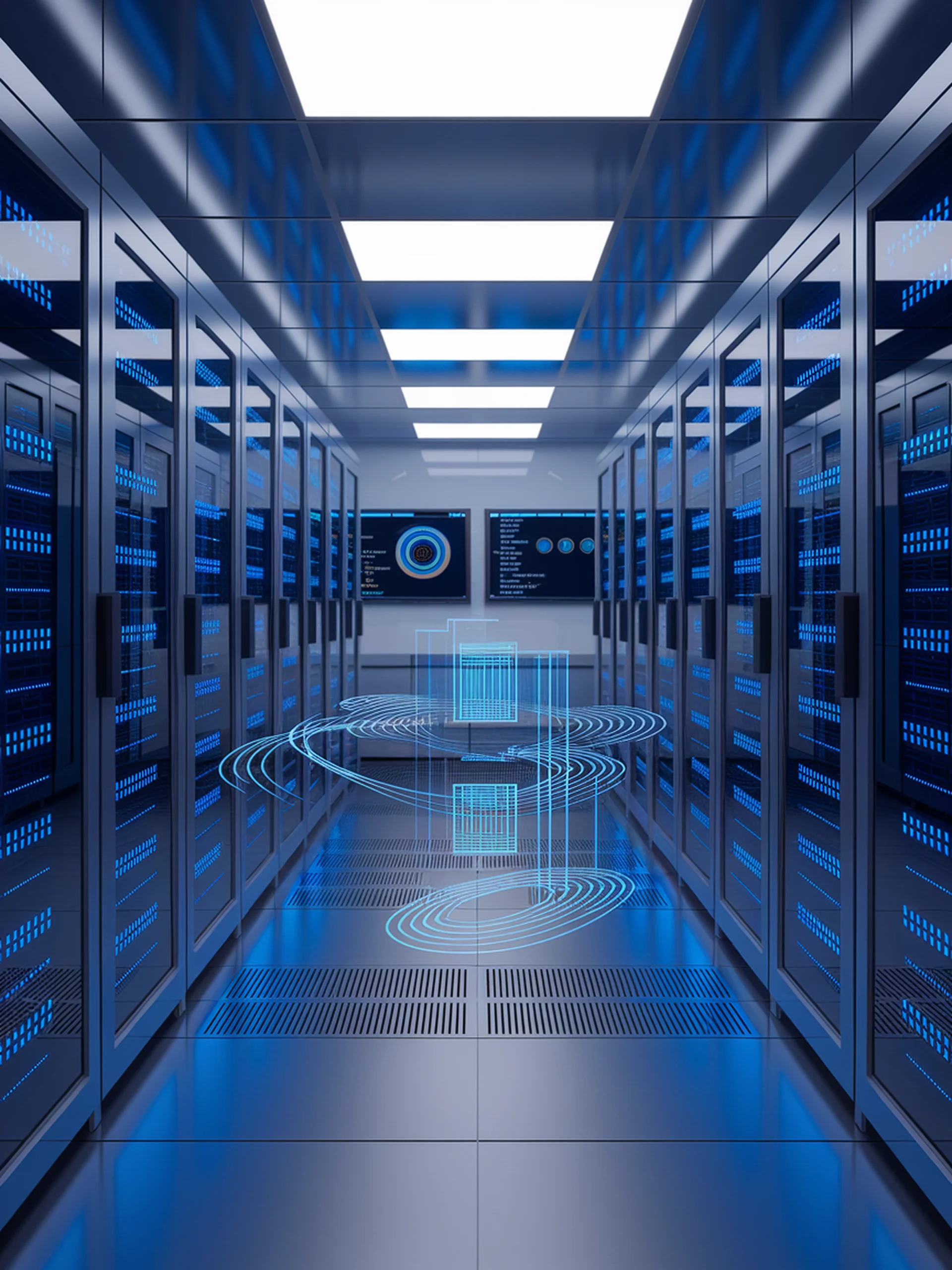
Cloud-Scale LLM Serving Breakthrough
A serverless architecture for efficient, scalable AI deployment
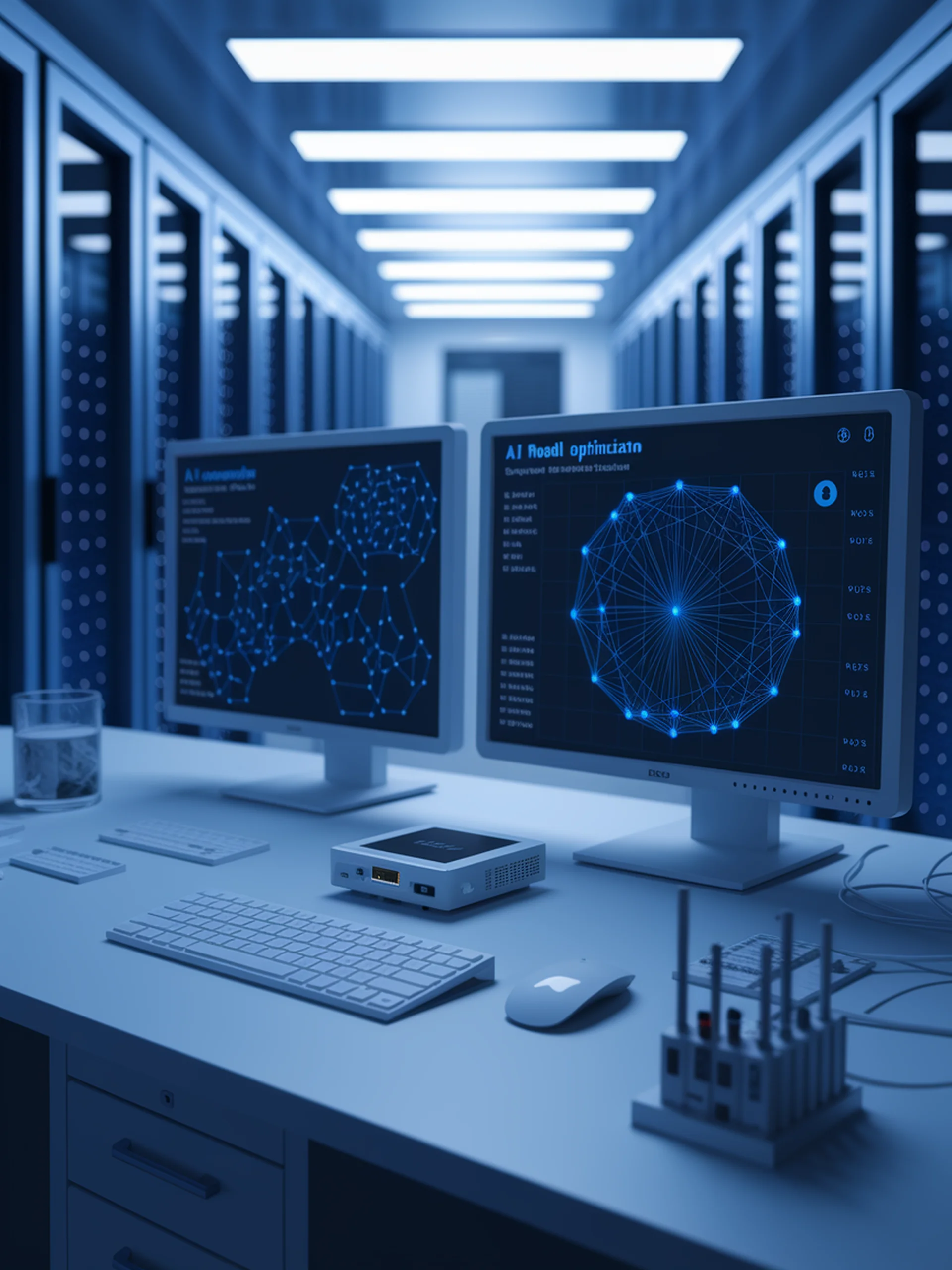
Shrinking LLMs Without Sacrificing Performance
A novel approach to efficient AI with selective pruning and weight-sharing
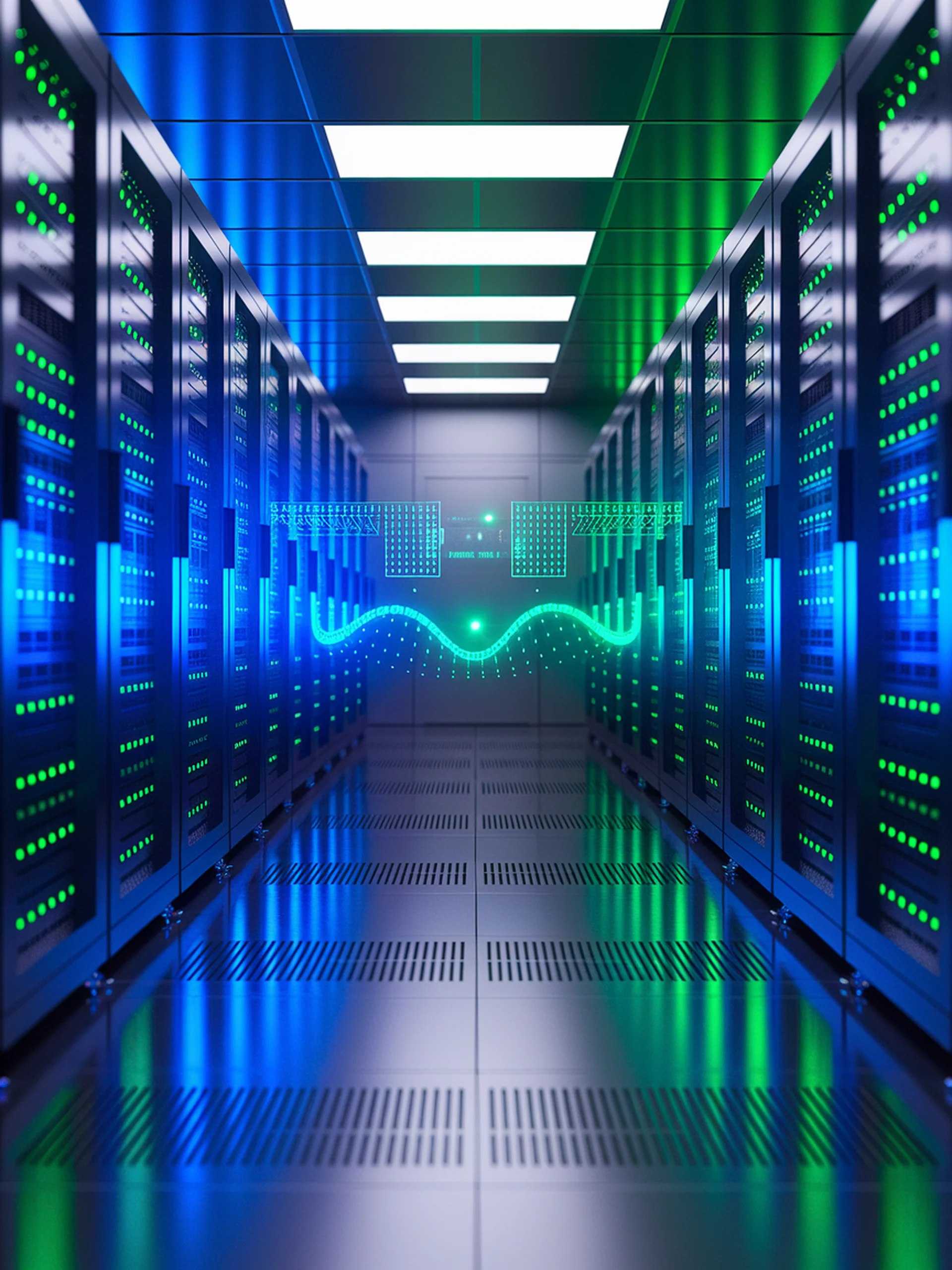
Boosting LLM Efficiency Through Smart Resource Sharing
How HyGen intelligently co-locates online and offline workloads
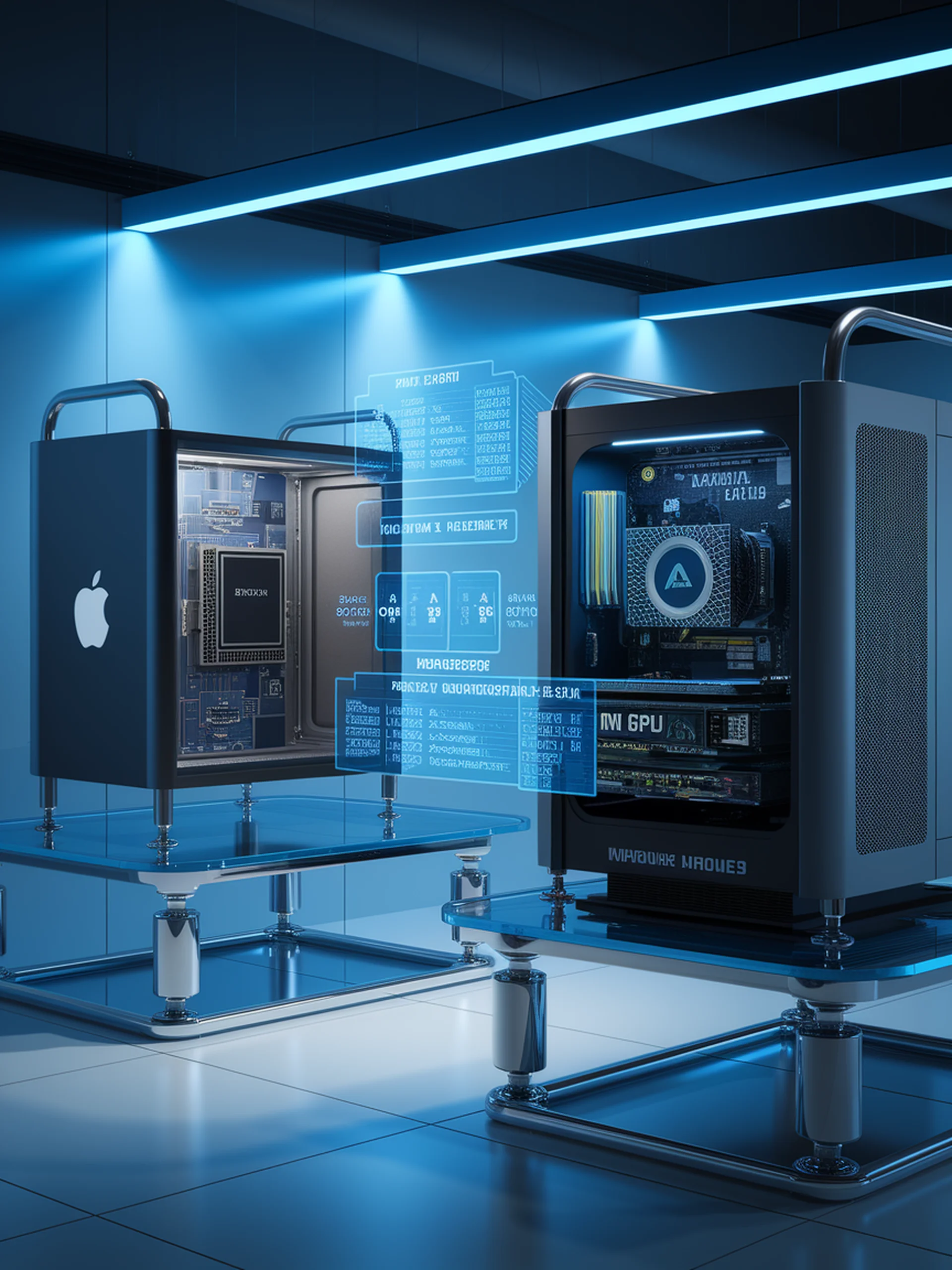
Apple Silicon vs. NVIDIA for ML Training
Evaluating Unified Memory Architecture Performance
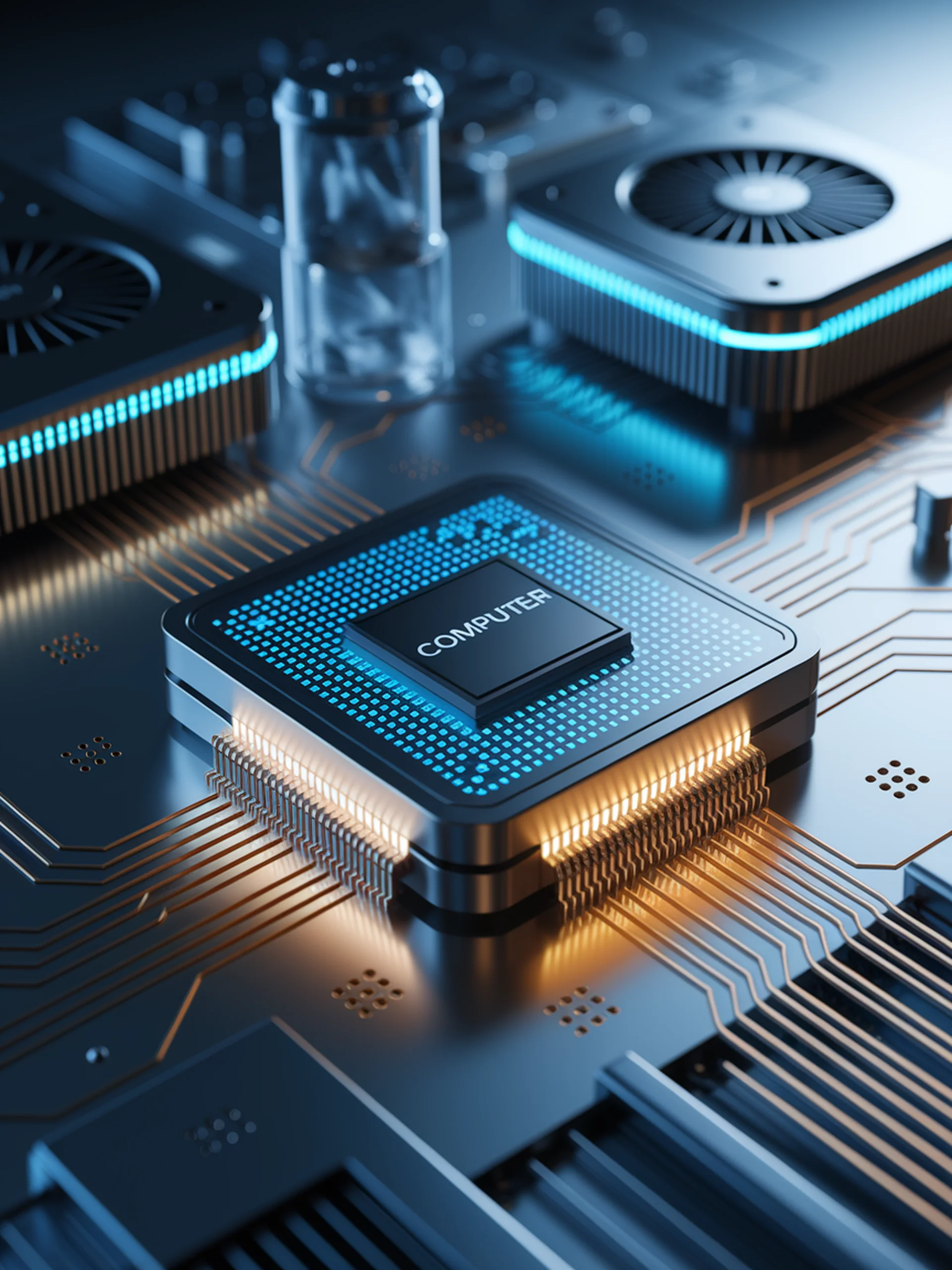
Smarter AI Compression: The One-Shot Approach
Policy-based pruning eliminates calibration dataset requirements
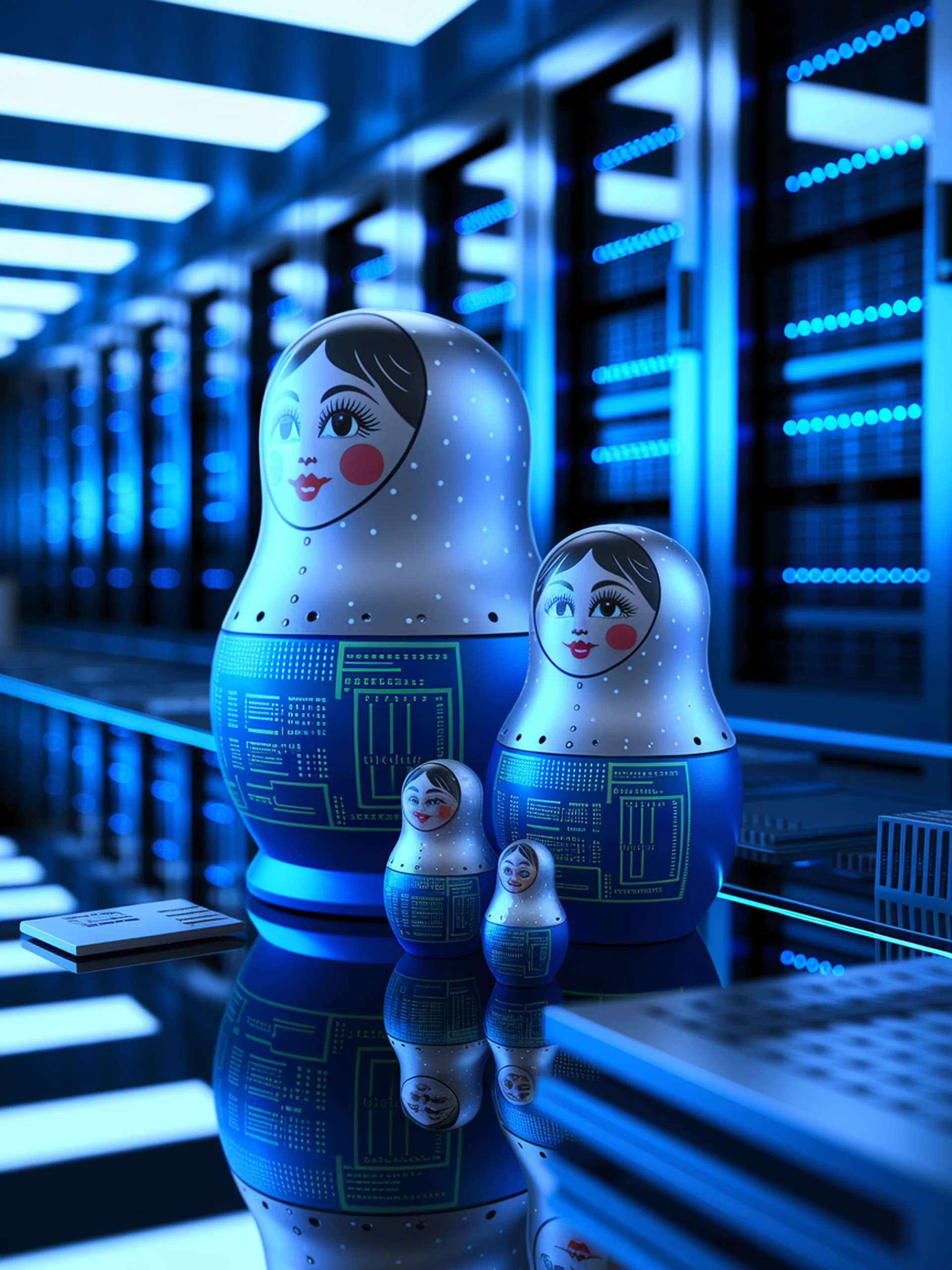
Flexible Re-Ranking for LLMs
Customizable Architecture for Security-Performance Balance
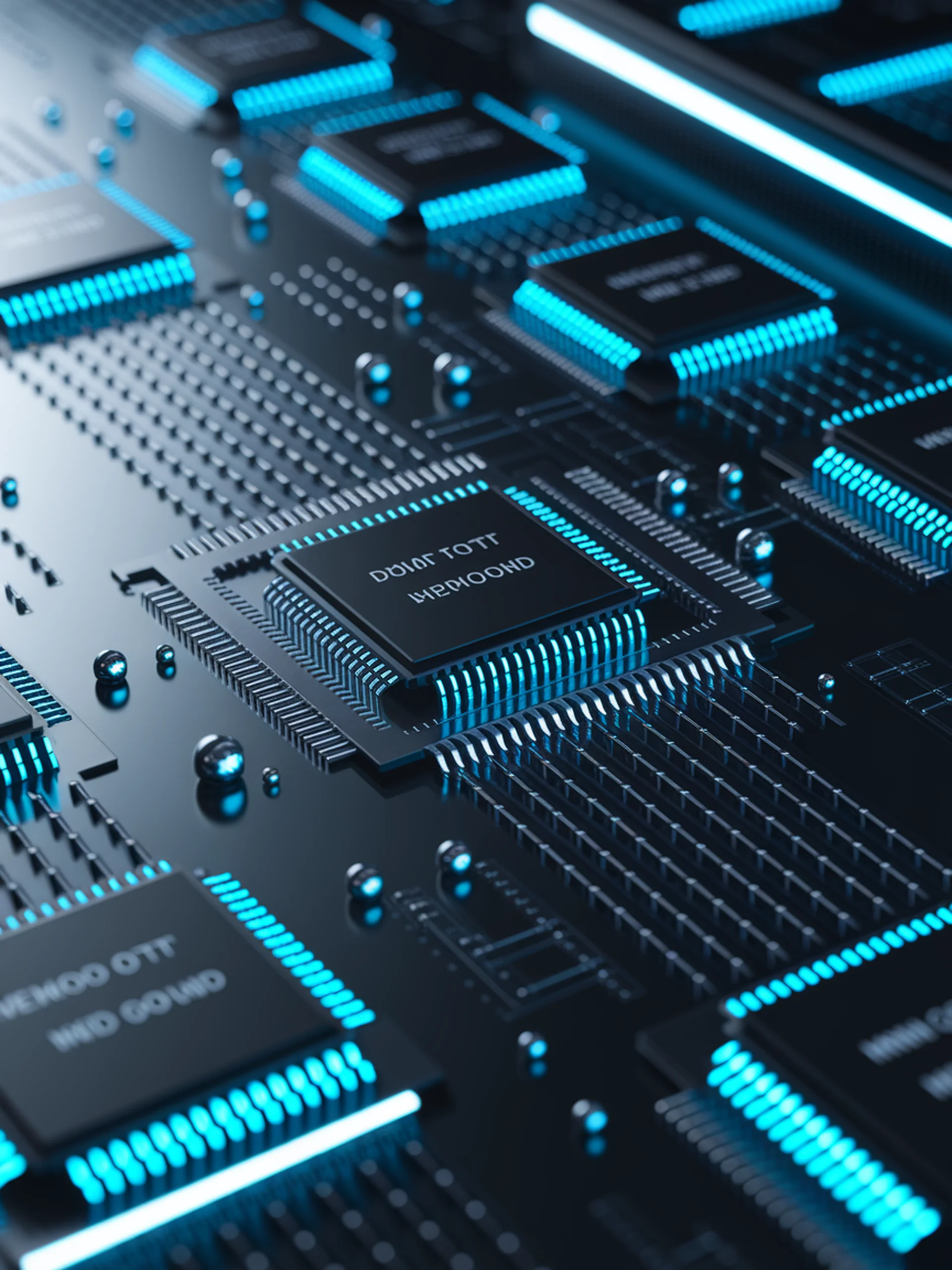
Squeezing More from LLM Memory
2-bit KV Cache Compression with Robust Performance
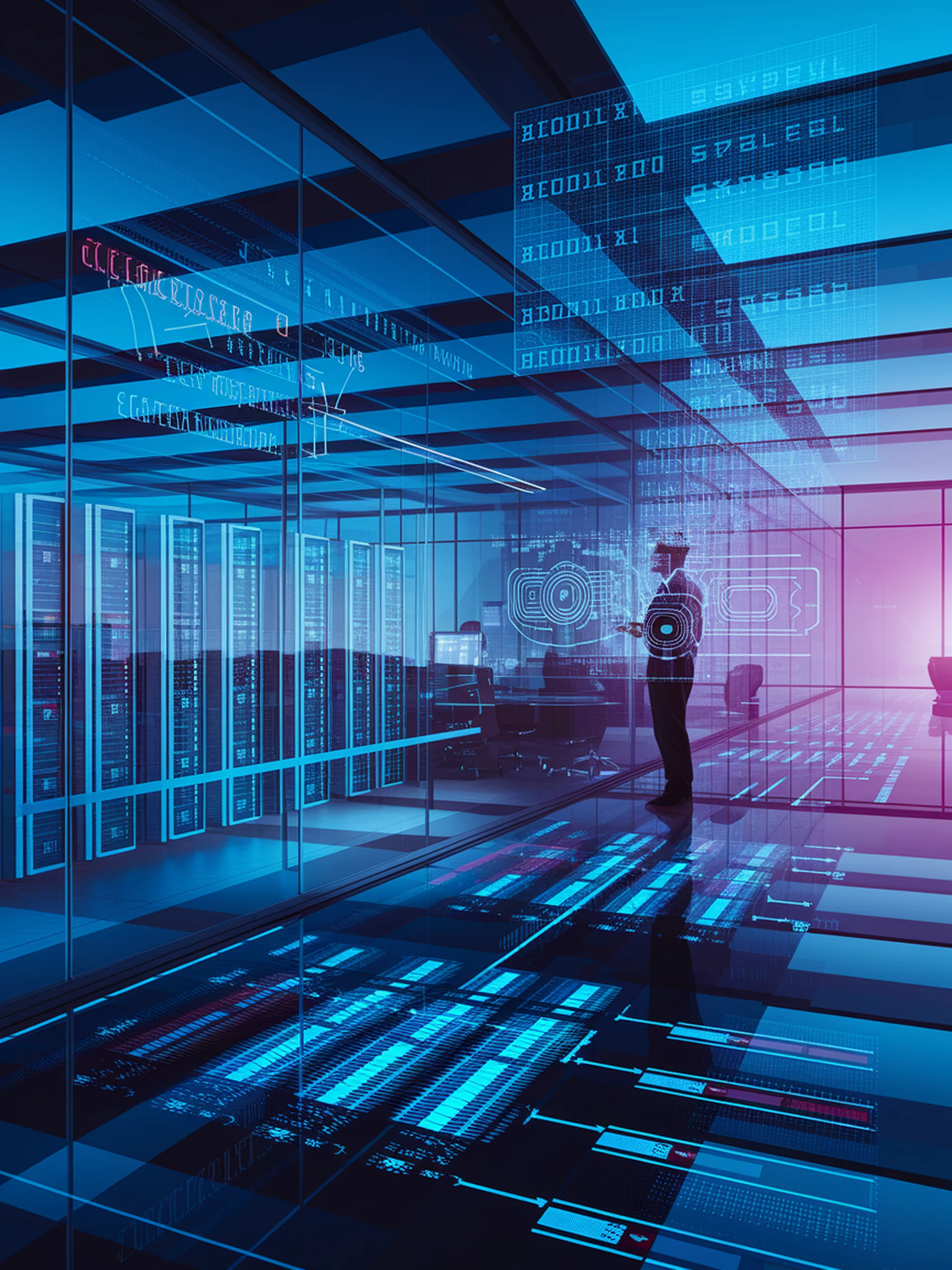
Optimizing LLM Workflows Automatically
Gradient-based prompt optimization for complex LLM pipelines
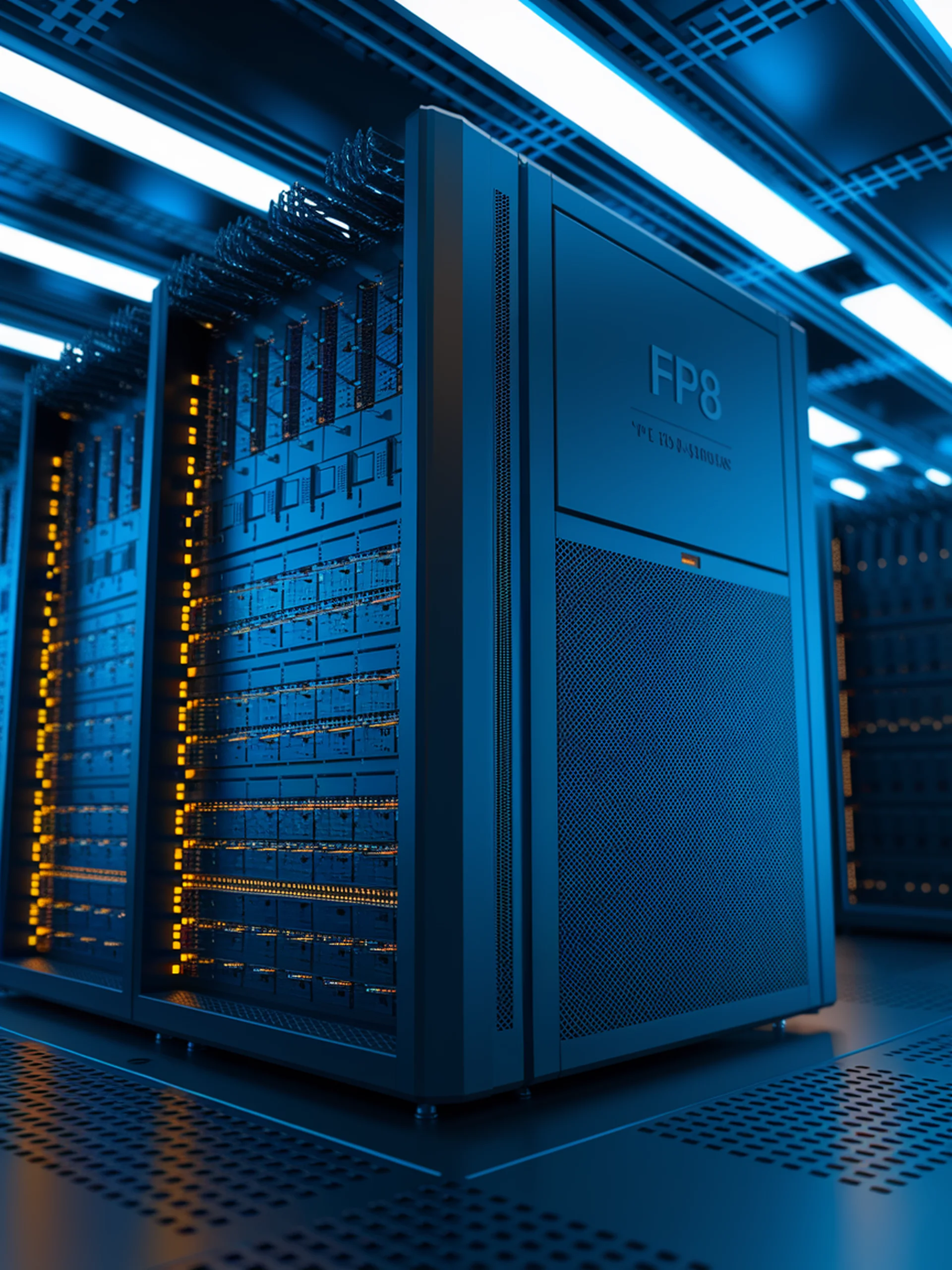
Breaking the FP8 Barrier in LLM Training
First successful implementation of FP4 precision for efficient LLM training
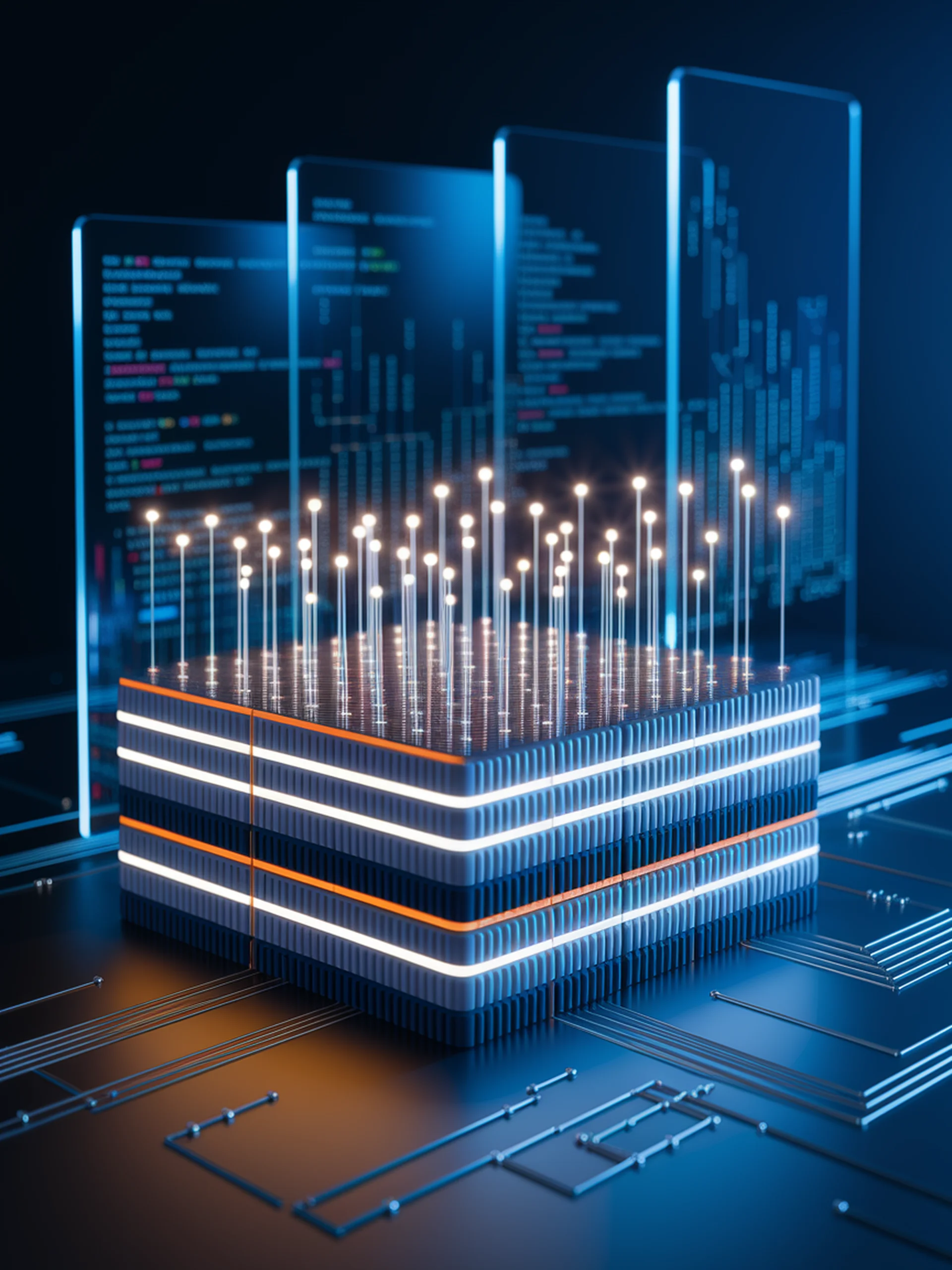
Enhancing LLM Memory for Long Texts
A novel technique to maintain contextual consistency without computational overhead
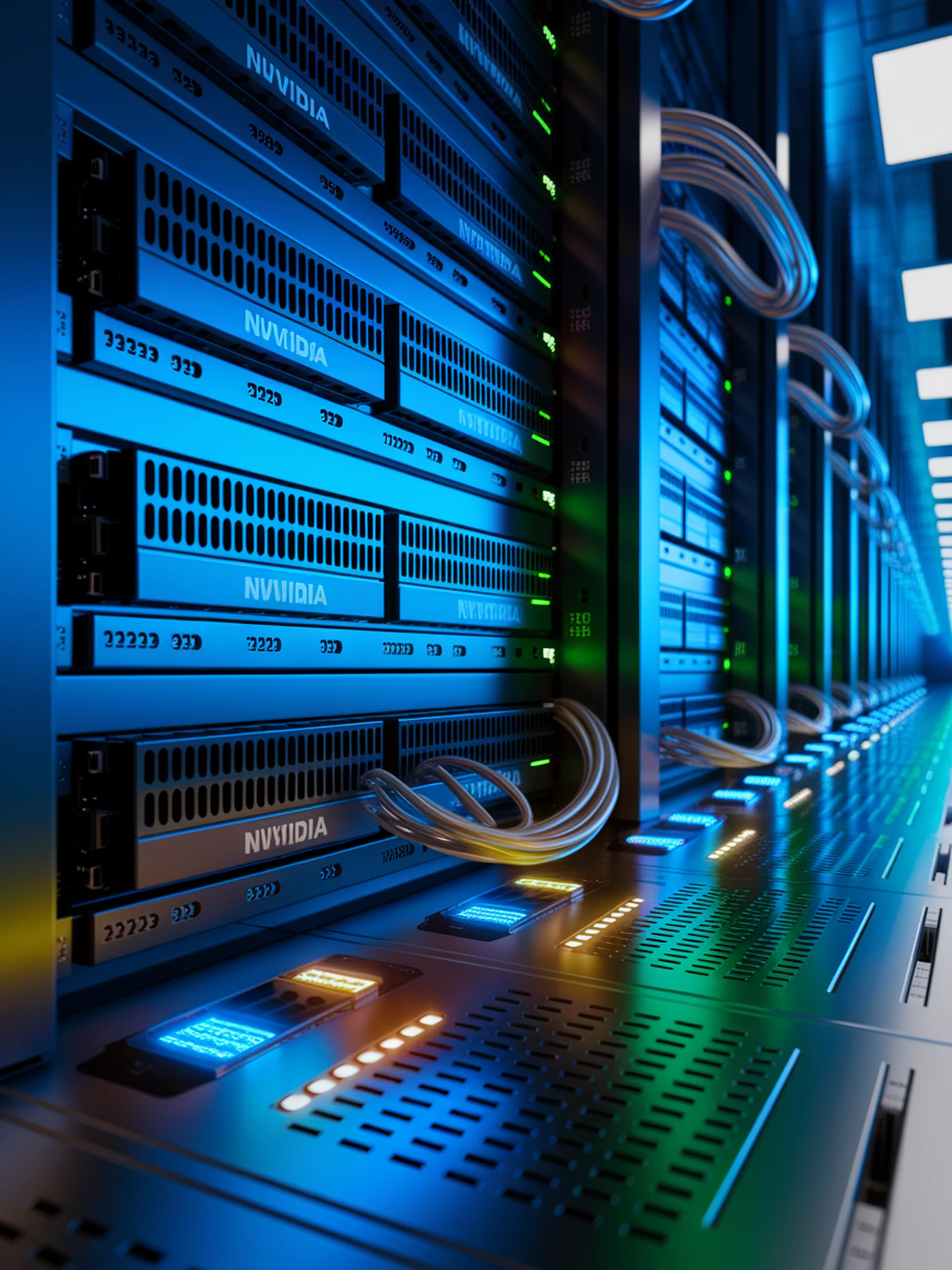
Smart Power Distribution for GPU Sharing
Accurately tracking power consumption in multi-tenant GPU environments
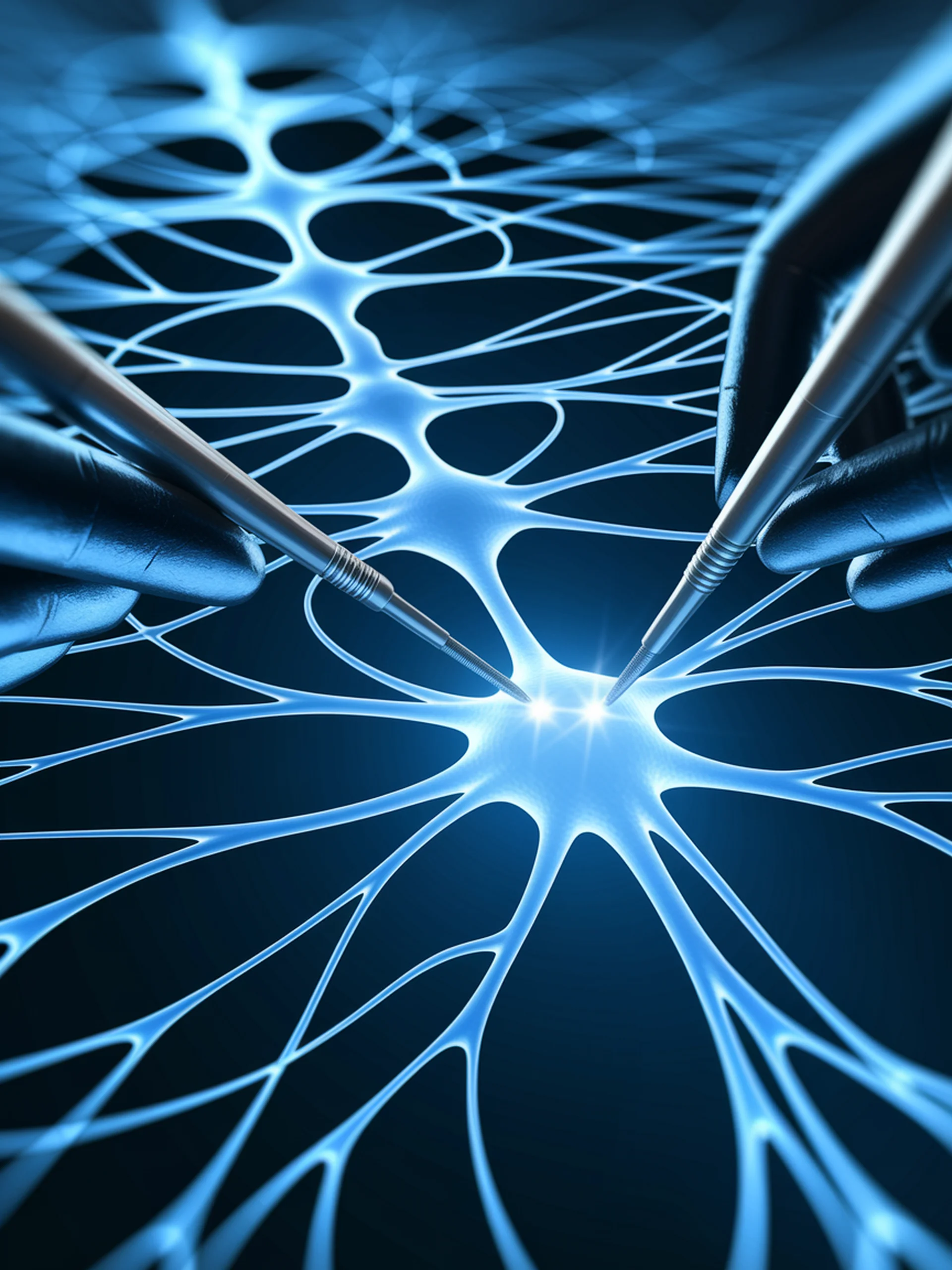
Smarter, Leaner LLMs
A Two-Stage Approach to Efficient Model Pruning
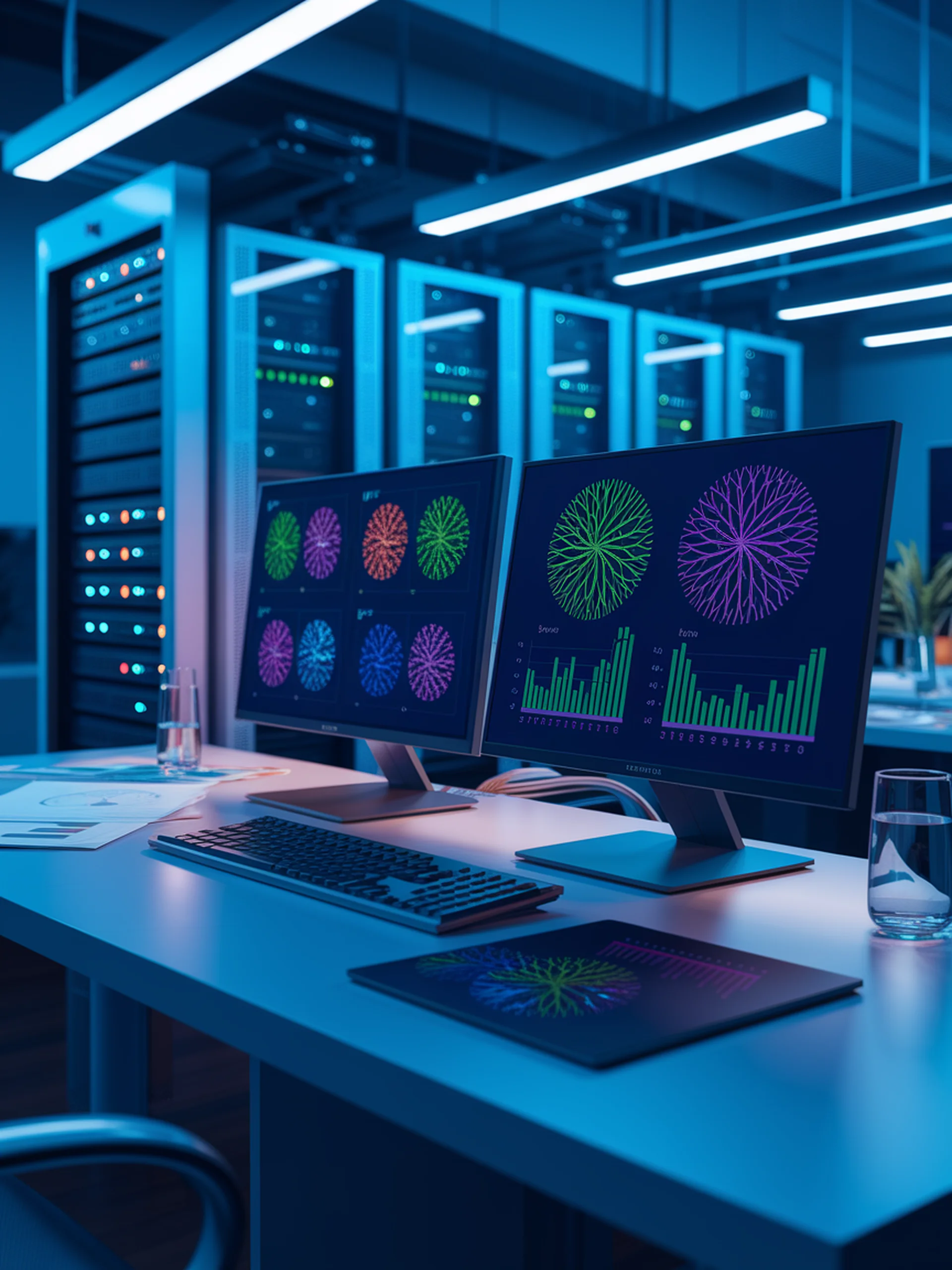
Streamlining LLMs for Better Efficiency
A data-driven approach to pruning without performance loss
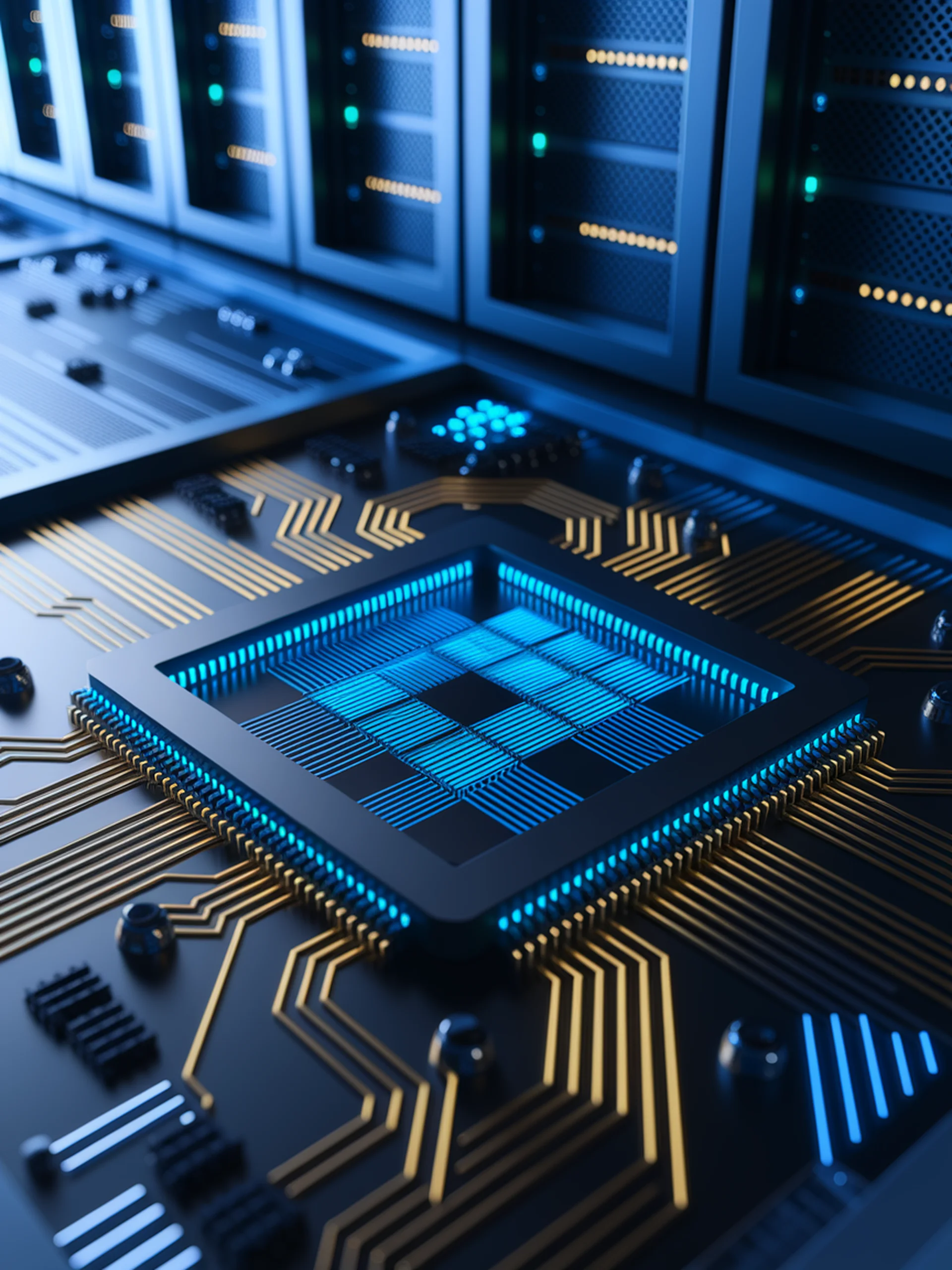
Smarter Parameter Updates for LLMs
Enhancing training efficiency with selective parameter optimization
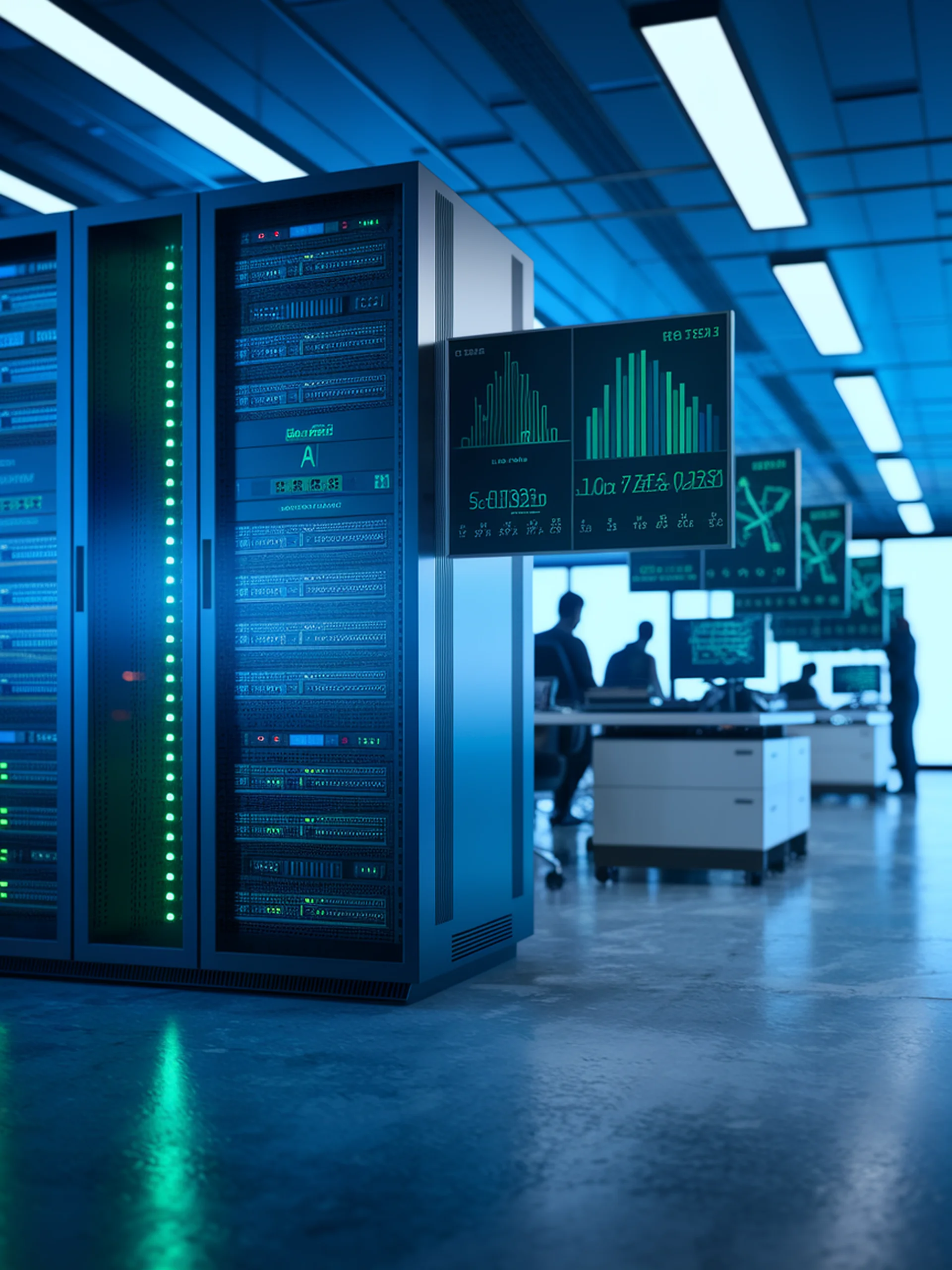
Optimizing LLMs for Inference Speed
Rethinking scaling laws to balance performance and efficiency
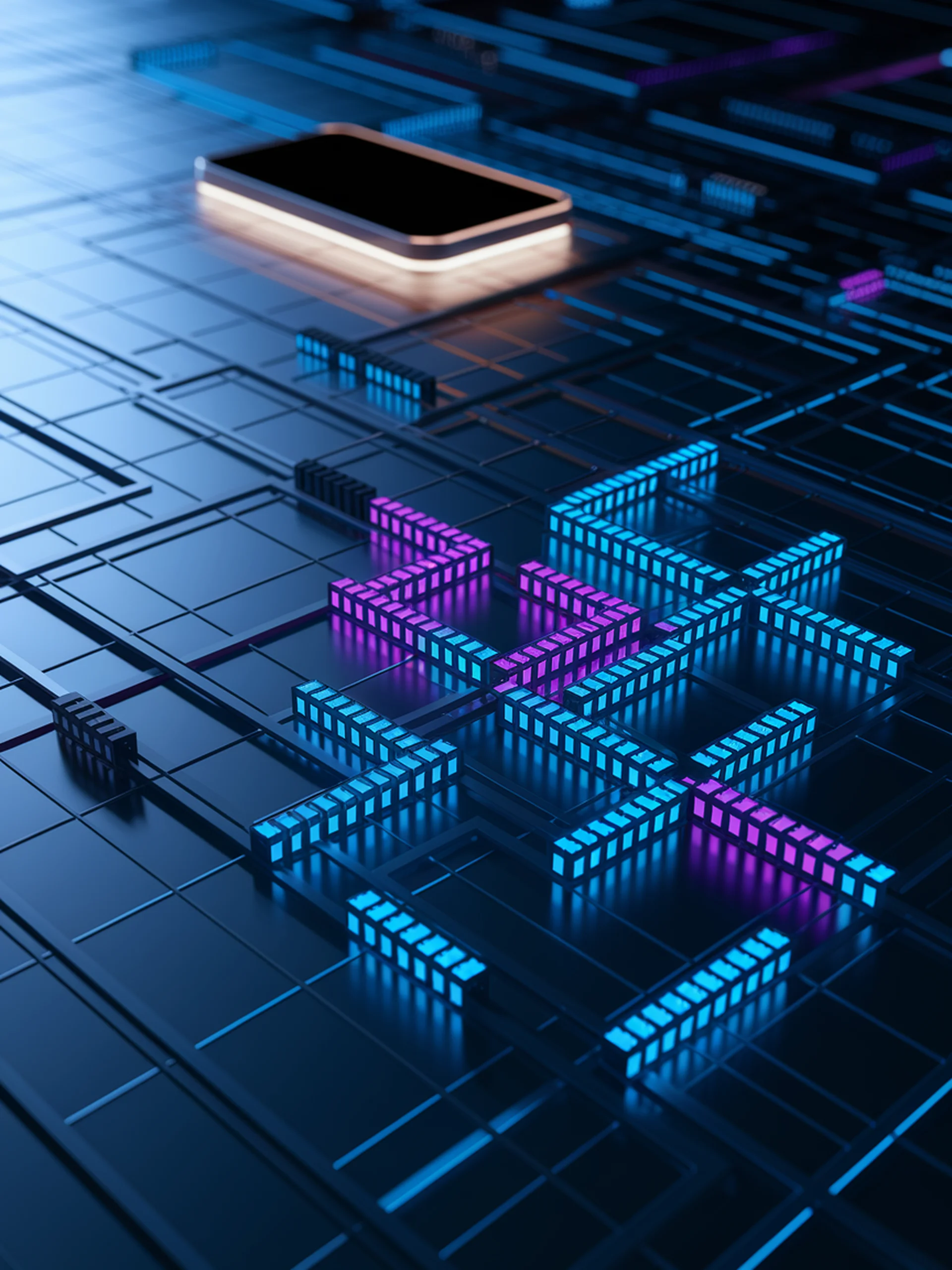
Smarter LLM Compression
Graph Neural Networks Enable Ultra-Low-Bit Model Quantization
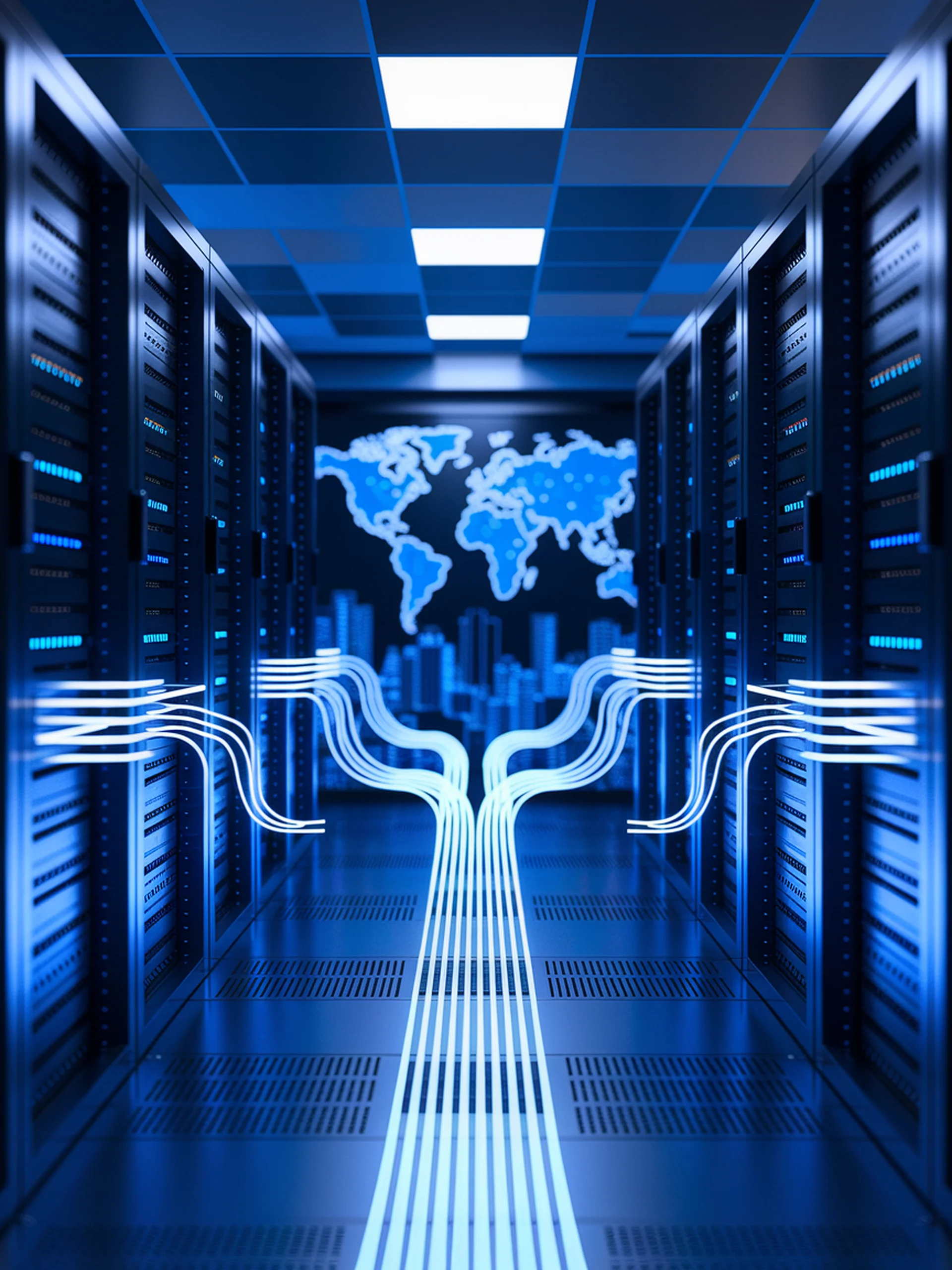
Breaking Distance Barriers in LLM Training
Optimizing distributed learning with overlapping communication
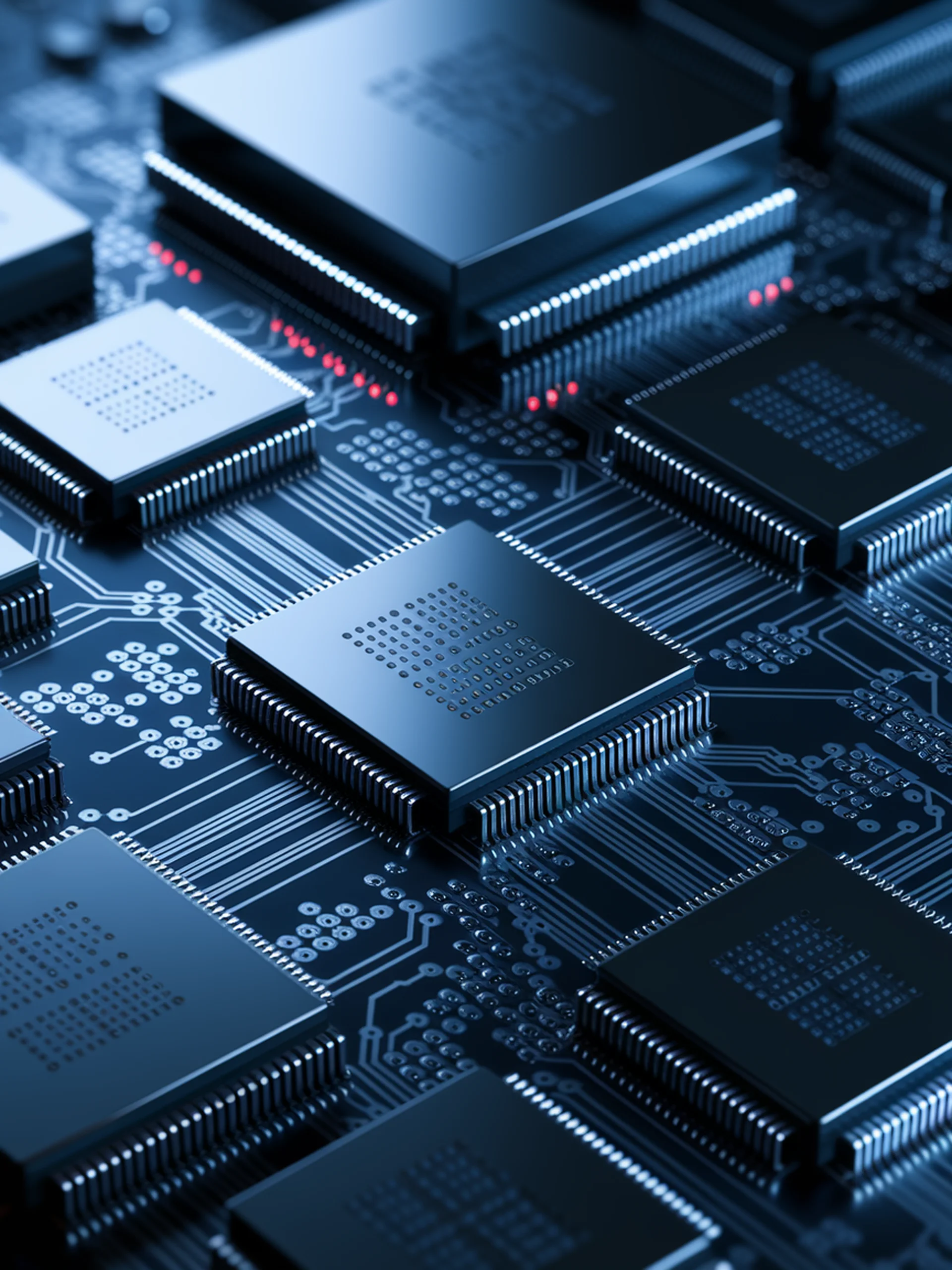
Boosting LLM Efficiency Through Symbolic Compression
A formal approach to enhance token efficiency while maintaining interpretability
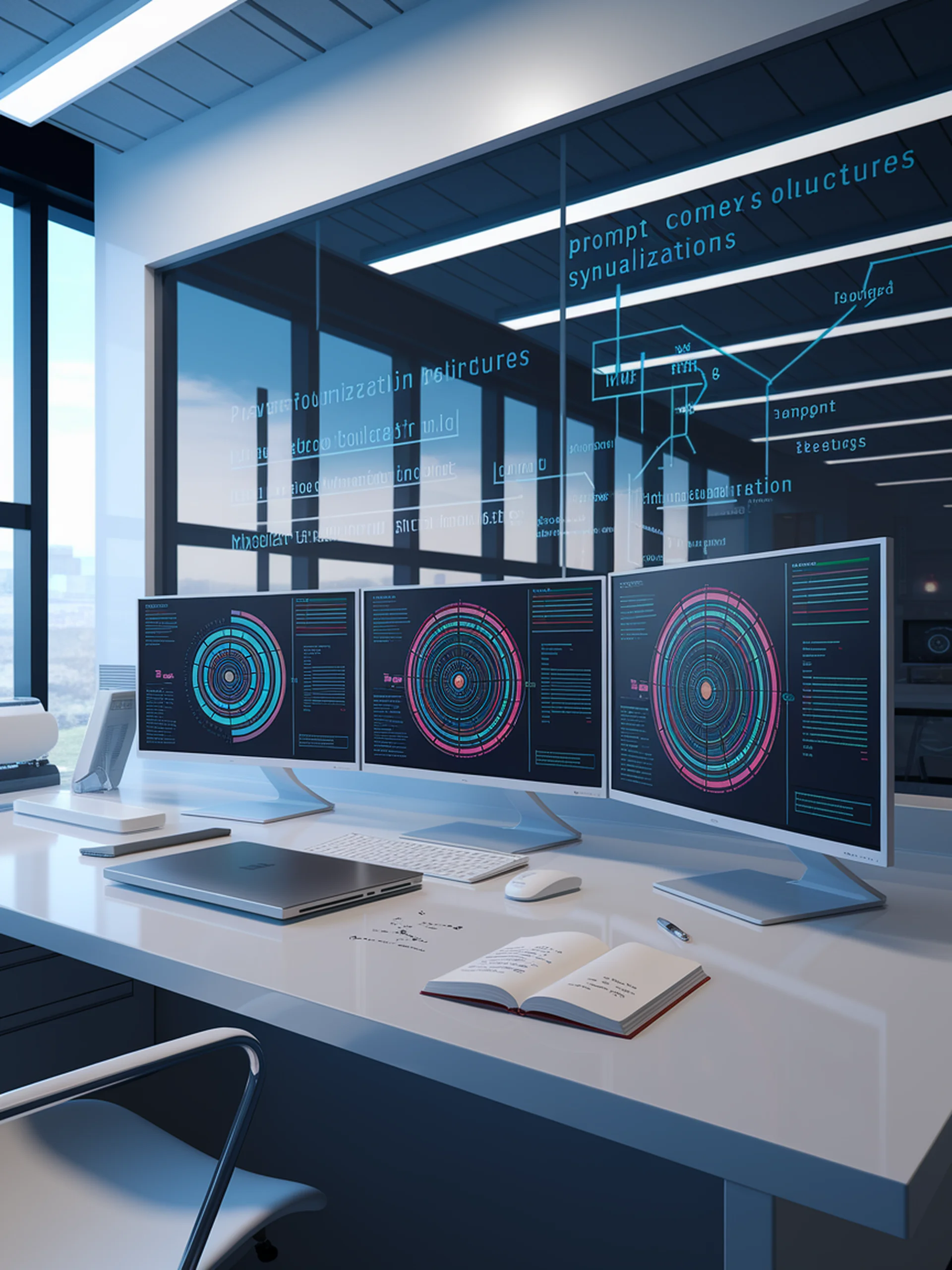
Smarter Prompts, Better Results
A Novel Approach to Optimize LLM Interactions
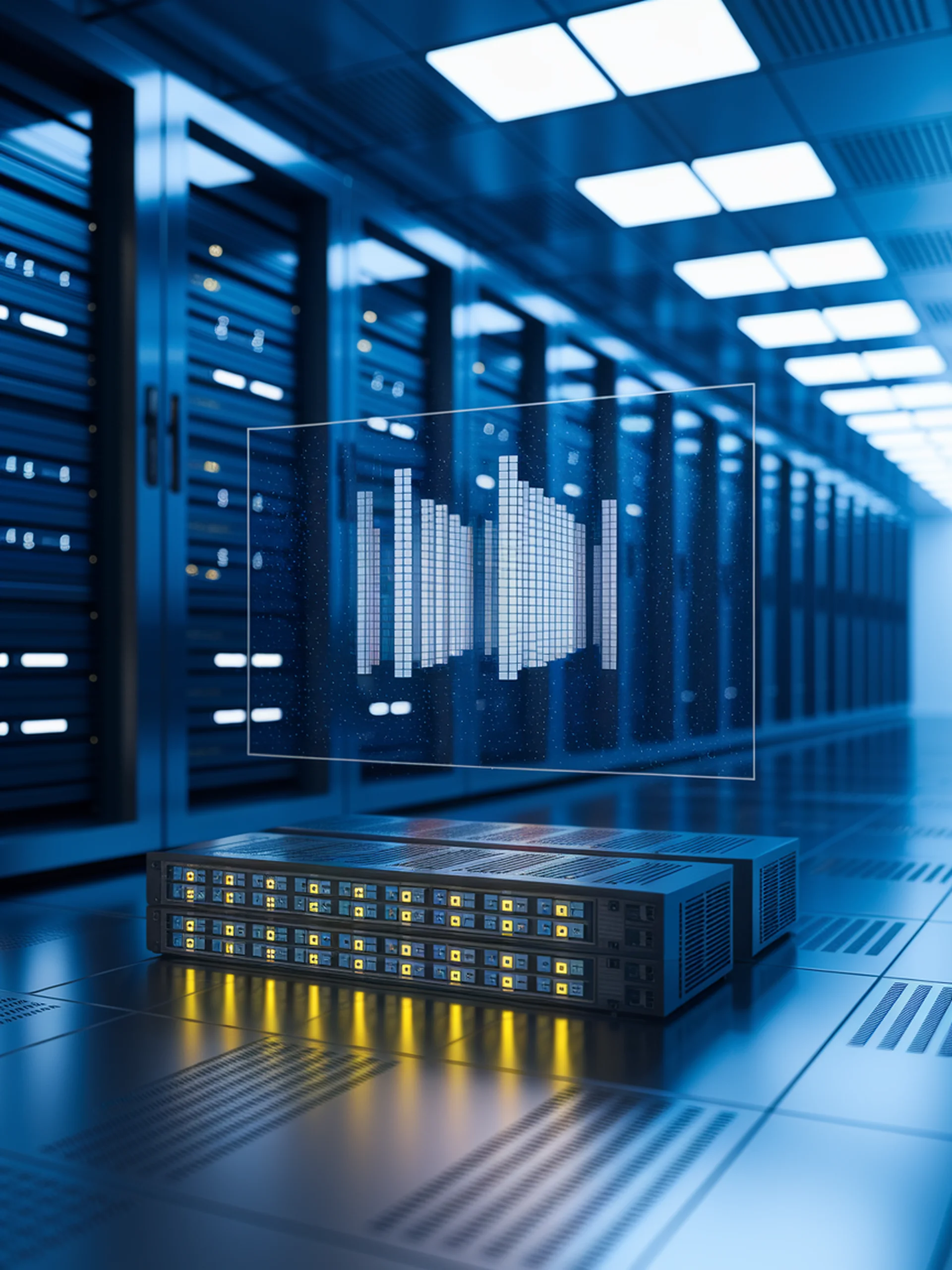
Advancing LLM Fine-tuning Efficiency
Leveraging Temporal Low-Rankness in Zeroth-Order Optimization
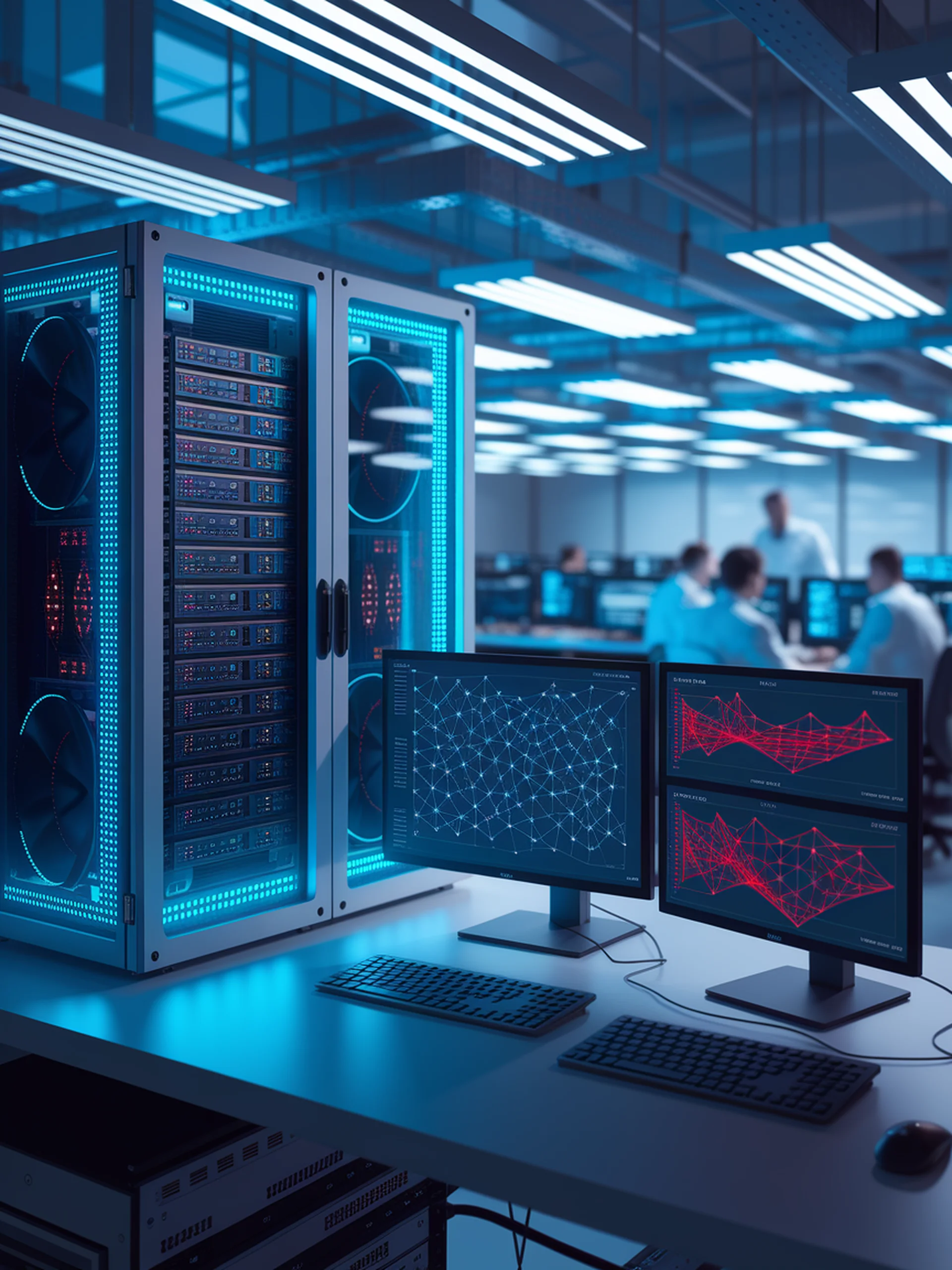
PIFA: Revolutionizing LLM Compression
A Novel Low-Rank Pruning Approach for Efficient AI Deployment
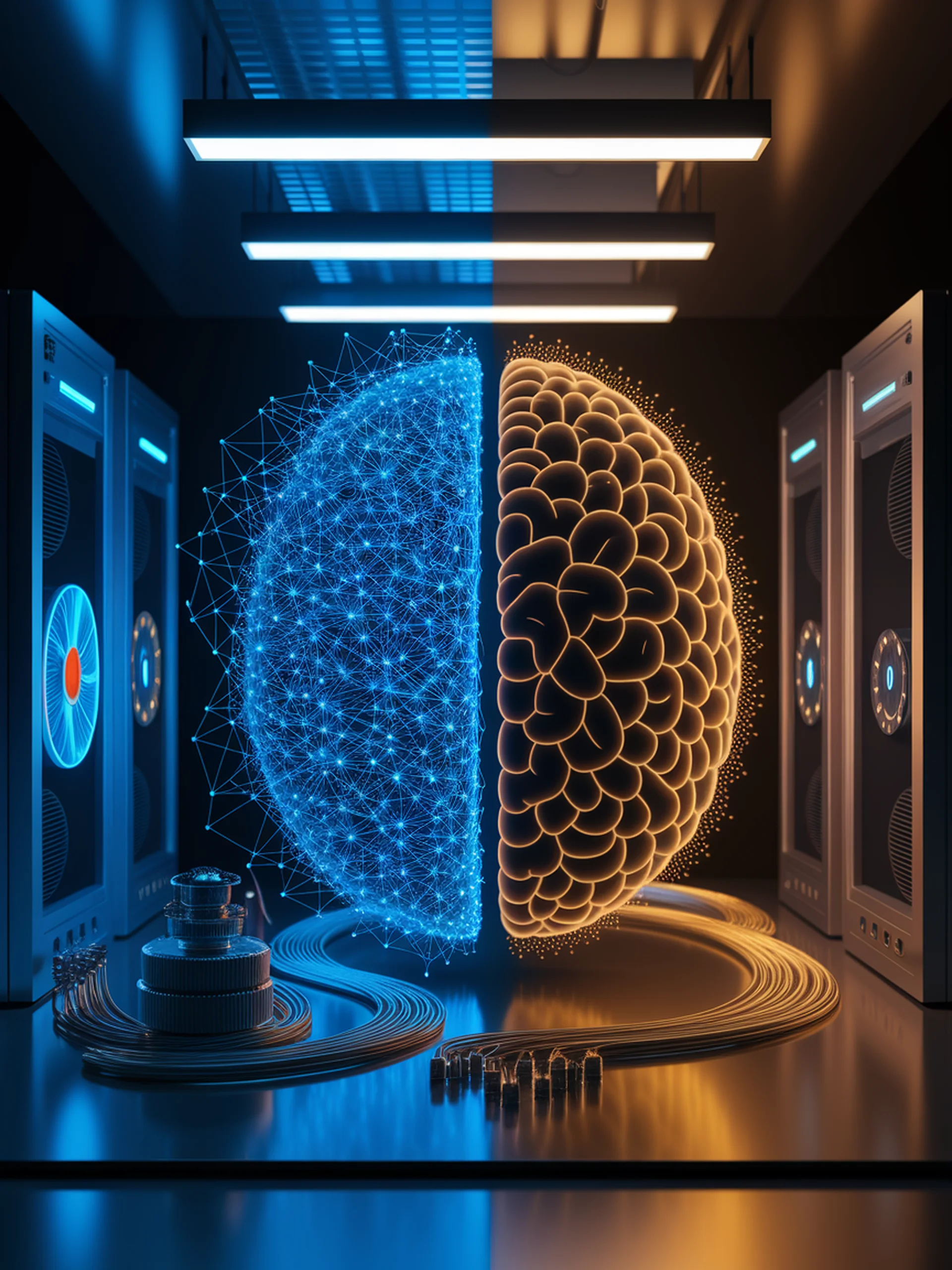
Brain-Inspired Sparse Training for LLMs
Achieving Full Performance with Reduced Computational Resources
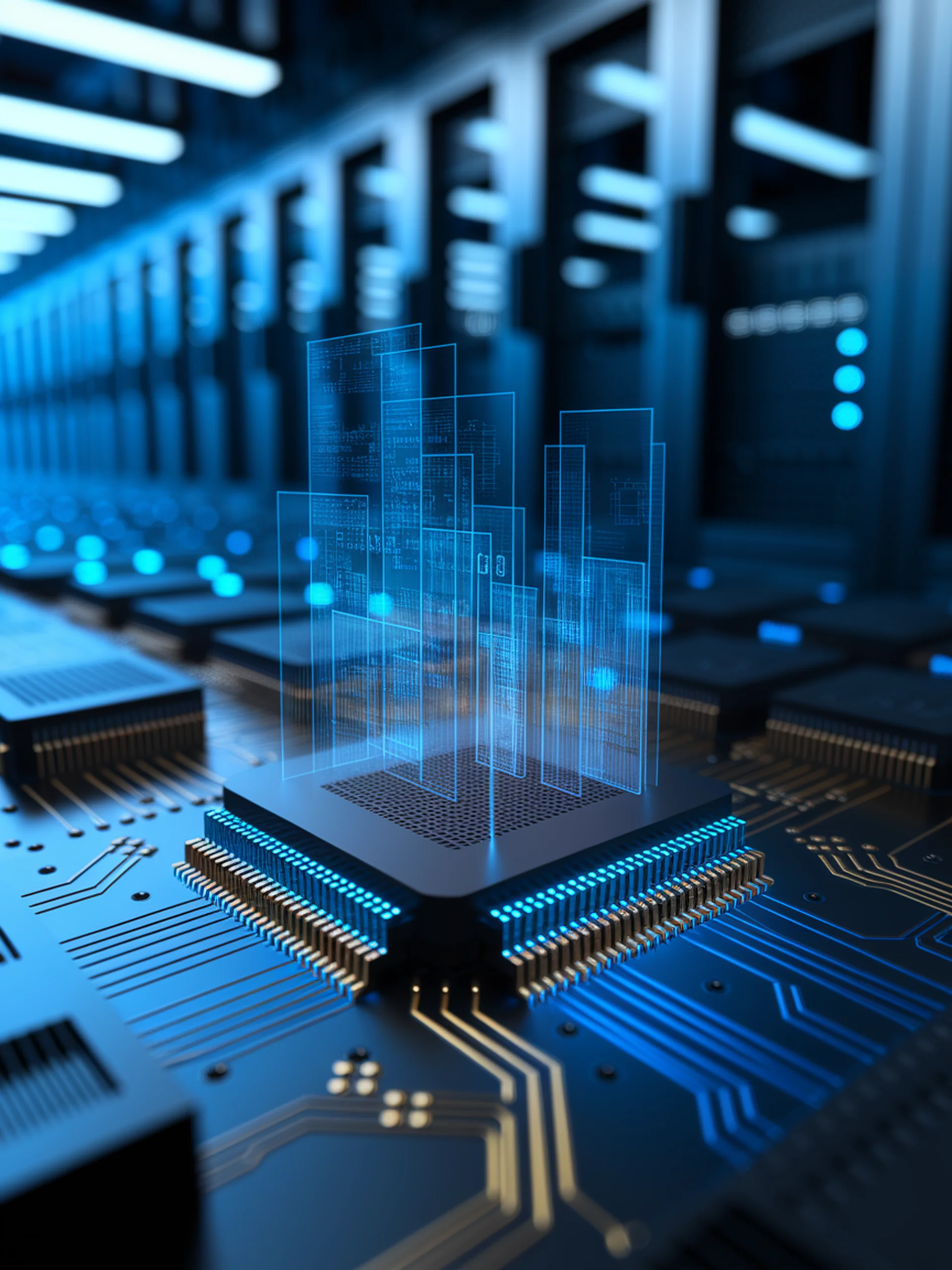
Supercharging LLMs with Hardware-Efficient Compression
Tensor-Train Decomposition for FPGA Acceleration
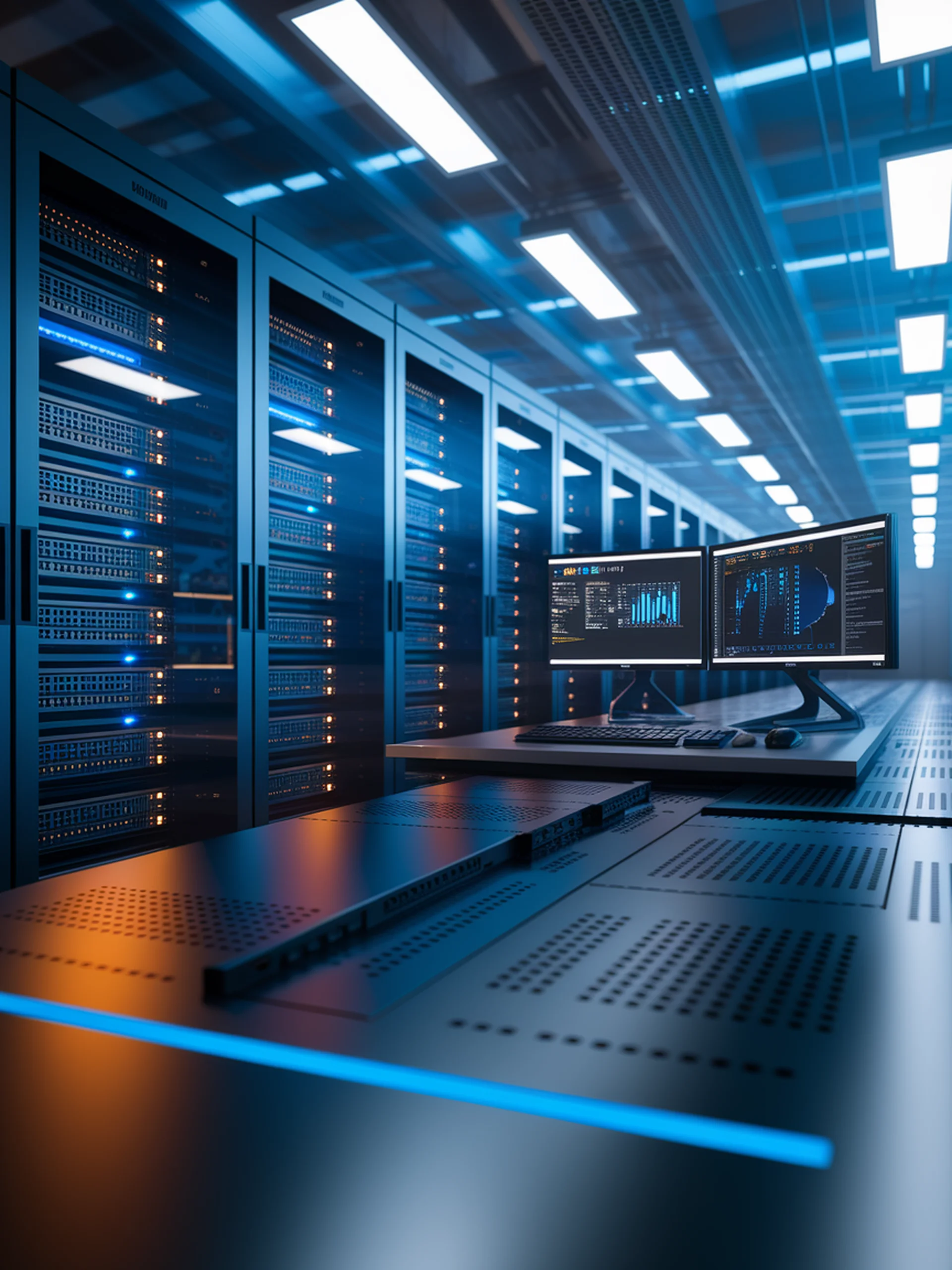
Accelerating LLM Inference with Judge Decoding
Beyond Alignment: A New Paradigm for Faster Speculative Sampling
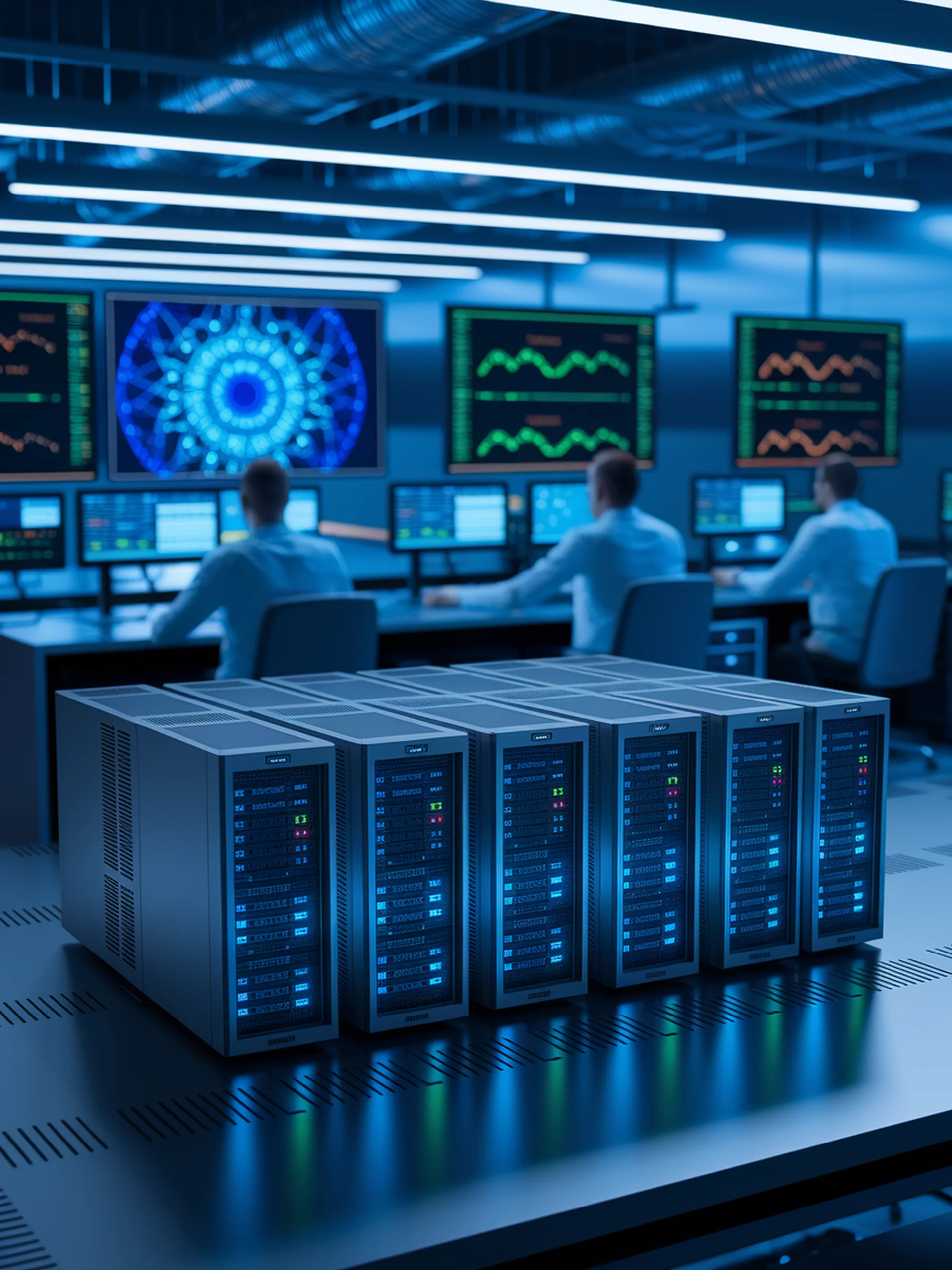
Faster, Smarter LLM Reasoning
Boosting efficiency with reward-guided speculative decoding
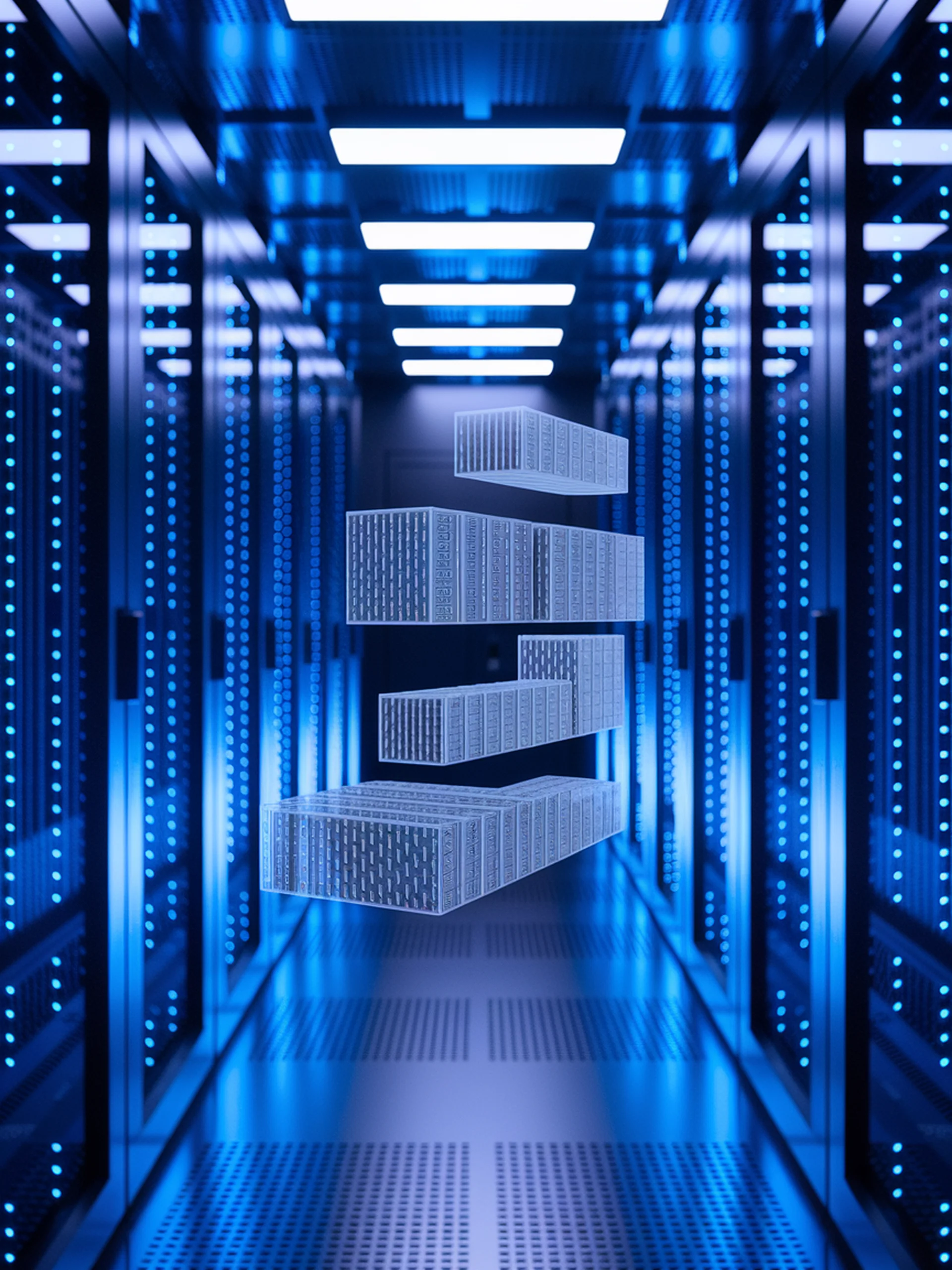
Cache Me If You Must: Adaptive Key-Value Quantization for La...
By Alina Shutova, Vladimir Malinovskii...
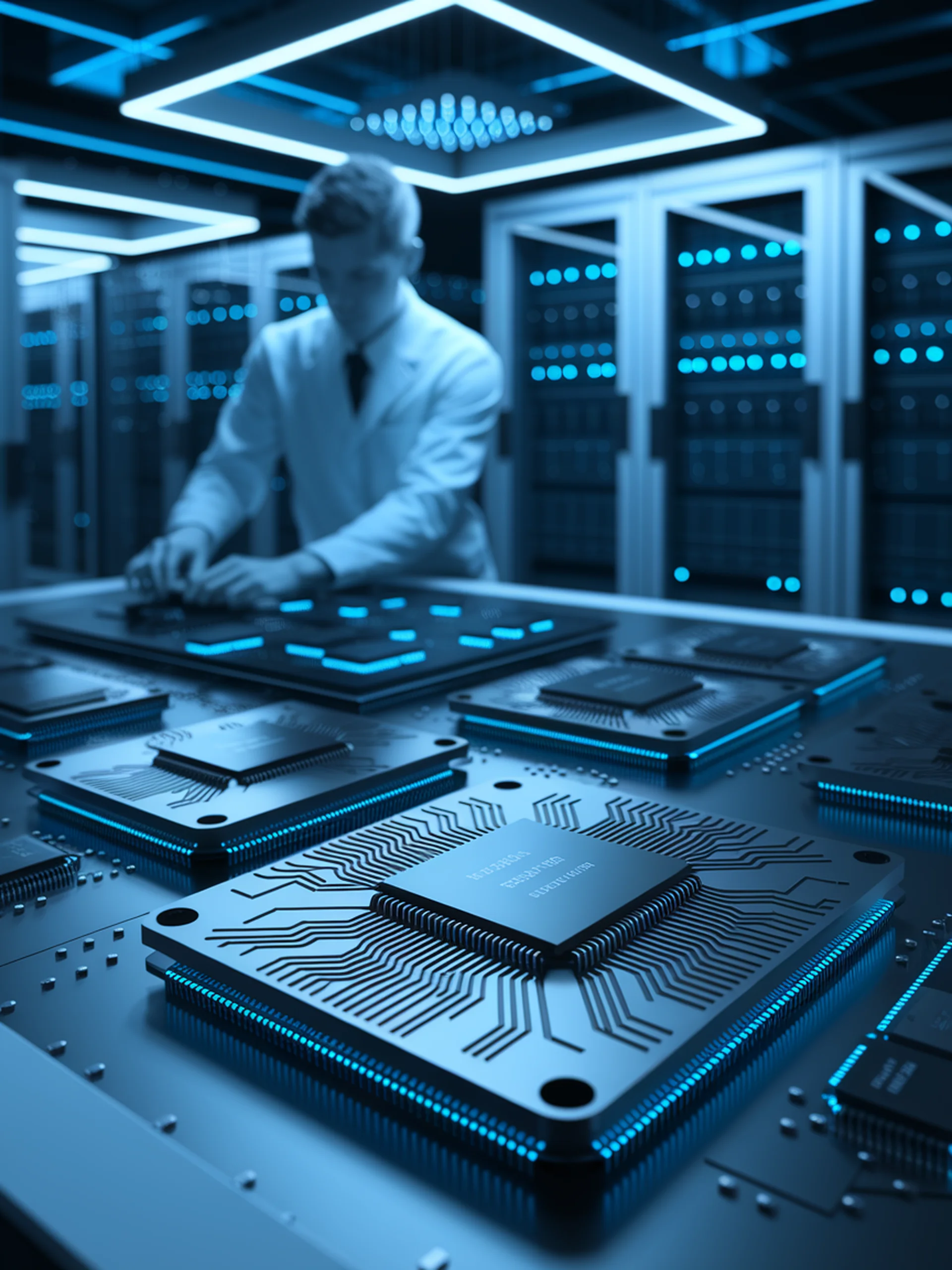
Optimizing LLMs with Block Floating Point
Breaking efficiency barriers for nonlinear operations in LLMs

Accelerating LLM Beam Search
Novel Trie-Based Decoding for Efficient, High-Quality Text Generation

Advancing LLMs with Tensorial Reconfiguration
A breakthrough approach for handling long-range dependencies
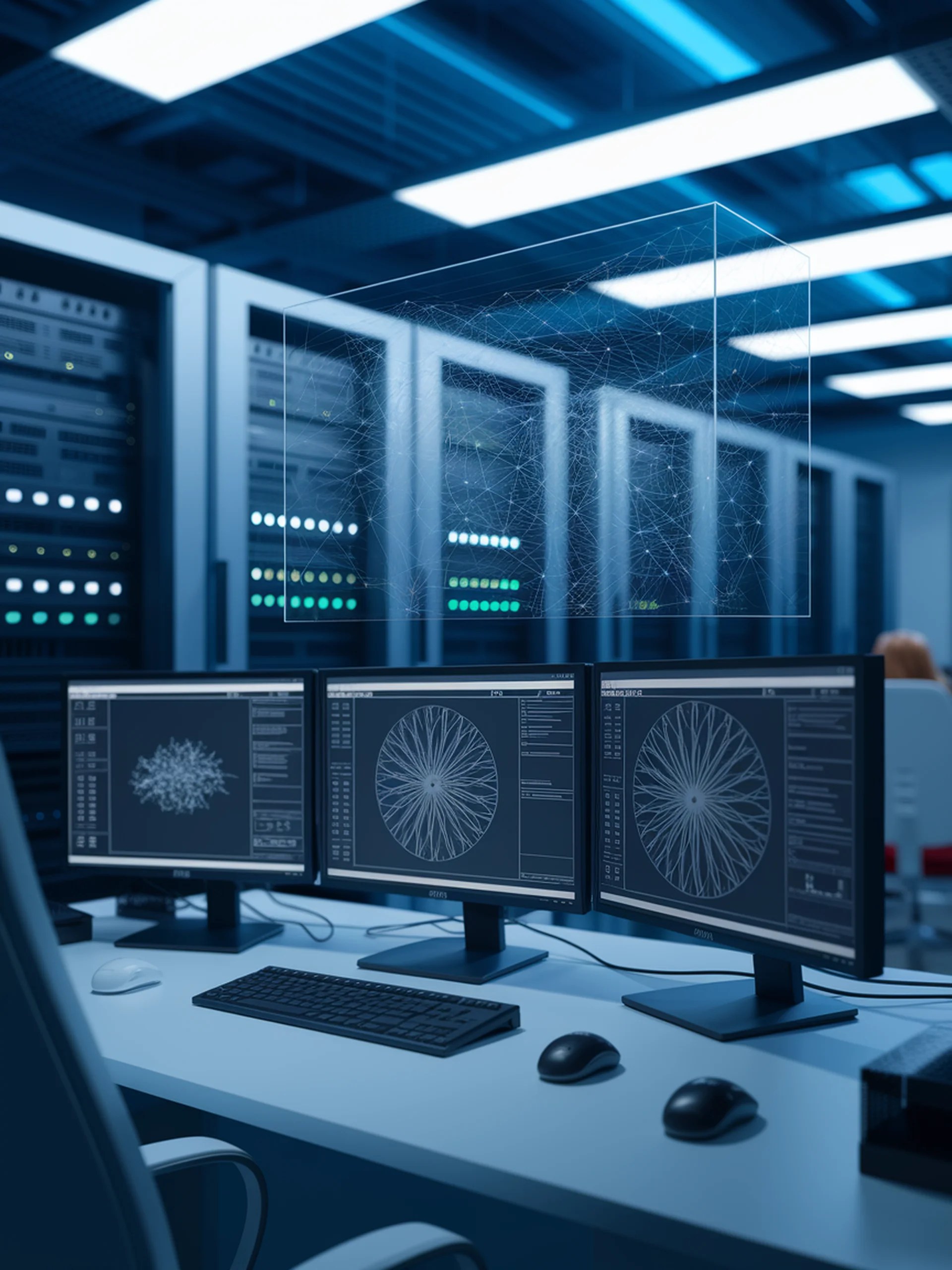
Smart Compression for Large Language Models
Learning-based pruning for more efficient LLMs
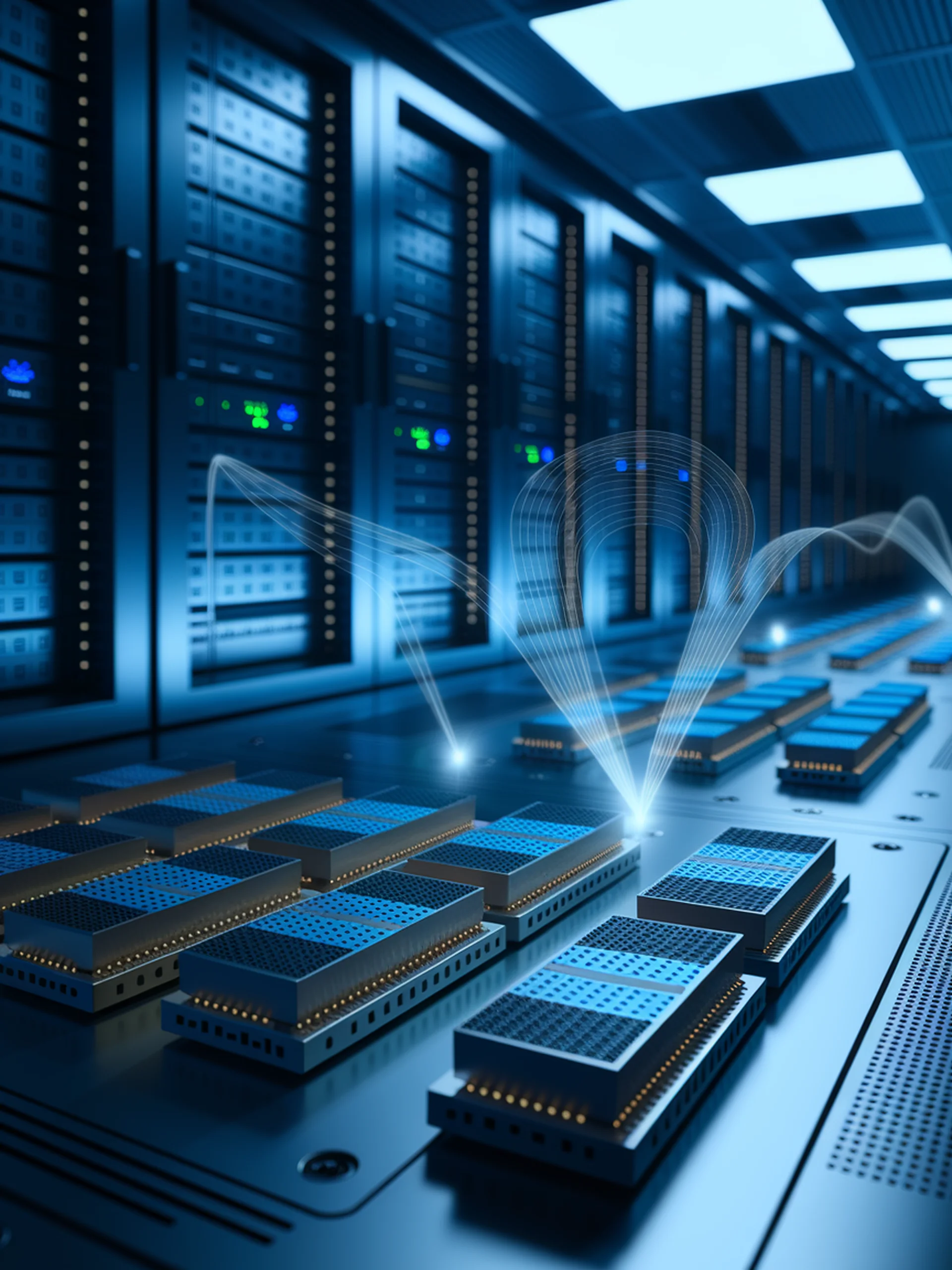
Making LLMs Memory-Efficient
Semantic-Aware Compression for Long-Context AI
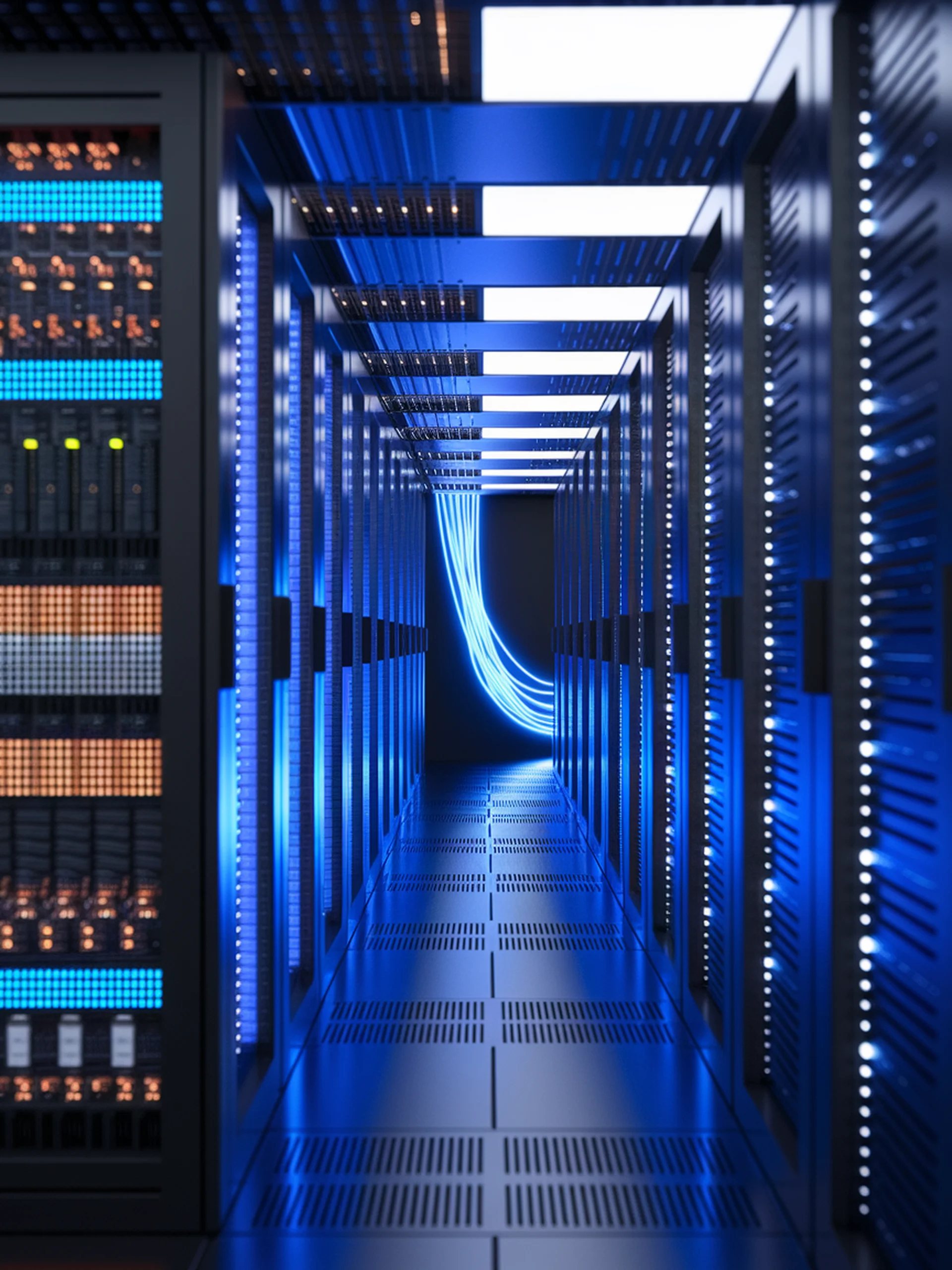
Accelerating LLM Training with Smarter Token Filtering
How Collider transforms sparse operations into efficient dense computations
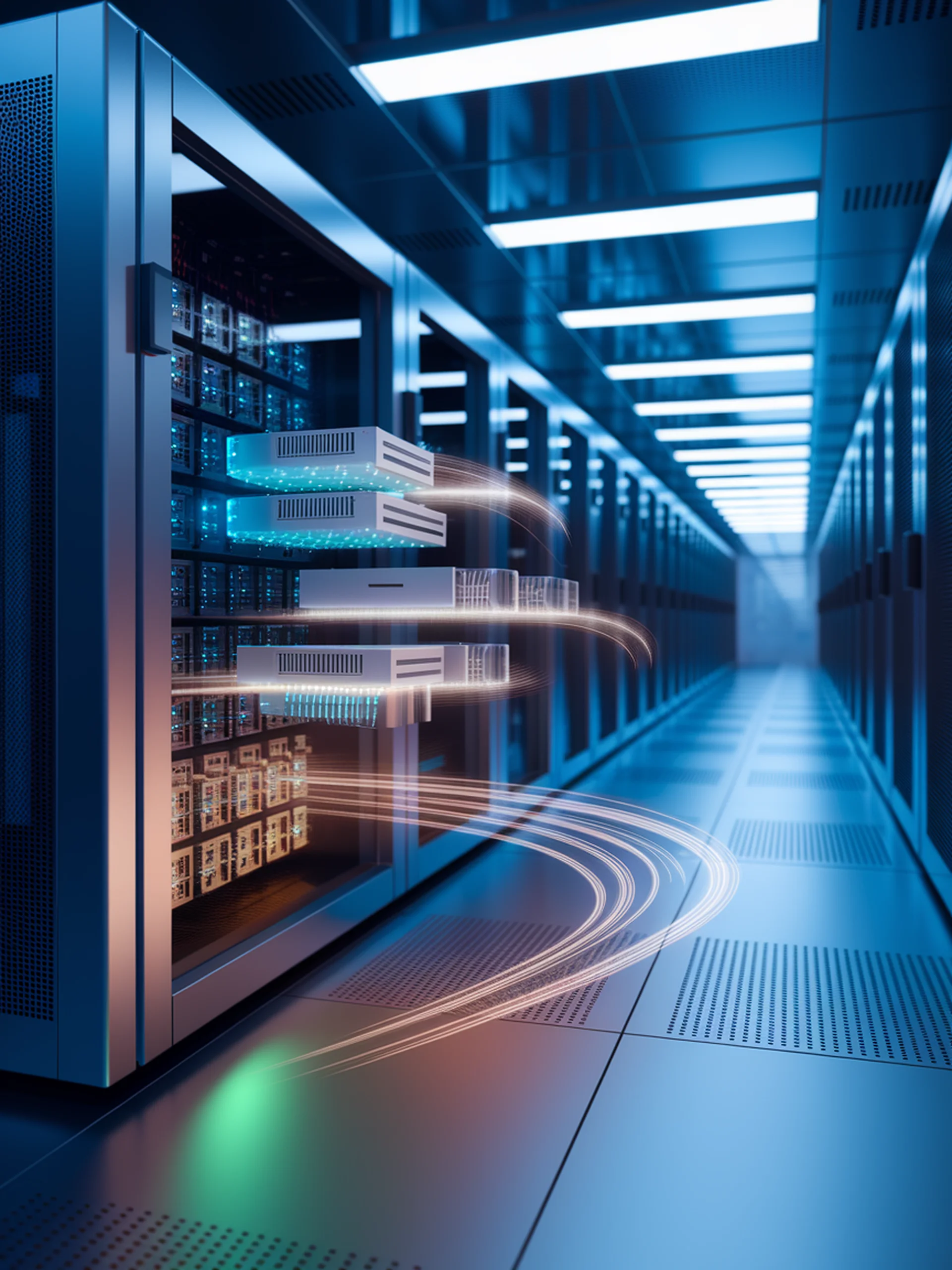
Making Multimodal AI Faster & Lighter
Full Static Quantization for Efficient Multimodal LLMs
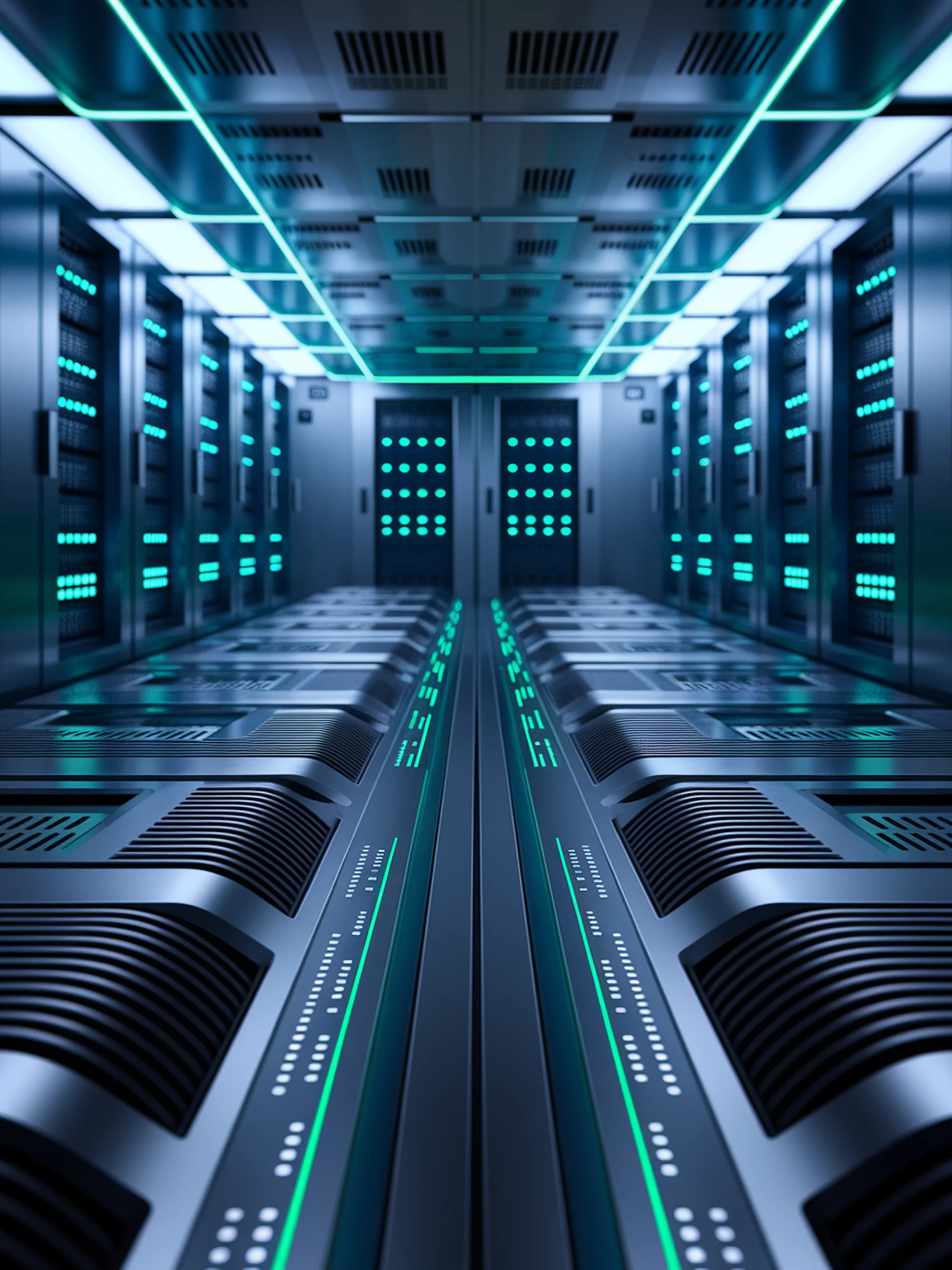
Optimizing LLM Inference Performance
Reducing costs through unified softmax operations
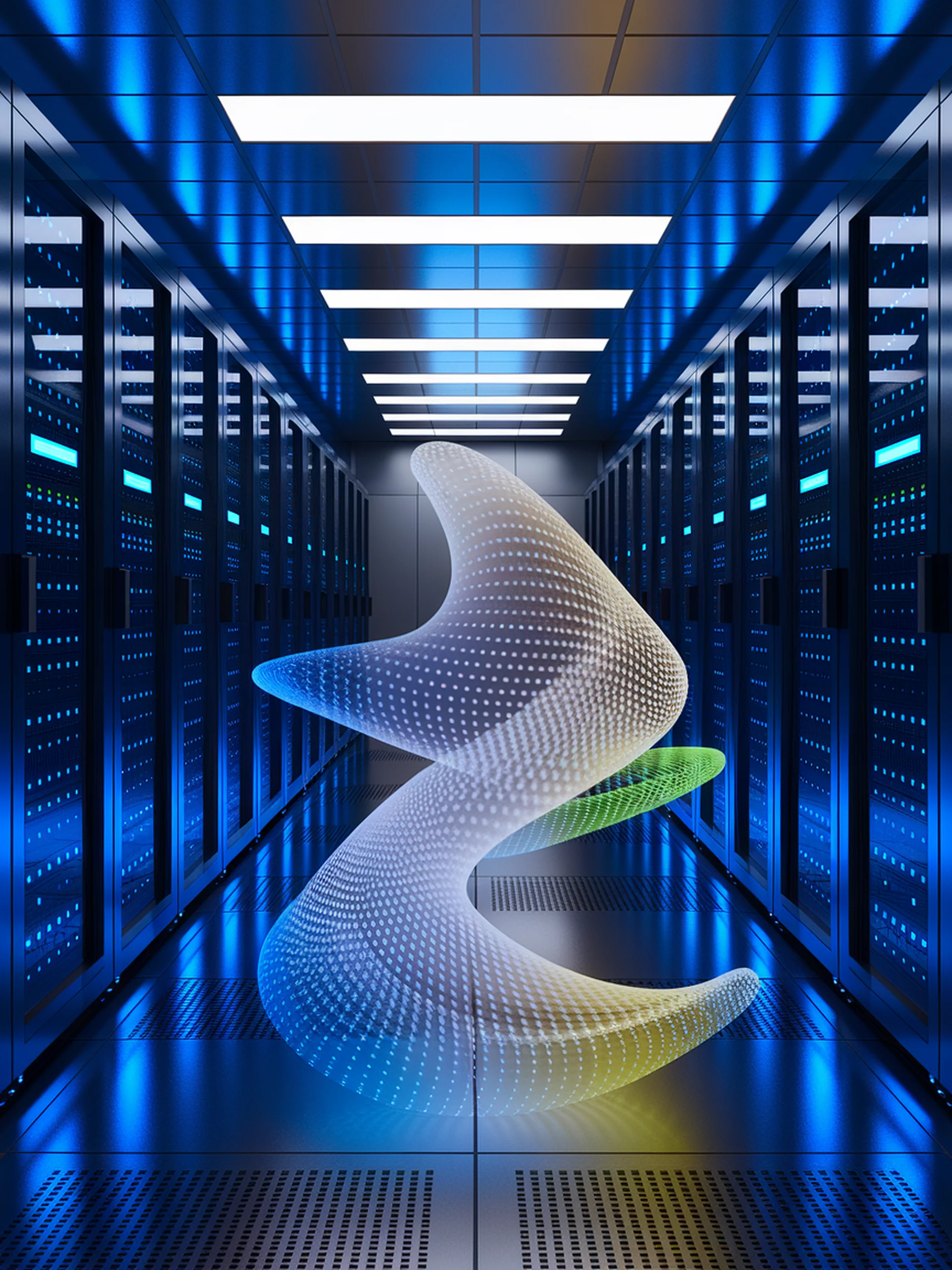
PolarQuant: Slashing Memory Costs in LLMs
A breakthrough approach to key cache quantization
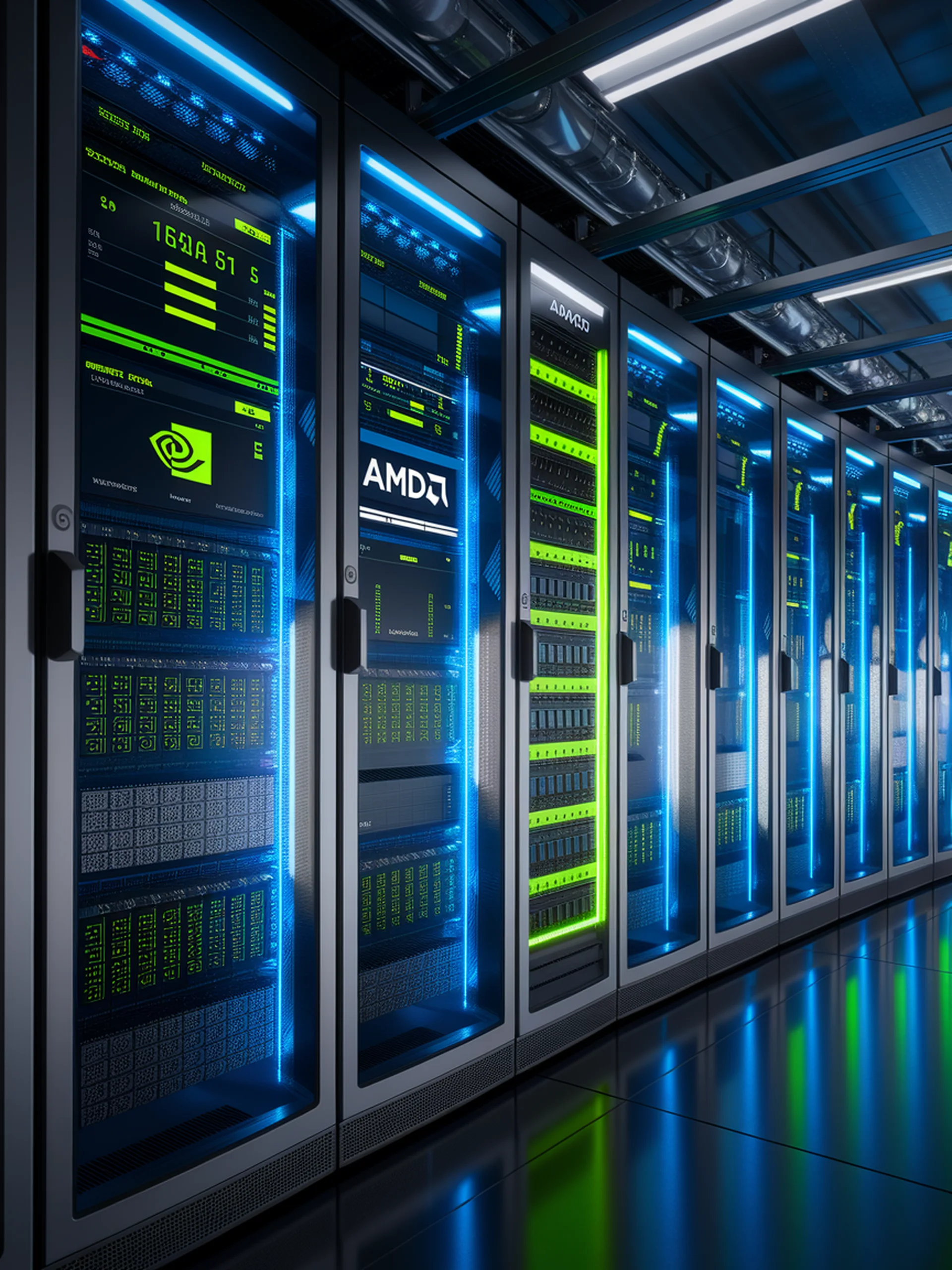
Optimizing LLM Costs with Heterogeneous GPUs
Improving cost-efficiency by matching LLM requests with appropriate GPU resources
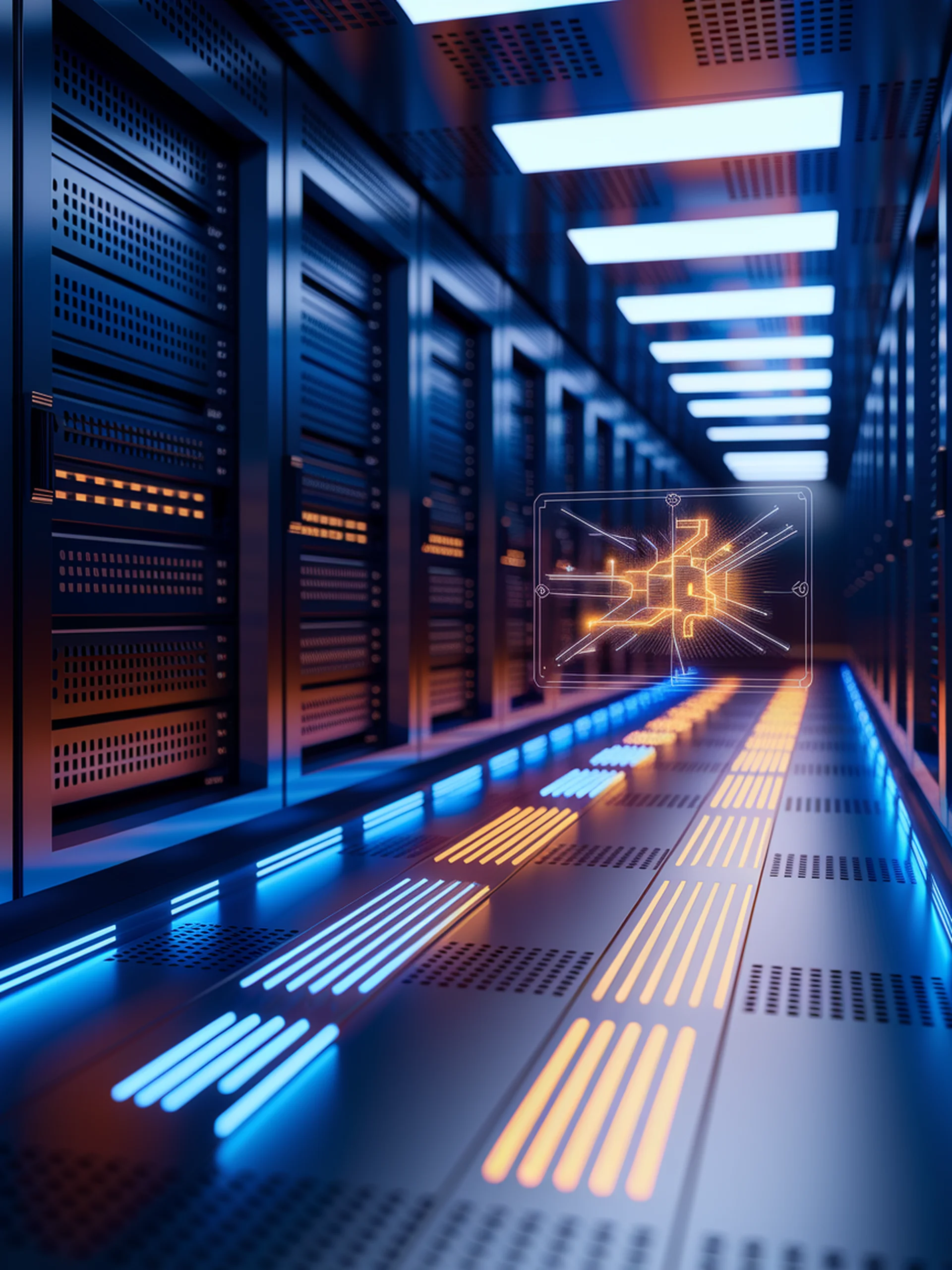
Boosting LLM Efficiency Through Smart Shortcuts
Reducing AI inference latency while maintaining quality
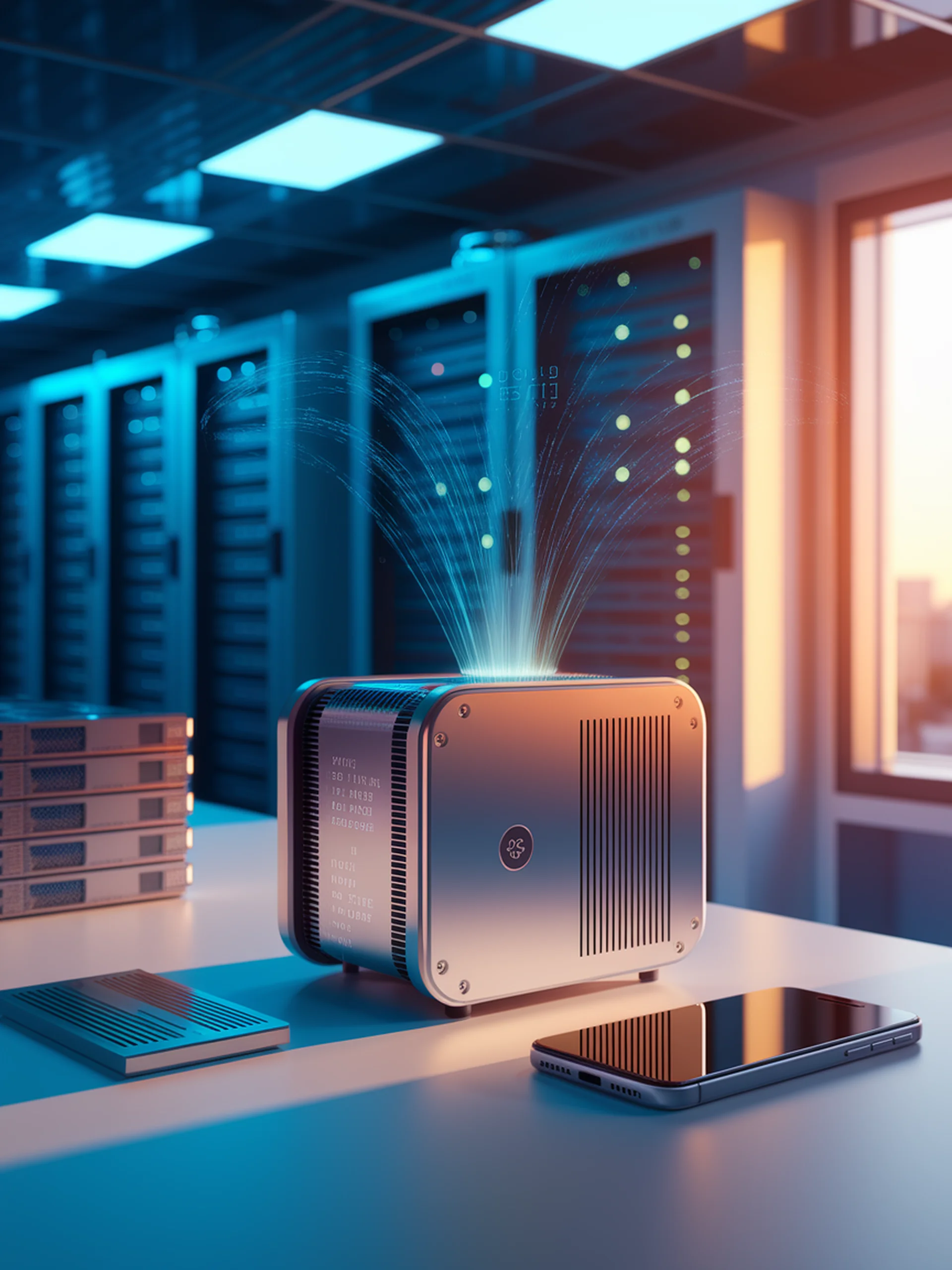
Lossless Compression for LLMs
Enabling efficient AI on edge devices without performance loss
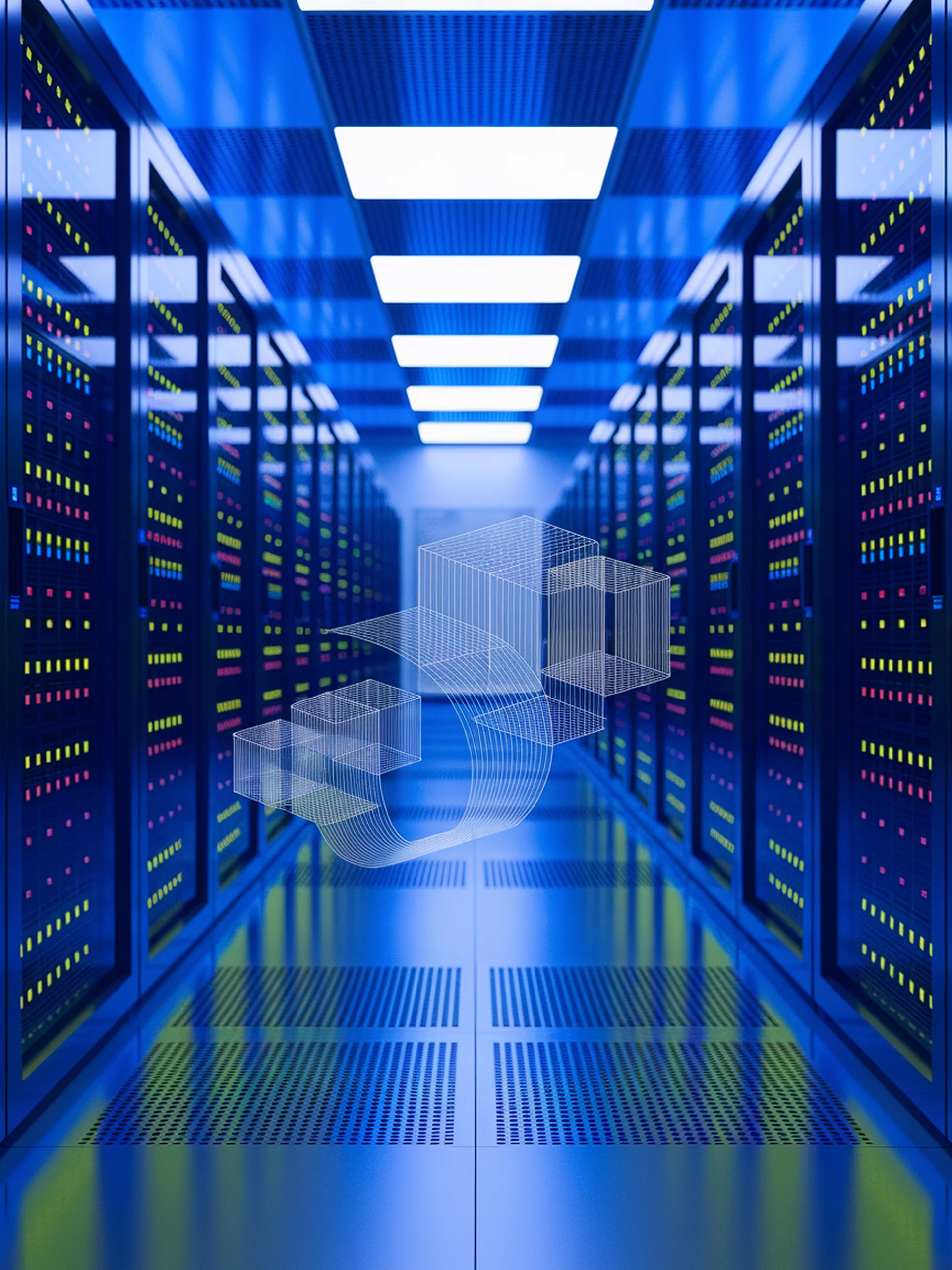
Accelerating Long-Context LLMs
FastKV: Optimizing Memory and Speed for Extended Context Processing
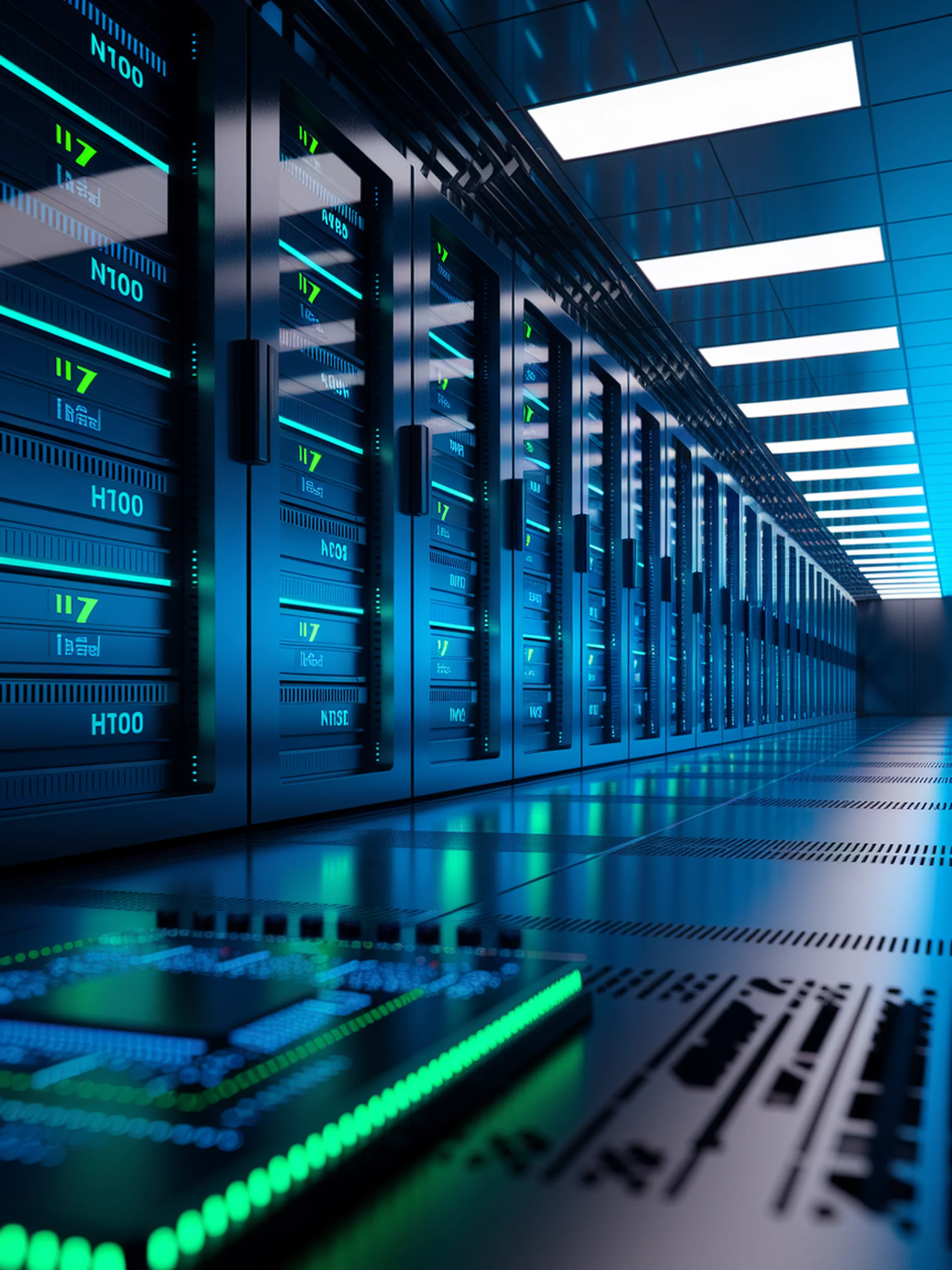
FP8 Precision for LLM Inference
Comparing implementation across NVIDIA and Intel accelerators
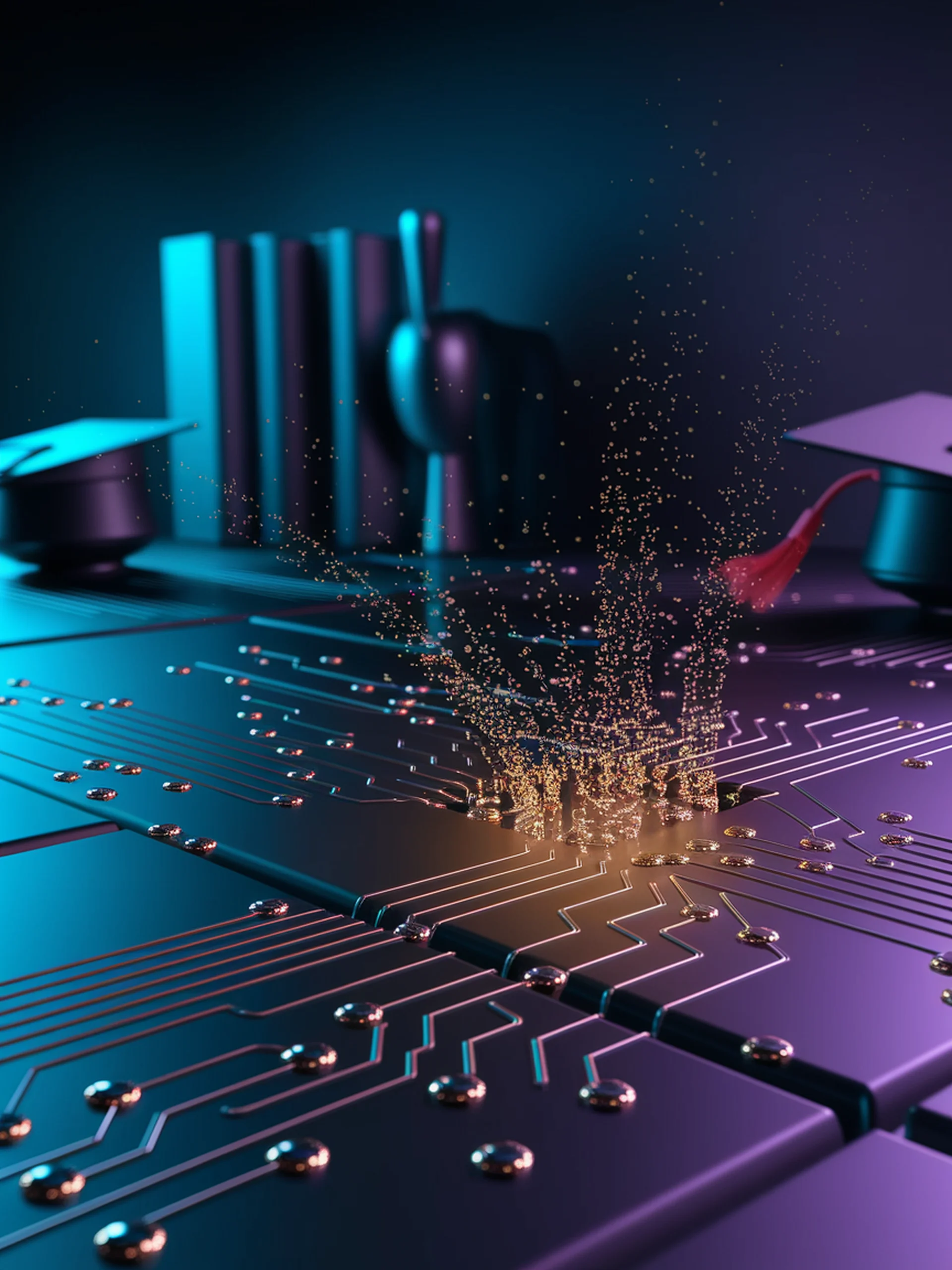
Smarter Weight Generation with Diffusion Models
Enhancing AI adaptability through trajectory-based learning
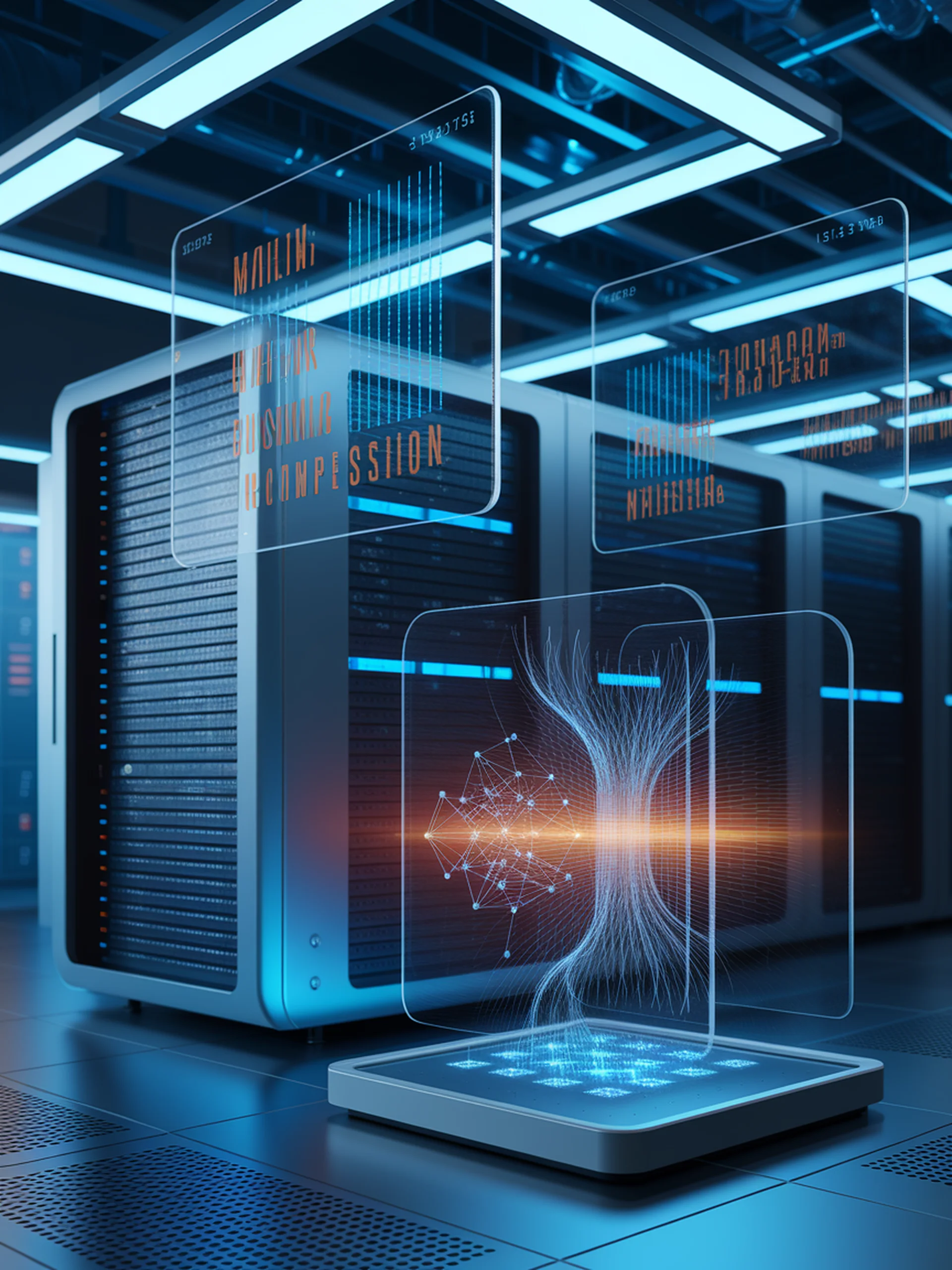
Smarter Model Compression for LLMs
Adaptive SVD: Enhancing compression while preserving performance
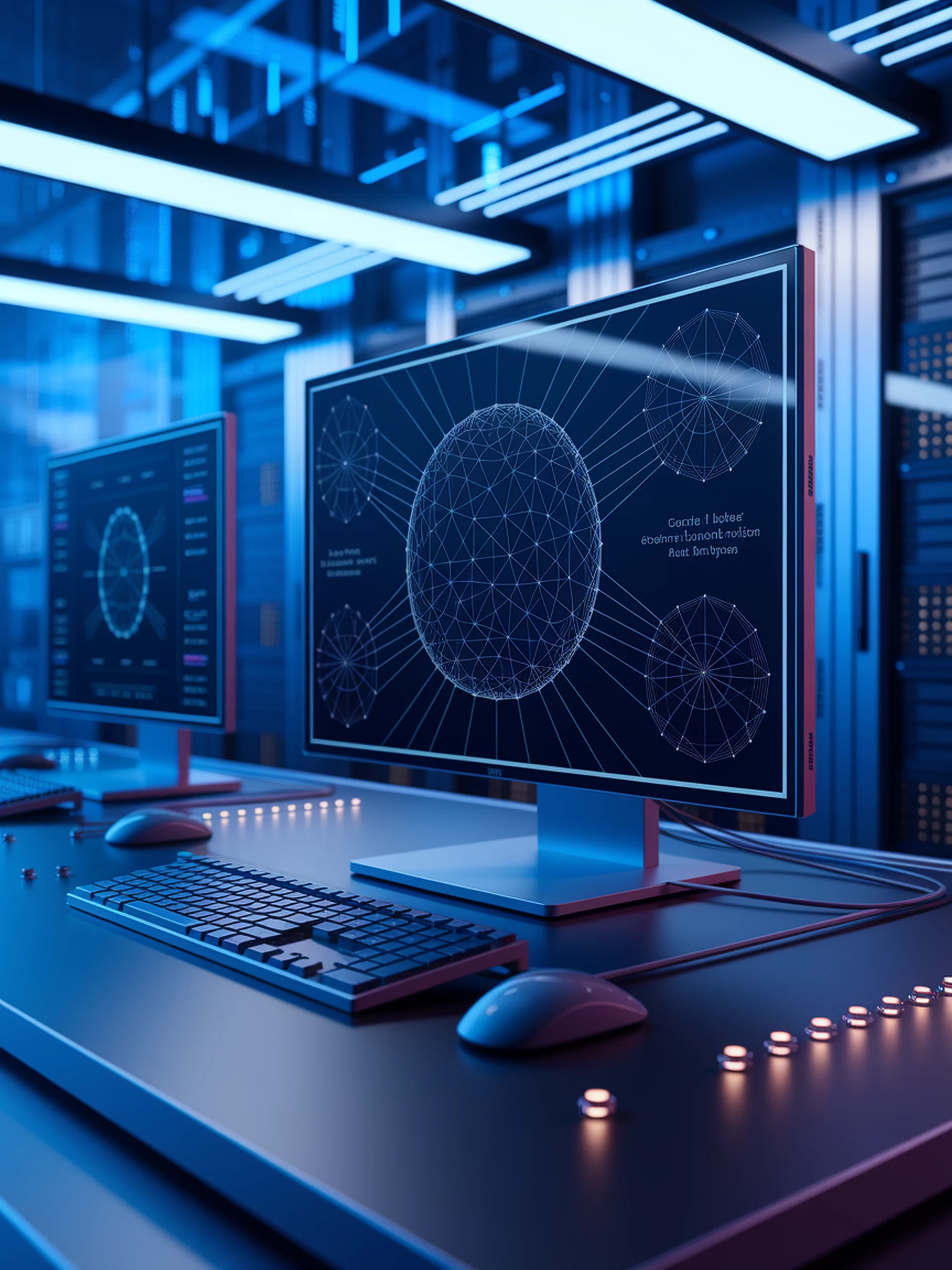
ReGLA: Refining Gated Linear Attention
By Peng Lu, Ivan Kobyzev...
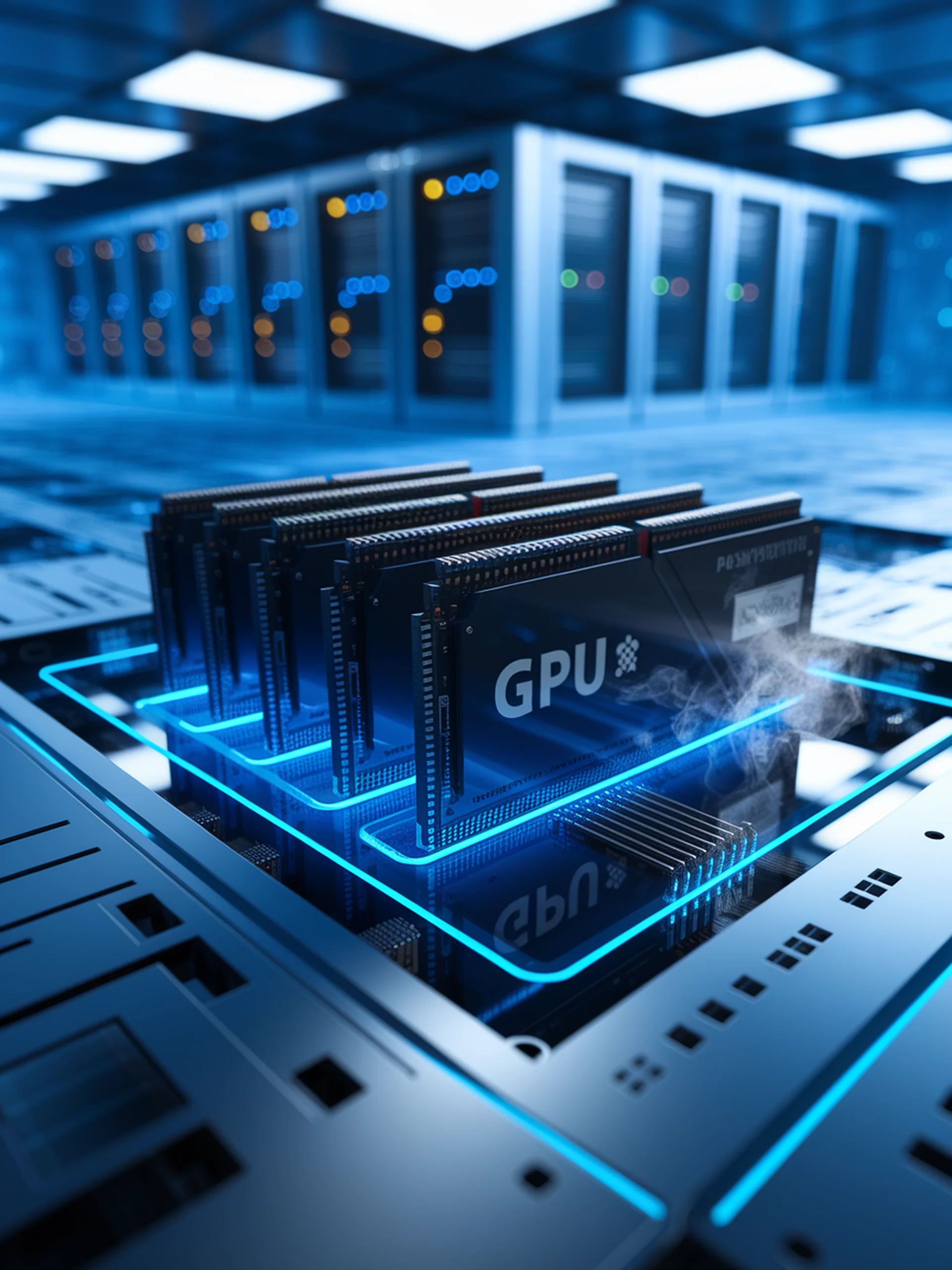
Memory-Efficient LLM Training
A novel approach to reduce computational costs without sacrificing performance
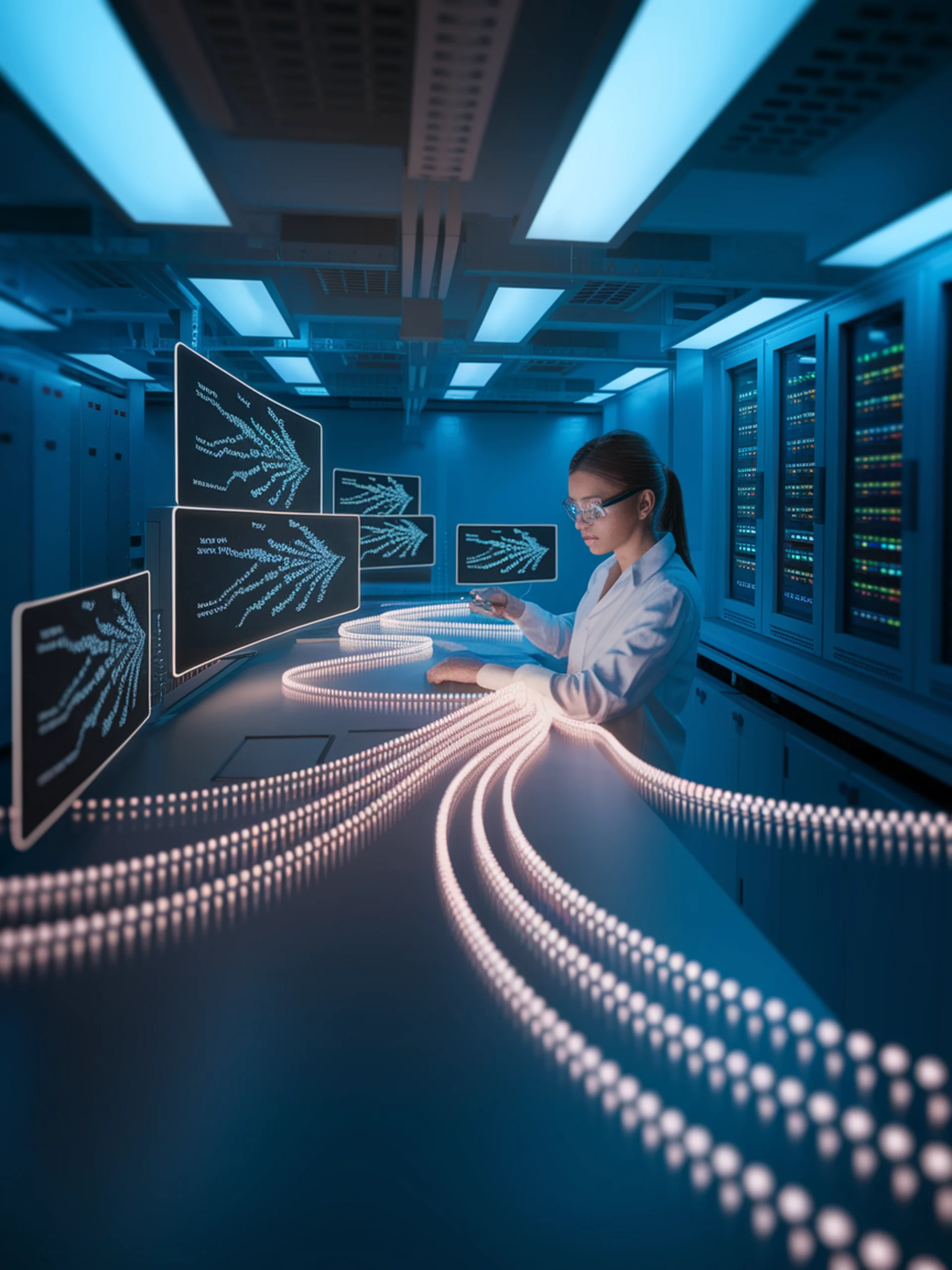
Rethinking LLM Scaling: Beyond Model Size
A probabilistic approach to inference-time optimization using Monte Carlo methods

Optimizing LLaMA 2 Inference Across Languages
Comparative performance analysis of programming frameworks for LLM efficiency

Breaking Transformer's Length Barriers
Overcoming Context Limitations with Sparse Graph Processing
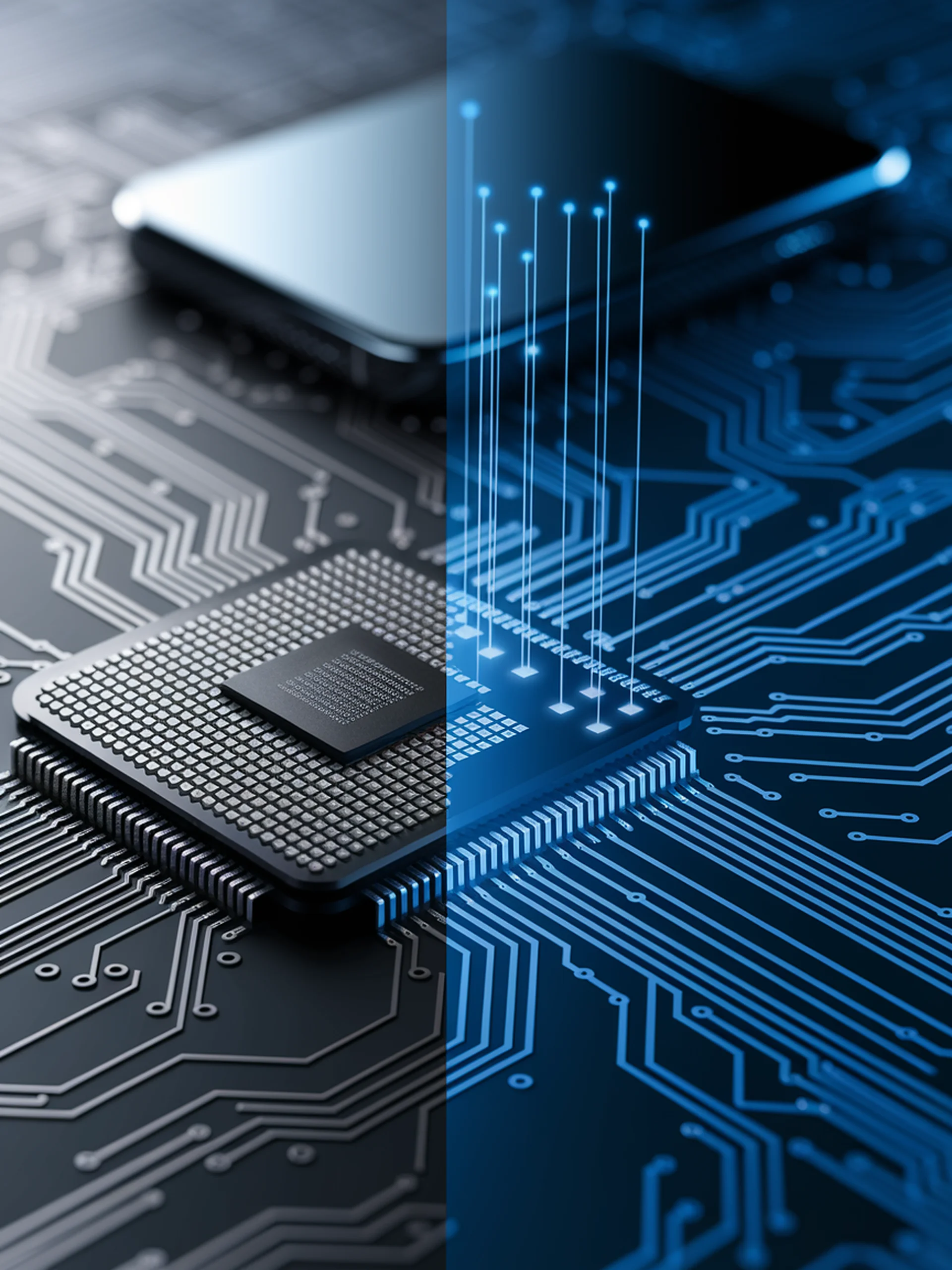
Optimizing LLMs for Resource-Constrained Devices
A novel approach combining binarization with semi-structured pruning
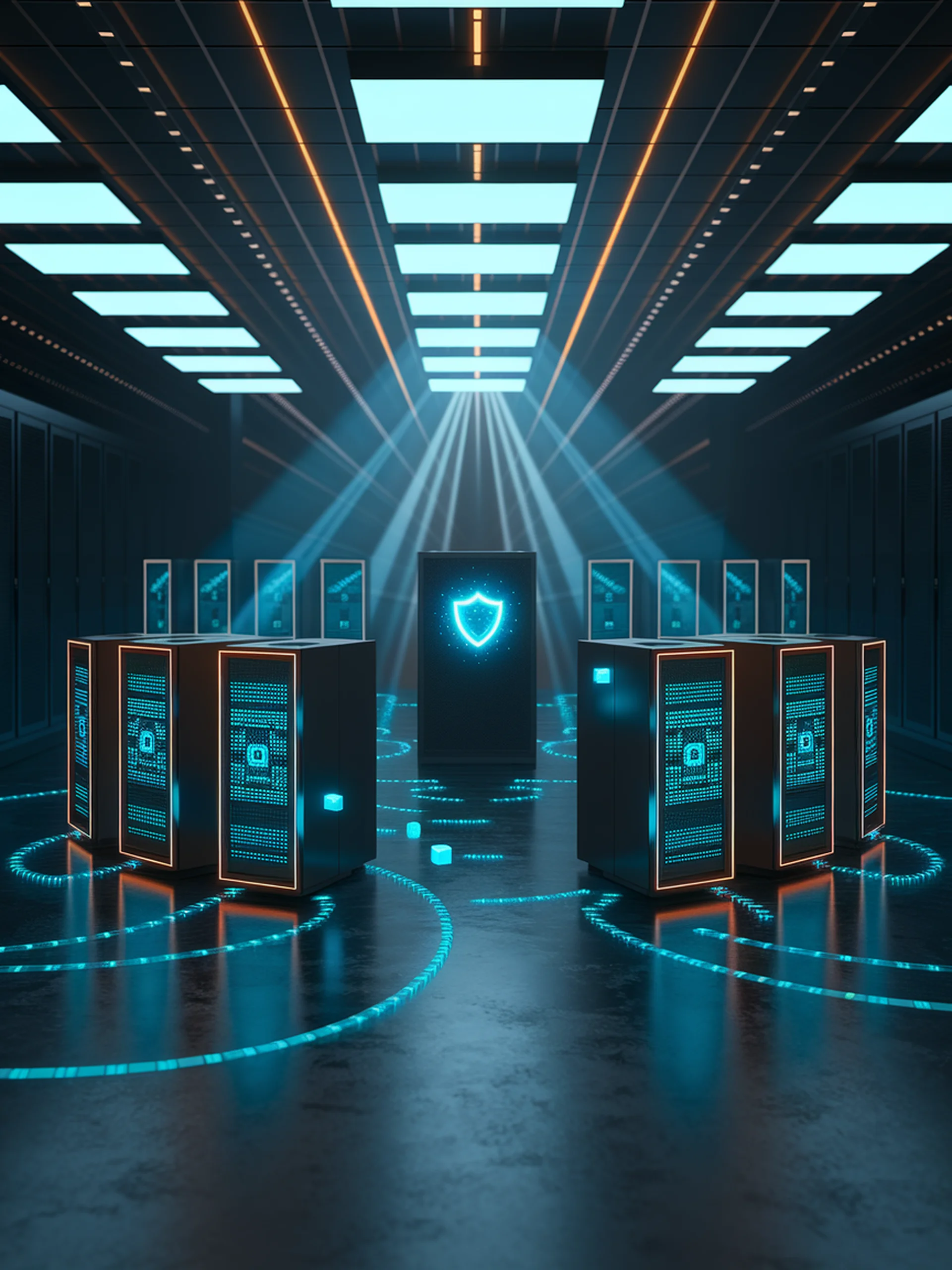
RoLoRA: Making Federated LLM Fine-tuning More Robust
Alternating optimization approach for secure collaborative model training
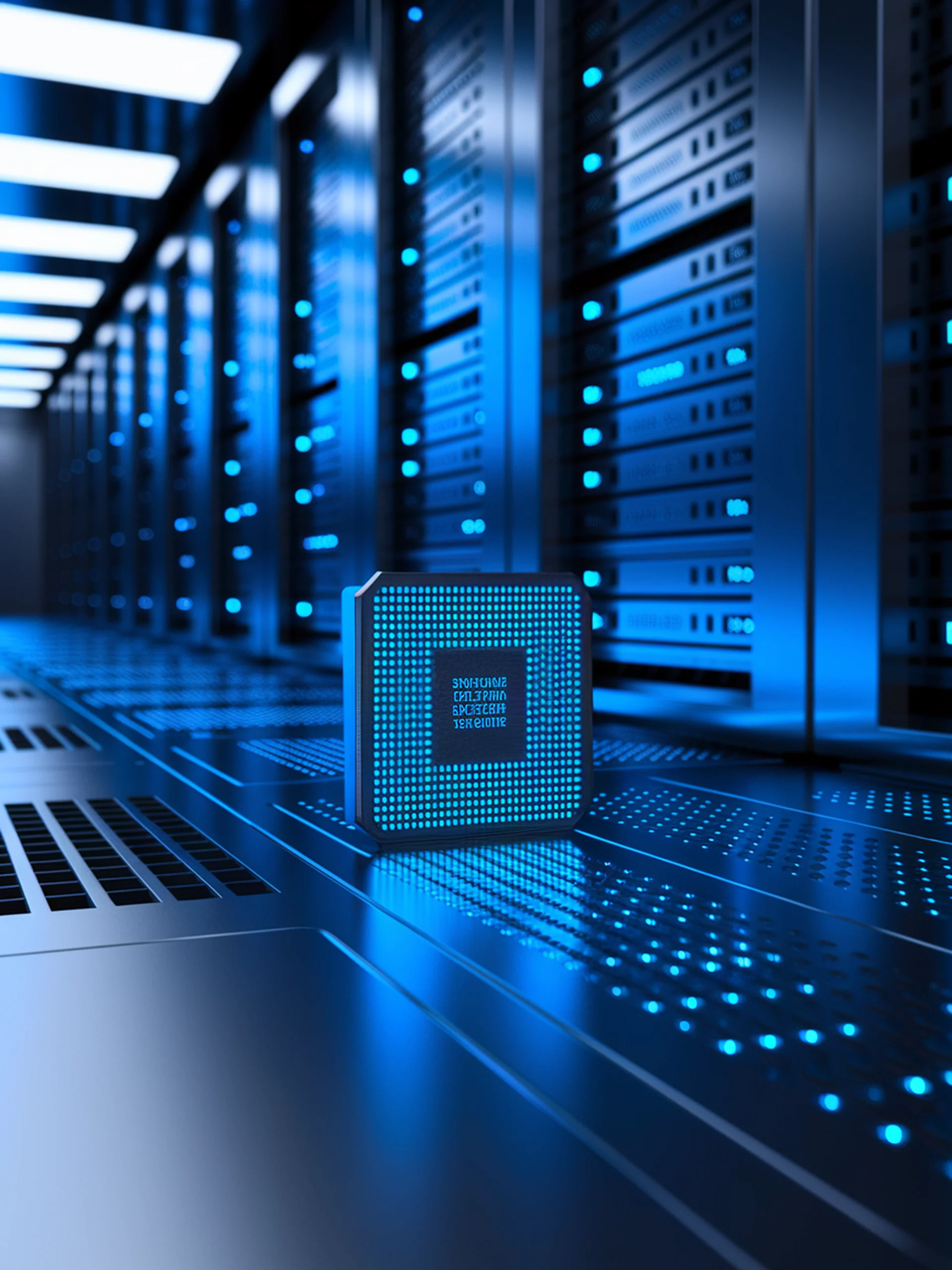
One-Bit Revolution for AI Efficiency
How one-bit unrolling transforms Large Inference Models
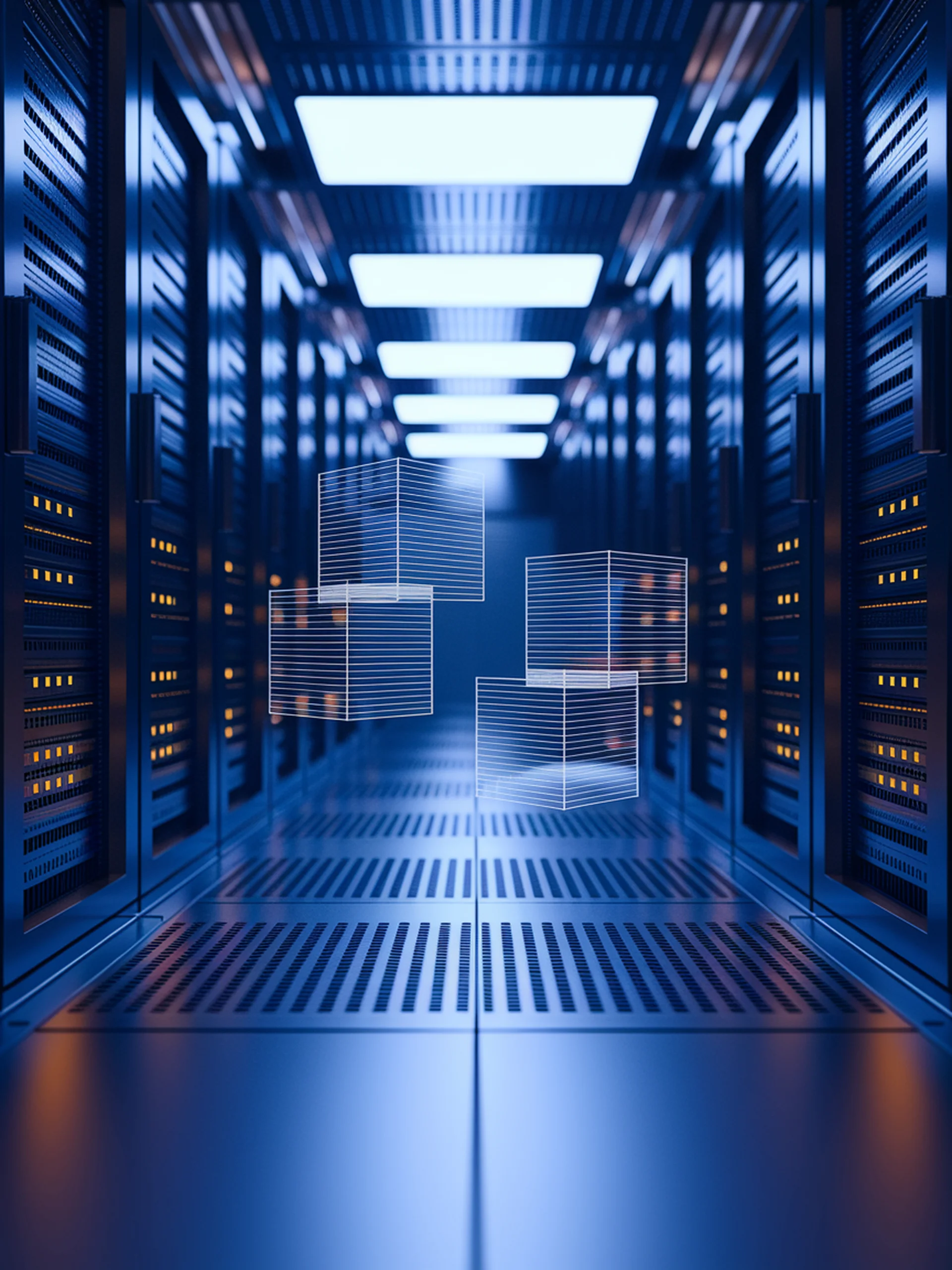
Optimizing LLMs without Sacrificing Core Abilities
Preserving model quality during KV cache compression

Smarter Caching for Multimodal AI
Position-Independent Caching for Efficient MLLM Serving

Accelerating LLM Inference
Smart collaboration between large and small models

Breaking the Context Barrier
Wavelet-Based Approach for Extended Language Model Contexts
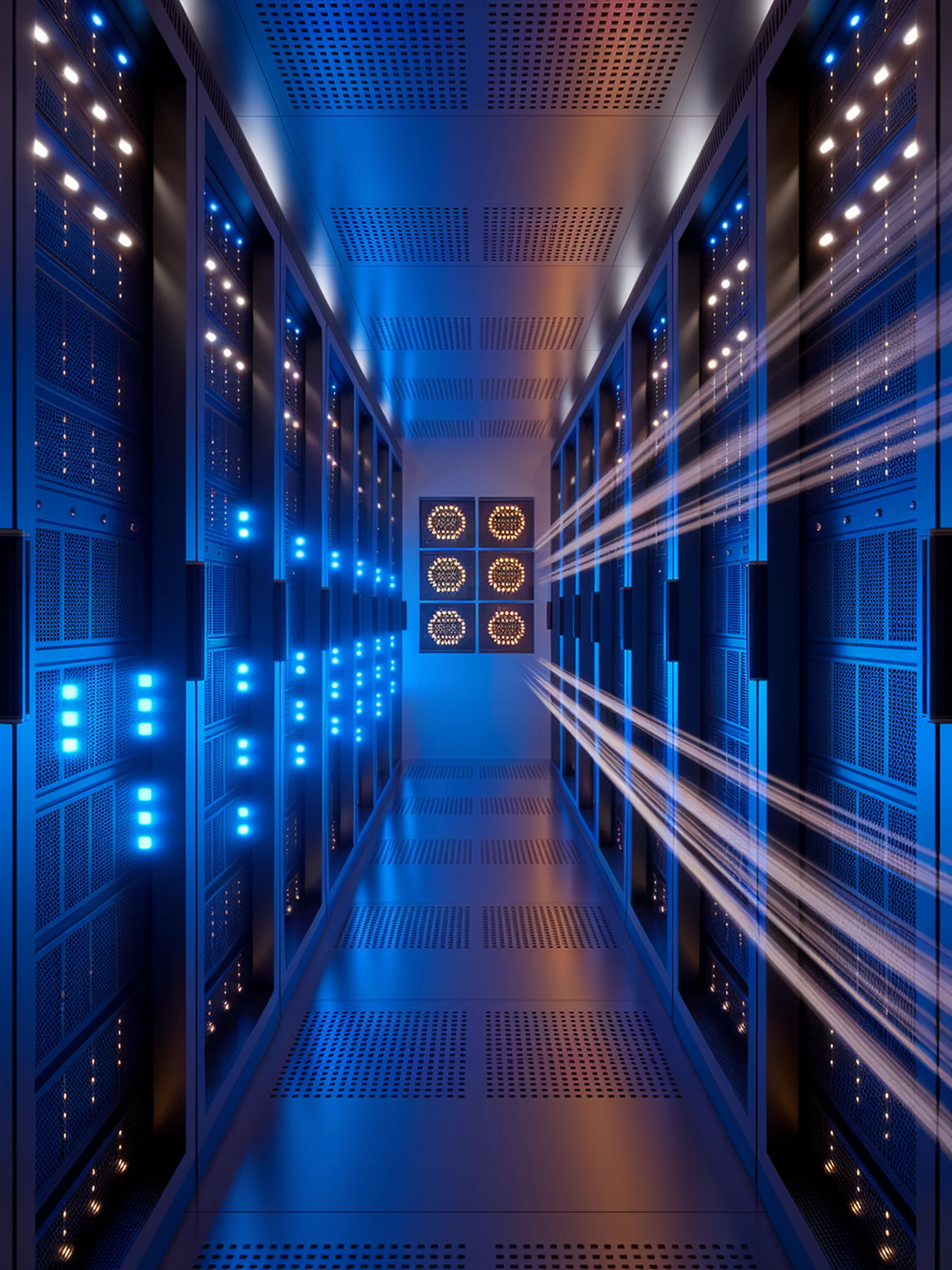
Optimizing LLM Inference with Smart Residuals
Adaptive multi-rate processing for faster, more efficient generation
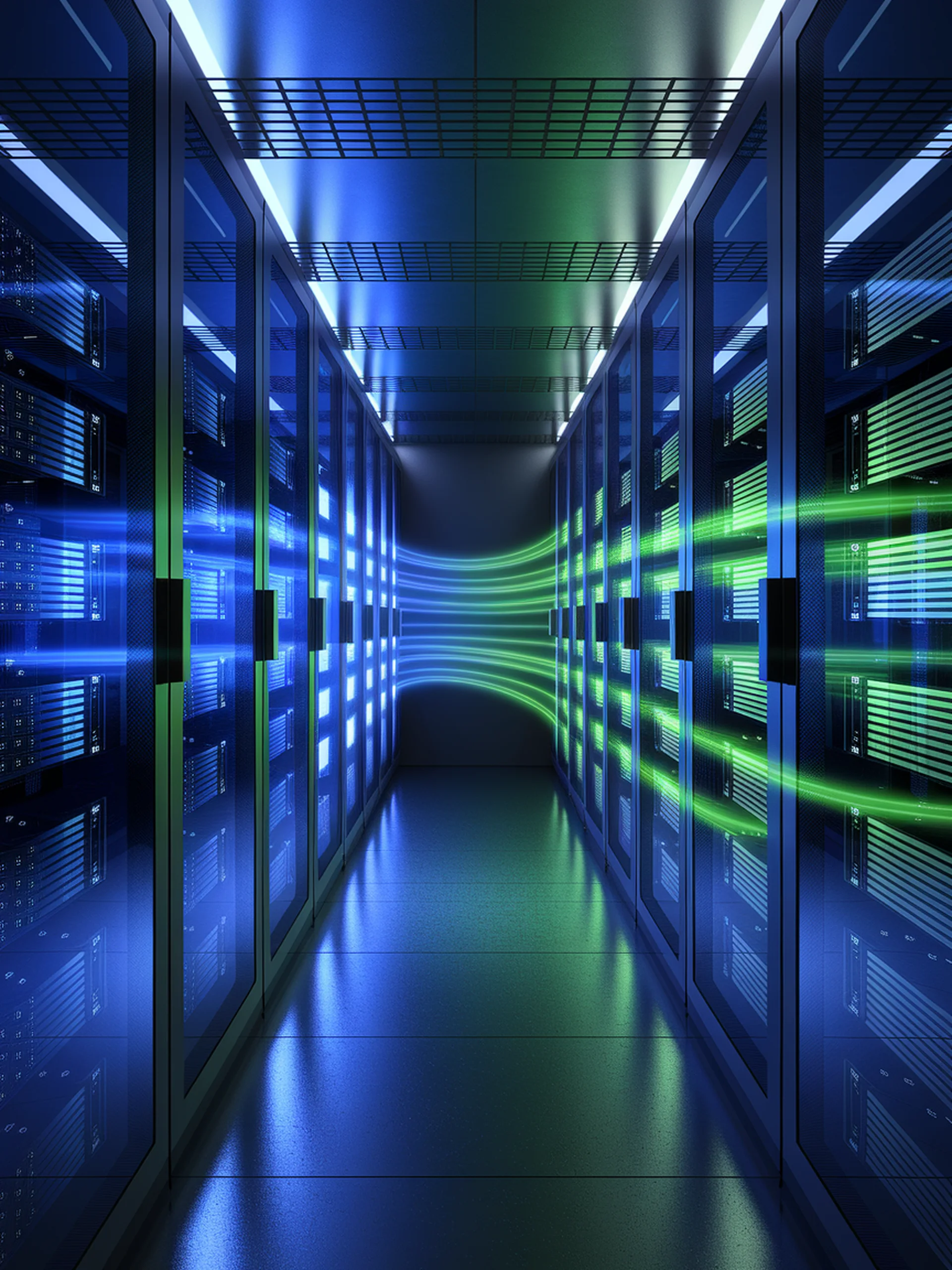
Maximizing GPU Efficiency in LLM Inference
A Layer-Parallel Approach to Speculative Decoding
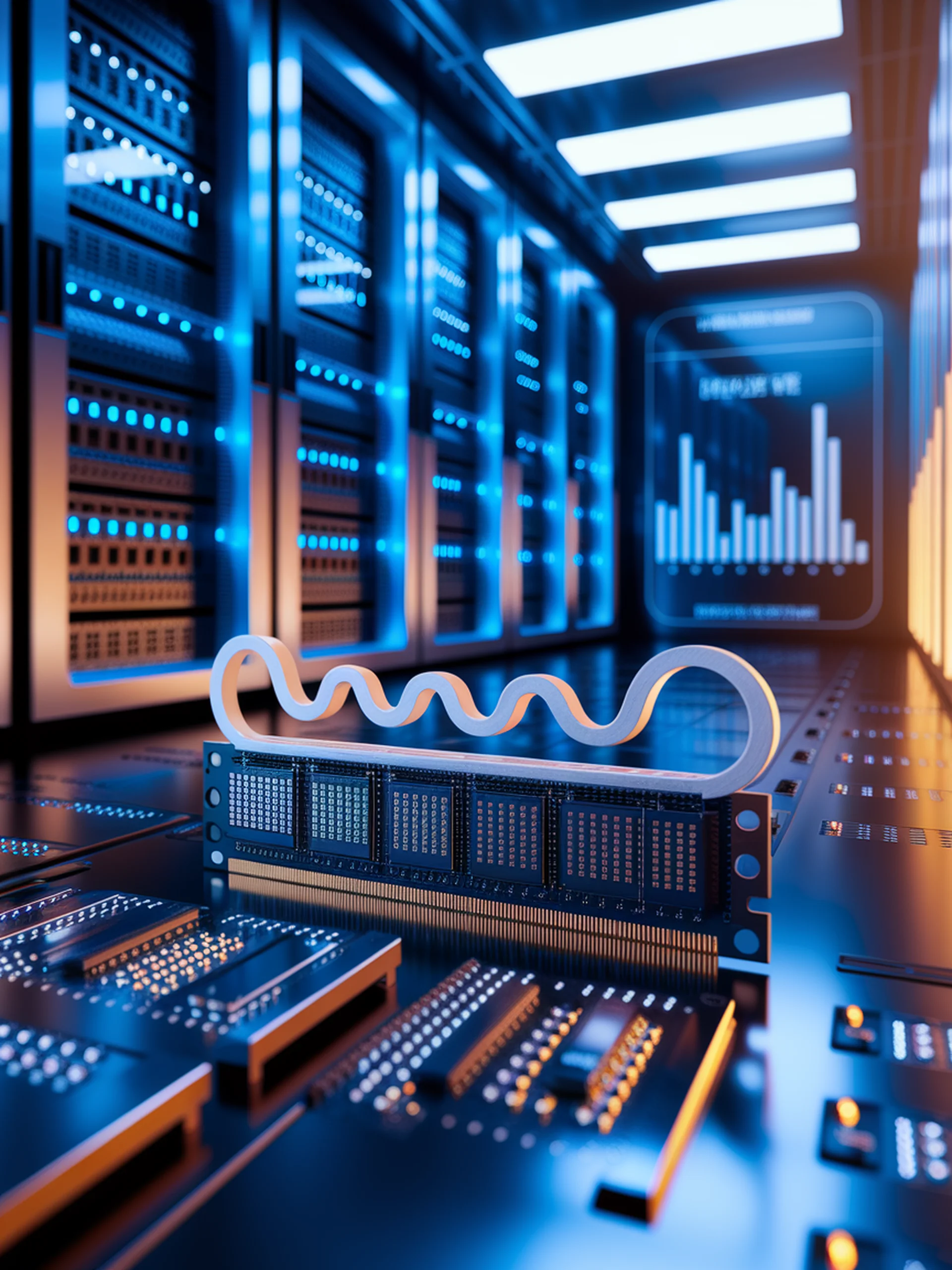
Optimizing Memory for LLMs
PolarQuant: A Novel Approach to KV Cache Compression
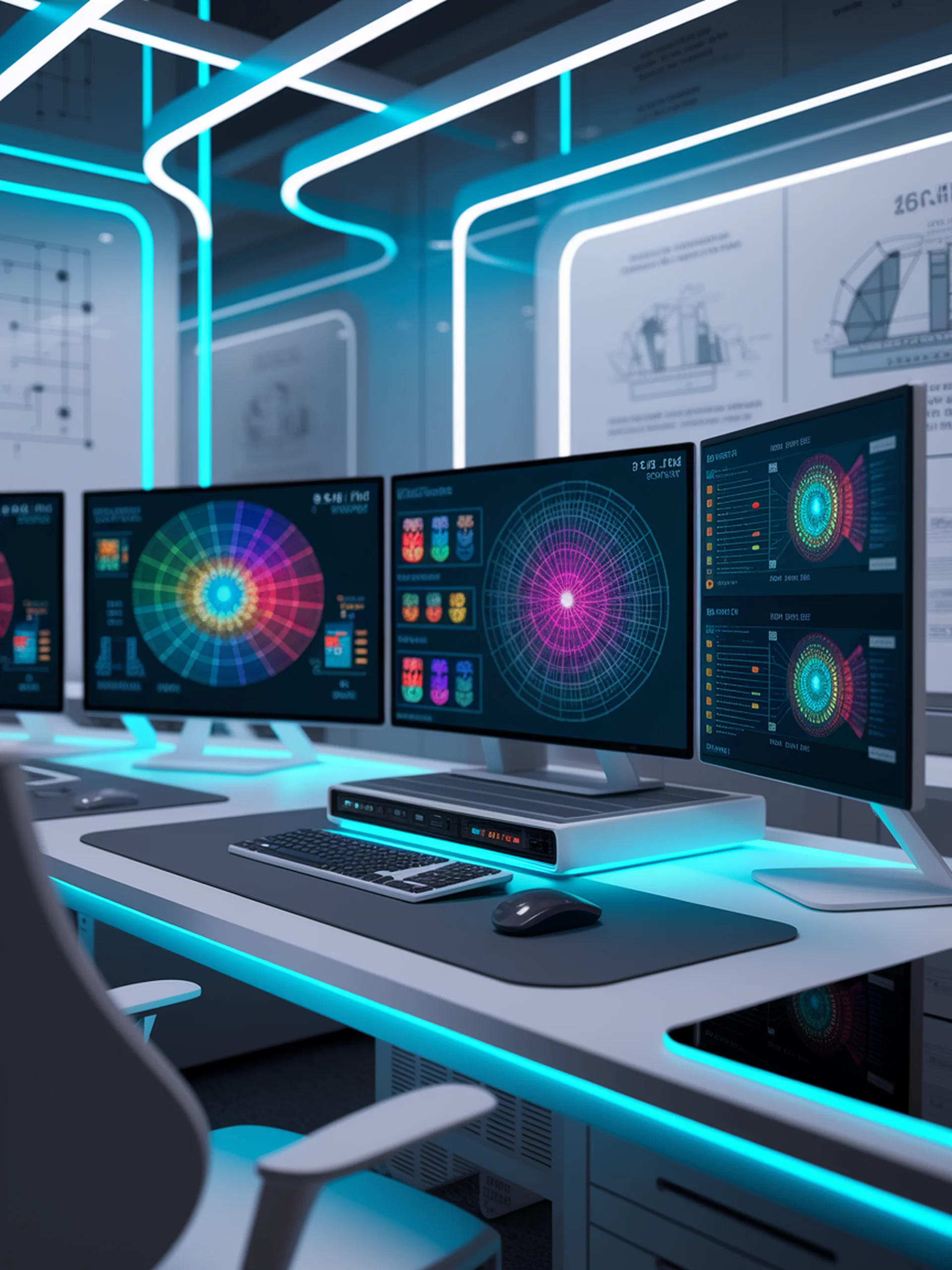
Rethinking Layer Normalization in Transformers
A new approach to improve LLM training stability and efficiency
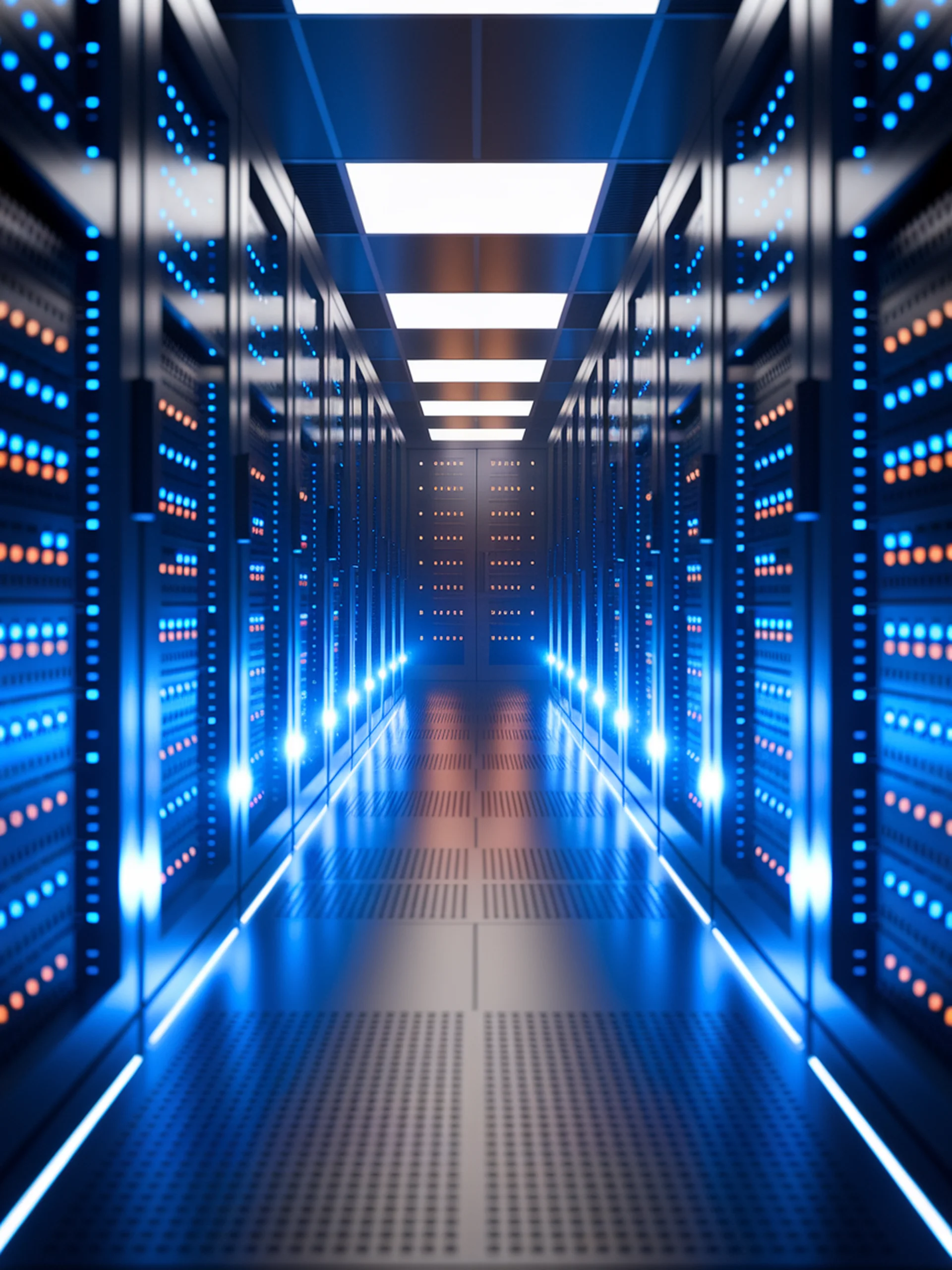
Adaptive Attention Sparsity for LLMs
Dynamic efficiency for long-context language models
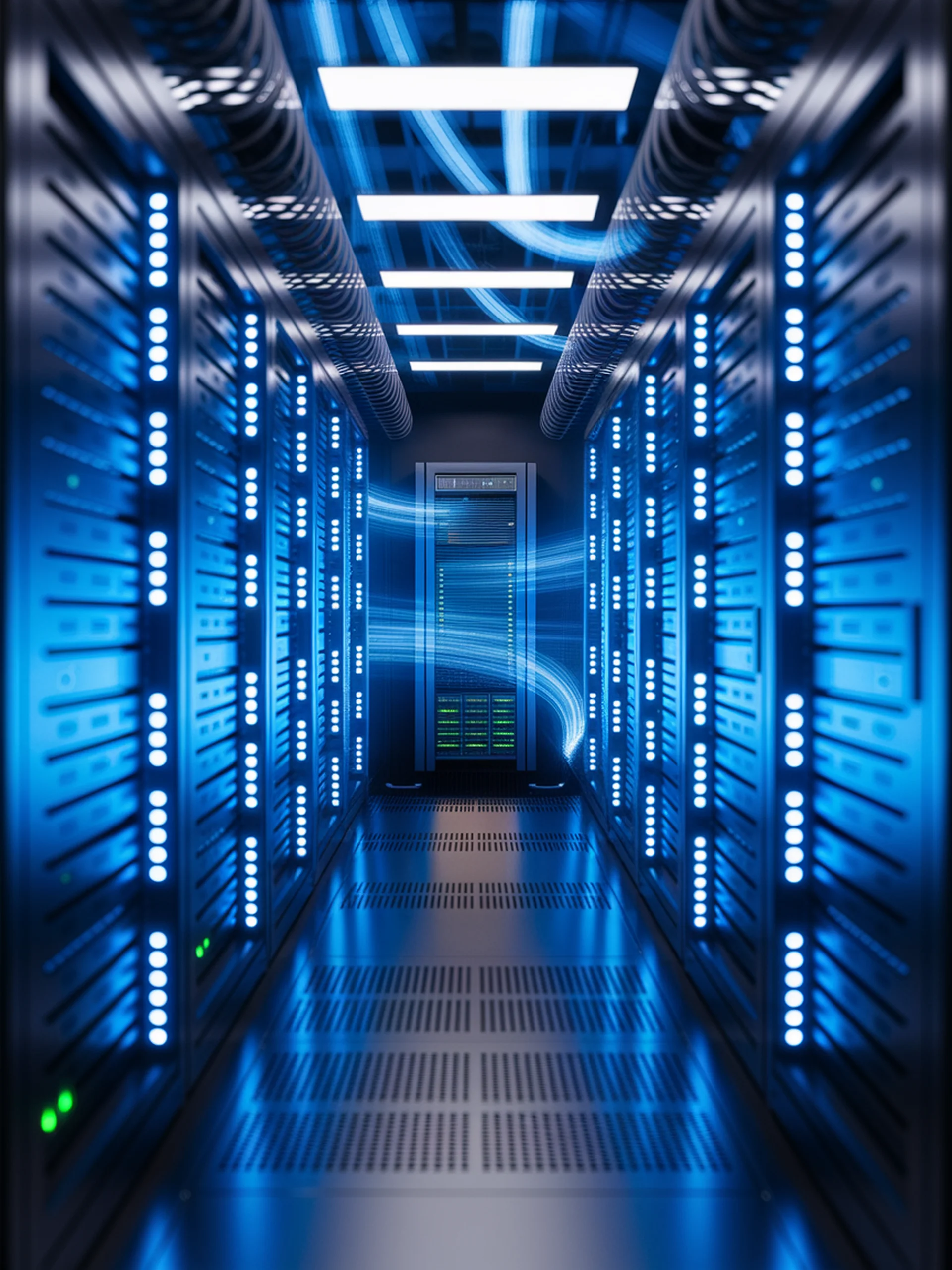
Accelerating LLM Response Times
Speeding Up First Token Generation Without Additional Training
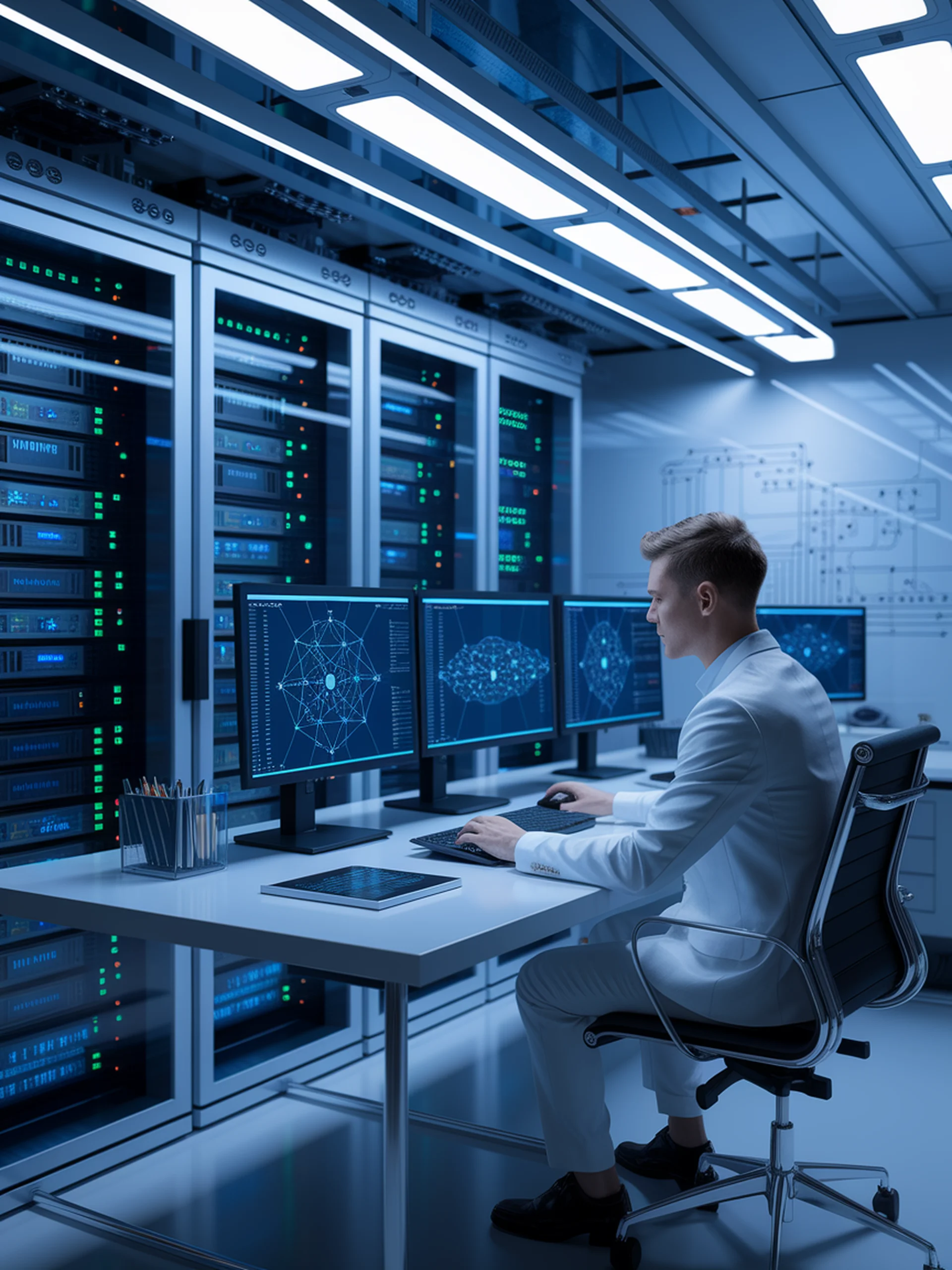
Streamlining LLMs for Efficient Inference
Reducing model depth without sacrificing performance
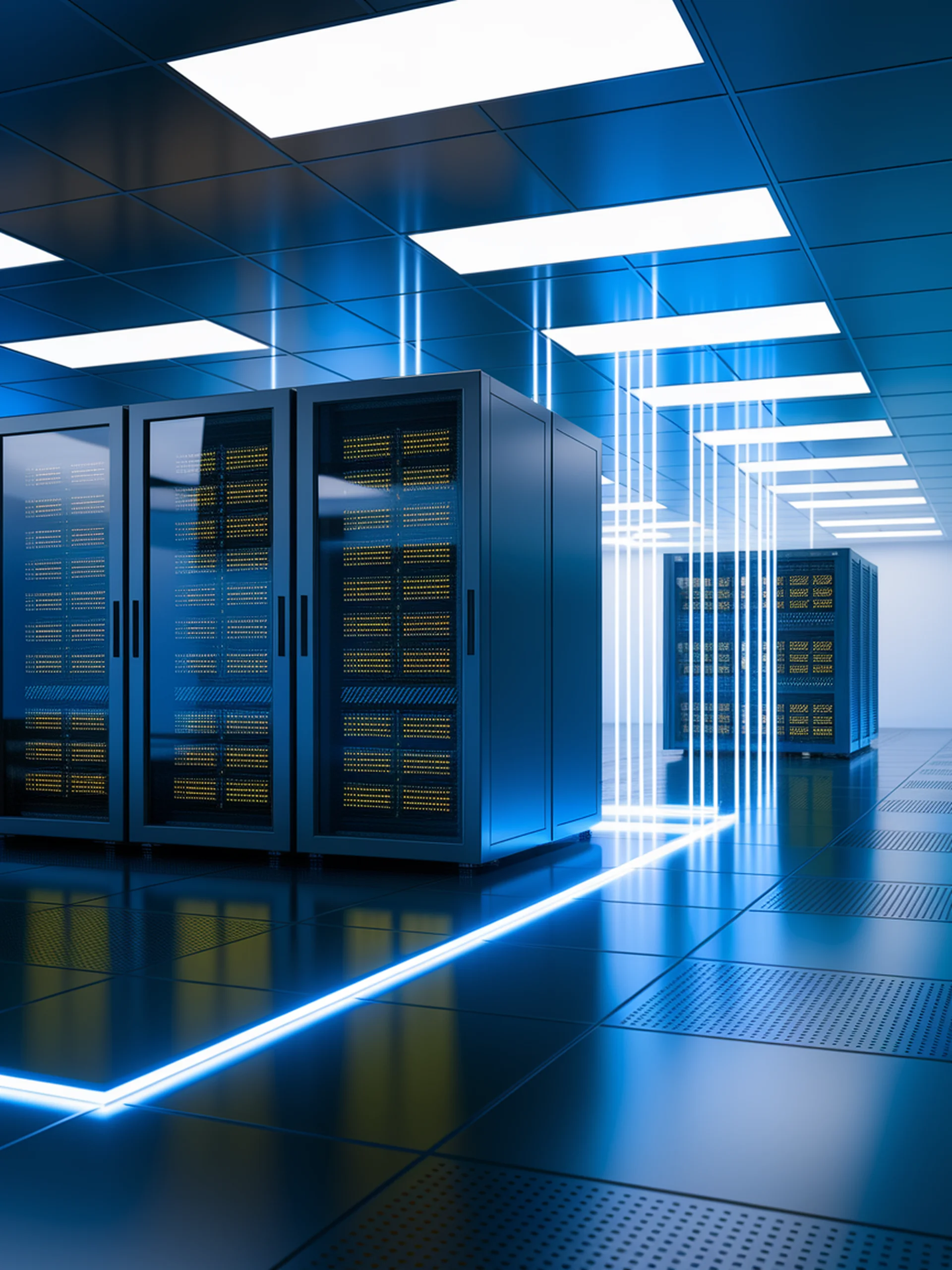
SQL-Powered LLMs: Democratizing AI Deployment
Running AI models through standard database systems
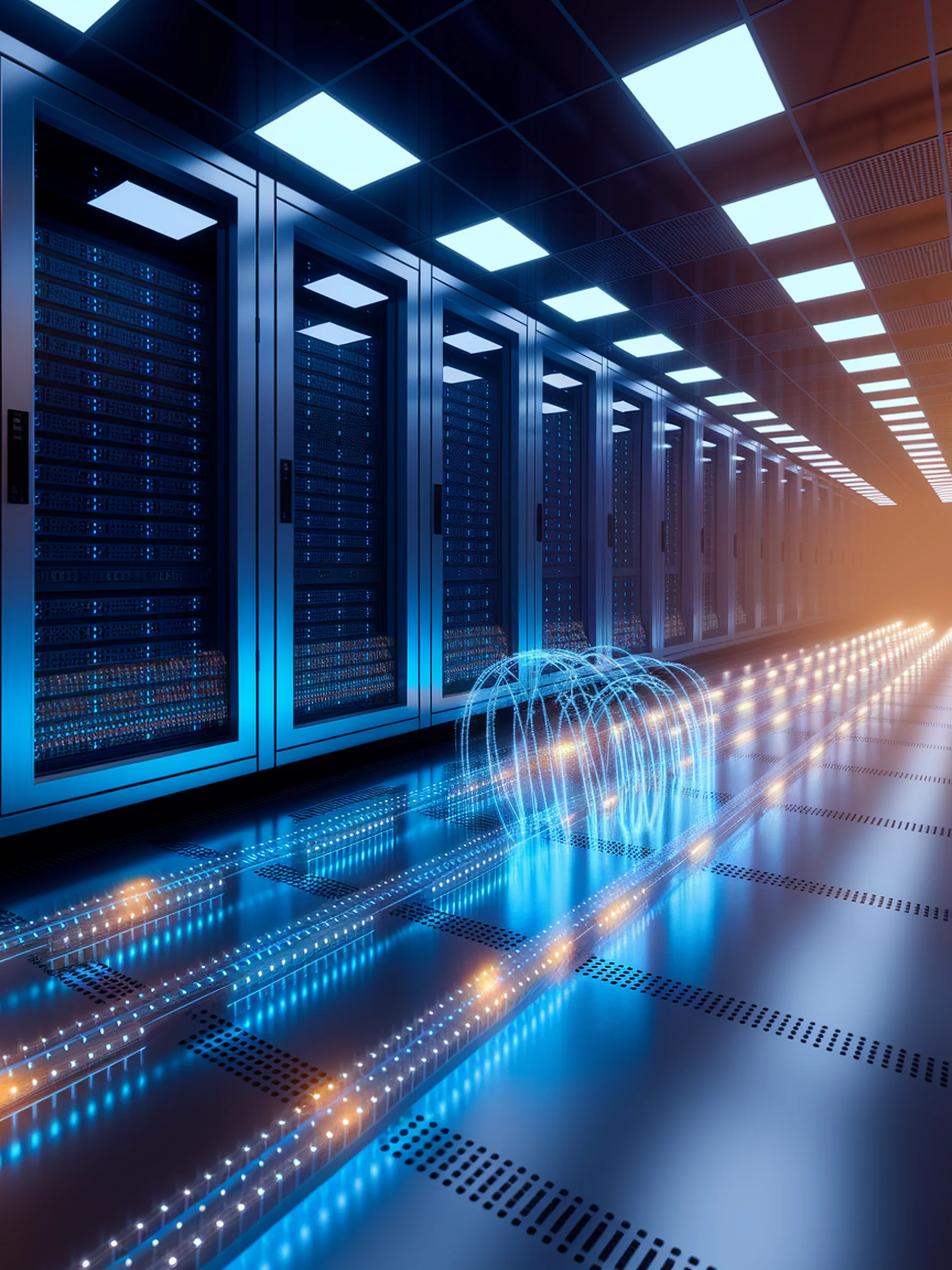
Smarter Memory Management in LLMs
Dynamic token selection for enhanced sequence processing
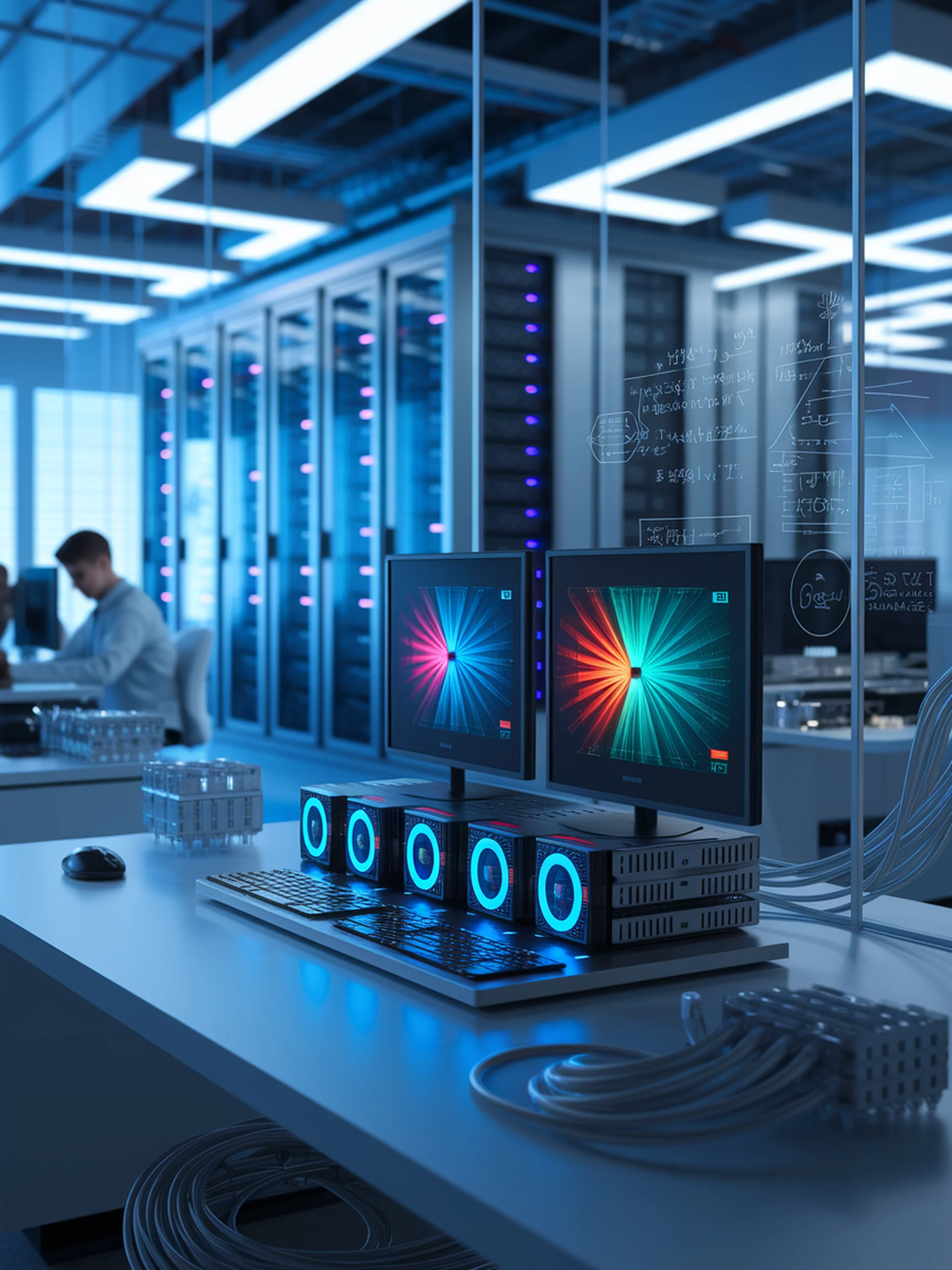
Memory-Efficient LLM Fine-Tuning Breakthrough
Accelerating Zero-Order Optimization for Real-World Deployment
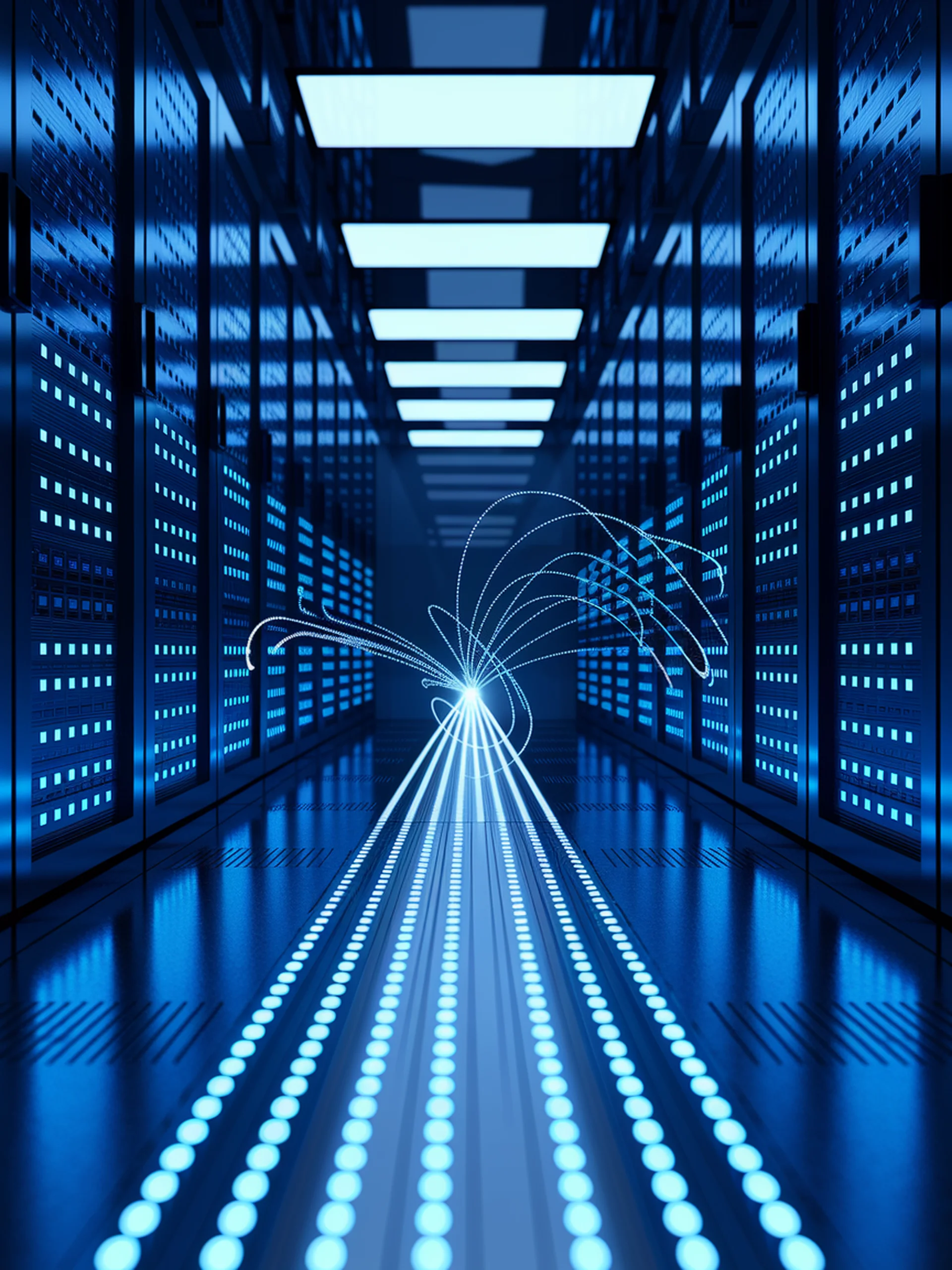
Accelerating LLM Inference Through Smart Compression
Boosting performance with innovative Key-Value cache optimization

Optimizing LLM Efficiency Through Smarter KV Cache
A formal approach to reducing memory and computational costs in large language models
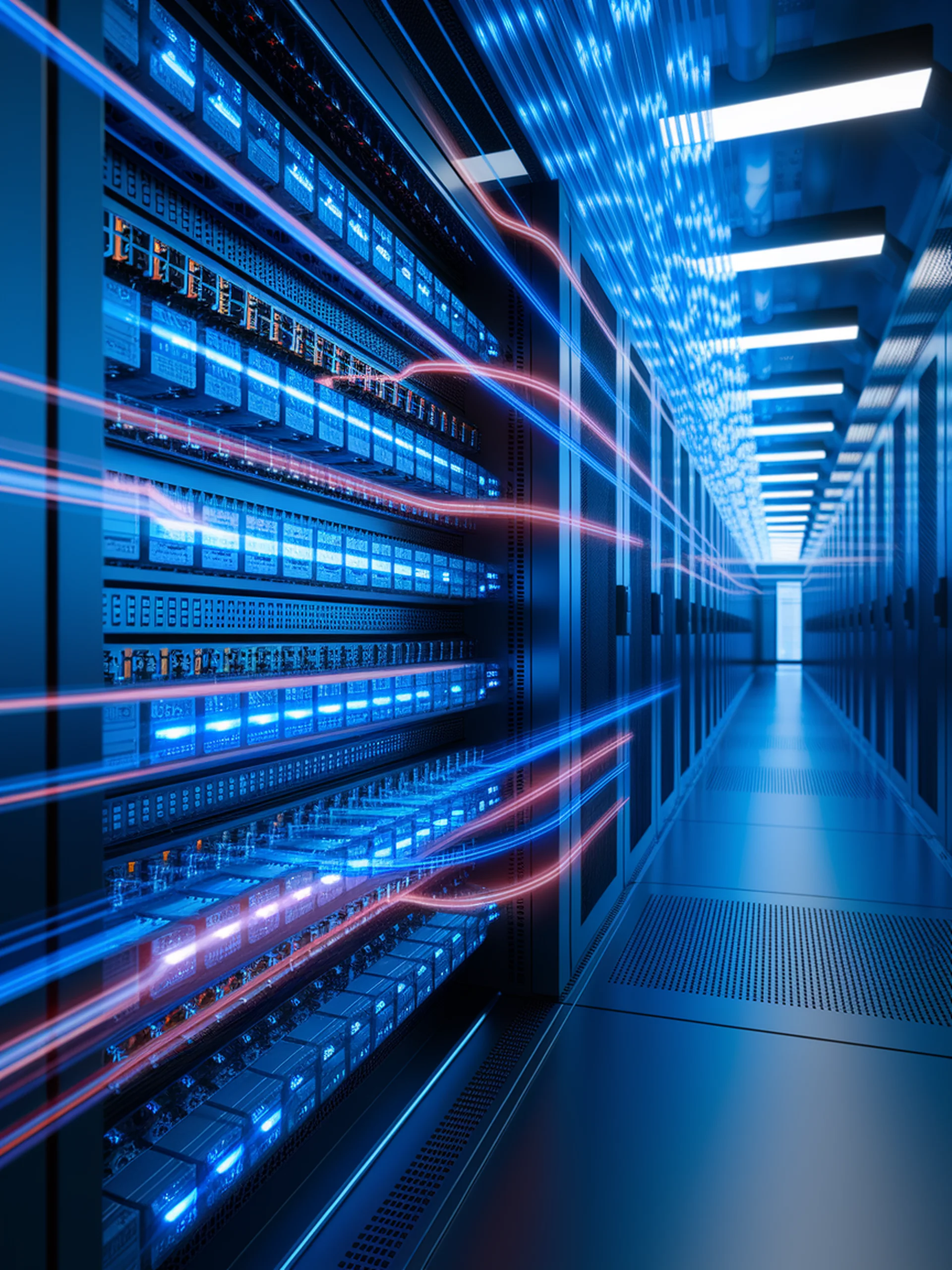
Revolutionizing AI Infrastructure with Optical Networks
A scalable, cost-effective architecture for large language model training
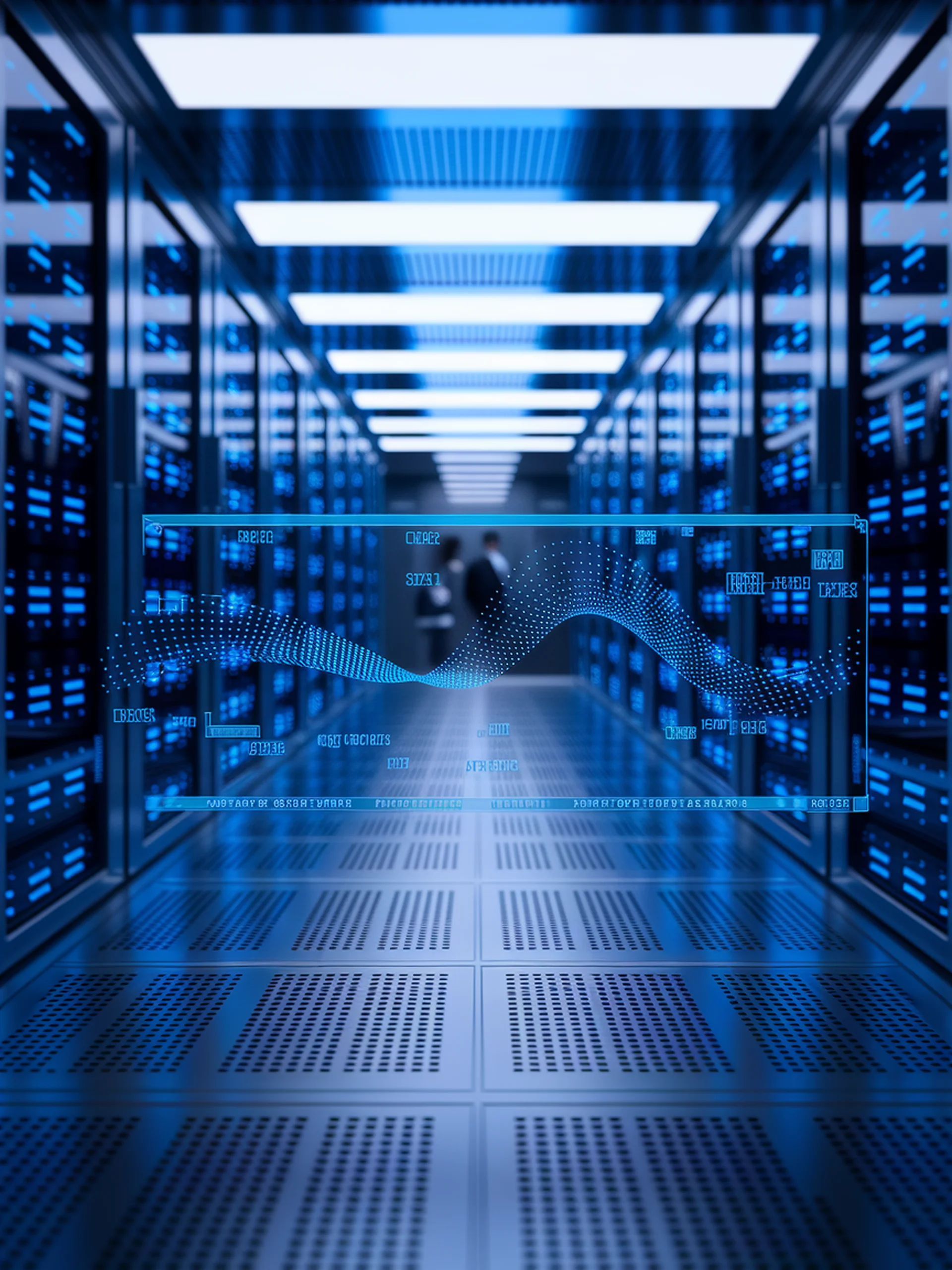
Smarter KV Cache Compression for LLMs
Leveraging Temporal Attention Patterns for Efficient Inference
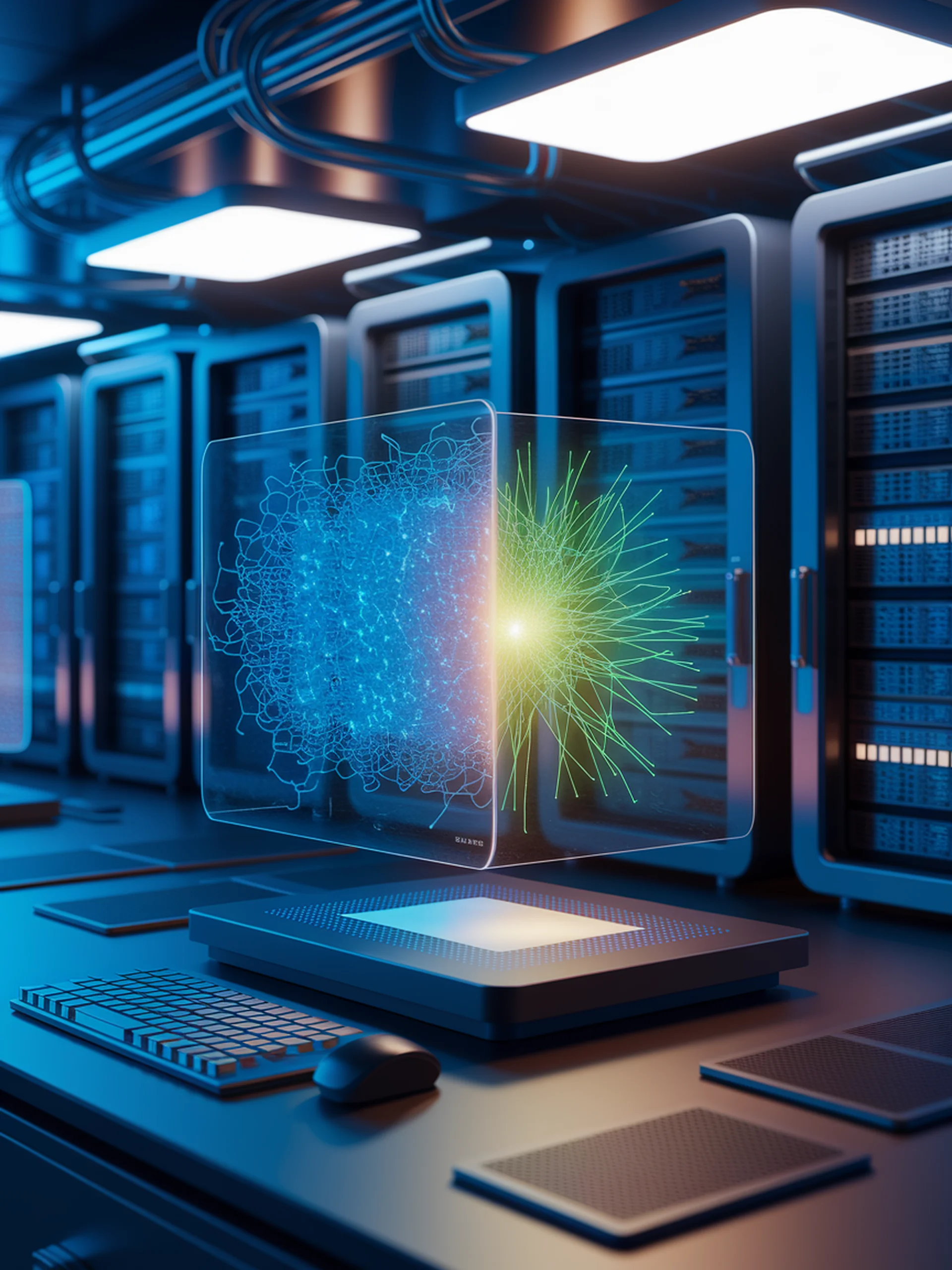
Accelerating Spiking Neural Networks for LLMs
A two-stage conversion approach that maintains performance and reduces computational costs
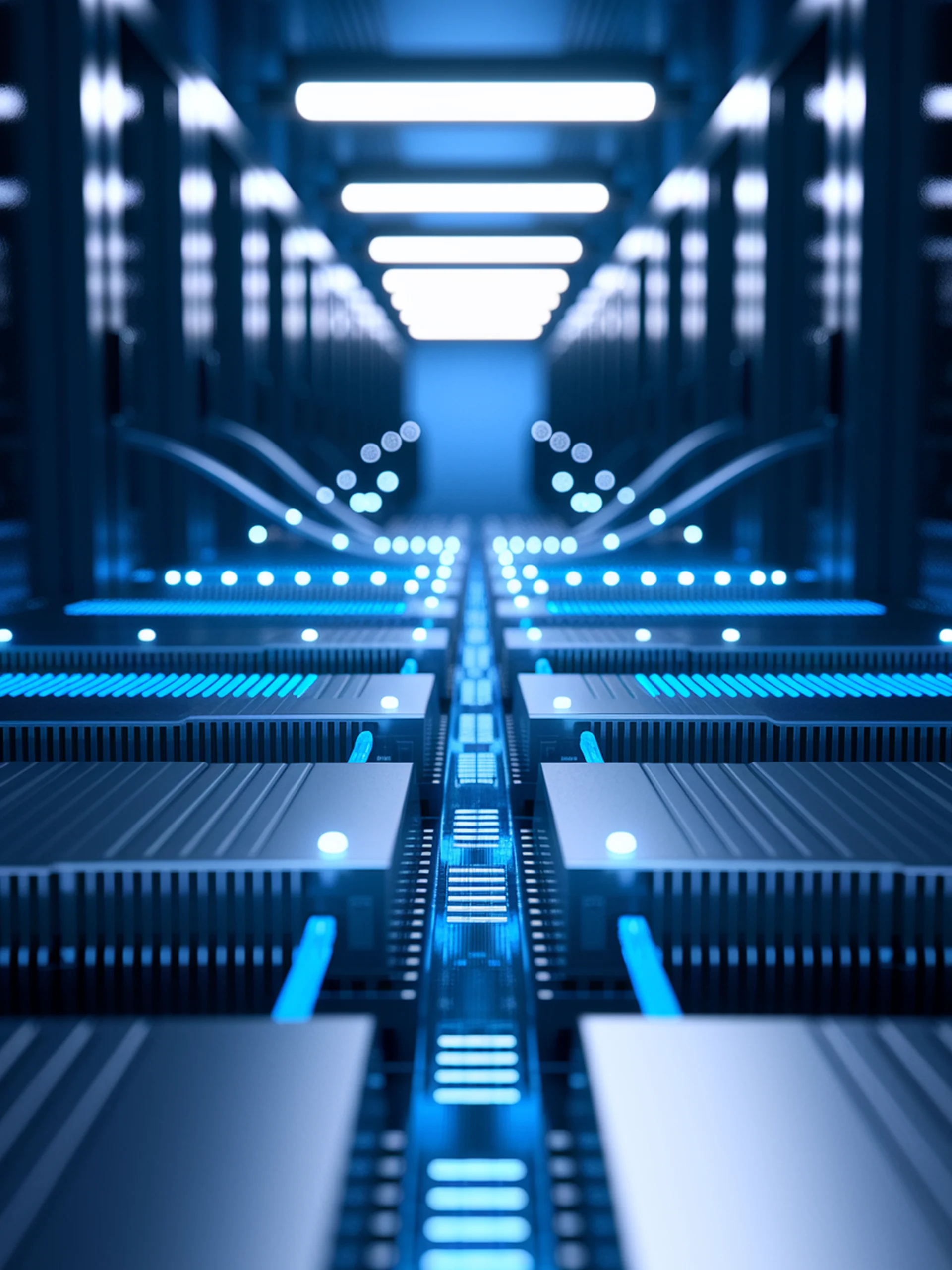
Smart KV Cache Quantization
Boosting LLM inference efficiency without sacrificing performance
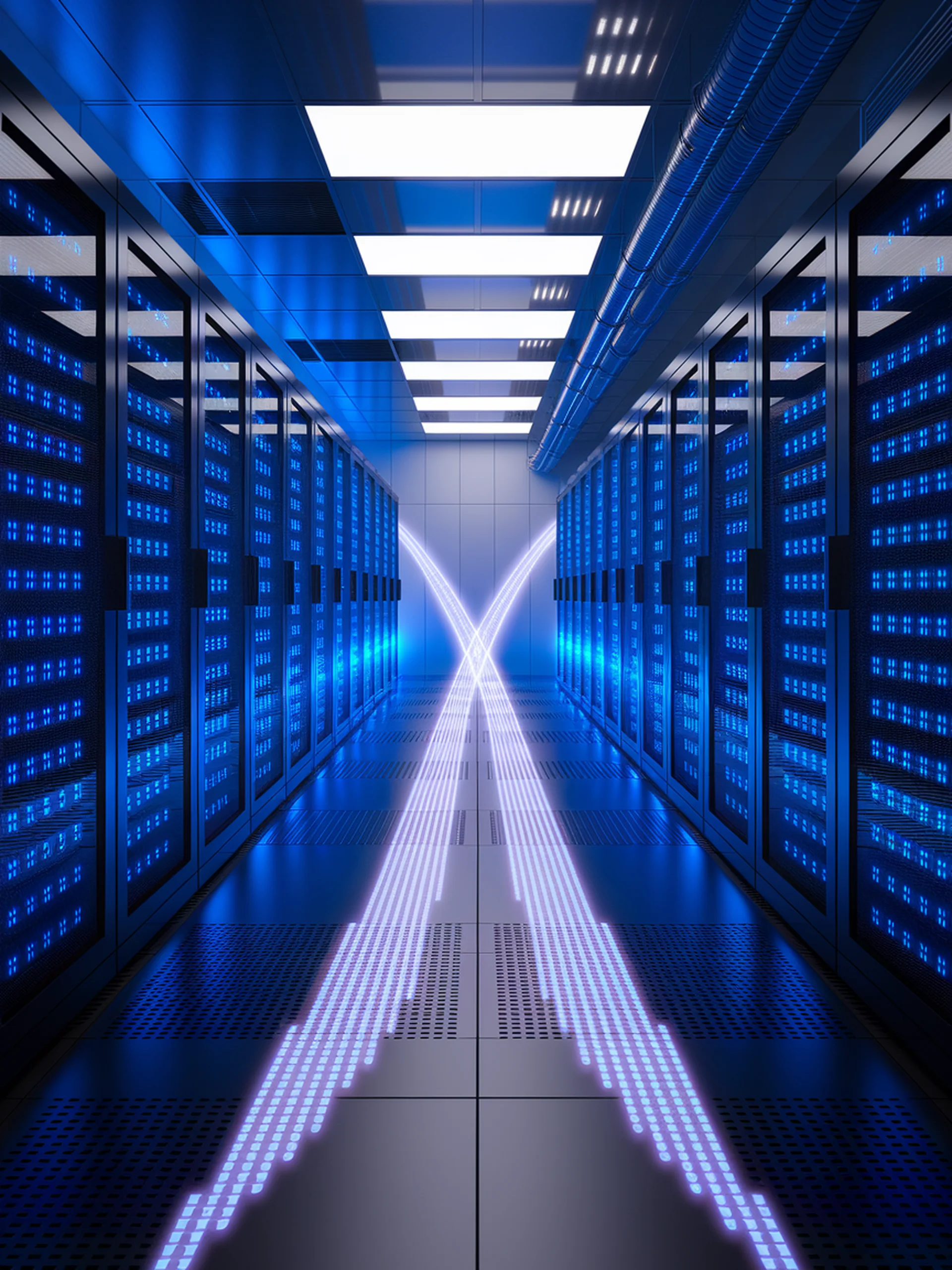
Optimizing LLM Query Processing
Innovative Scheduling with Prefix Reuse for Faster Response Times
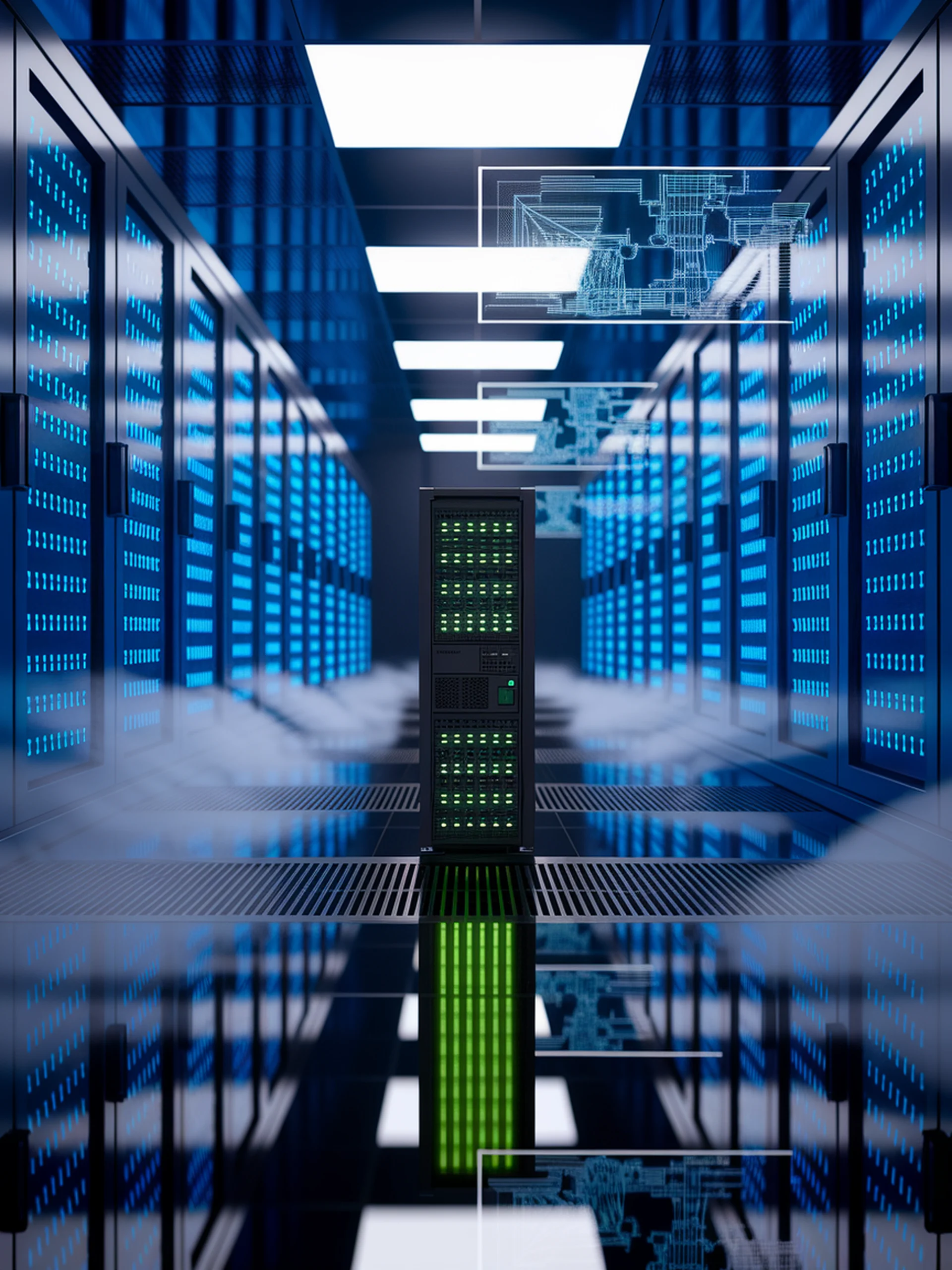
1-Bit LLM Training Breakthrough
Stable Training of Large Language Models with Extreme Quantization
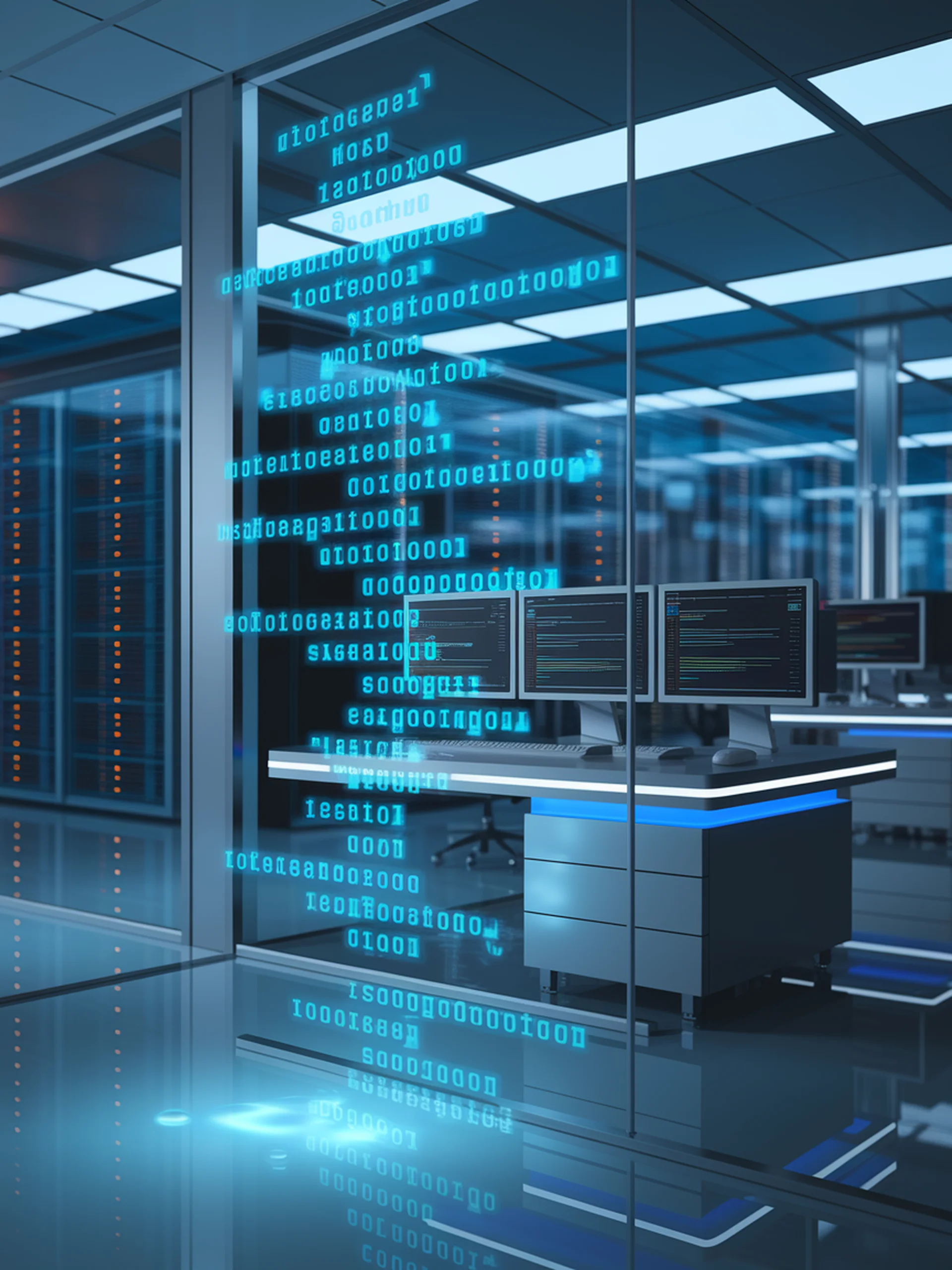
Grammar-Constrained Generation: A Breakthrough
17.71x Faster Syntax Control for LLM Outputs
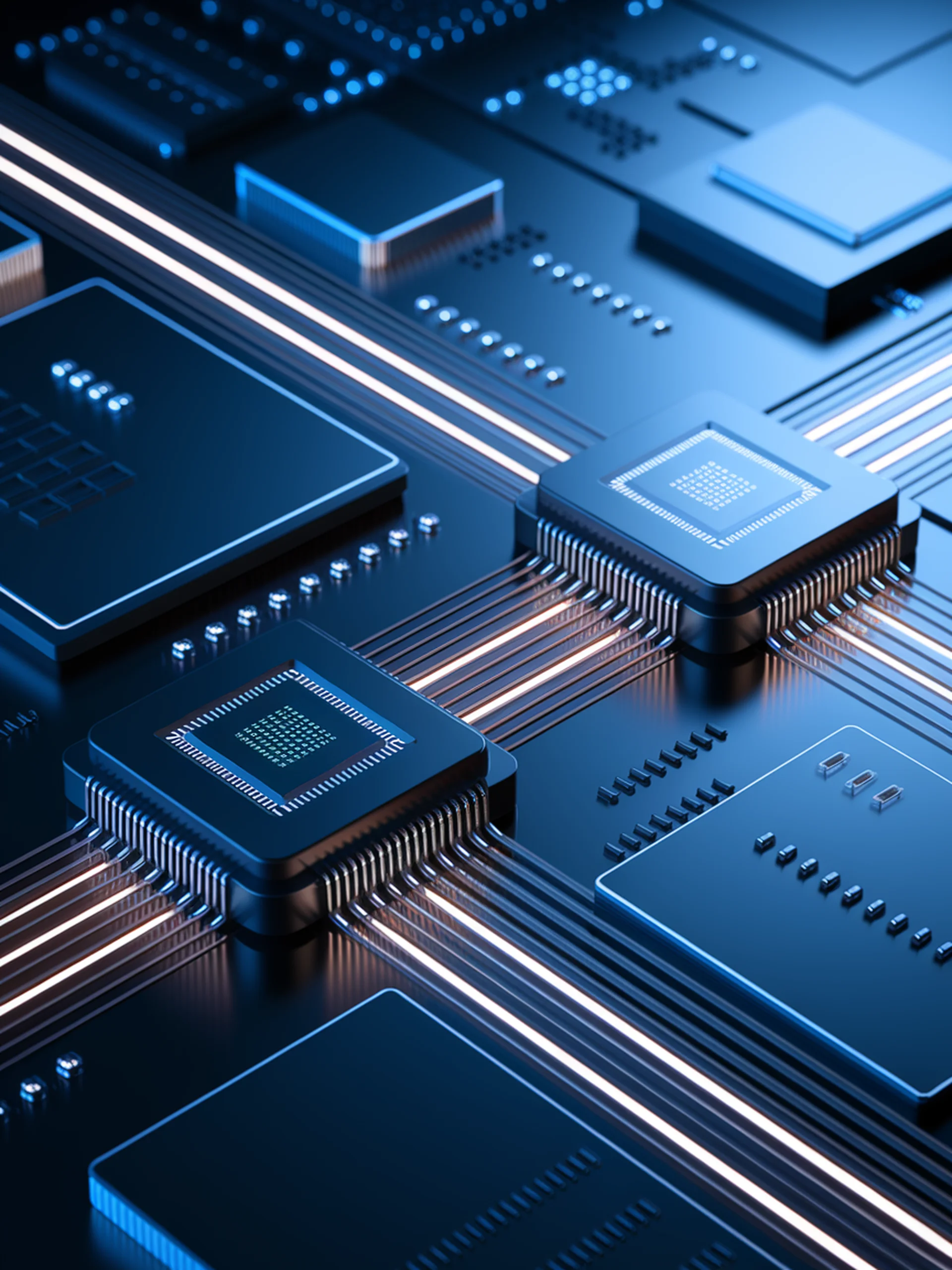
Breaking Vocabulary Barriers in LLM Inference
New speculative decoding algorithms accelerate LLMs without vocabulary constraints
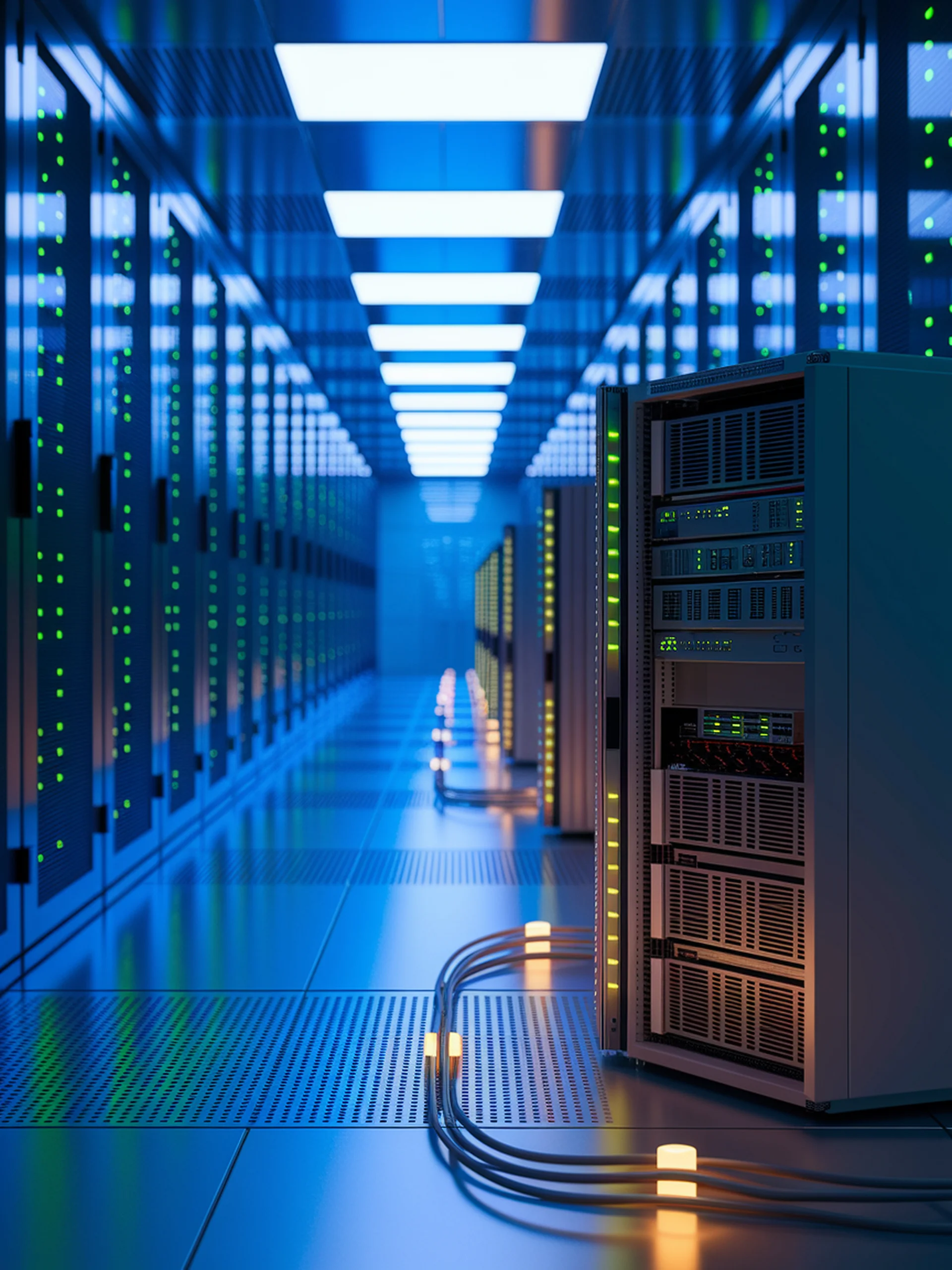
Optimizing Memory for Giant AI Models
A novel approach for efficient MoE model serving
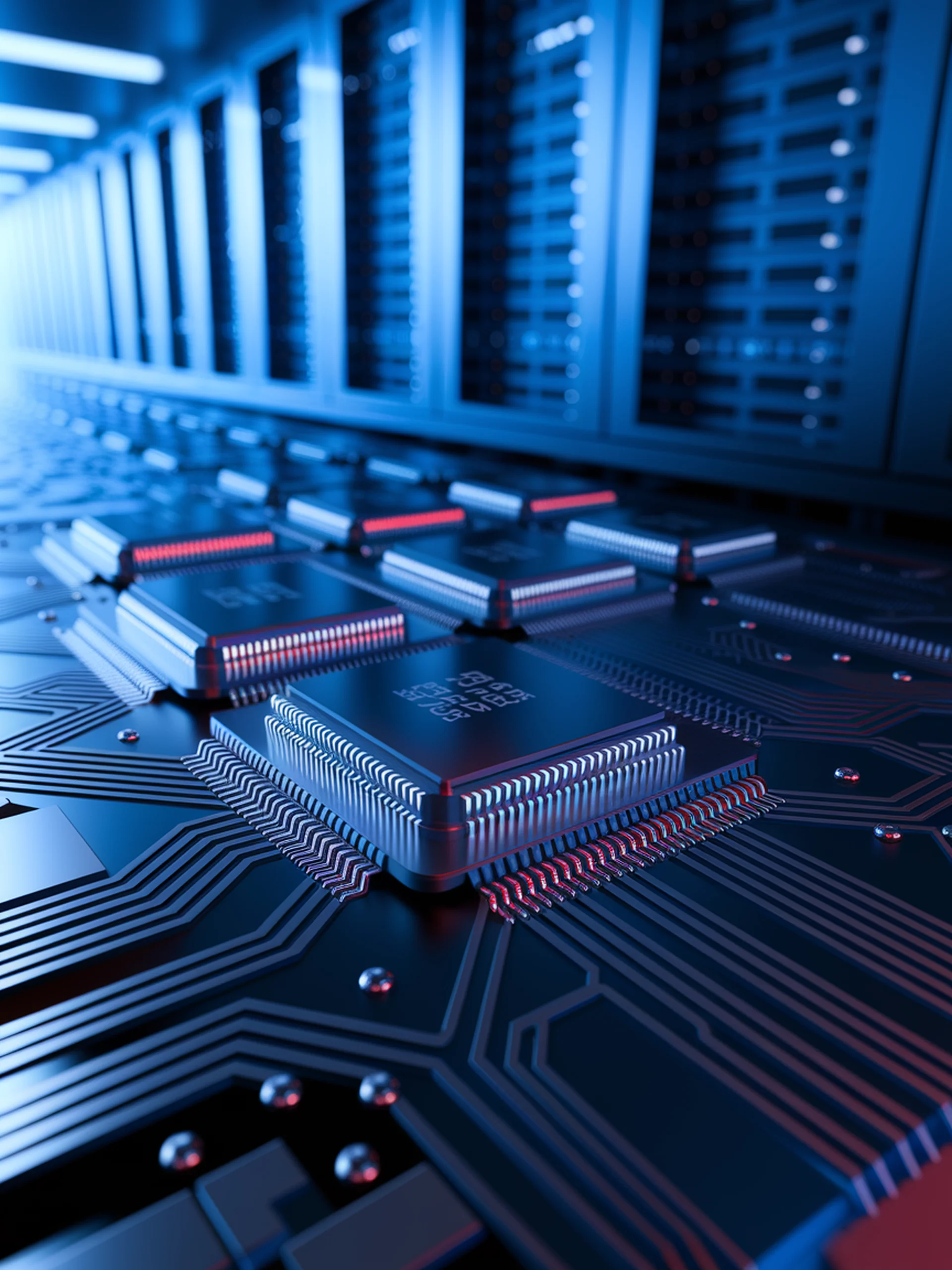
4-bit LLM Inference Breakthrough
Enabling Ultra Low-Precision Models Without Retraining
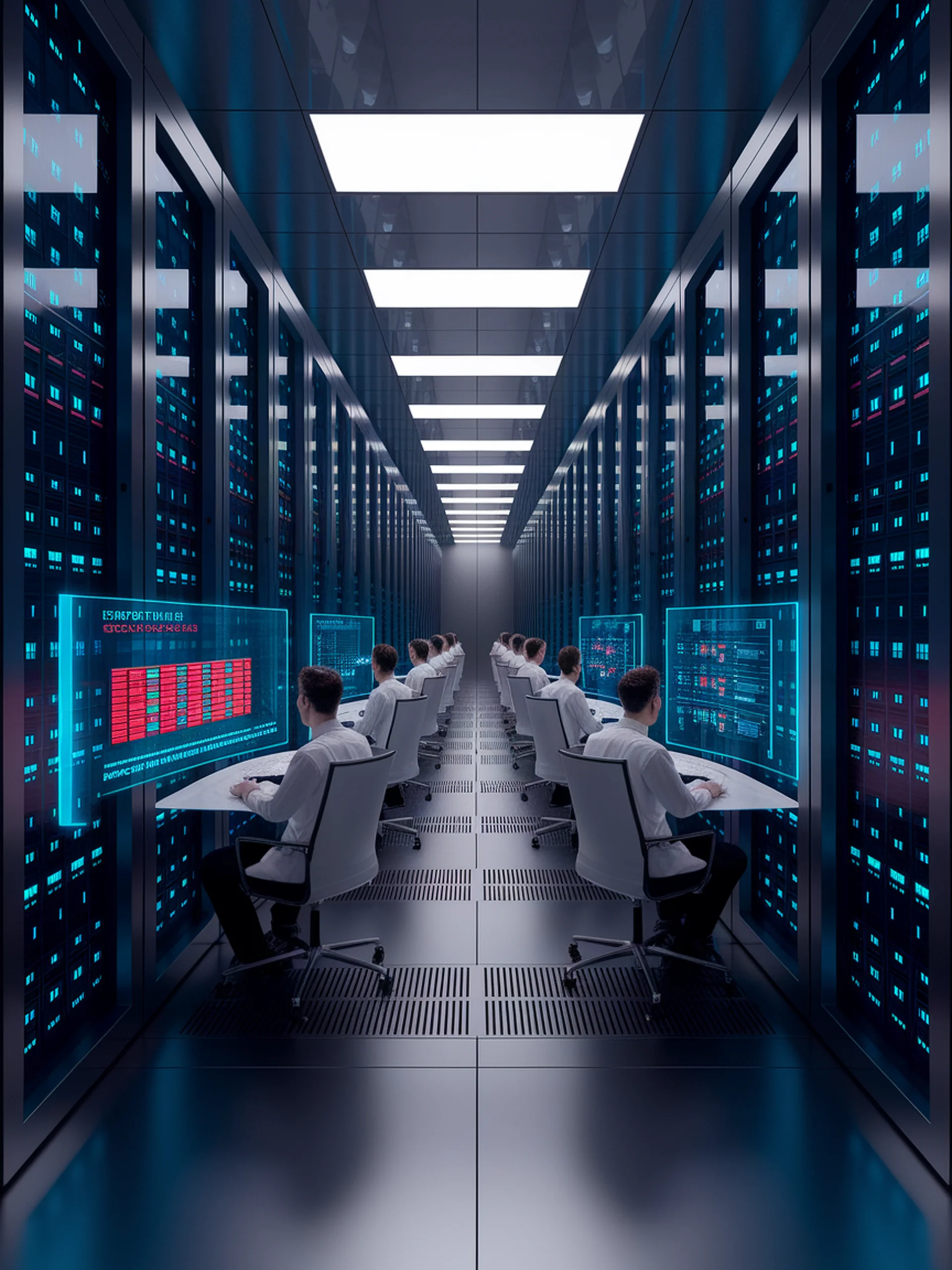
Diagnosing LLM Training Anomalies at Scale
A framework for real-time detection in thousand-plus GPU clusters
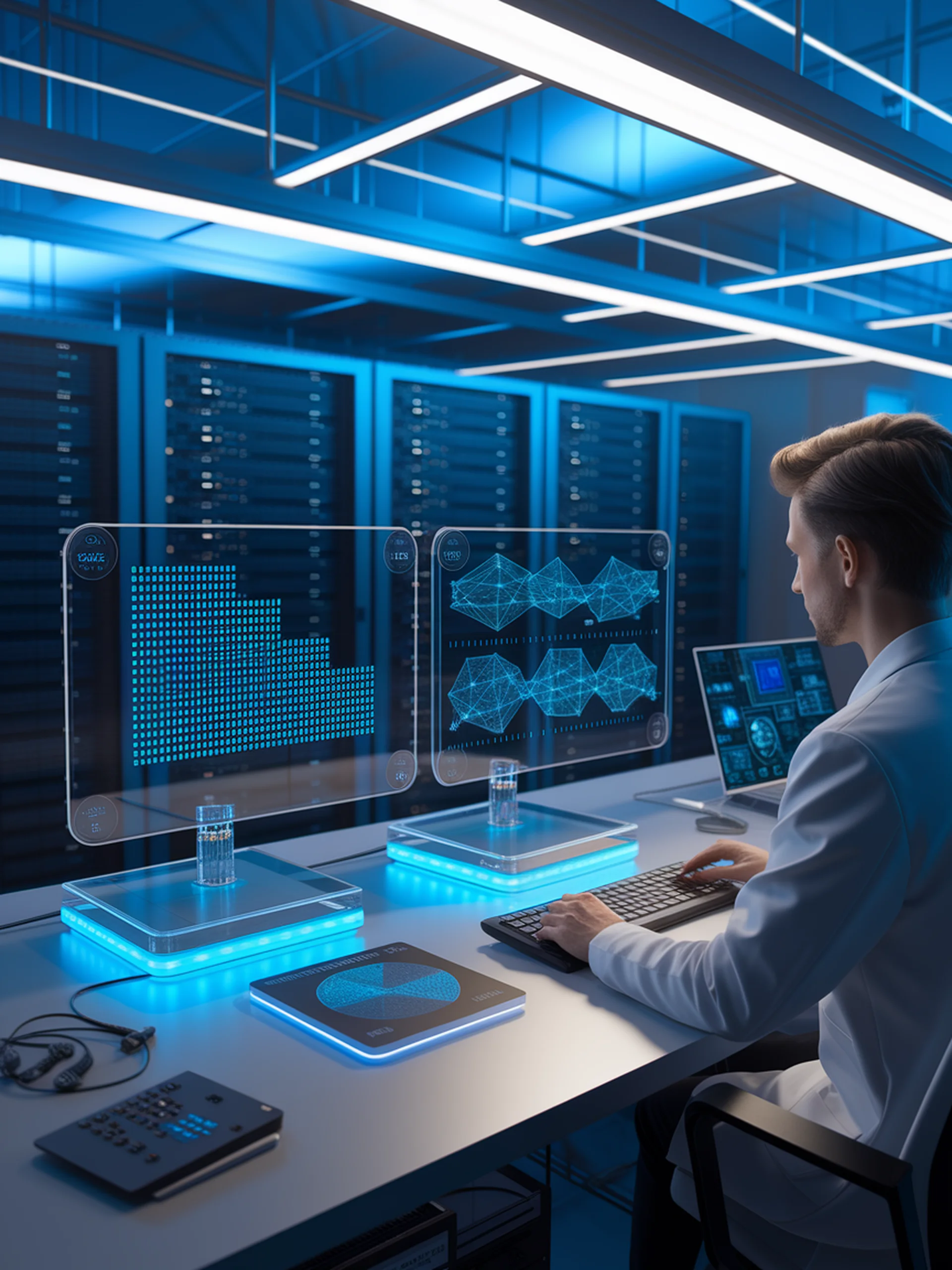
SSH: Revolutionizing LLM Fine-tuning
A more efficient alternative to LoRA with sparse spectrum adaptation

Accelerating LLMs with Hierarchical Drafting
Faster inference through smart token prediction based on temporal patterns
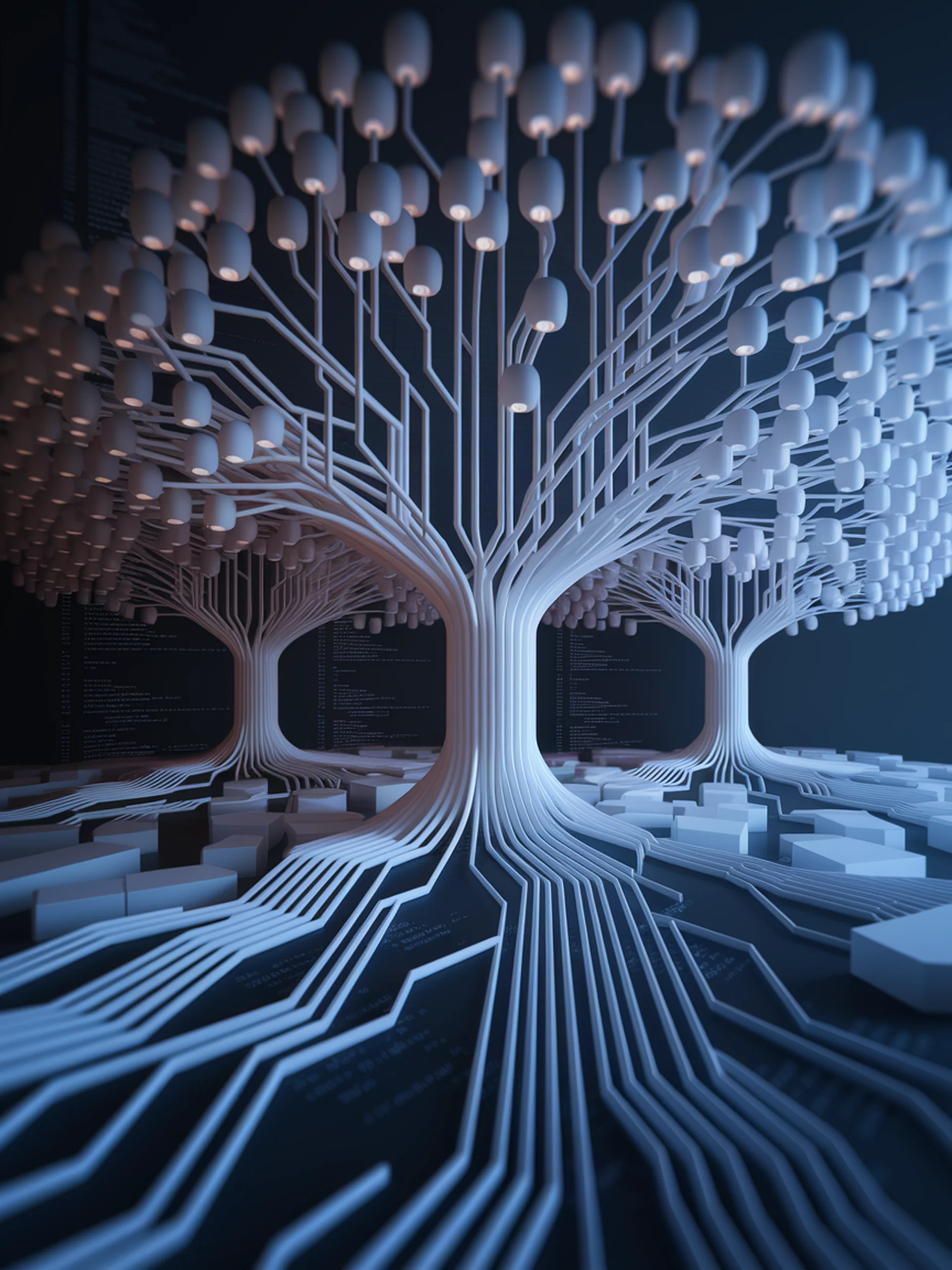
Speeding Up LLMs with Dynamic Tree Attention
A smarter approach to parallel token prediction
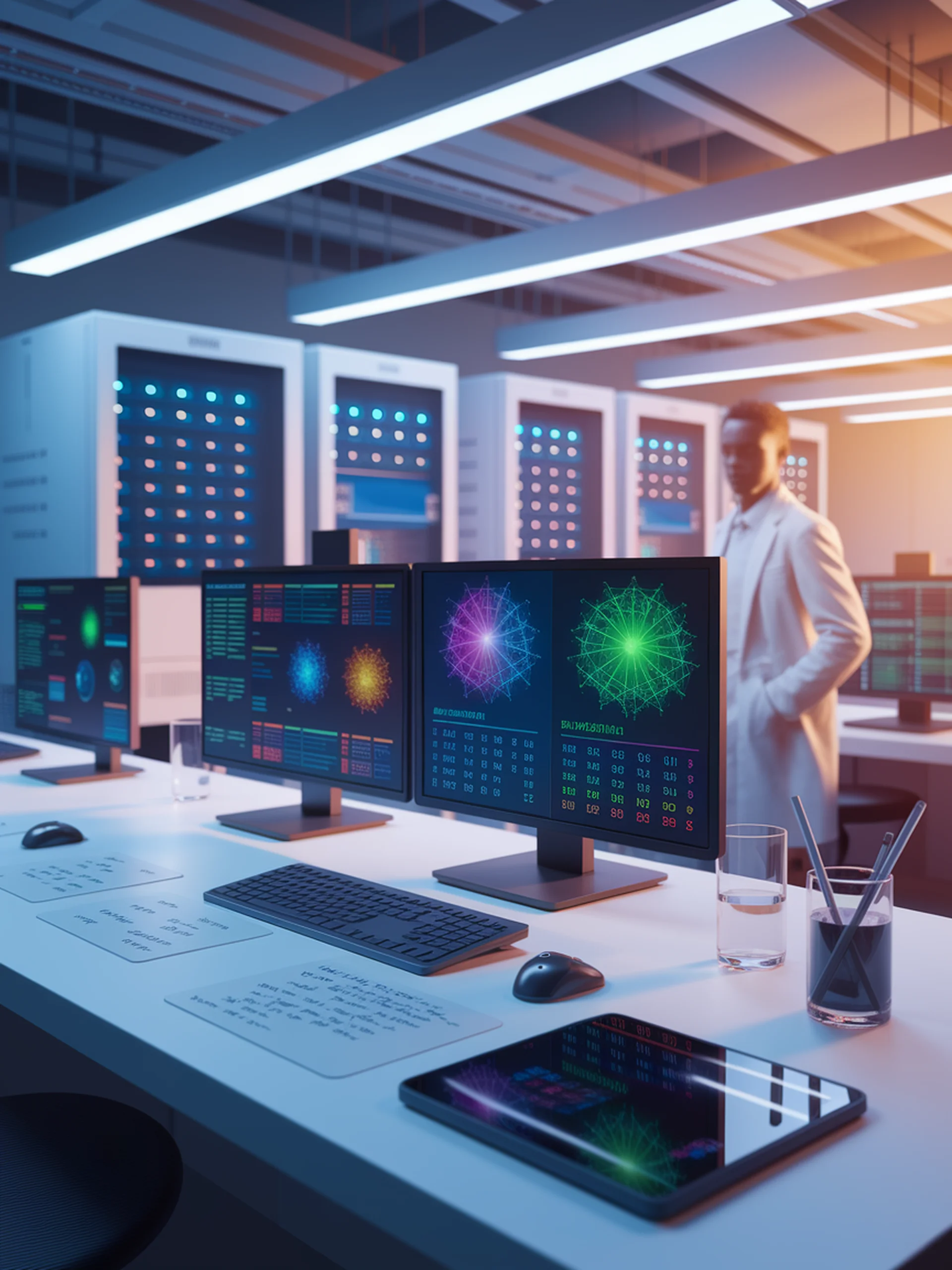
FP8 Training Breakthrough for LLMs
Efficient 8-bit precision without the complexity
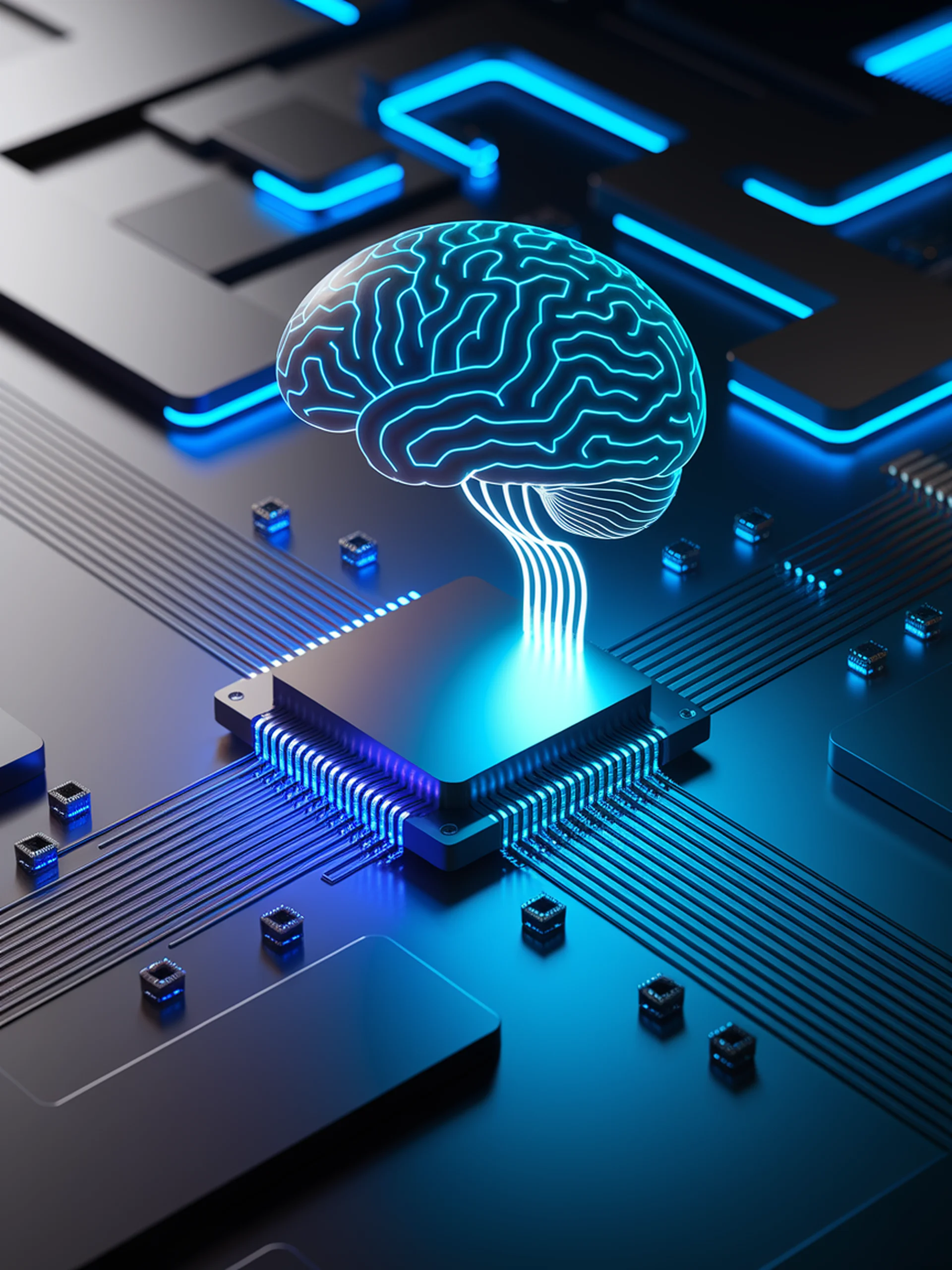
Enhancing AI Memory: The LM2 Breakthrough
Transformer models with auxiliary memory for superior reasoning
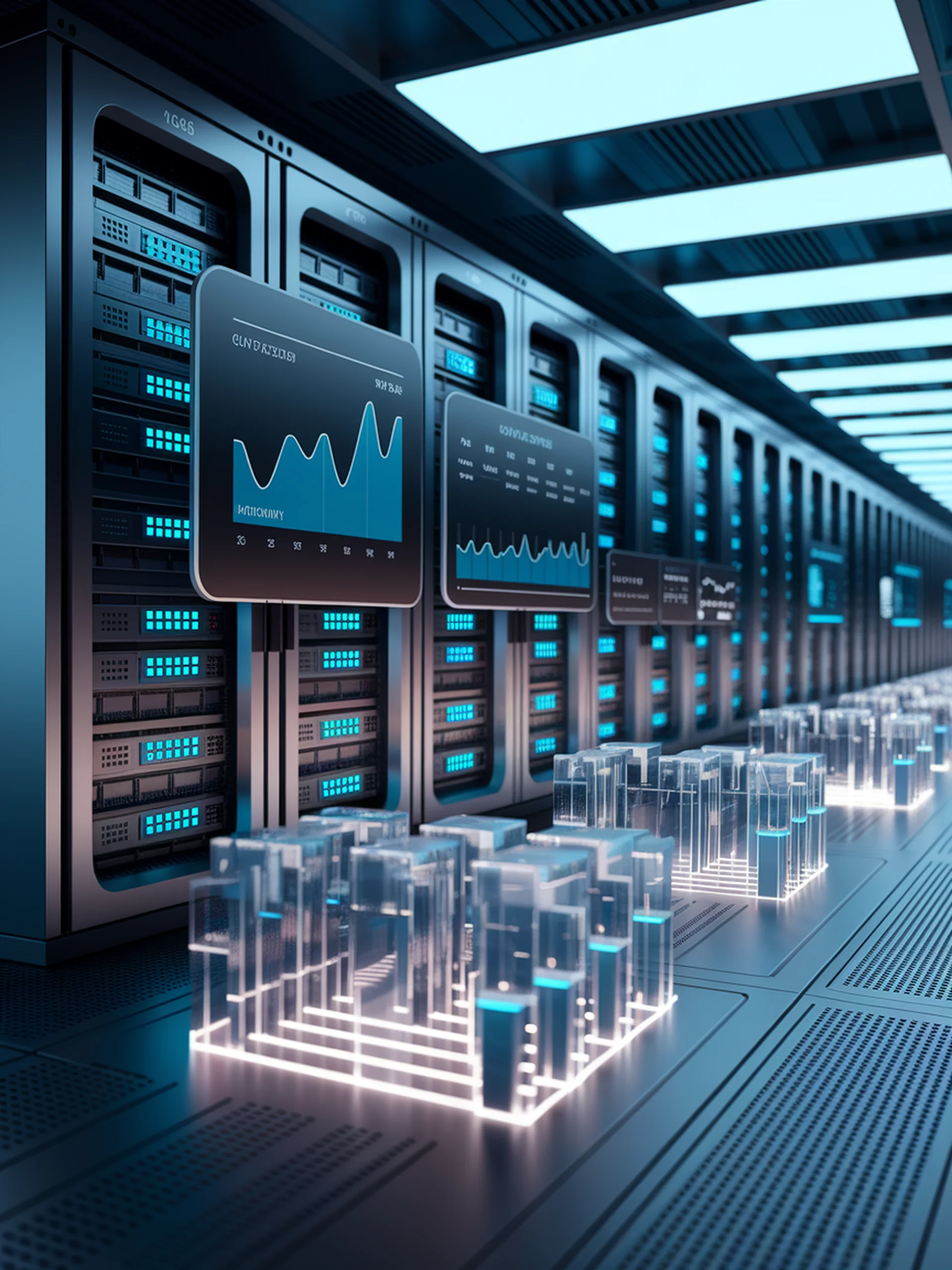
Stateless LLM Training: A Memory Breakthrough
Efficient LLM training without optimizer states
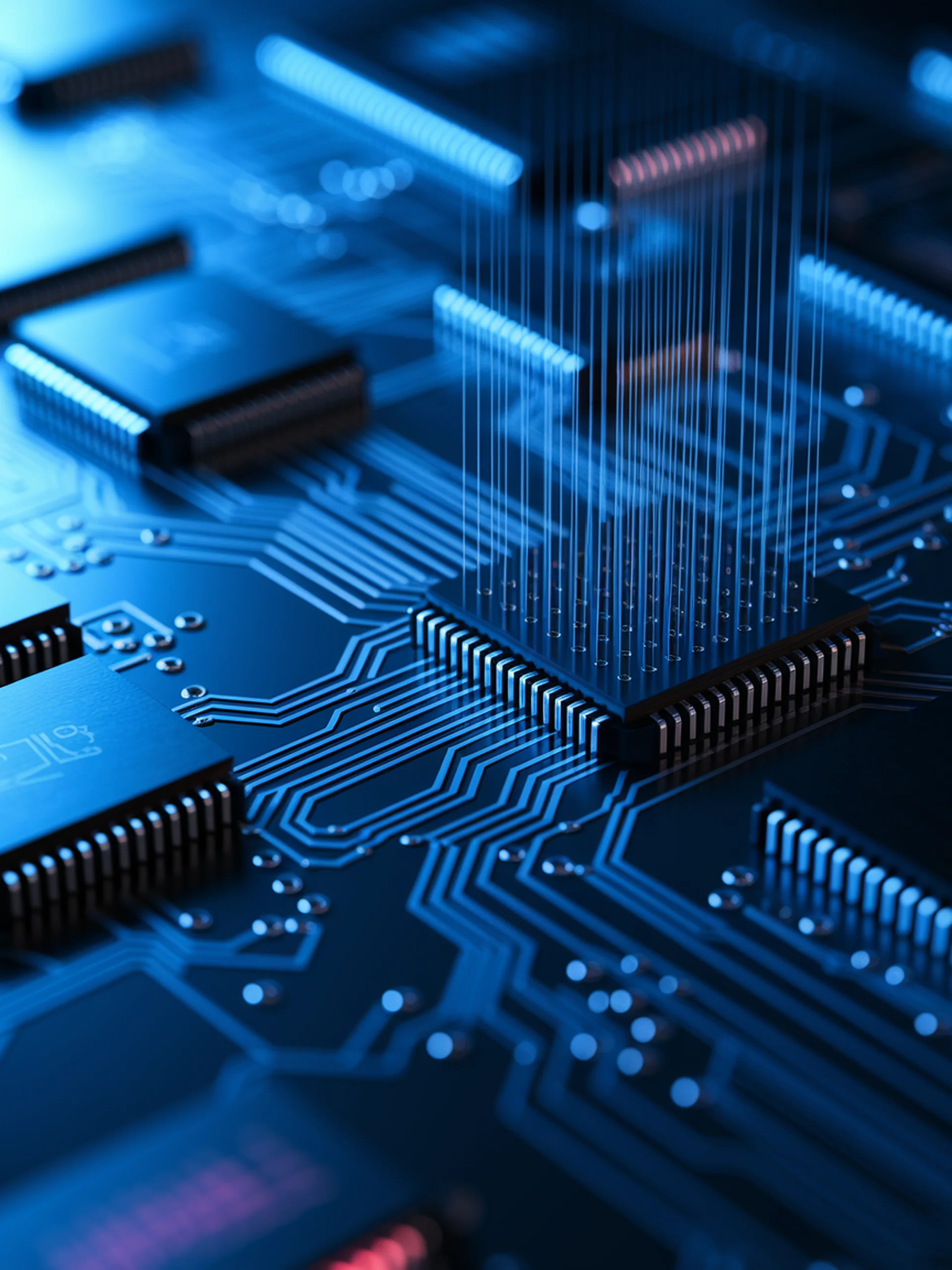
Quantum-Inspired Adapters for LLMs
Hyper-compressed fine-tuning for resource-constrained environments
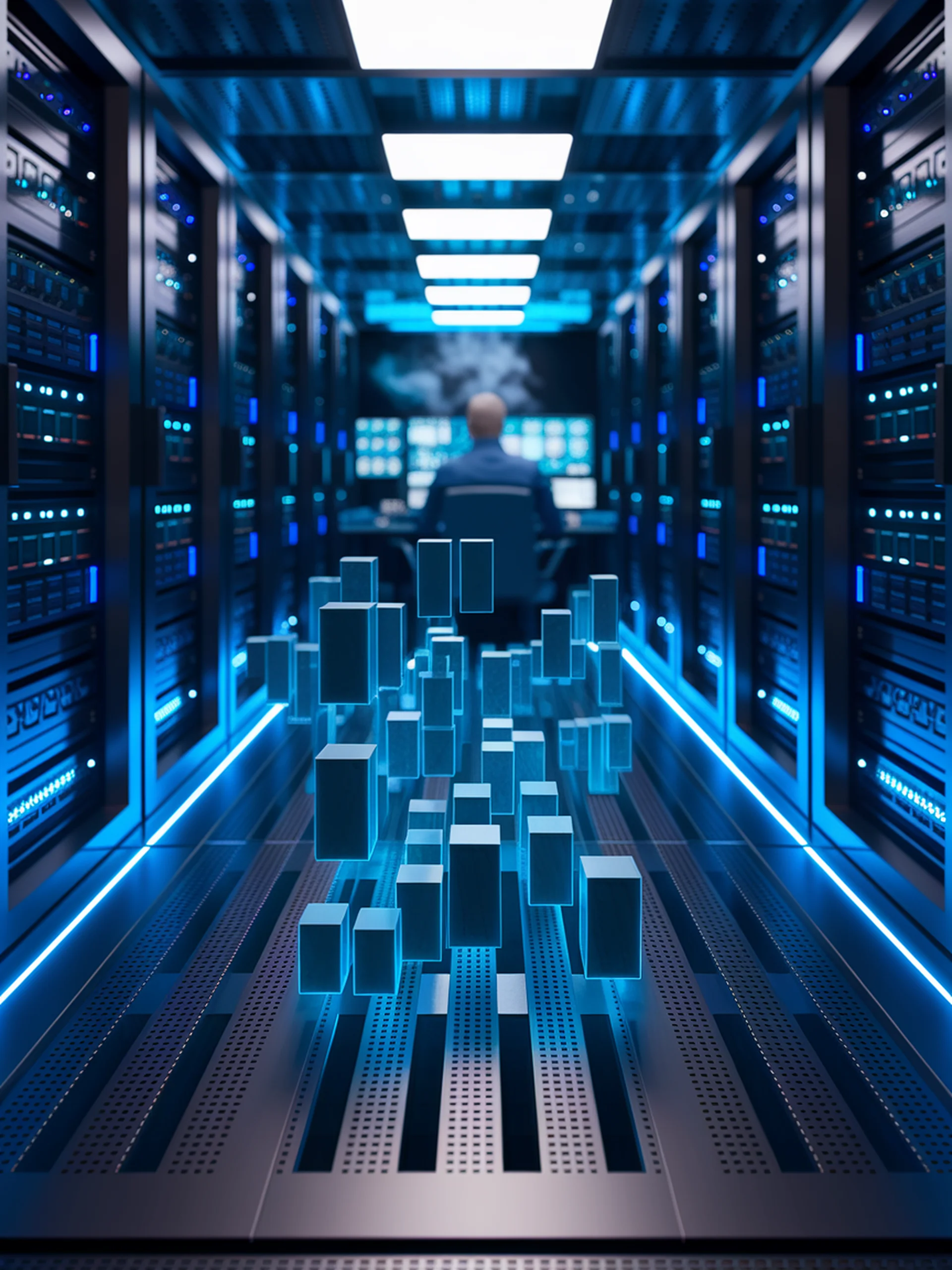
Optimizing LLM Performance under Memory Constraints
Novel scheduling approaches for efficient inference with KV cache limitations

Solving the Batch Editing Problem in LLMs
A novel approach for efficient knowledge editing in language models
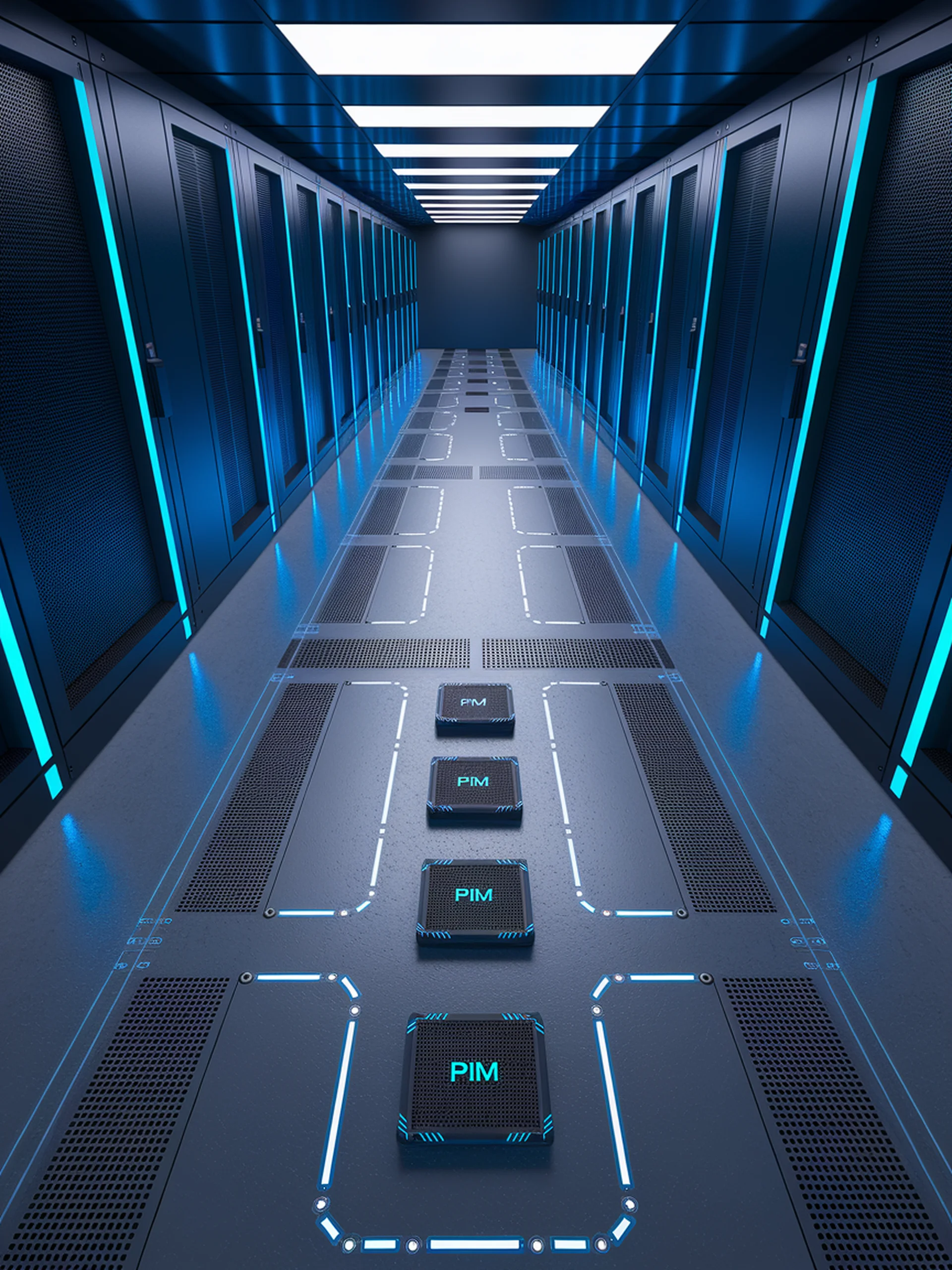
Revolutionizing LLM Inference with PIM Architecture
A GPU-free approach for efficient large language model deployment

Smarter, Faster LLM Optimizers
Revolutionizing training efficiency through structured matrix approximation

Evolutionary Compression of LLMs
Making Large Language Models Faster Through Intelligent Pruning
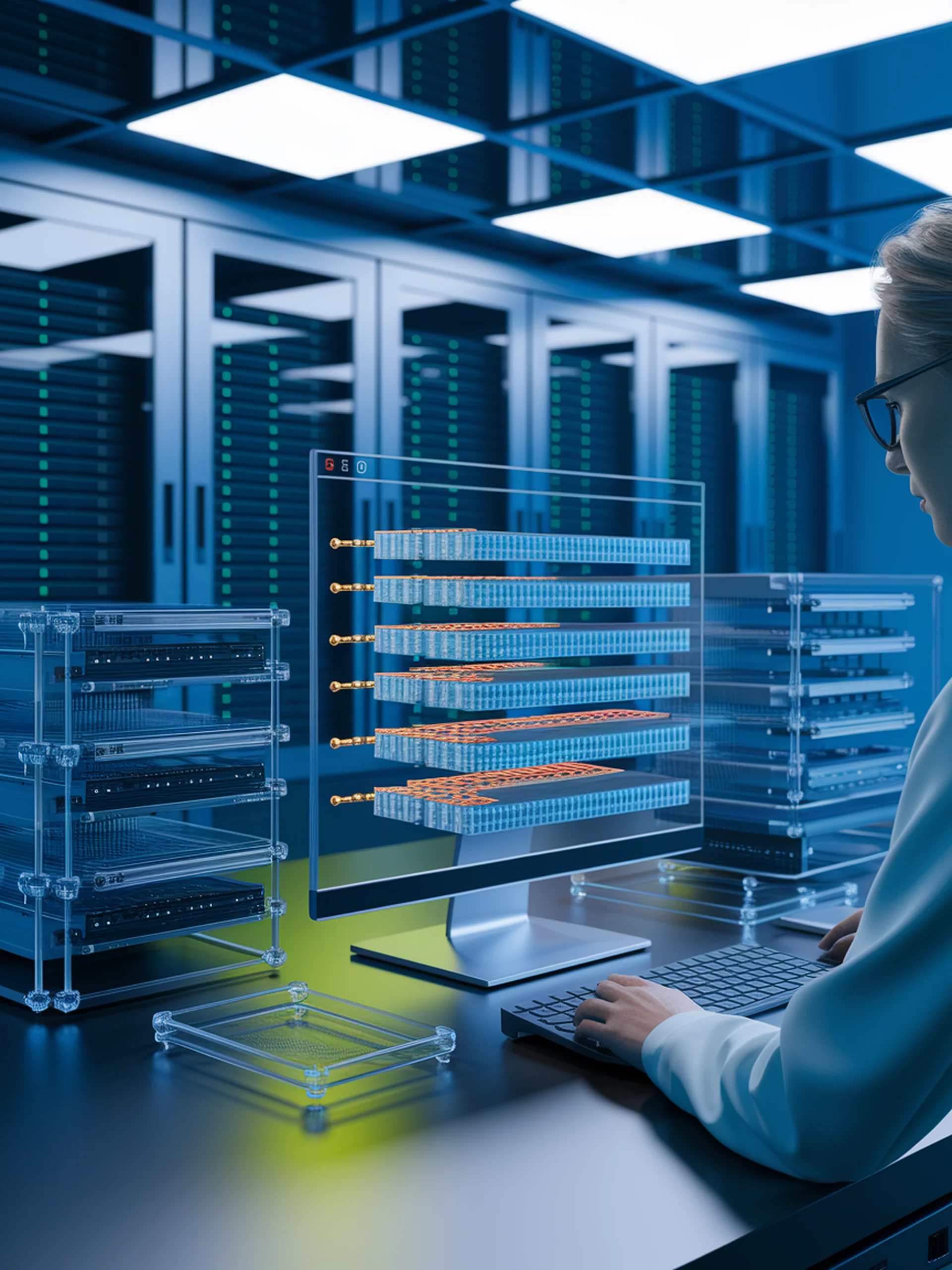
Accelerating LLM Inference with Parameter Sharing
Reducing memory footprint while preserving model performance

Efficient LLM Inference with Latent Attention
Reducing memory bottlenecks without sacrificing performance

Optimizing LLM Inference with HexGen-2
Efficient LLM deployment across heterogeneous GPU environments

Efficient Text Embeddings with Sparse Experts
Reducing Memory & Latency Without Sacrificing Performance

LowRA: Breaking the Fine-tuning Barrier
Enabling sub-2-bit LoRA fine-tuning for resource-efficient LLMs
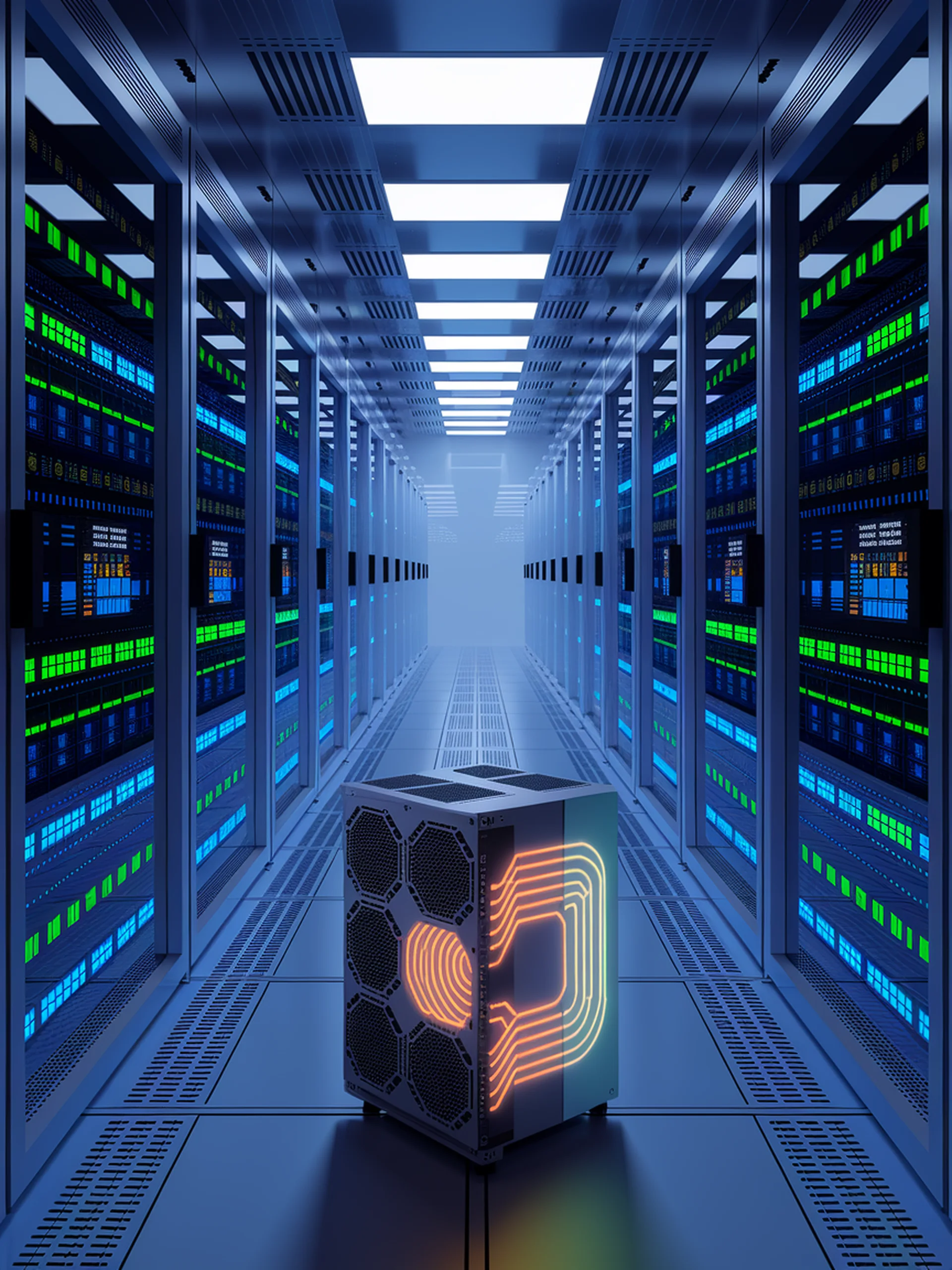
Optimizing LLM Memory for Real-World Performance
A novel approach to memory management with latency guarantees

Smarter LLM Compression
A Context-Aware Approach to Model Size Reduction

Boosting LLM Efficiency with Smart Attention
A Novel Approach to Reduce Computational Cost in Transformers

Breaking Context Barriers in LLMs
Enabling 3M token context on a single GPU
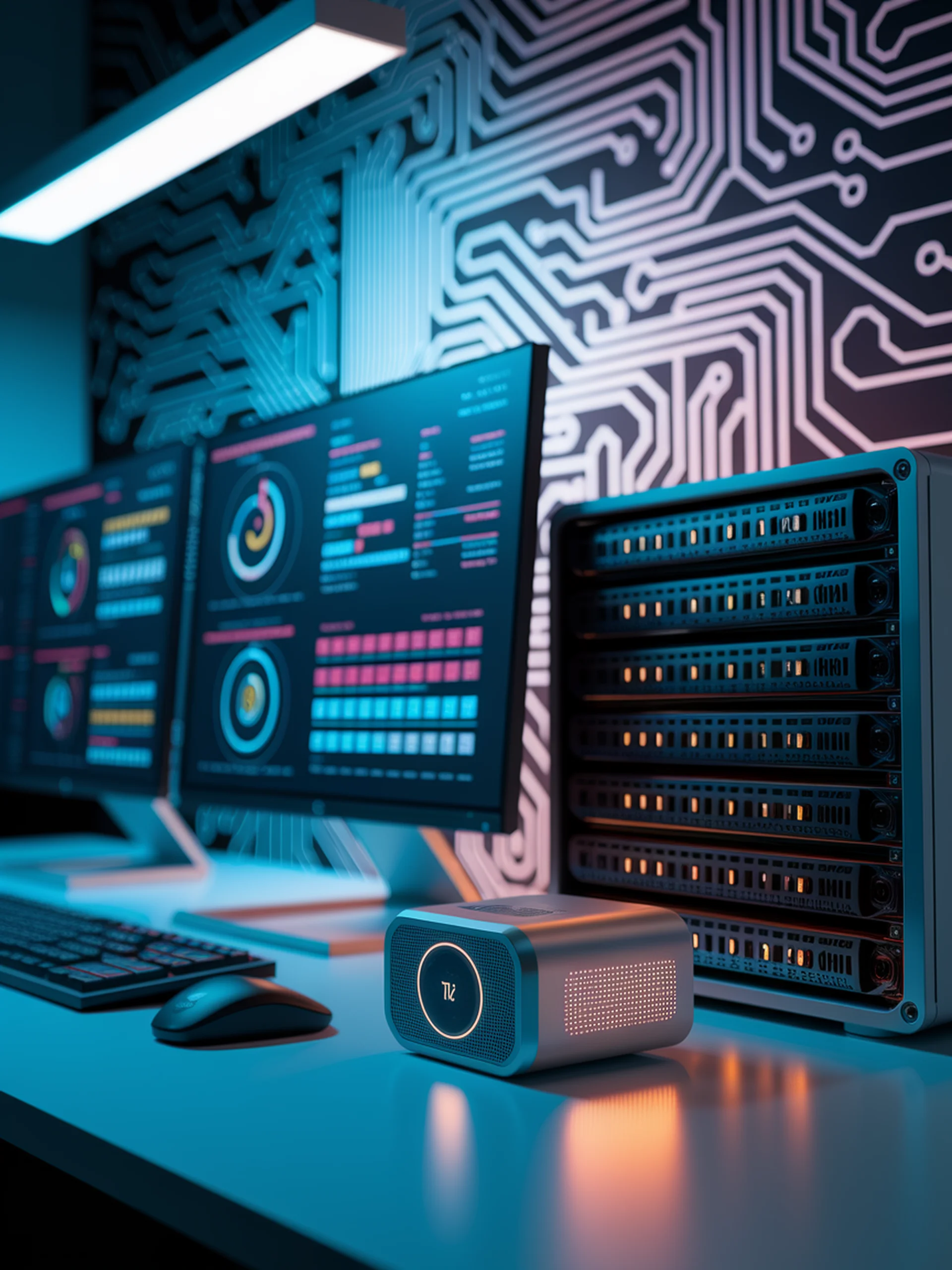
Streamlining LLM Deployment with RoSTE
A unified approach to quantization and fine-tuning

Optimizing LLM Deployment in the Cloud
High-performance, cost-effective LLM serving across diverse GPU resources

NestQuant: Optimizing LLM Efficiency
A breakthrough in post-training quantization using nested lattices
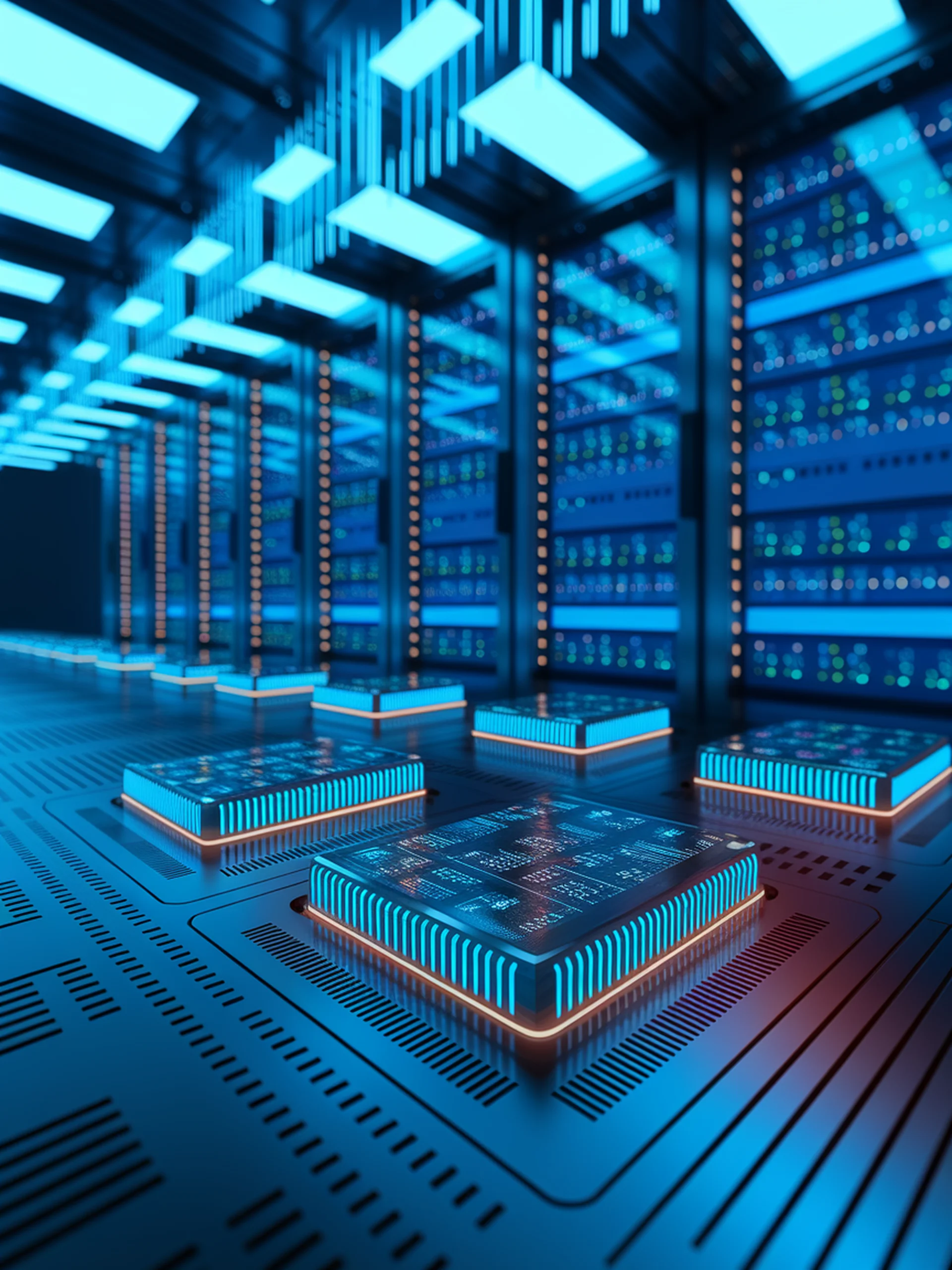
Near-Storage Processing: Supercharging LLM Inference
Boosting throughput for large language model deployment
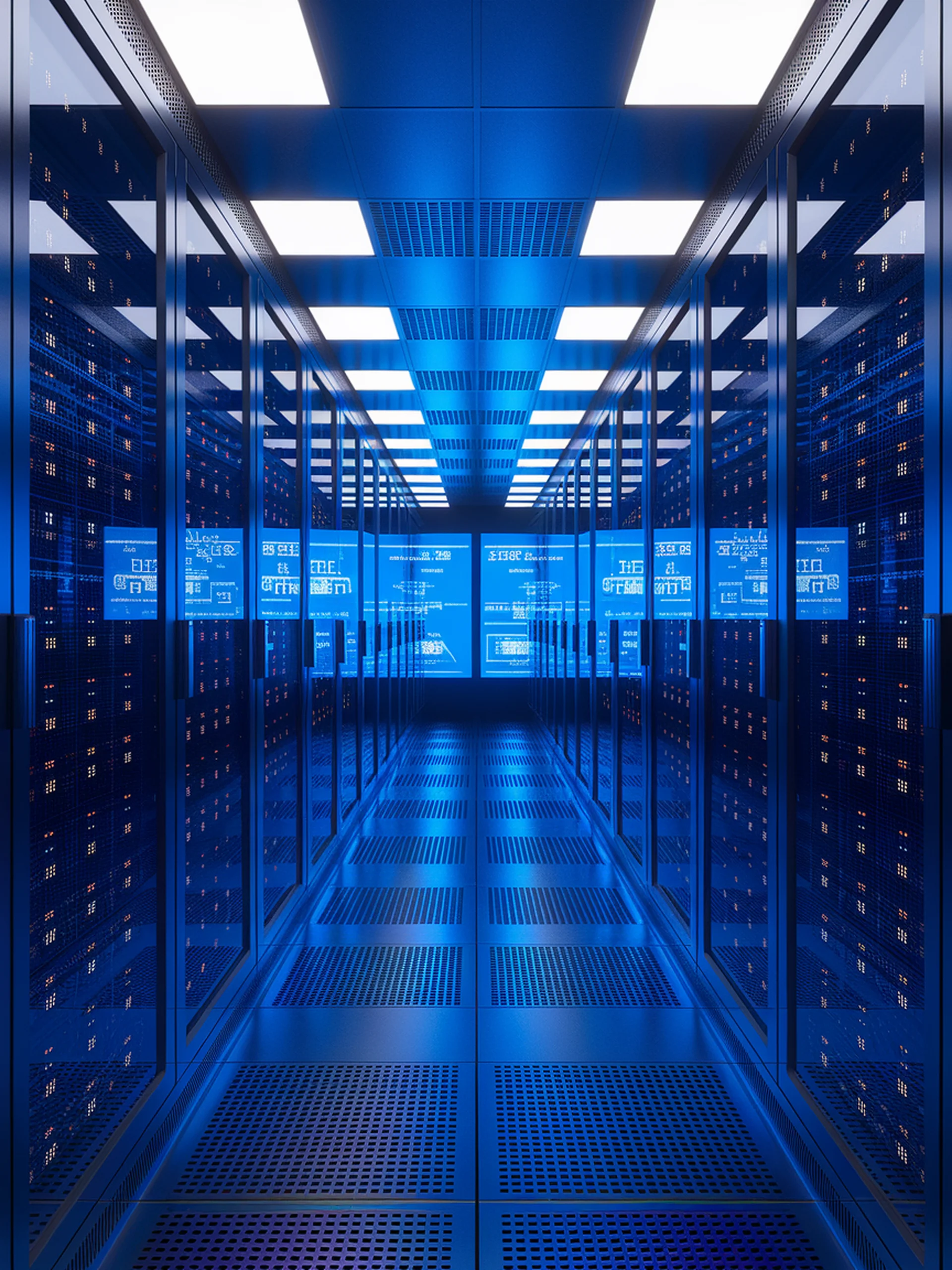
Accelerating LLM Scaling in the Cloud
Solving the serverless LLM inference bottleneck
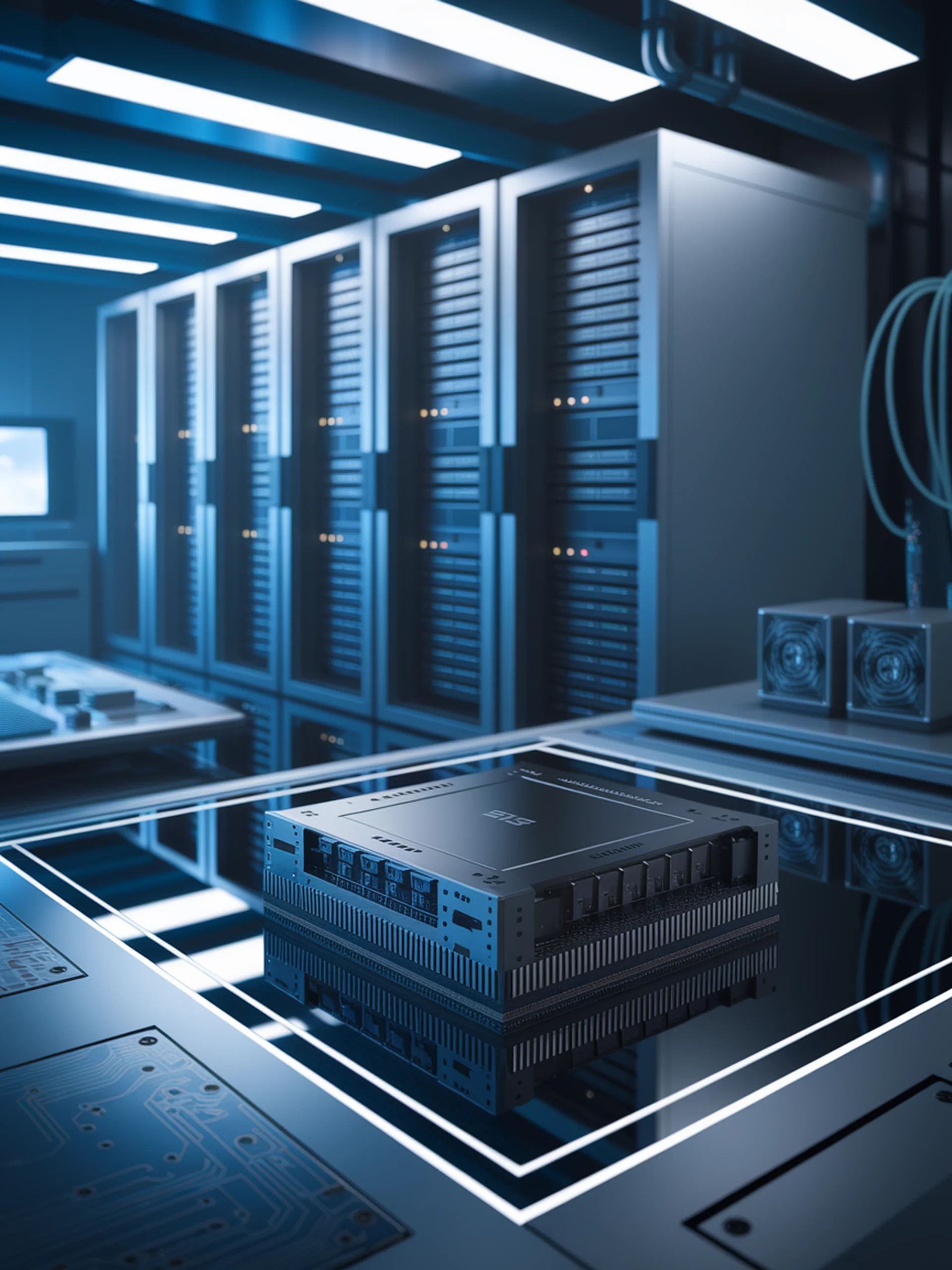
Speeding Up AI: QuantSpec Innovation
Faster LLM inference through self-speculative decoding with quantized memory

Synaptic Resonance: The Next Frontier in LLM Memory
Biologically-inspired approach to improve contextual coherence in large language models
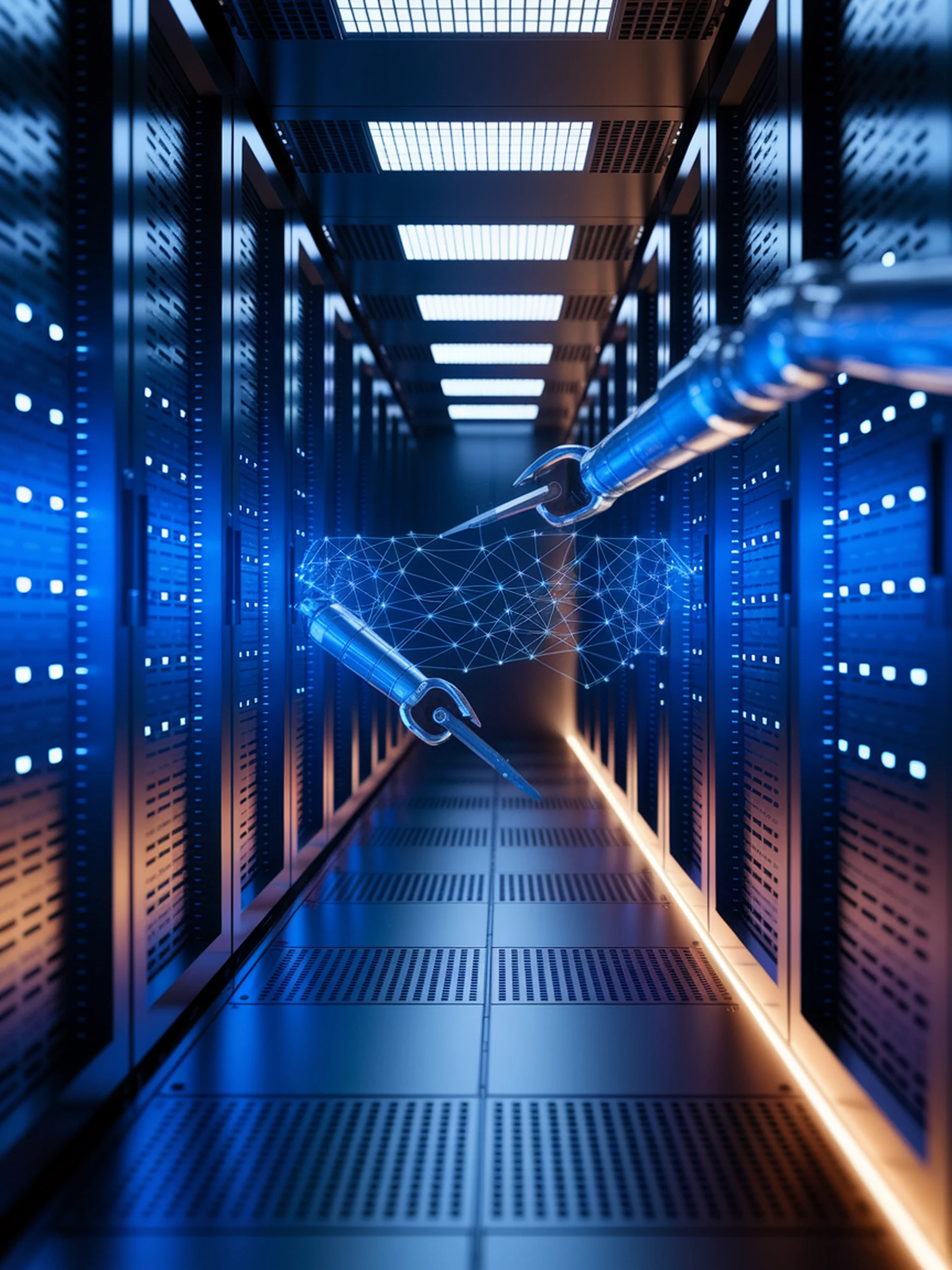
Efficient LLM Diet Plans
Evolutionary optimization for adaptive model pruning

PISA: A New Optimization Paradigm for Foundation Models
Overcoming Limitations of Traditional Training Methods
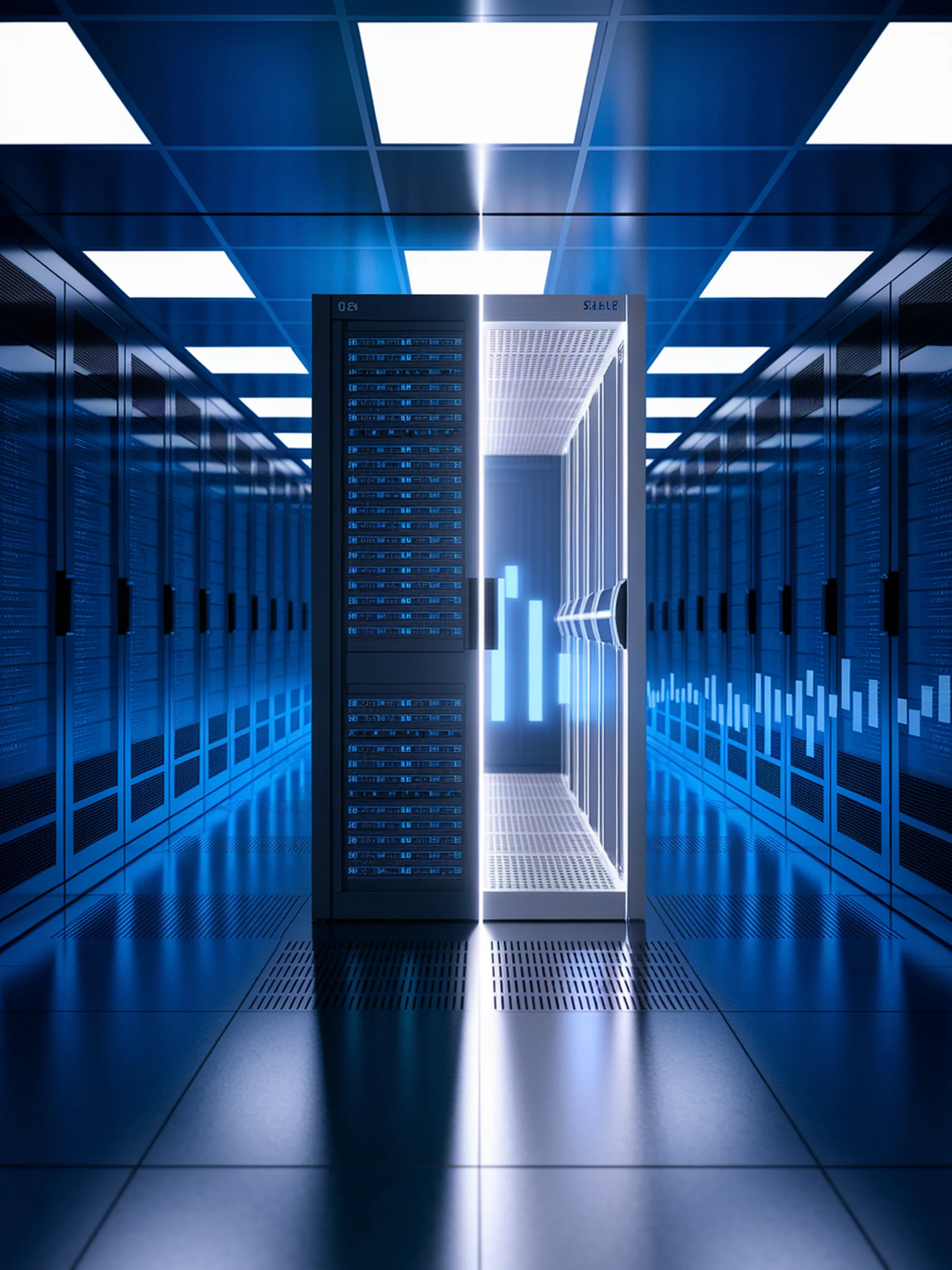
Efficient LLM Pre-Training Breakthrough
Reducing computational demands without sacrificing performance
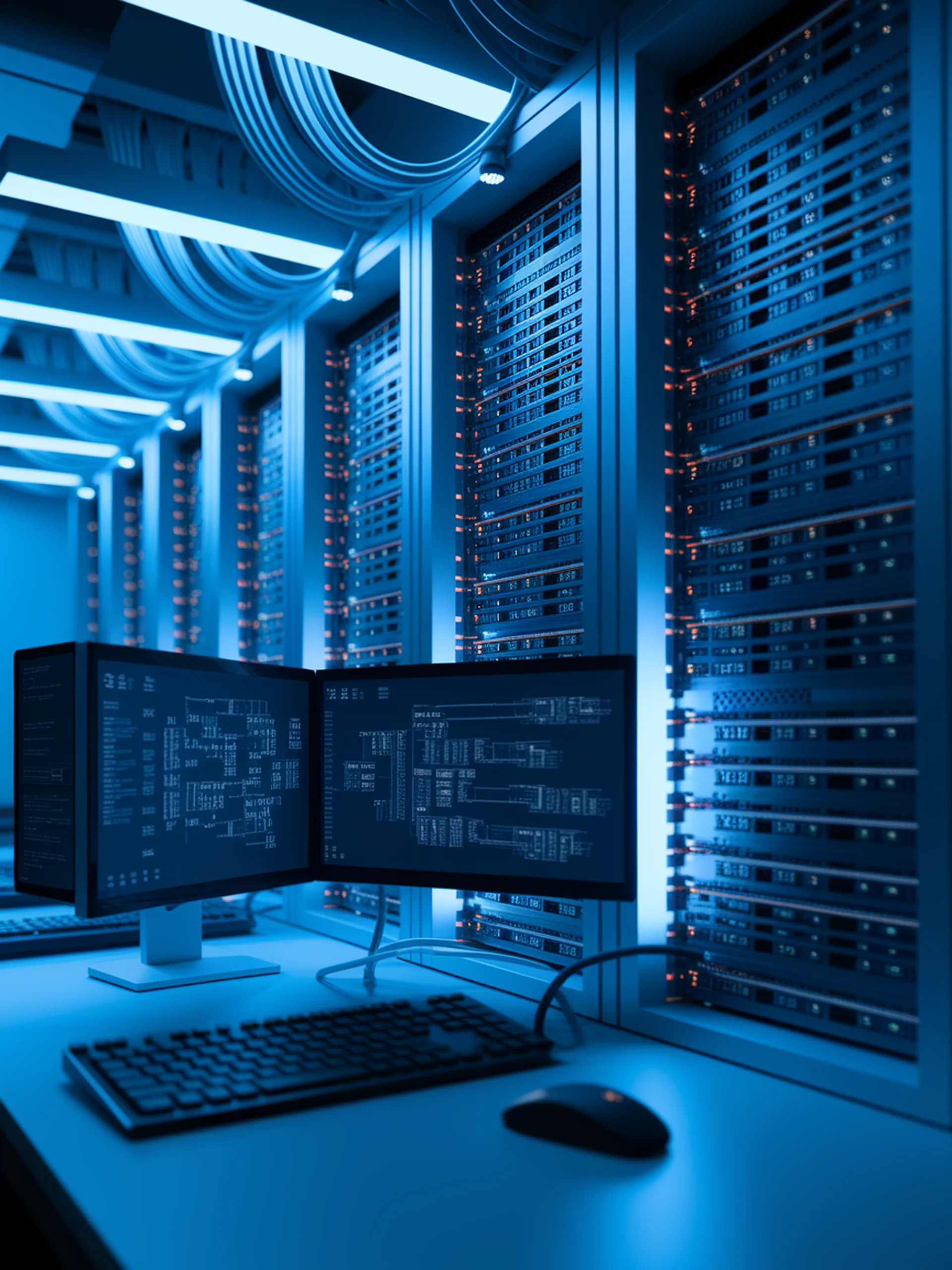
Accelerating LLM Inference with GRIFFIN
Solving Token Misalignment for Faster Speculative Decoding
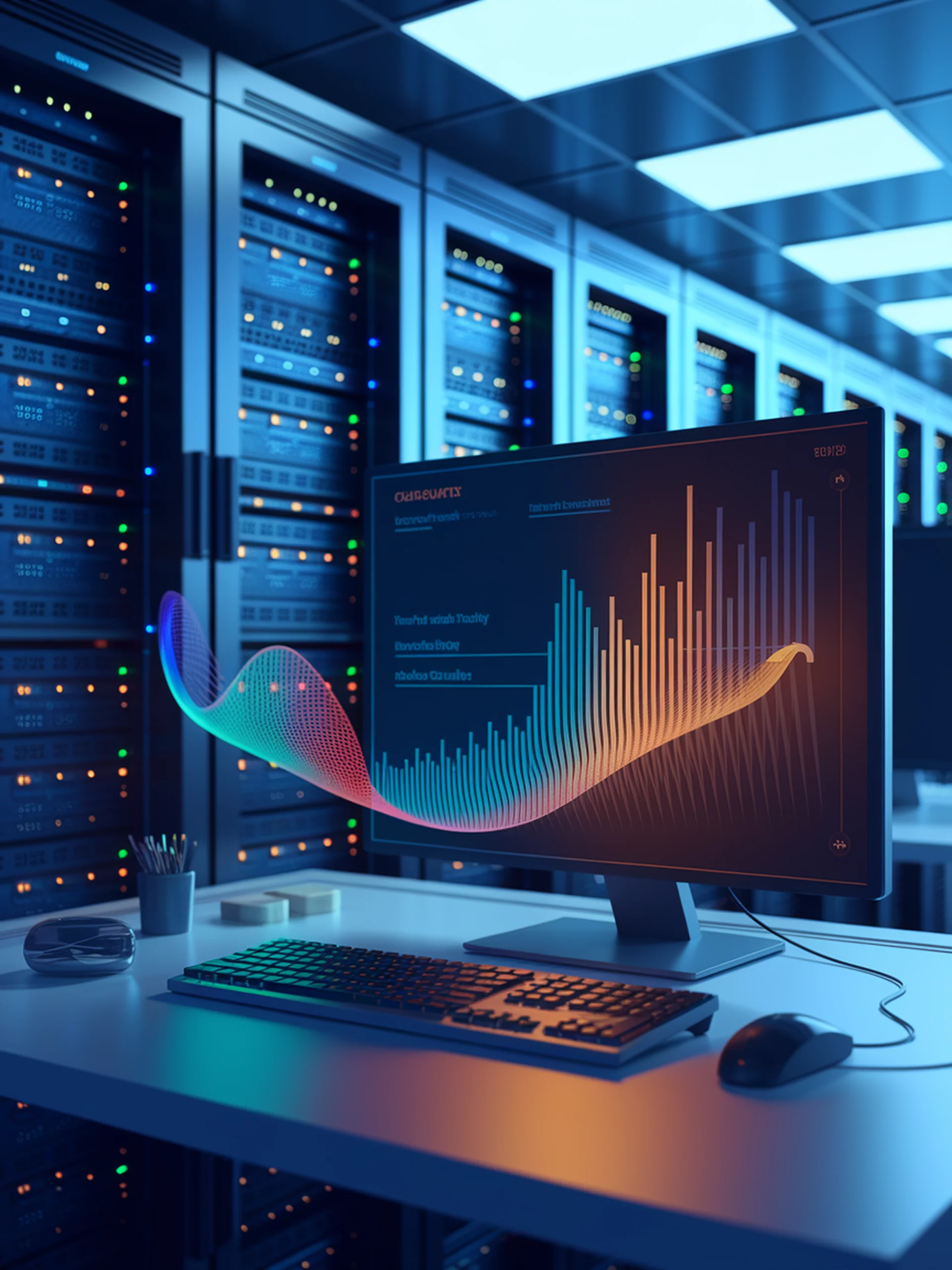
AdaGC: Stabilizing LLM Training
Adaptive gradient clipping for more efficient AI model development
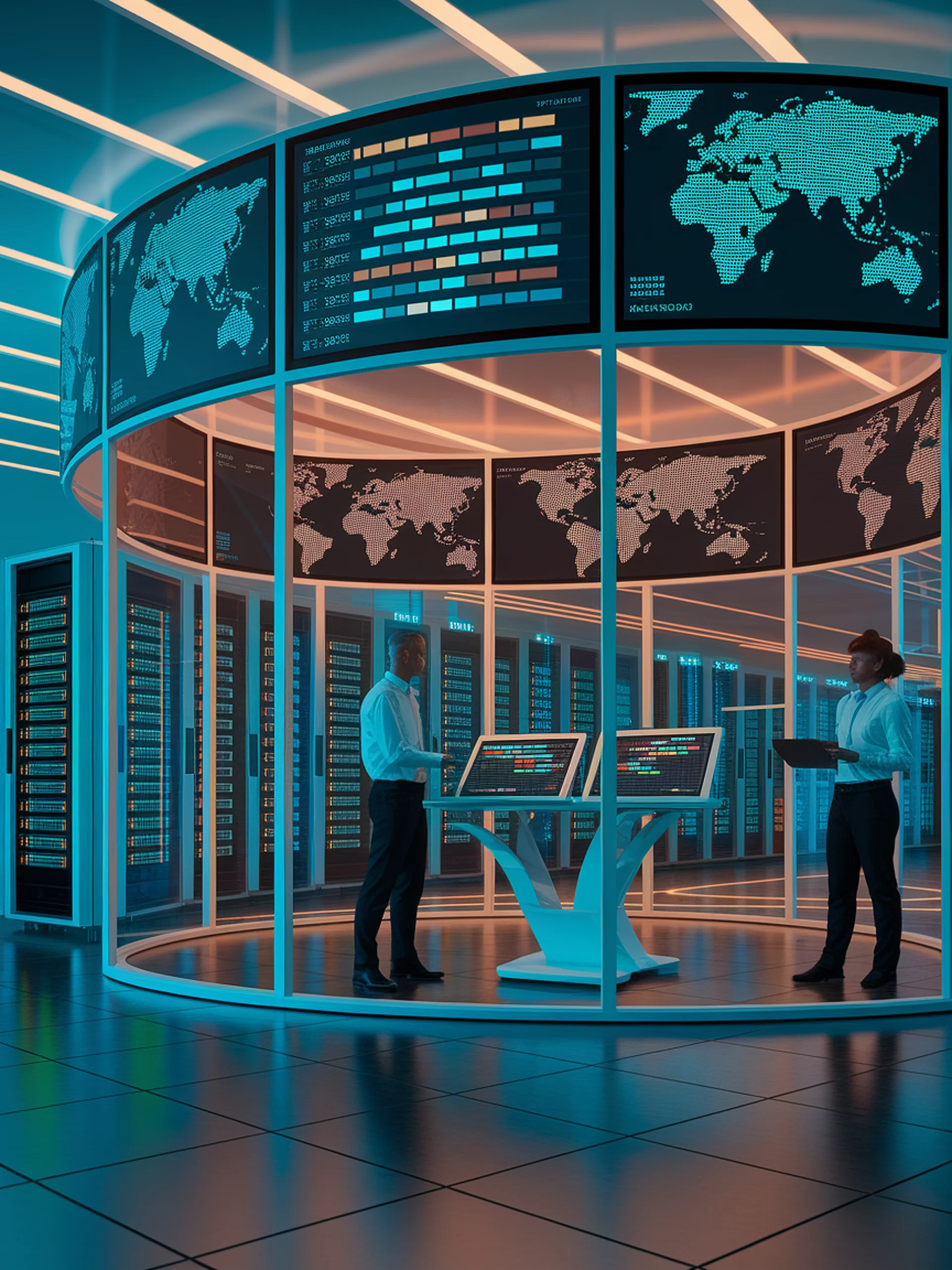
Accelerating LLM Training Across Distributed Data Centers
Layer-wise Scheduling for Efficient Data Parallel Training

Boosting LLM Efficiency with Smart Cache Management
Dynamic cache re-positioning for faster, more effective language models
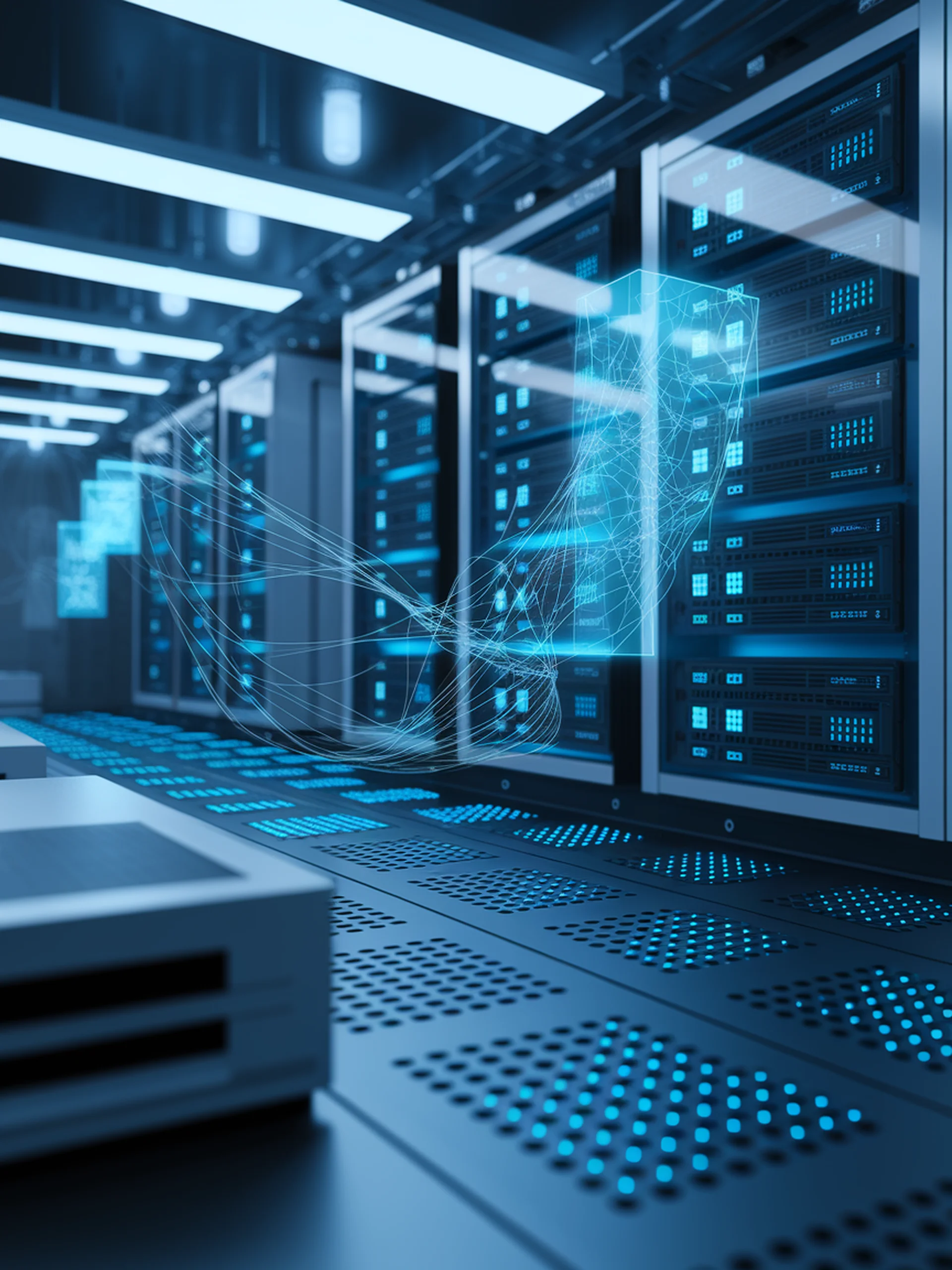
Faster Reasoning in LLMs
Breakthrough in Efficient Long-Decoding for Complex Tasks
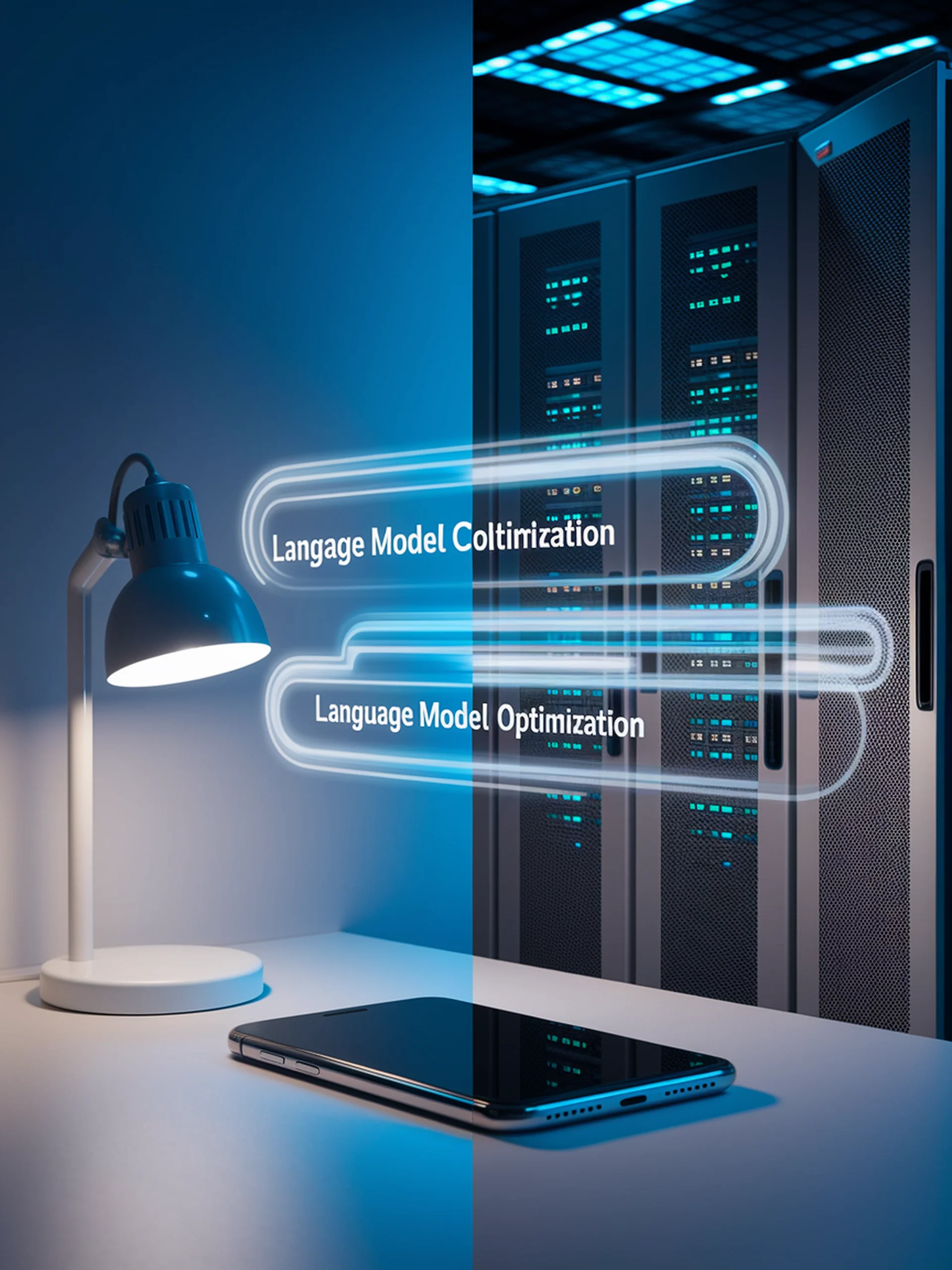
DiSCo: Optimizing LLM Text Streaming
A device-server collaborative approach for better performance and lower costs
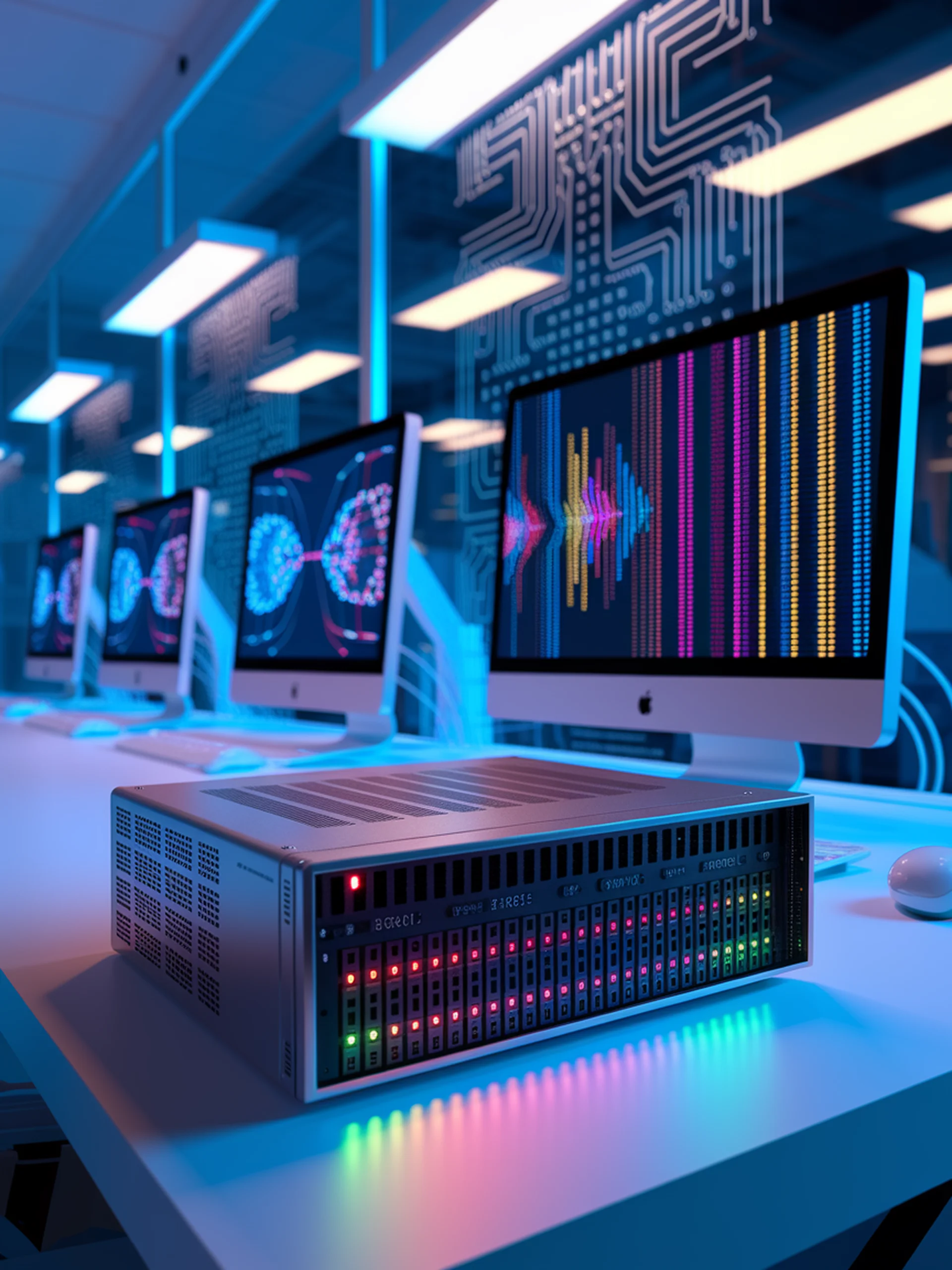
Efficient LLM Fine-Tuning for Resource-Constrained Teams
A Row-Based Sparse Approach to Reduce Memory & Computational Demands
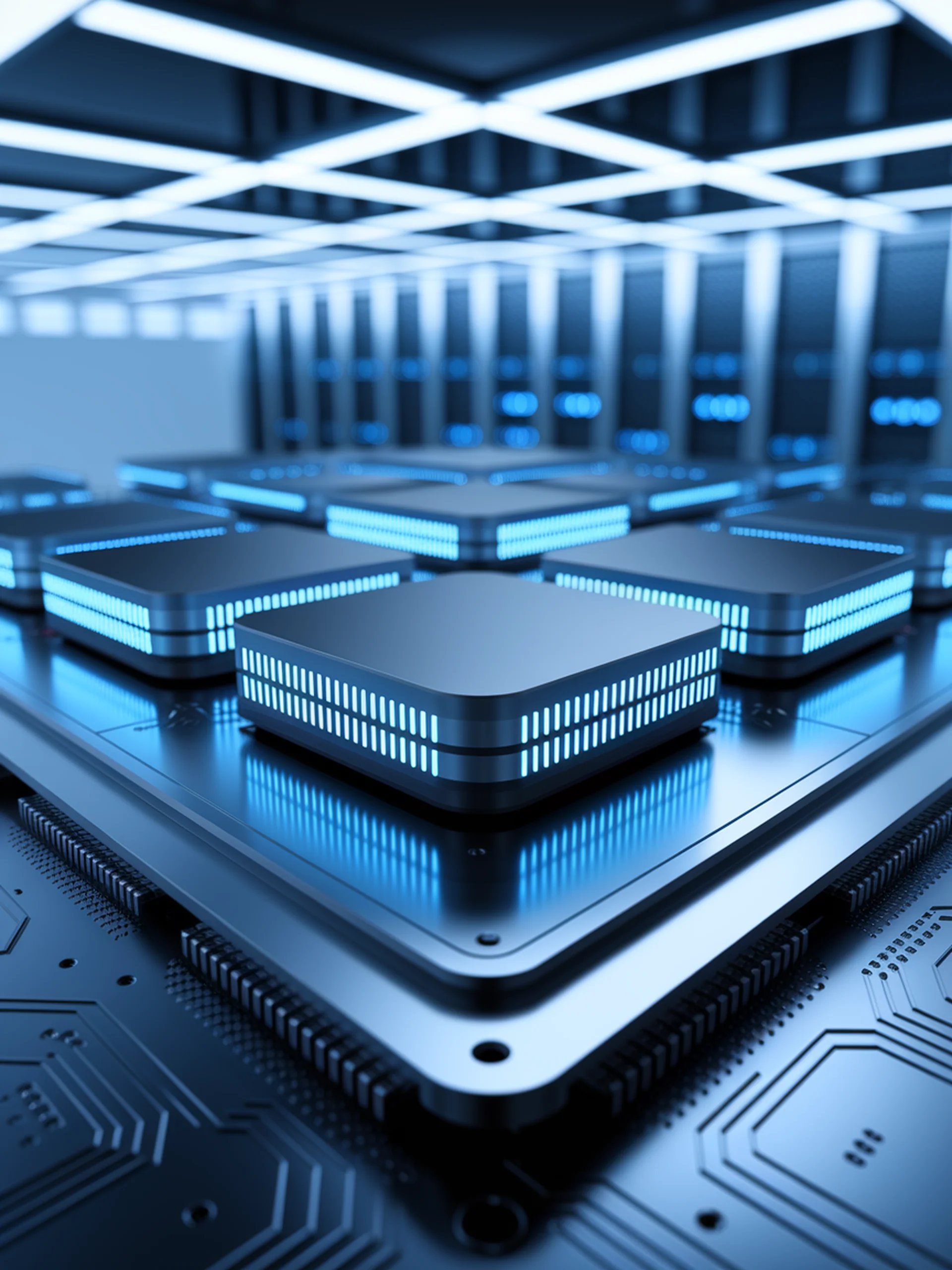
Breaking the Efficiency Barrier with FP4
Advancing LLM training with ultra-low precision quantization
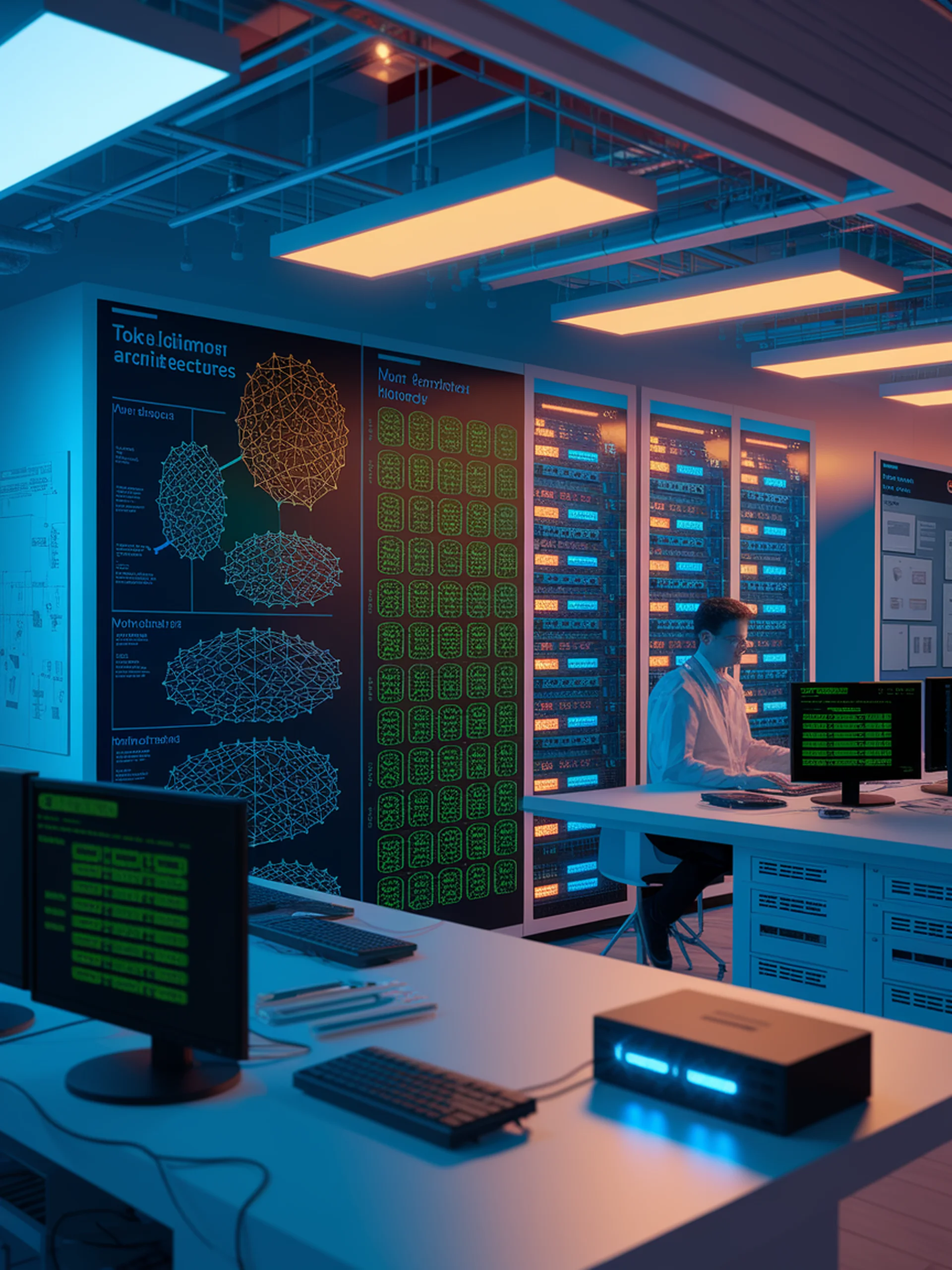
Rethinking Token Pruning in Multimodal LLMs
Why token importance may be the wrong focus for efficiency
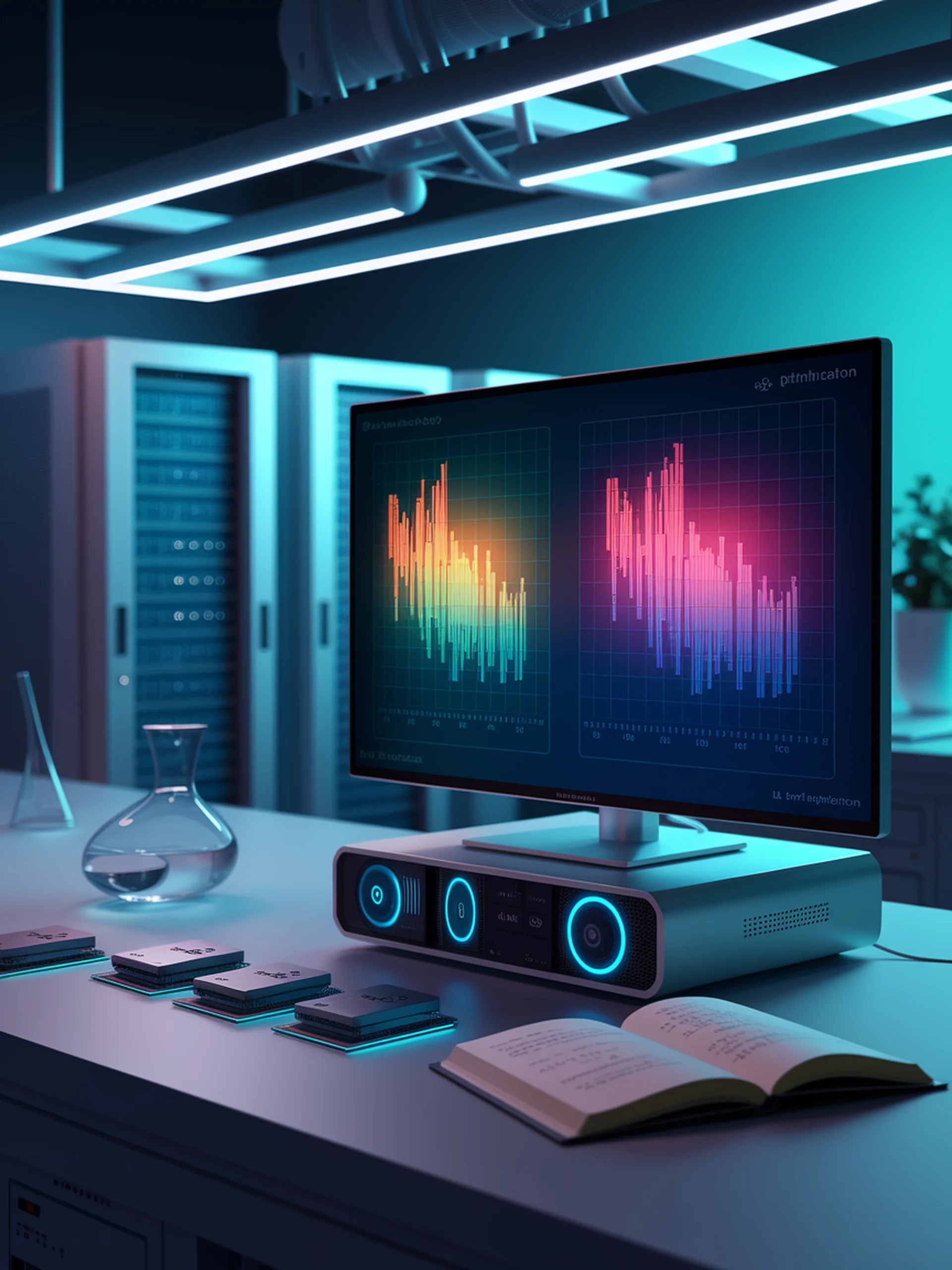
MaZO: Optimizing LLMs with Less Memory
A novel zeroth-order approach for multi-task fine-tuning
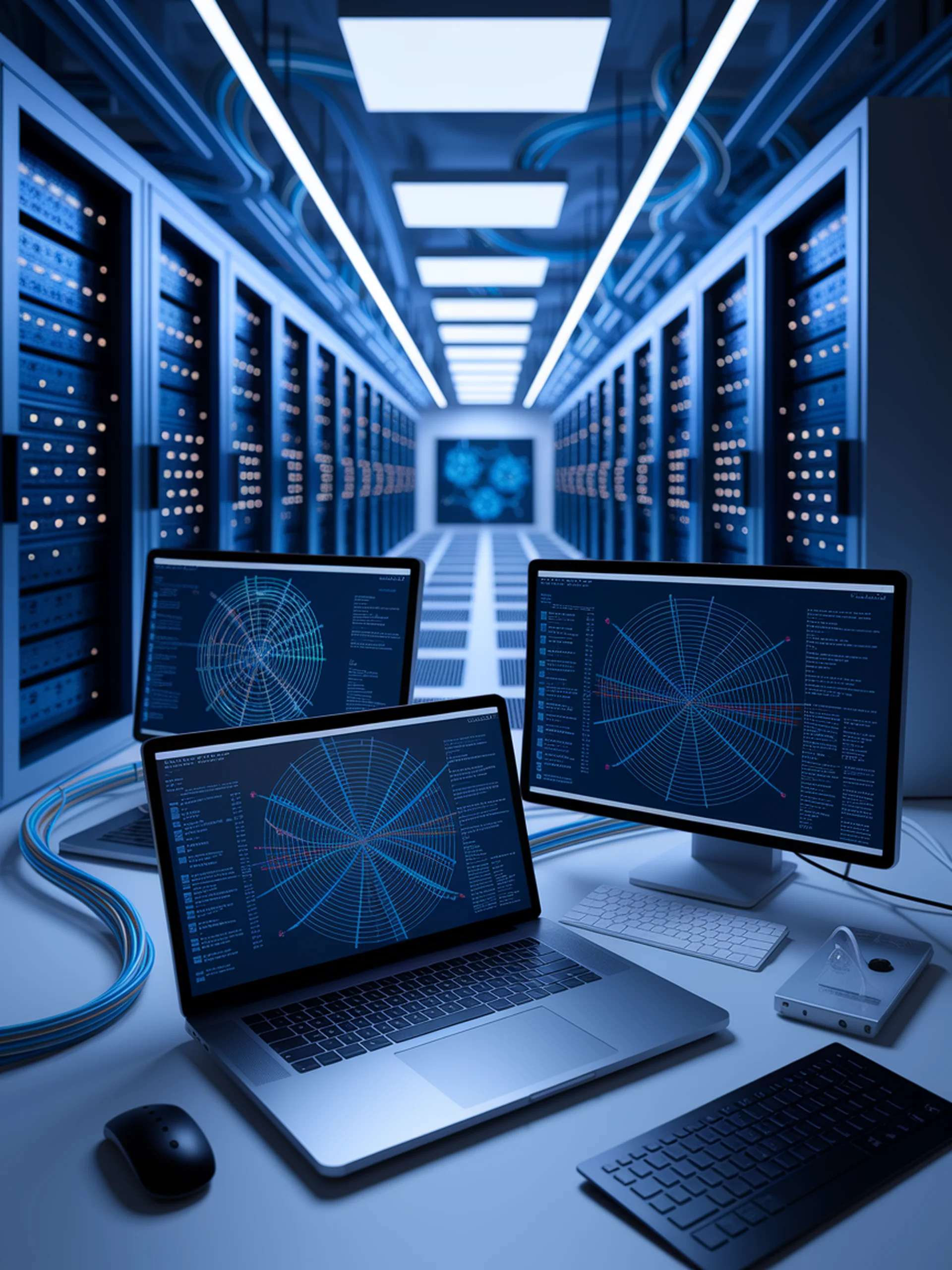
Learning to Keep a Promise: Scaling Language Model Decoding ...
By Tian Jin, Ellie Y. Cheng...
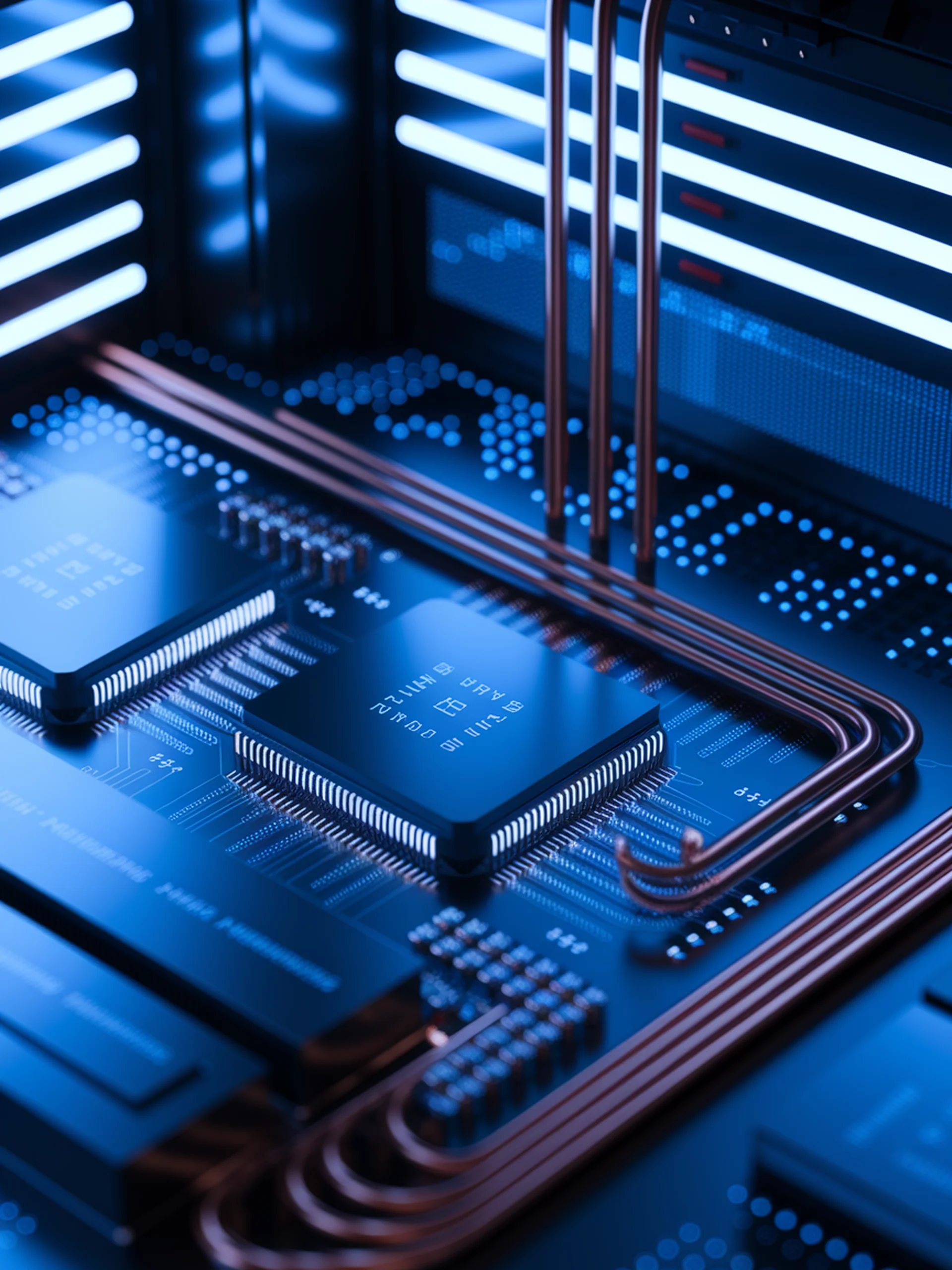
Accelerating LLM Performance
A Hardware-Software Co-Design Approach for Normalization Operations
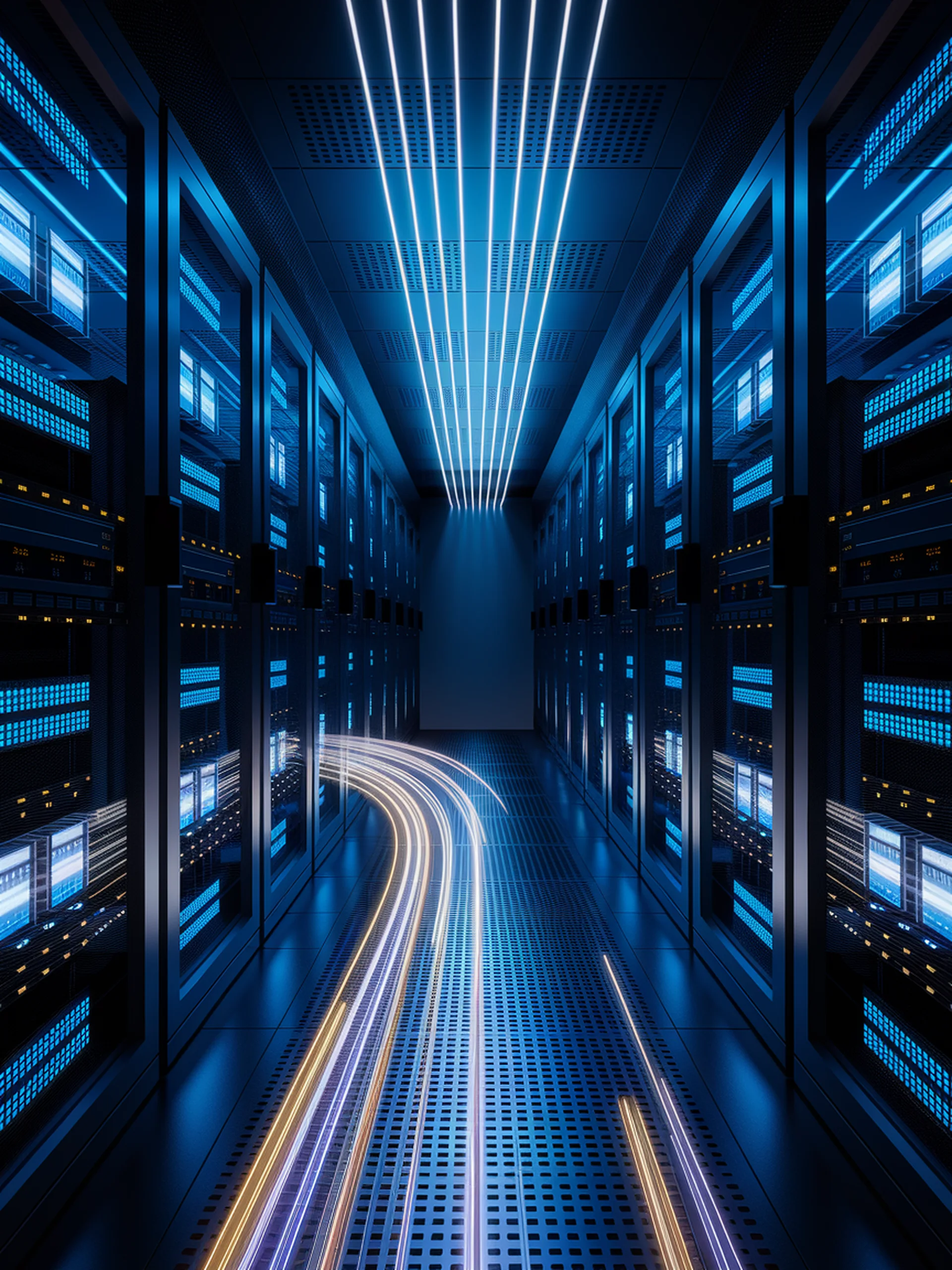
Breaking the Long-Context Bottleneck
Accelerating LLM inference by optimizing context block compression across GPUs
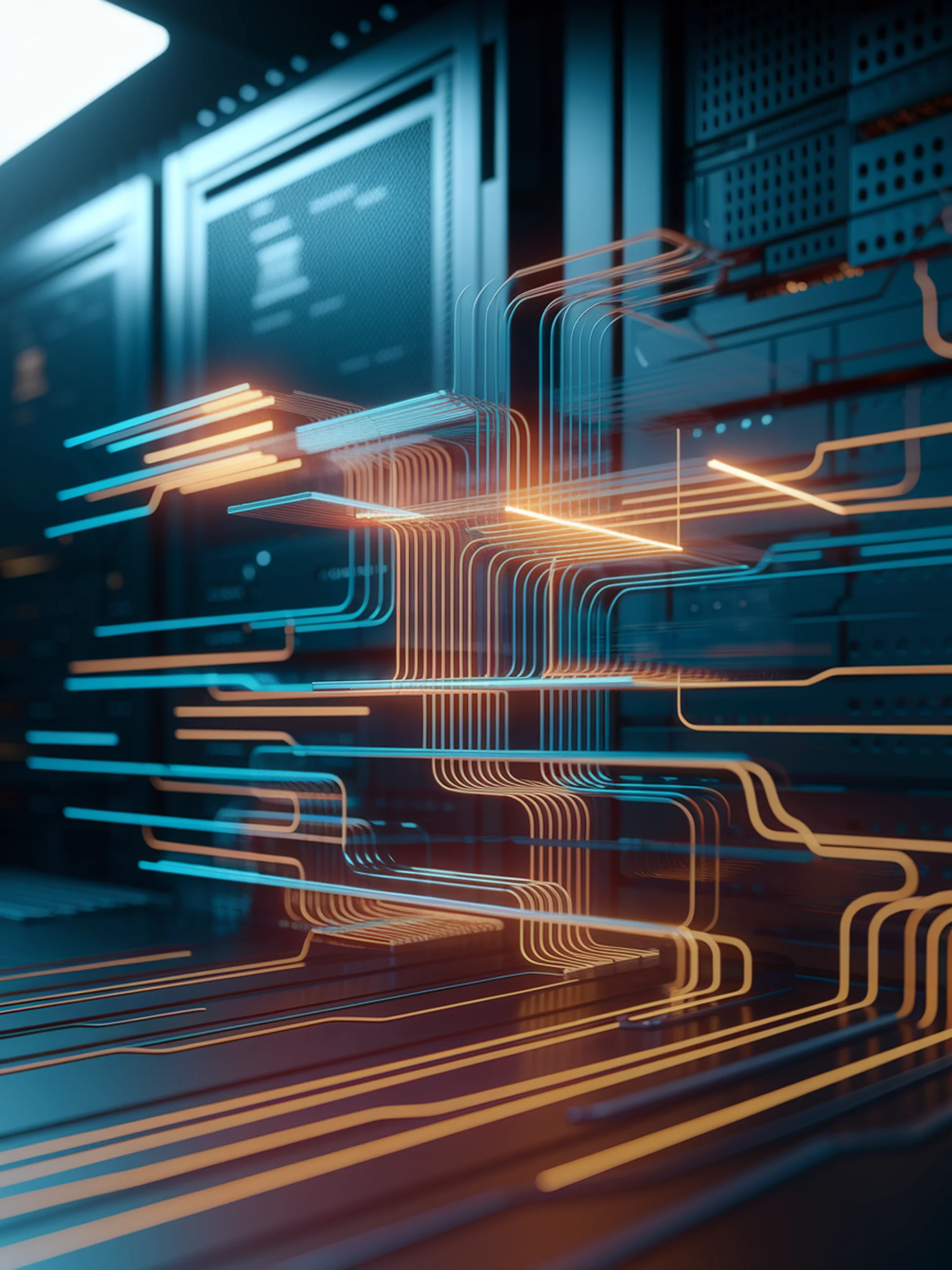
GoRA: Smarter Fine-Tuning for LLMs
Adaptive Low-Rank Adaptation with Gradient-Driven Optimization

Unlocking LLM Potential Without Adding Parameters
Enhancing model performance through cyclic parameter refinement
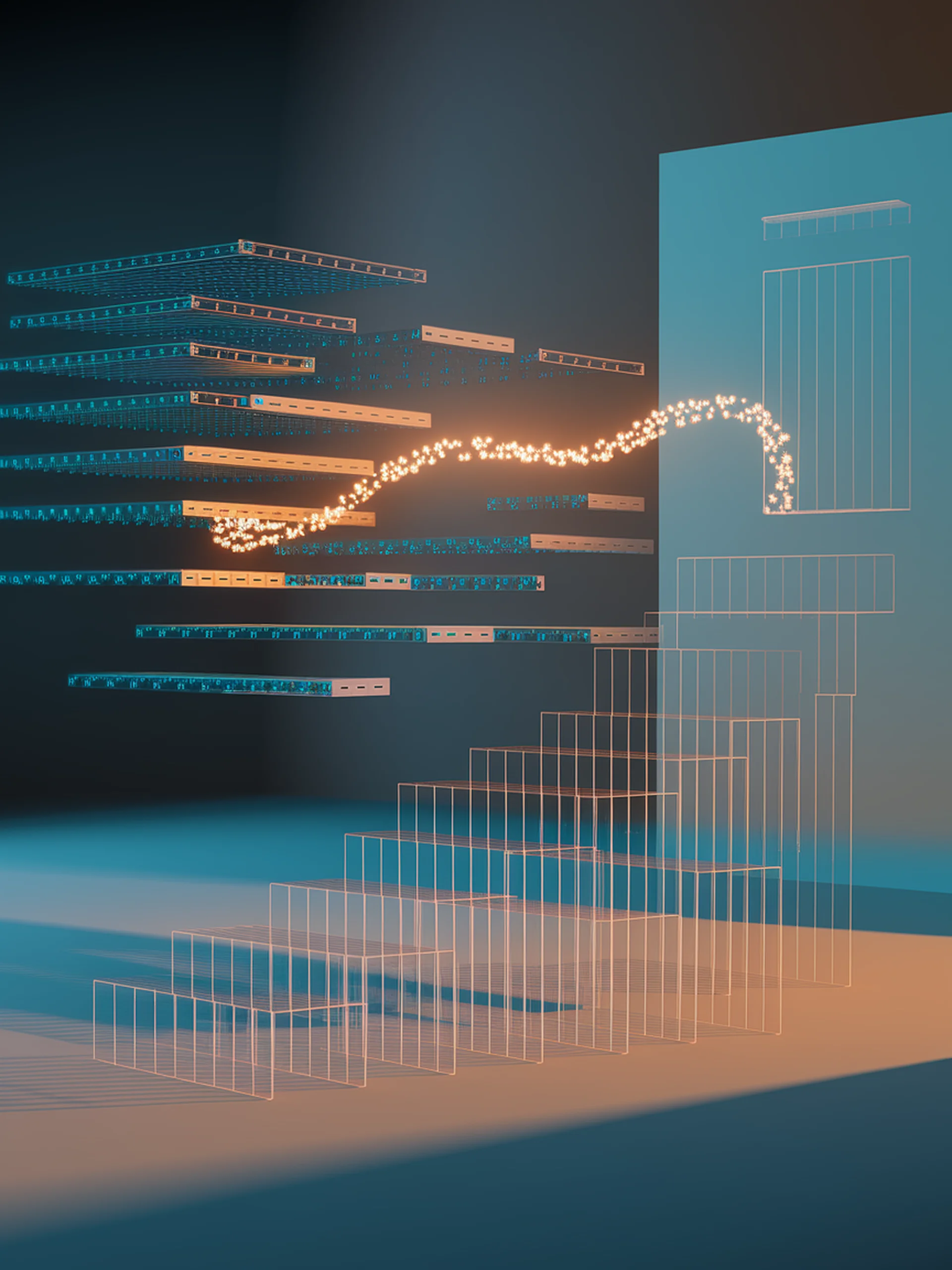
Adaptive Sparse Attention for Long-Context LLMs
Dynamic token selection that adapts to content importance
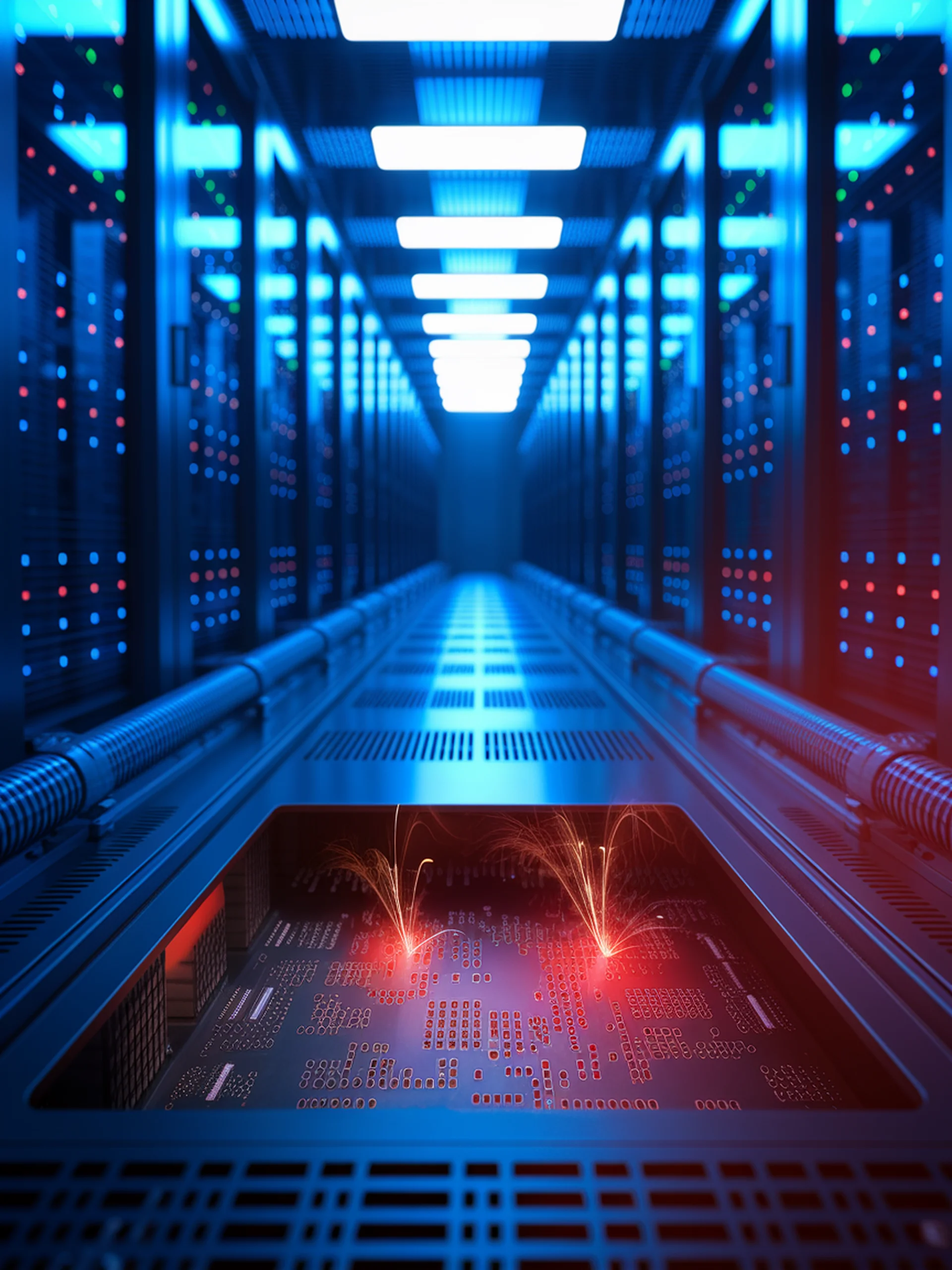
When Hardware Lies: Silent Data Corruption in LLM Training
First comprehensive analysis of hardware-induced corruption effects on large language models
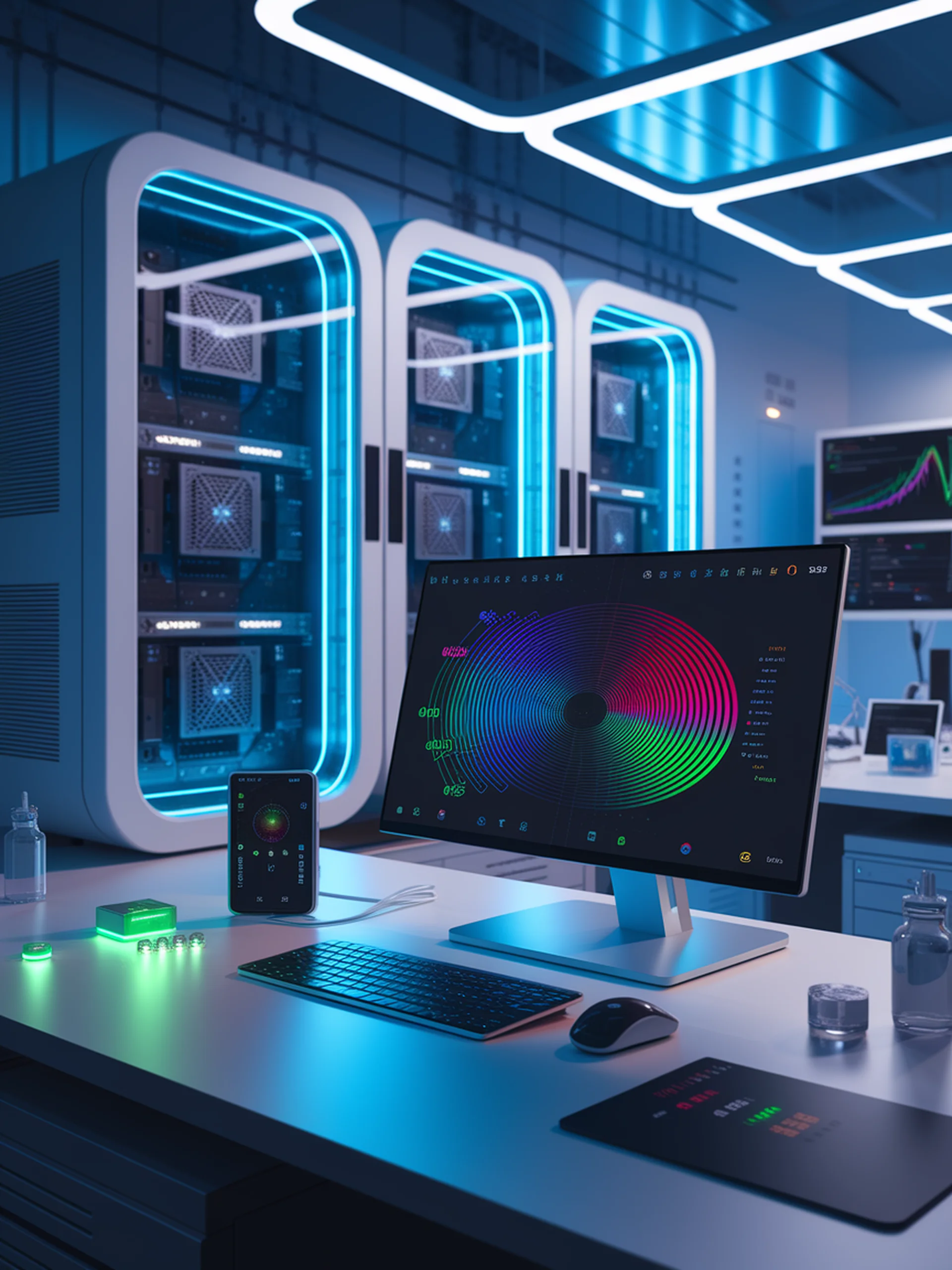
Optimizing LLMs in Low Precision
A Novel Framework for Quantized Fine-Tuning Without Backpropagation
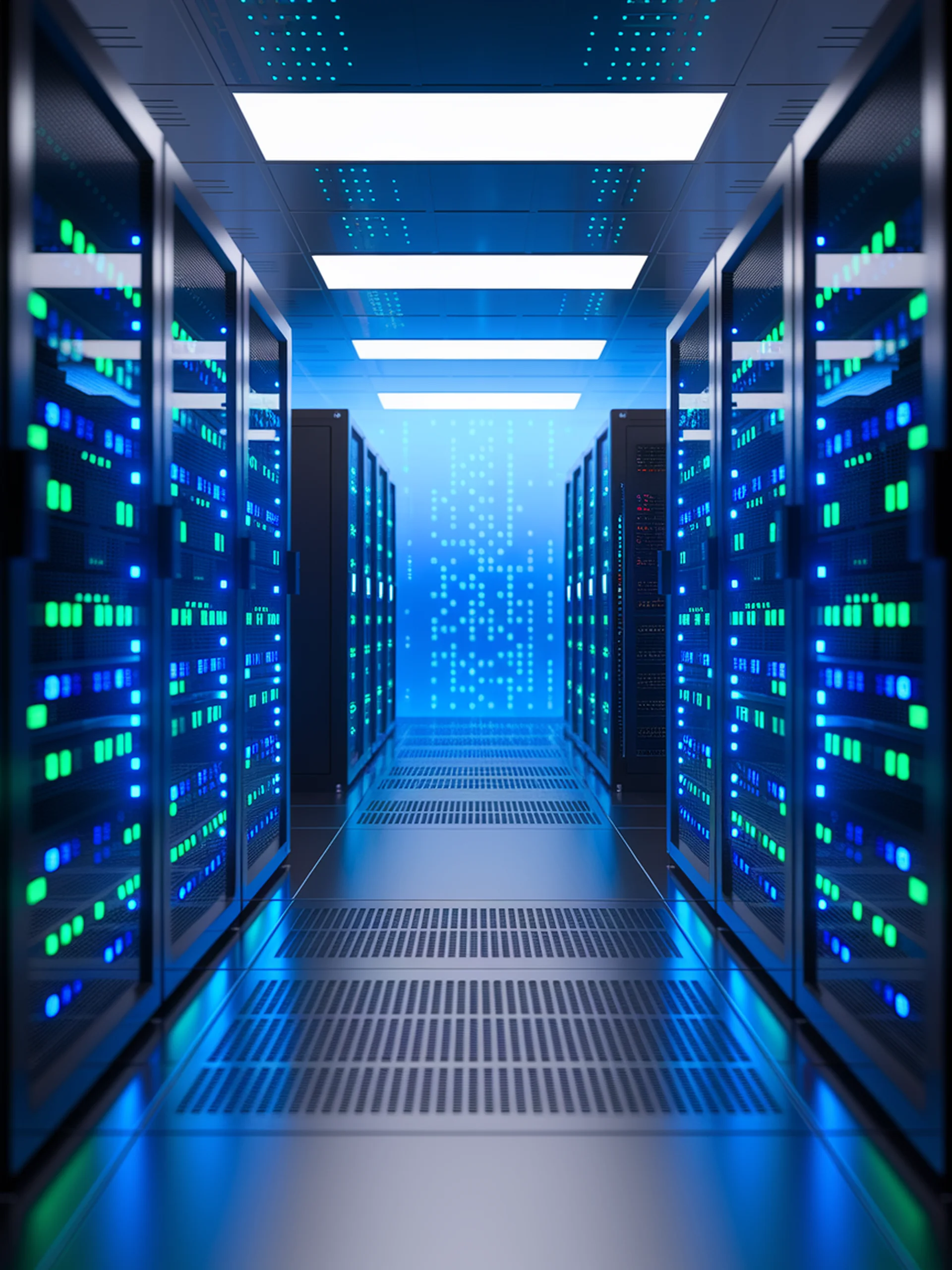
Making LLMs Leaner & Faster
Matrix-Partitioned Experts with Dynamic Routing
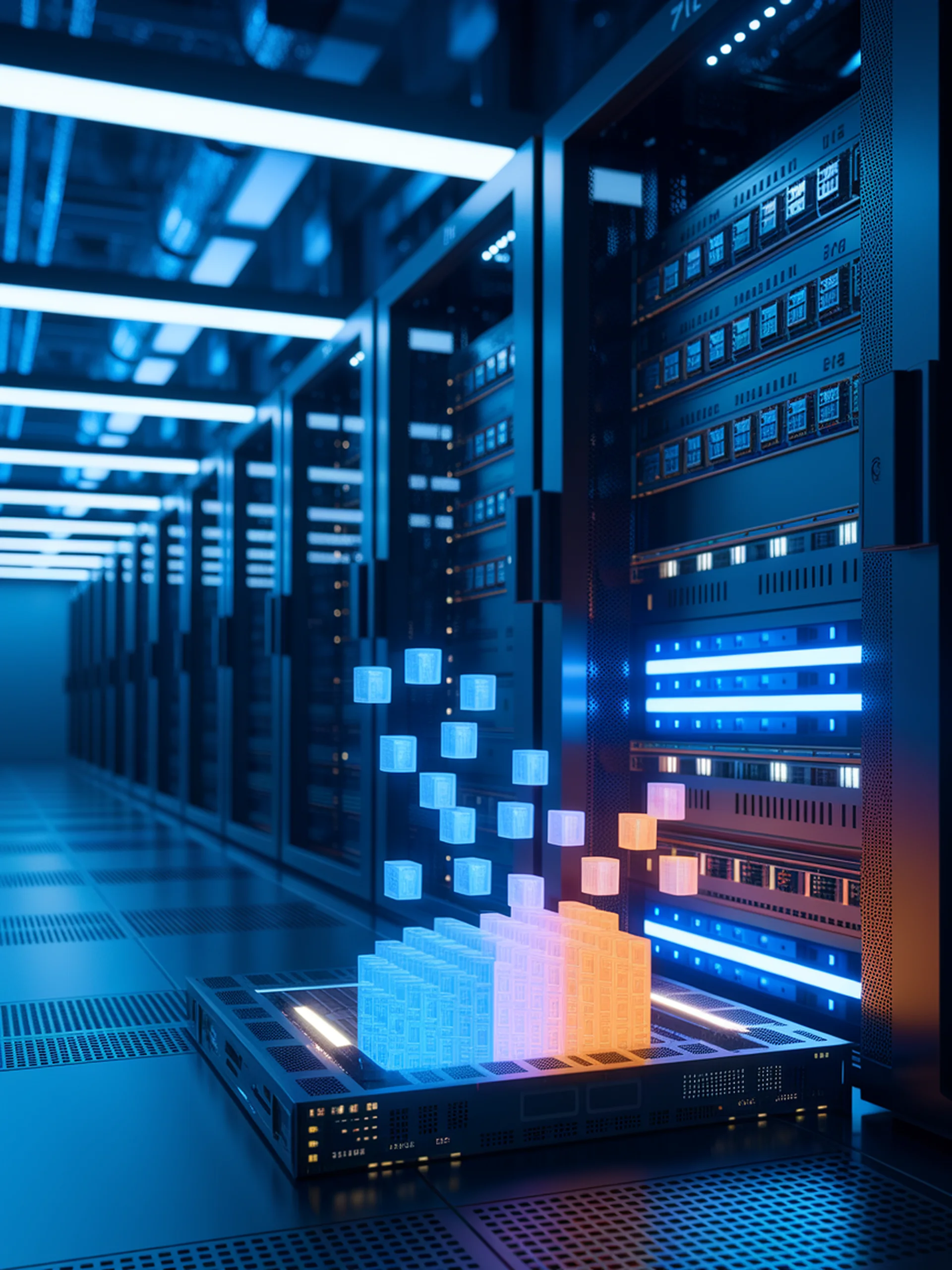
Memory-Efficient LLM Inference
Reducing GPU memory usage through head-wise KV cache offloading
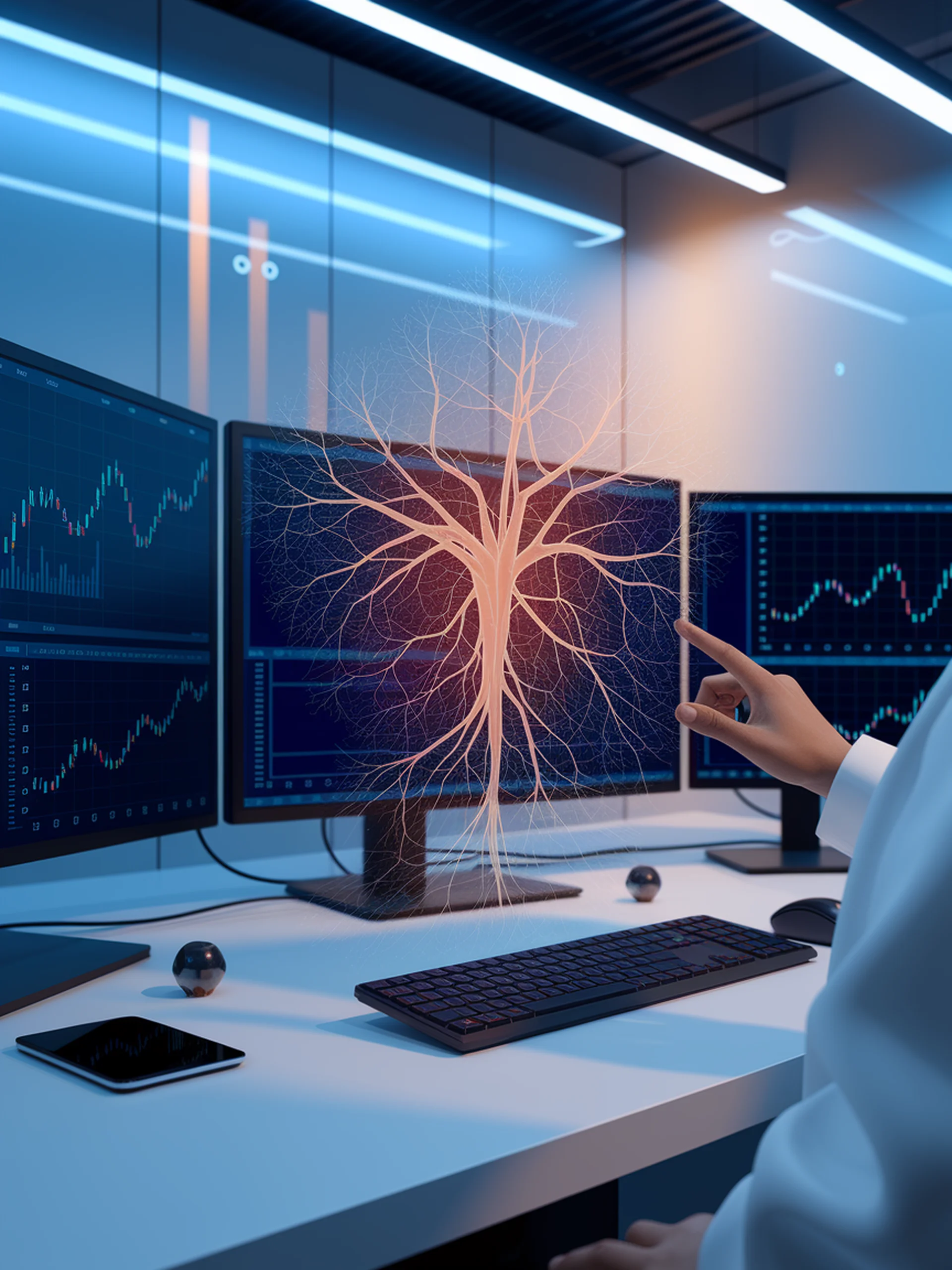
Smarter Recovery for Pruned LLMs
Efficient data selection for recovering pruned language models
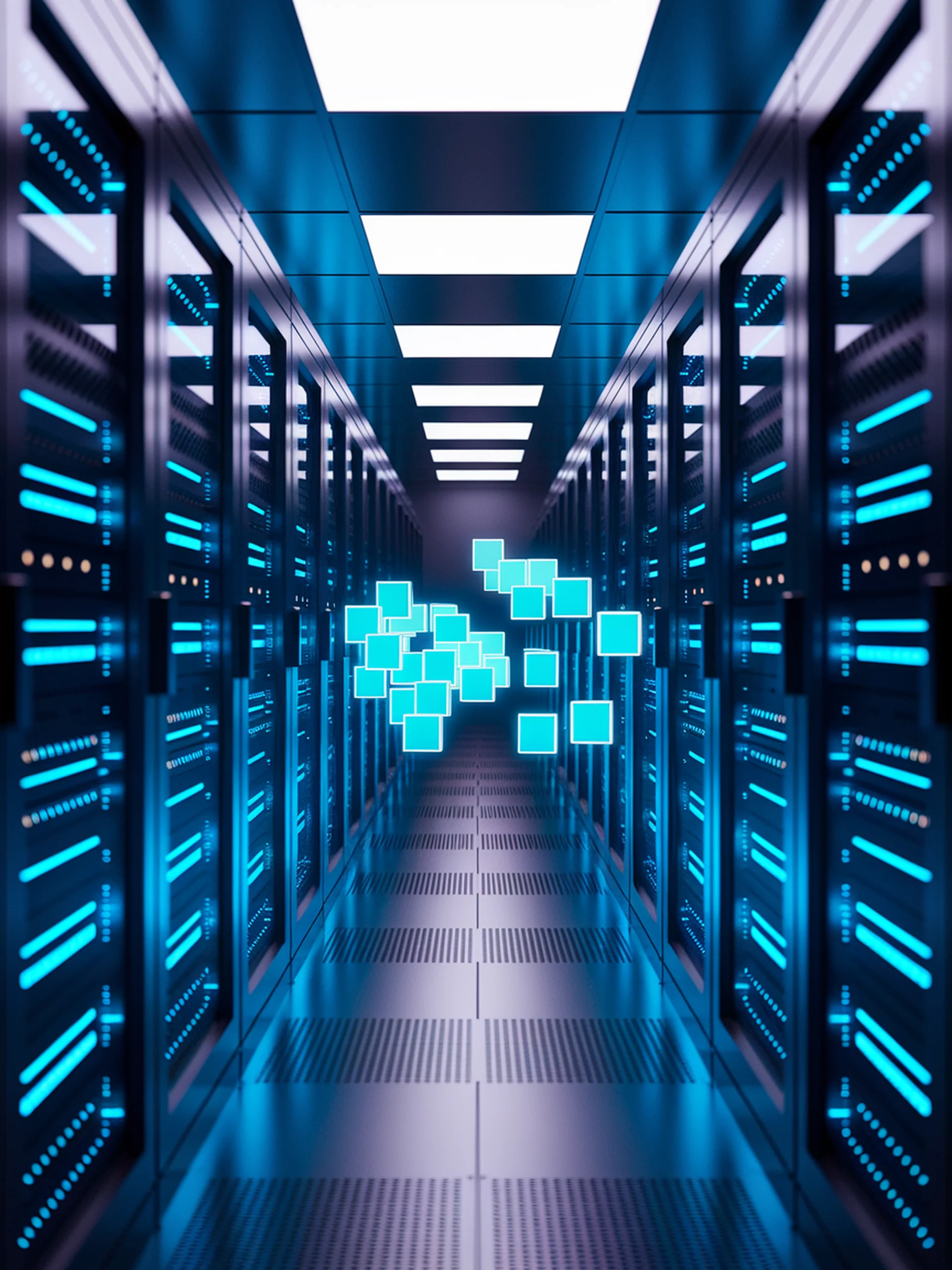
Making LLMs Faster with Smarter Memory Management
Novel techniques to reduce KV cache overhead for long-context models
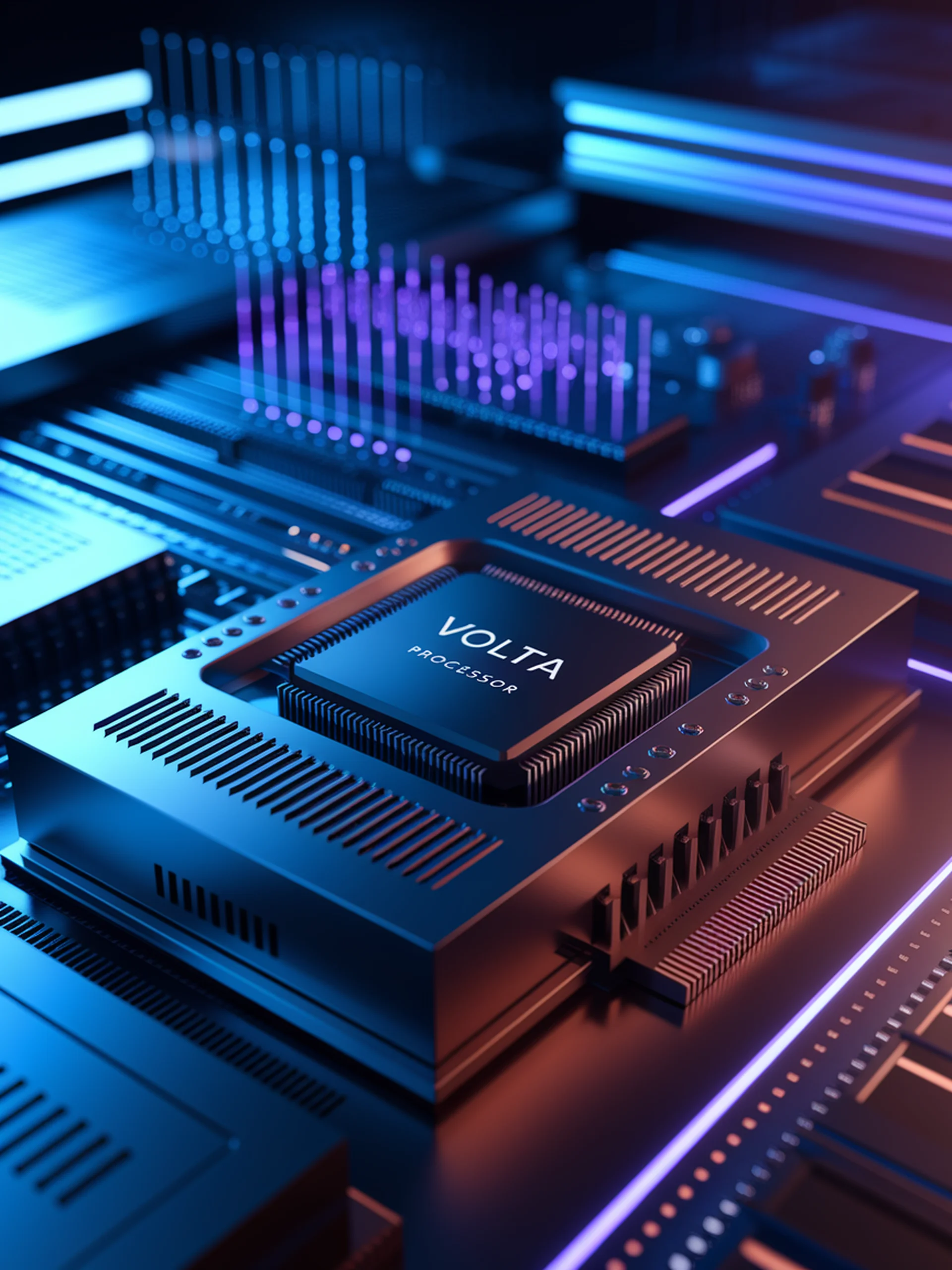
Accelerating AI with SparkAttention
Optimizing Multi-Head Attention for Volta GPUs
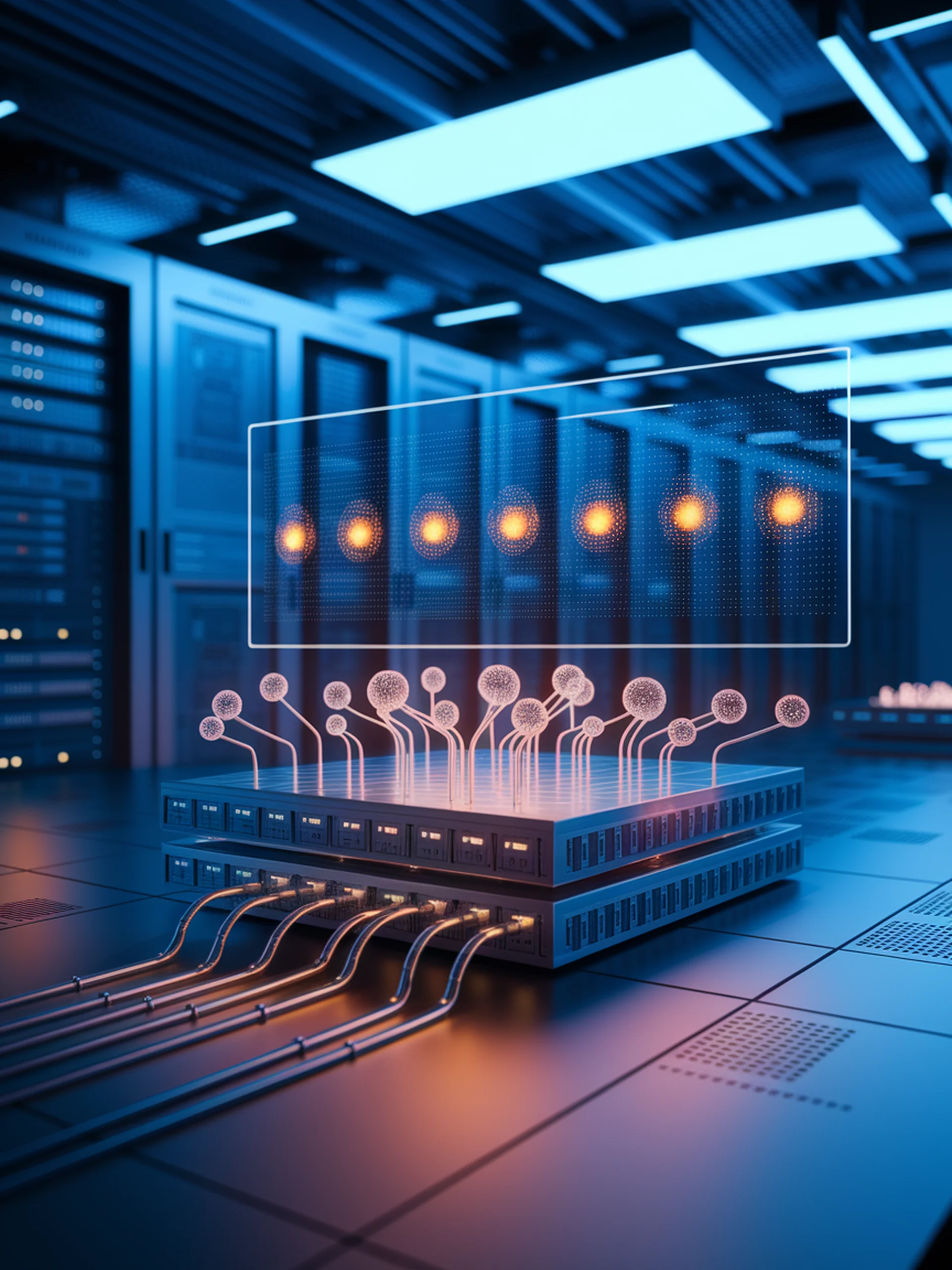
Overcoming Sparse Activation in LLMs
Enhancing model efficiency with multi-layer expert systems
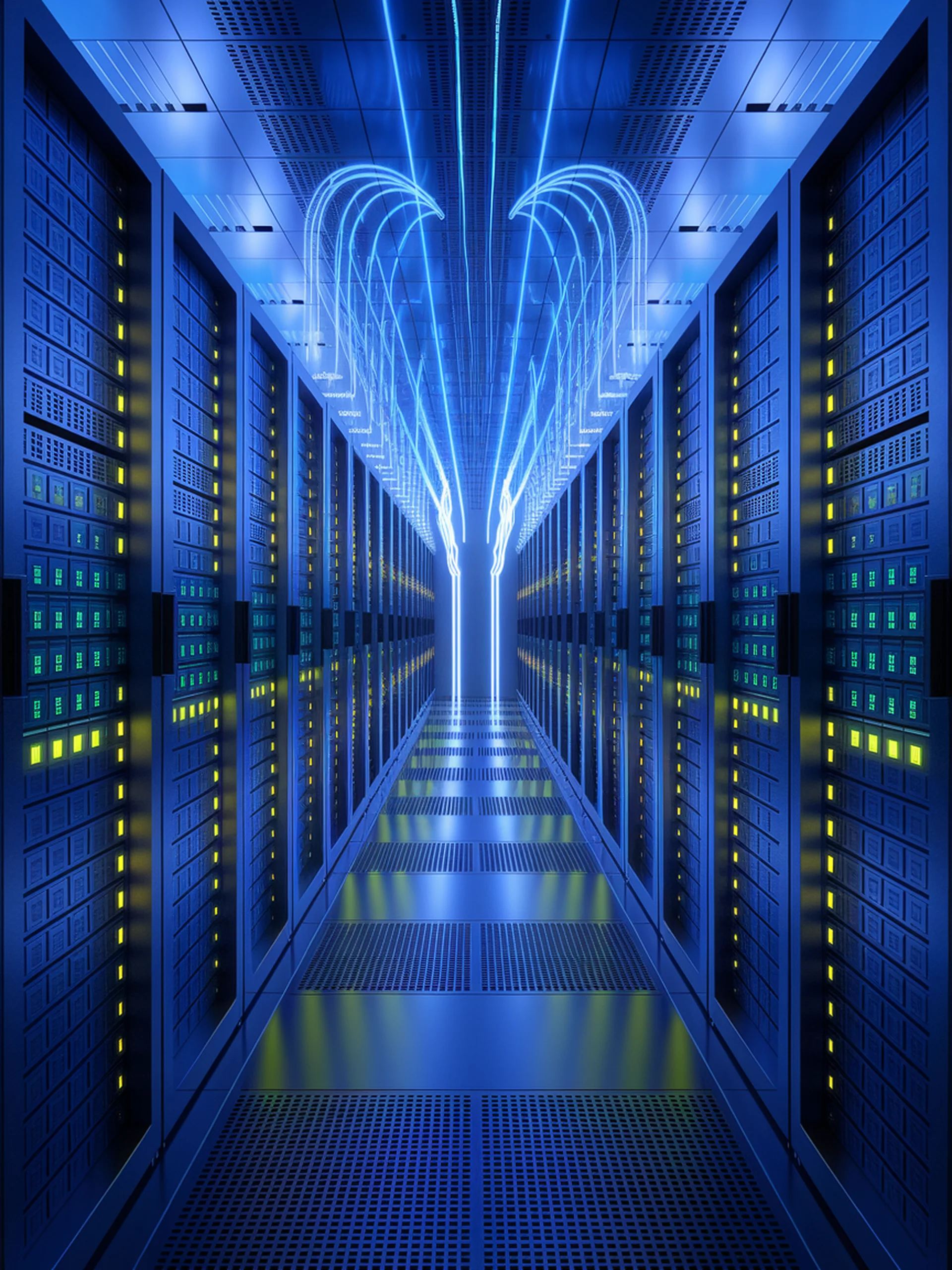
Accelerating Distributed AI Training
Efficient Communication-Computation Overlap in DiLoCo

Optimizing Memory for LLM Inference
Intelligent KV-cache allocation for longer contexts with less memory
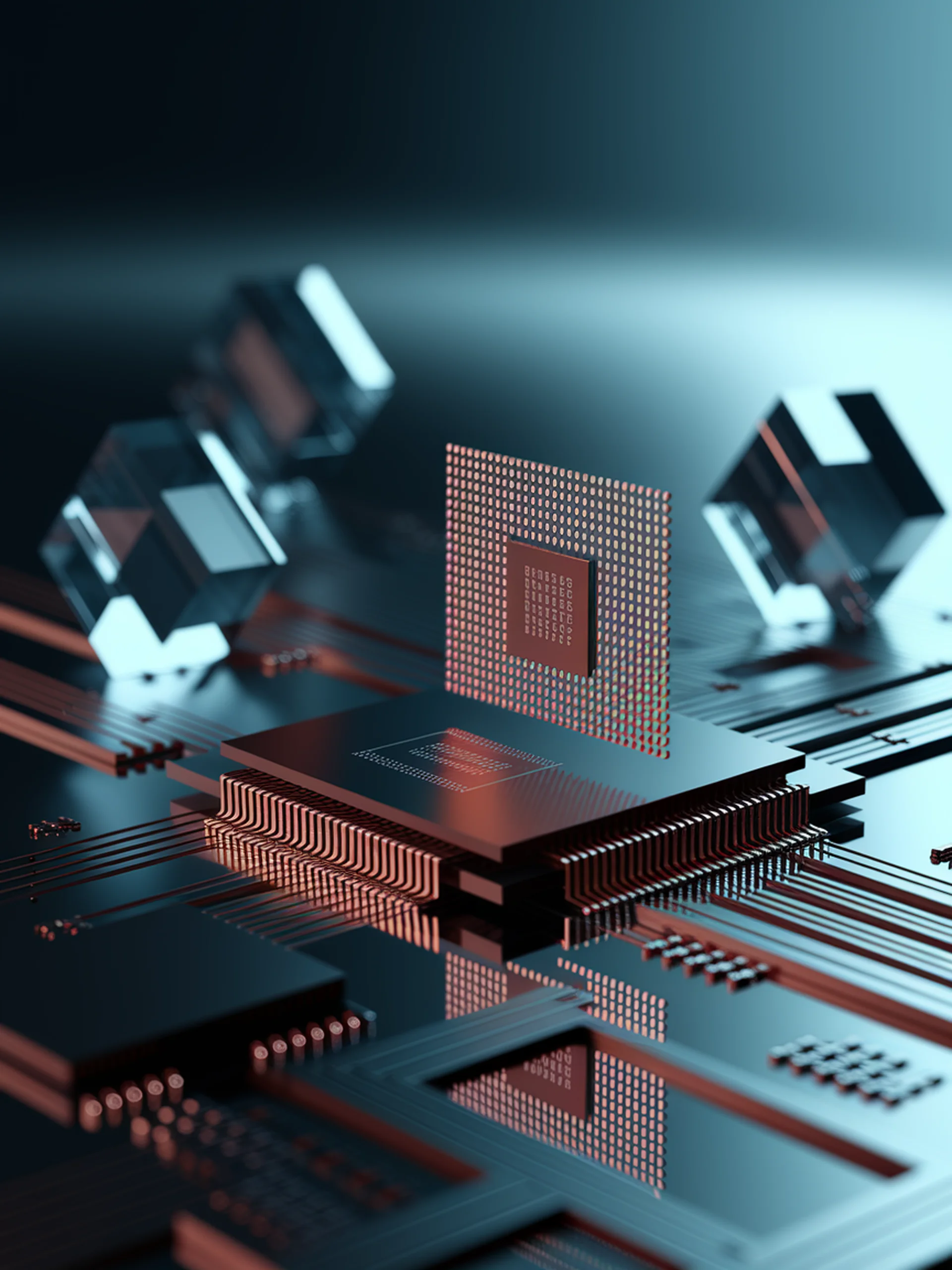
Optimizing LLMs Through Quantization
A Comprehensive Analysis of Post-Training Quantization Strategies
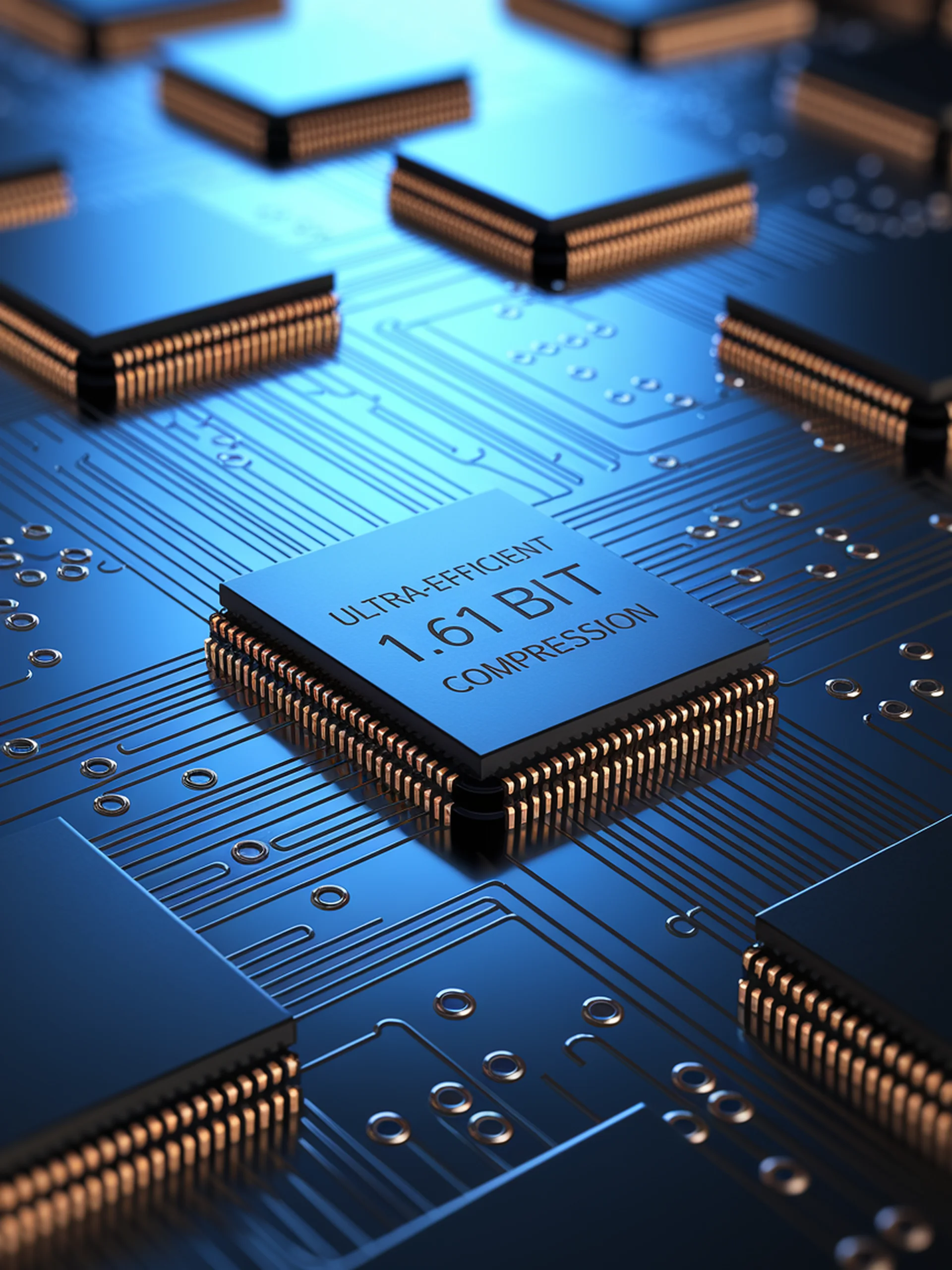
Breaking the 2-bit Barrier in LLM Compression
Pushing the limits of model efficiency with PTQ1.61
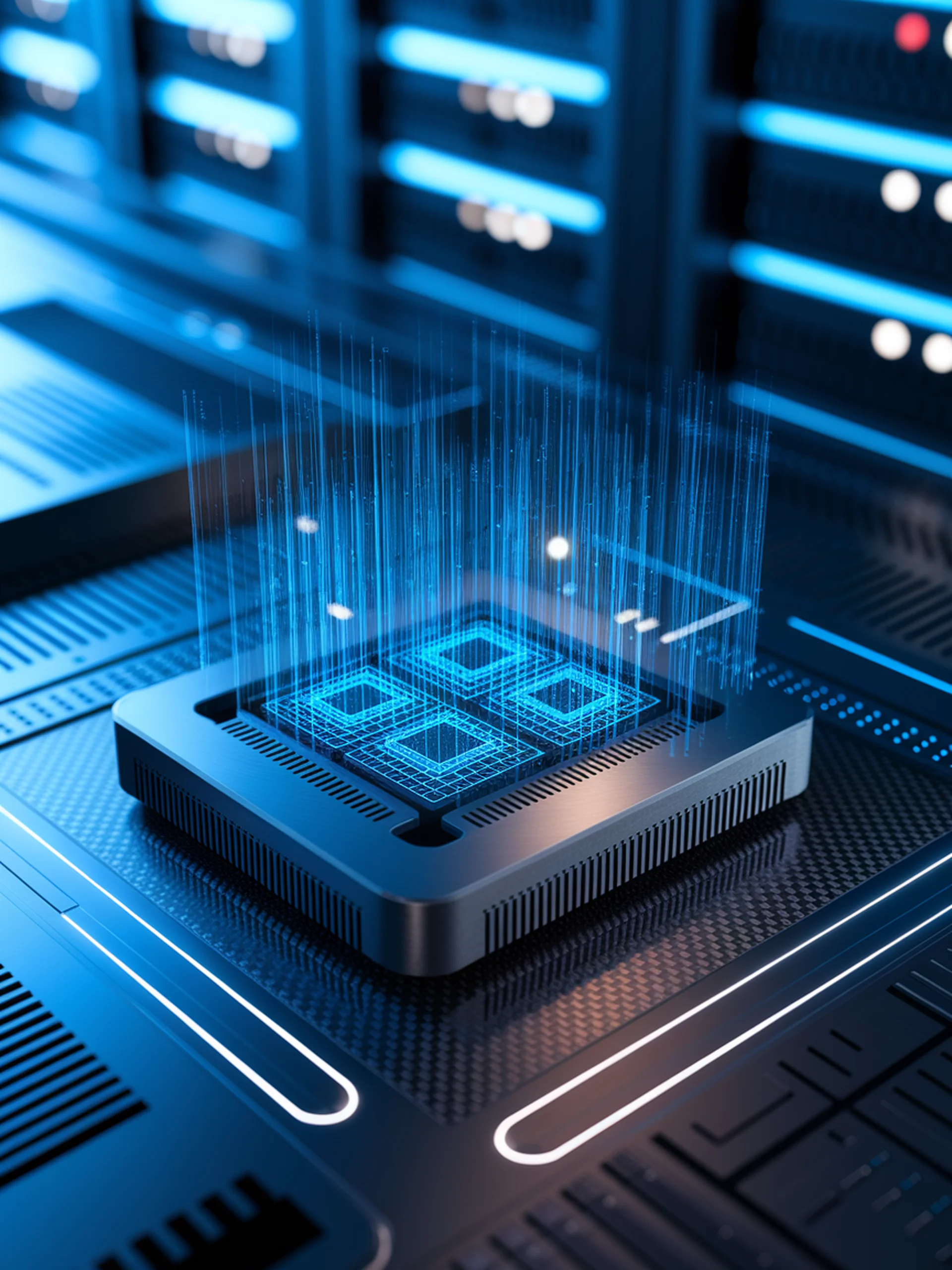
Streamlining LLMs with Tensor Operators
How PLDR-LLMs can replace their own neural networks at inference time
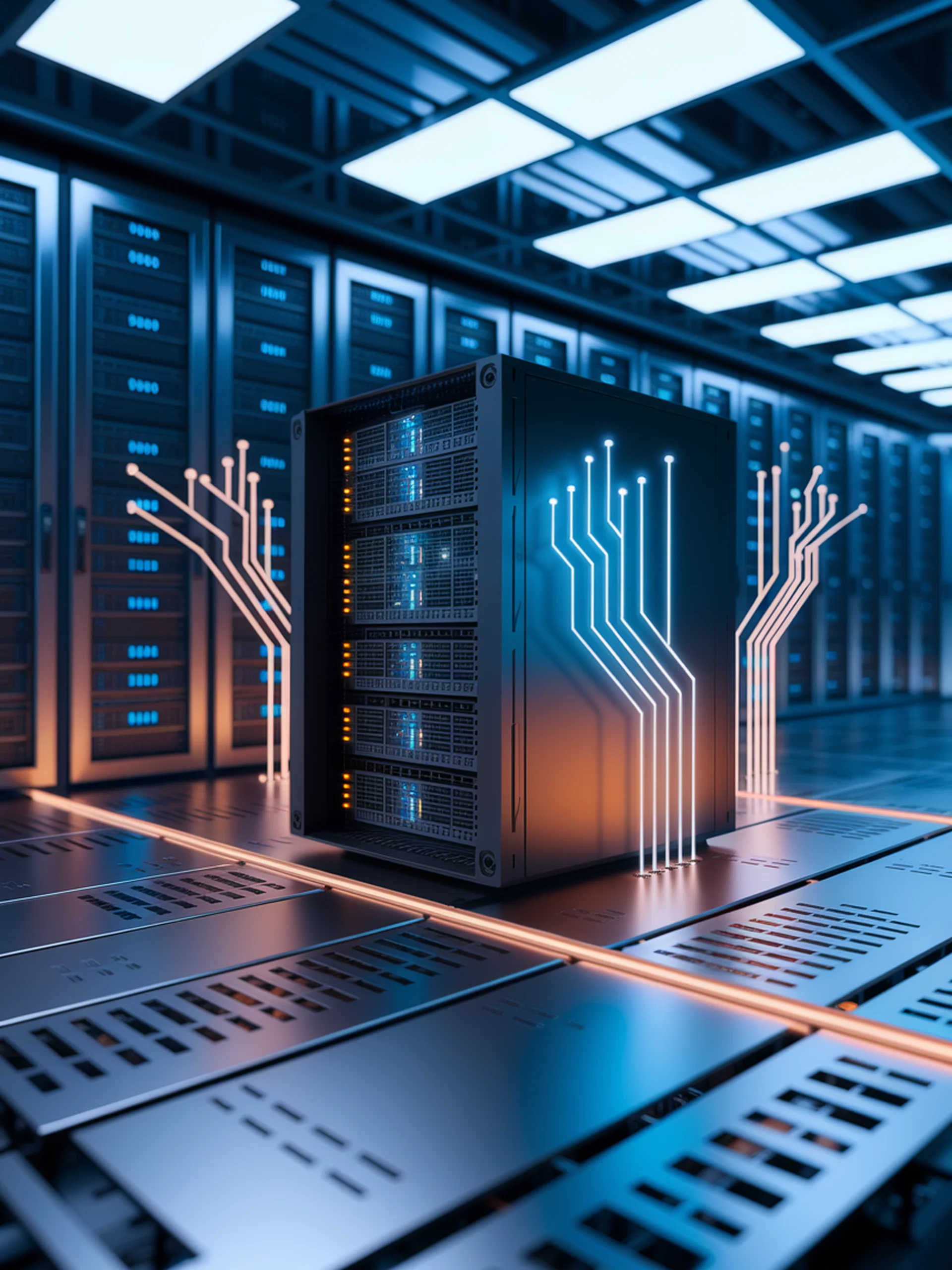
Accelerating LLM Inference with Smart Prediction
C2T: A classifier-based approach to optimize speculative decoding
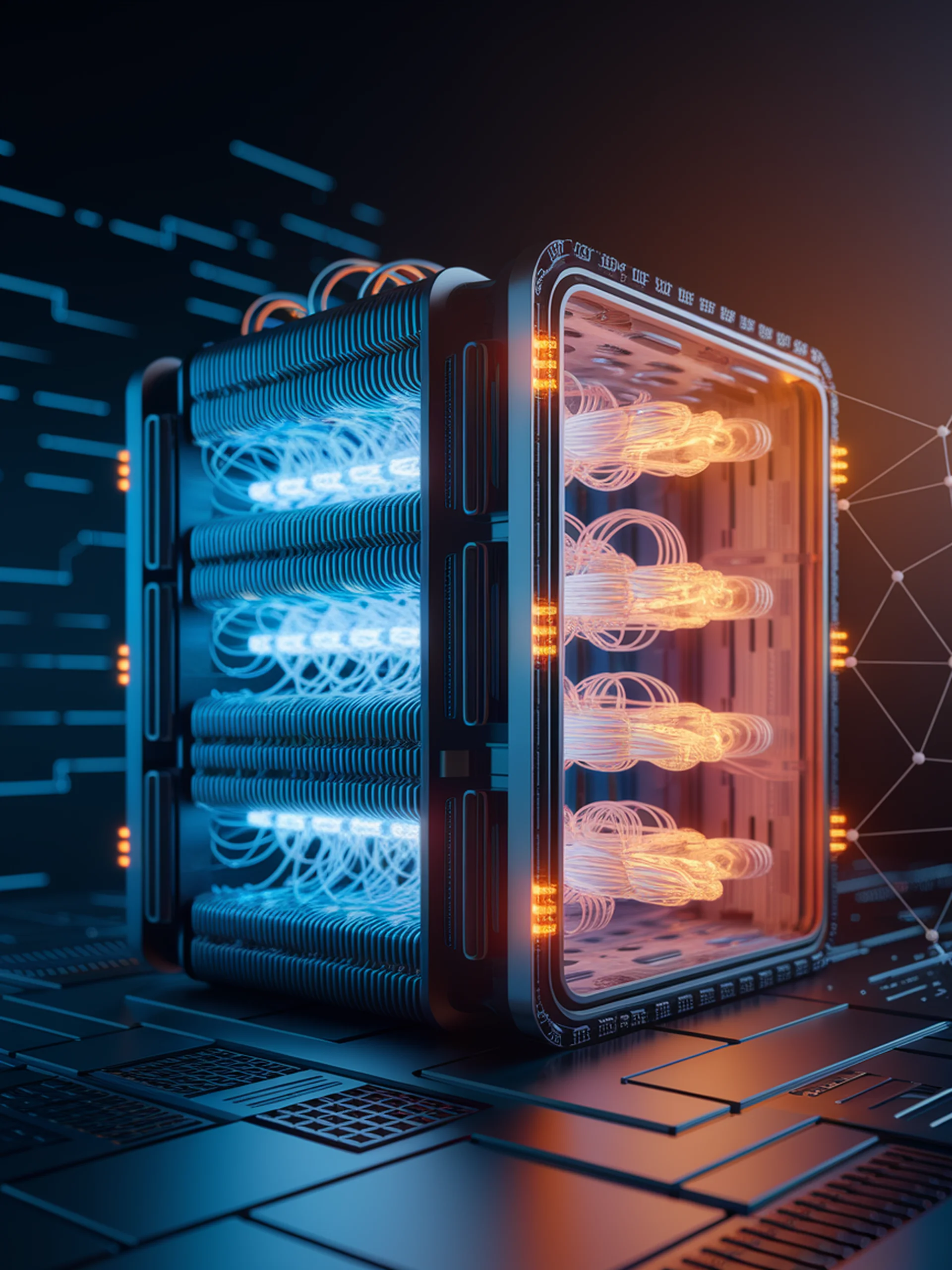
Adaptive Depth Scaling in LLMs
Enhancing reasoning capabilities through dynamic computation allocation
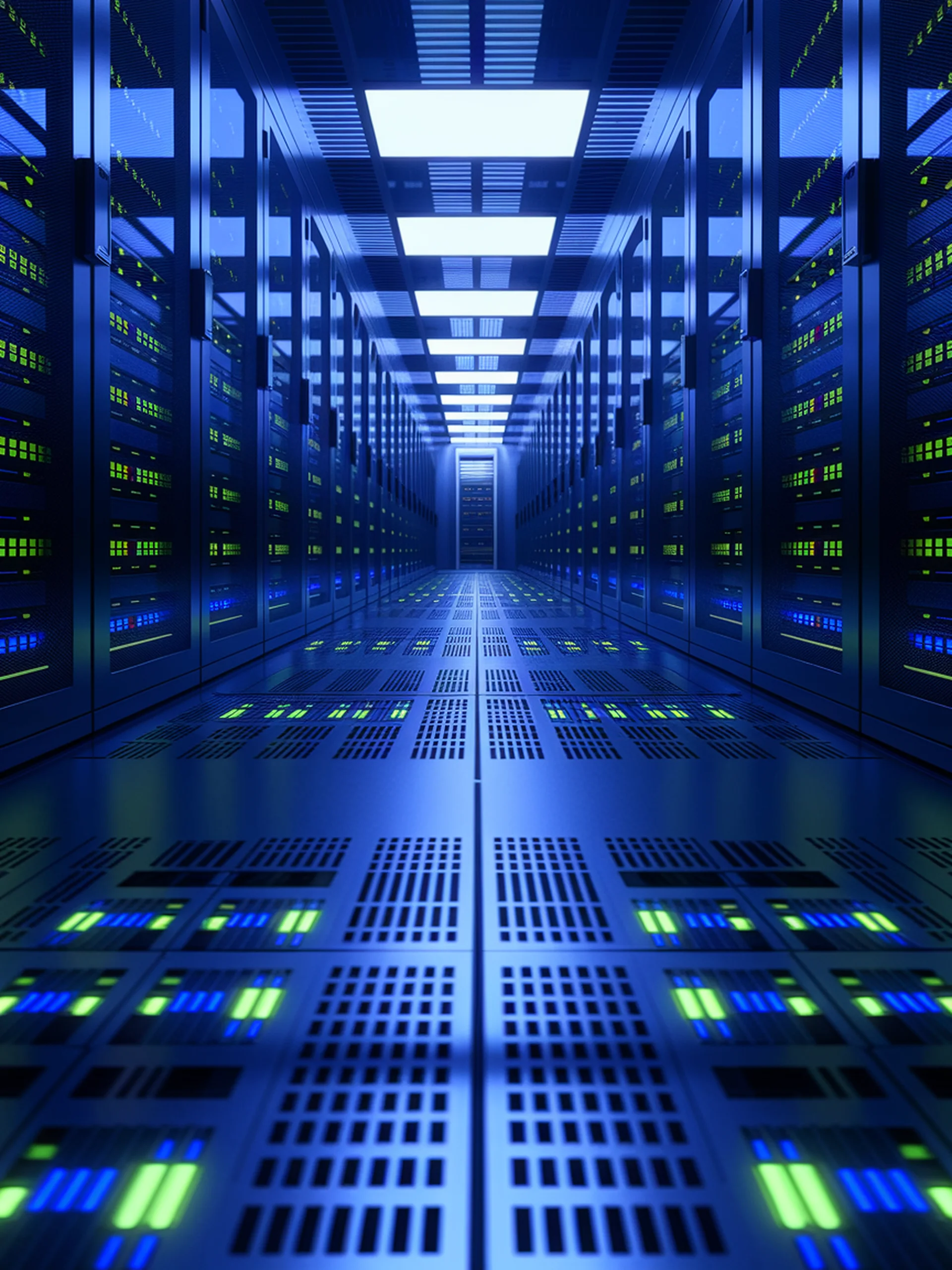
Optimizing LLM Agent Infrastructure
A new serving engine for efficient execution of AI agent programs
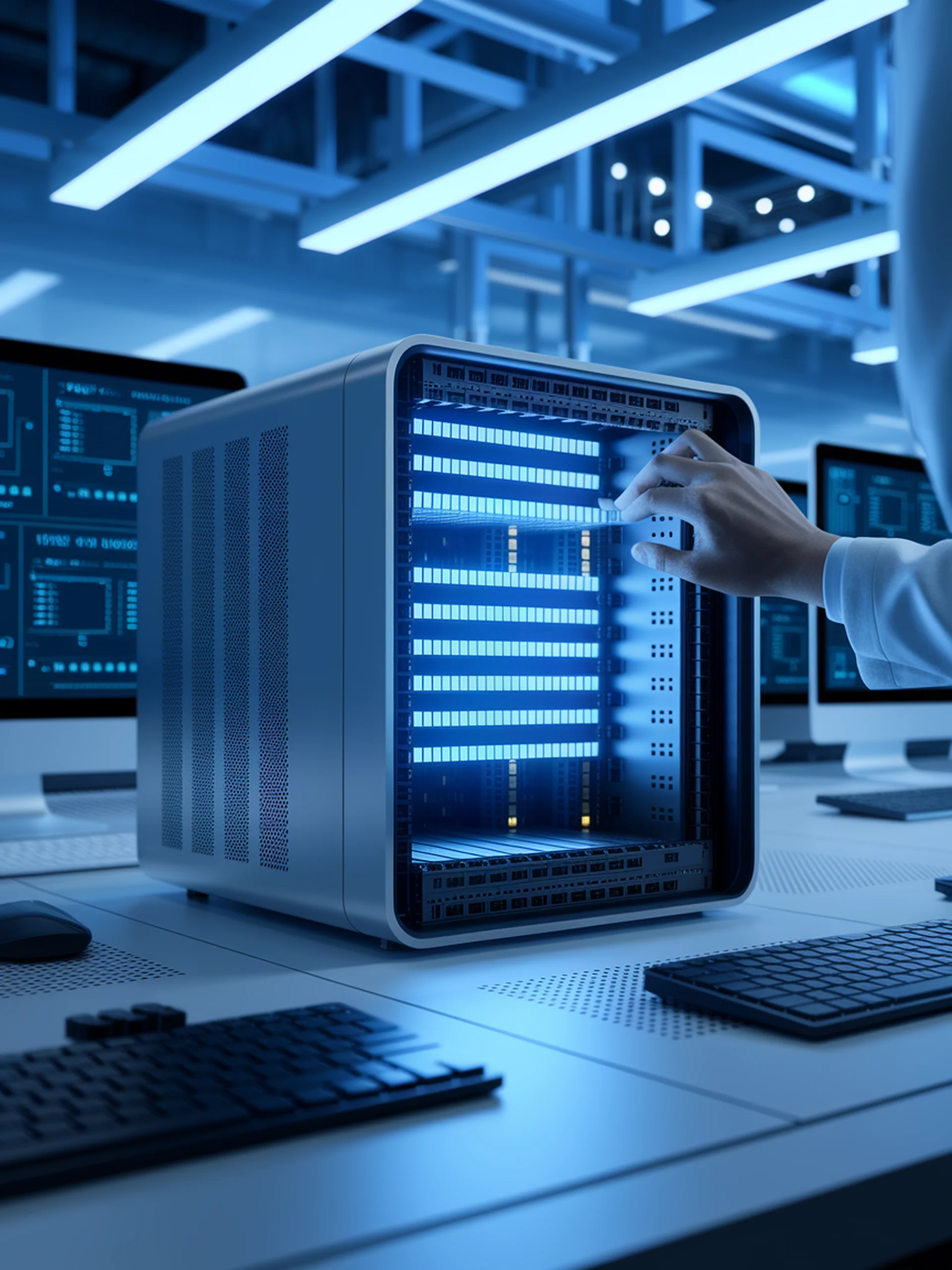
Smarter LLM Compression with MaskPrune
Achieving efficient pruning while maintaining uniform layer structure
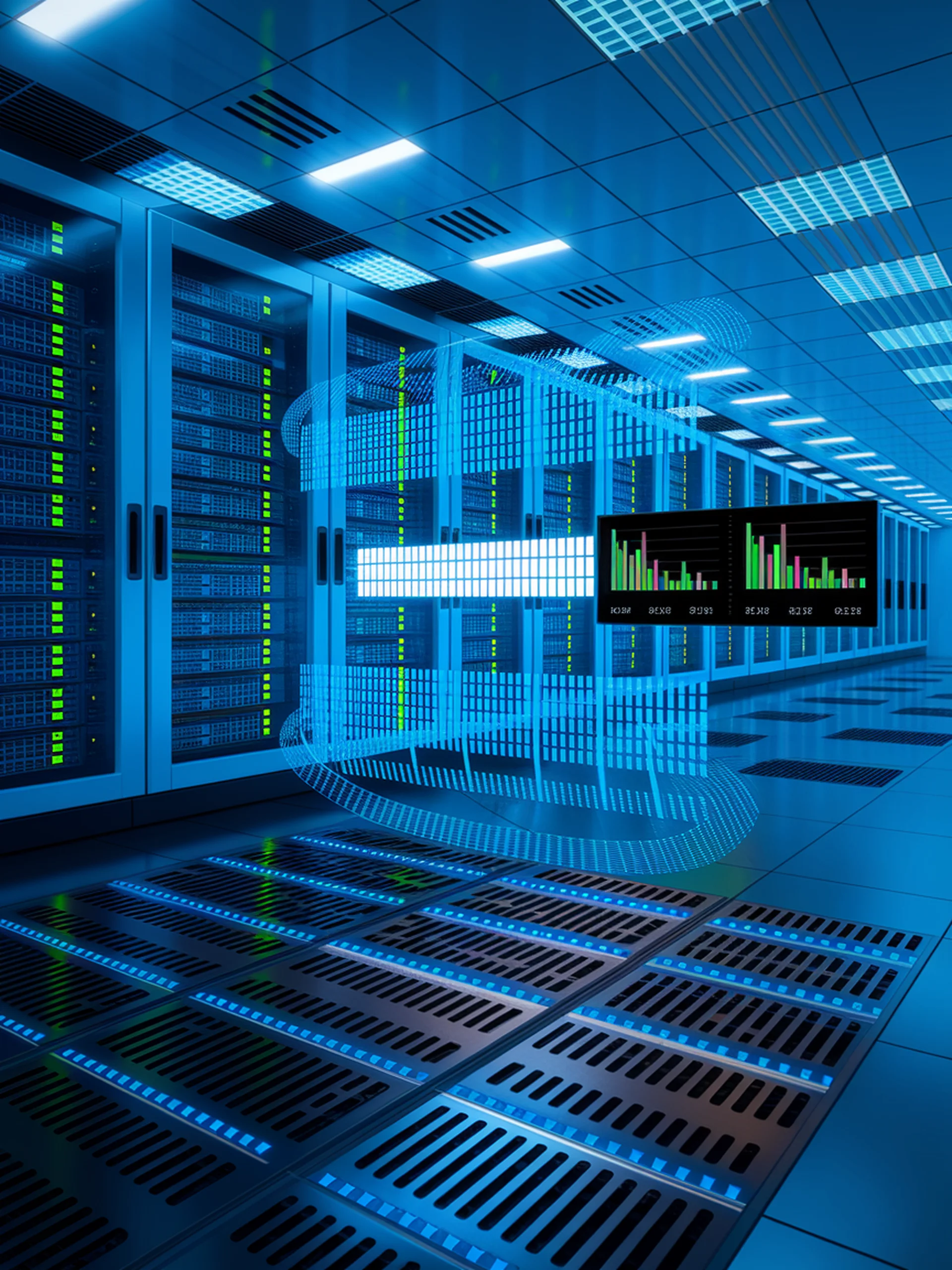
Speeding Up LLMs with Smart Memory Management
Two-Stage KV Cache Compression for Extended Context Handling
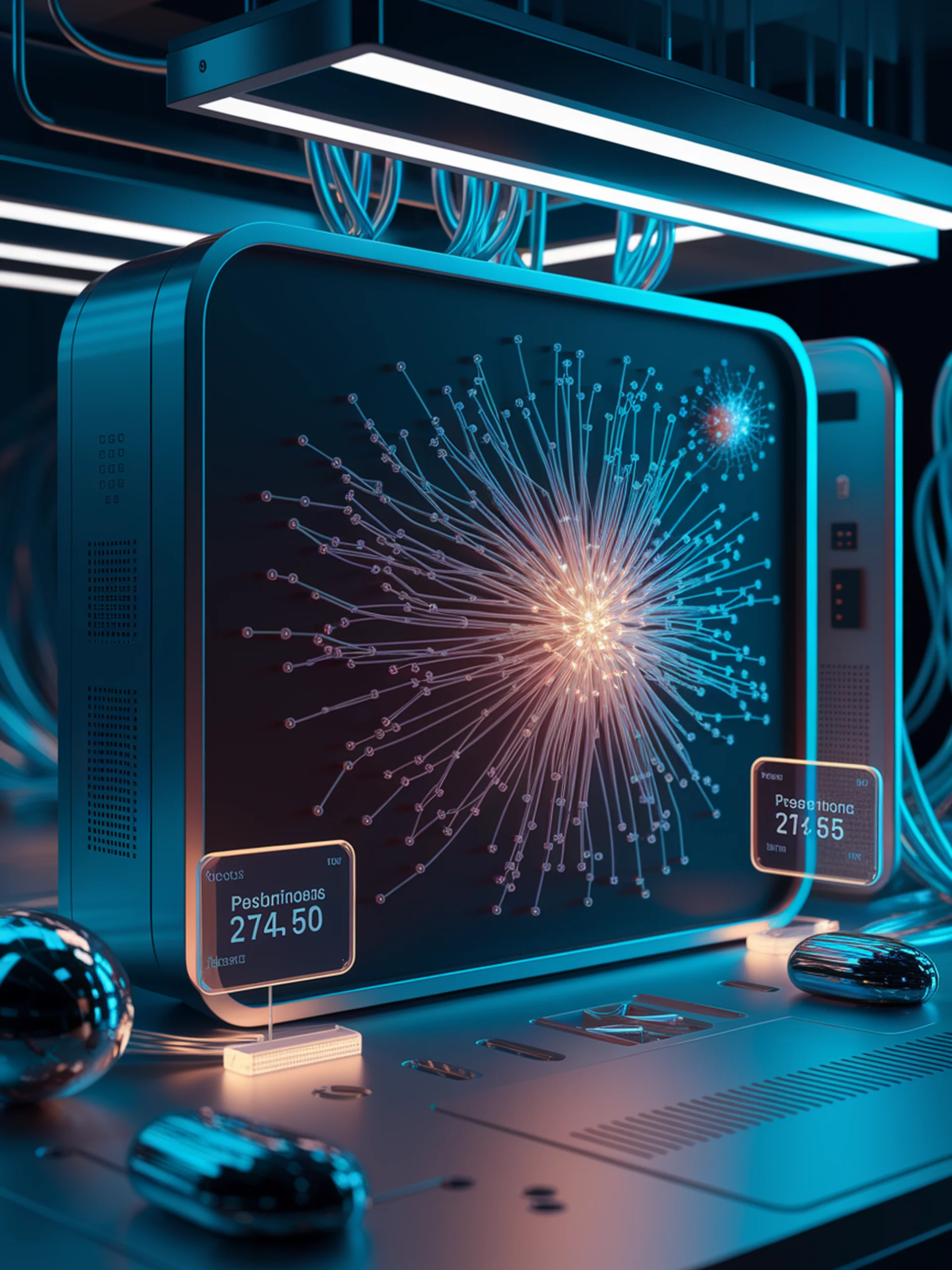
Self-Pruning LLMs: Smarter Size Reduction
Enabling models to intelligently determine their own pruning rates

Optimizing LLM Service Efficiency at Scale
A novel approach for managing diverse inference workloads

Optimizing Sparse LLMs
Dynamic Low-Rank Adaptation to Recover Performance
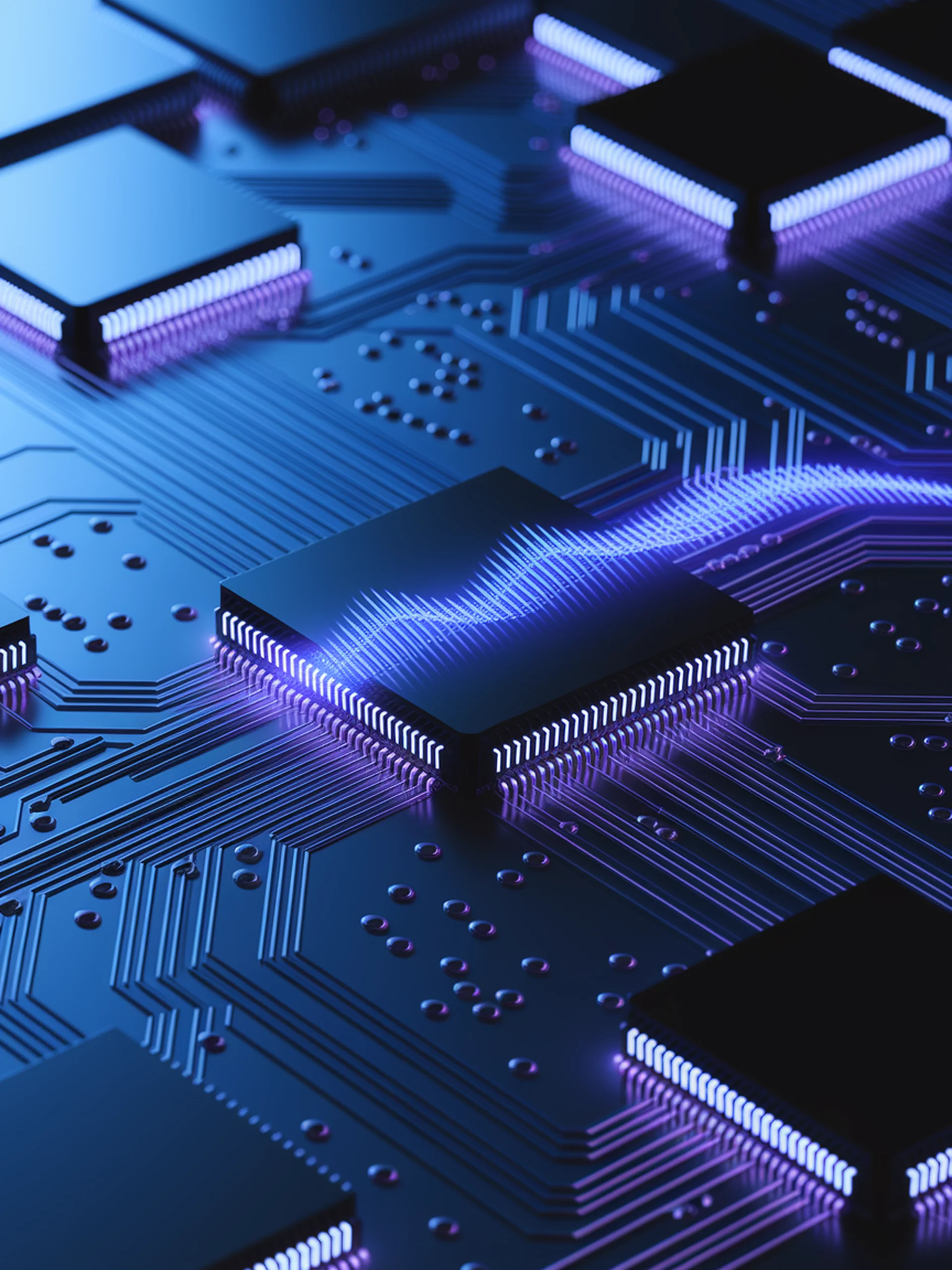
Accelerating LLMs with FR-Spec
A novel sampling technique for faster AI inference
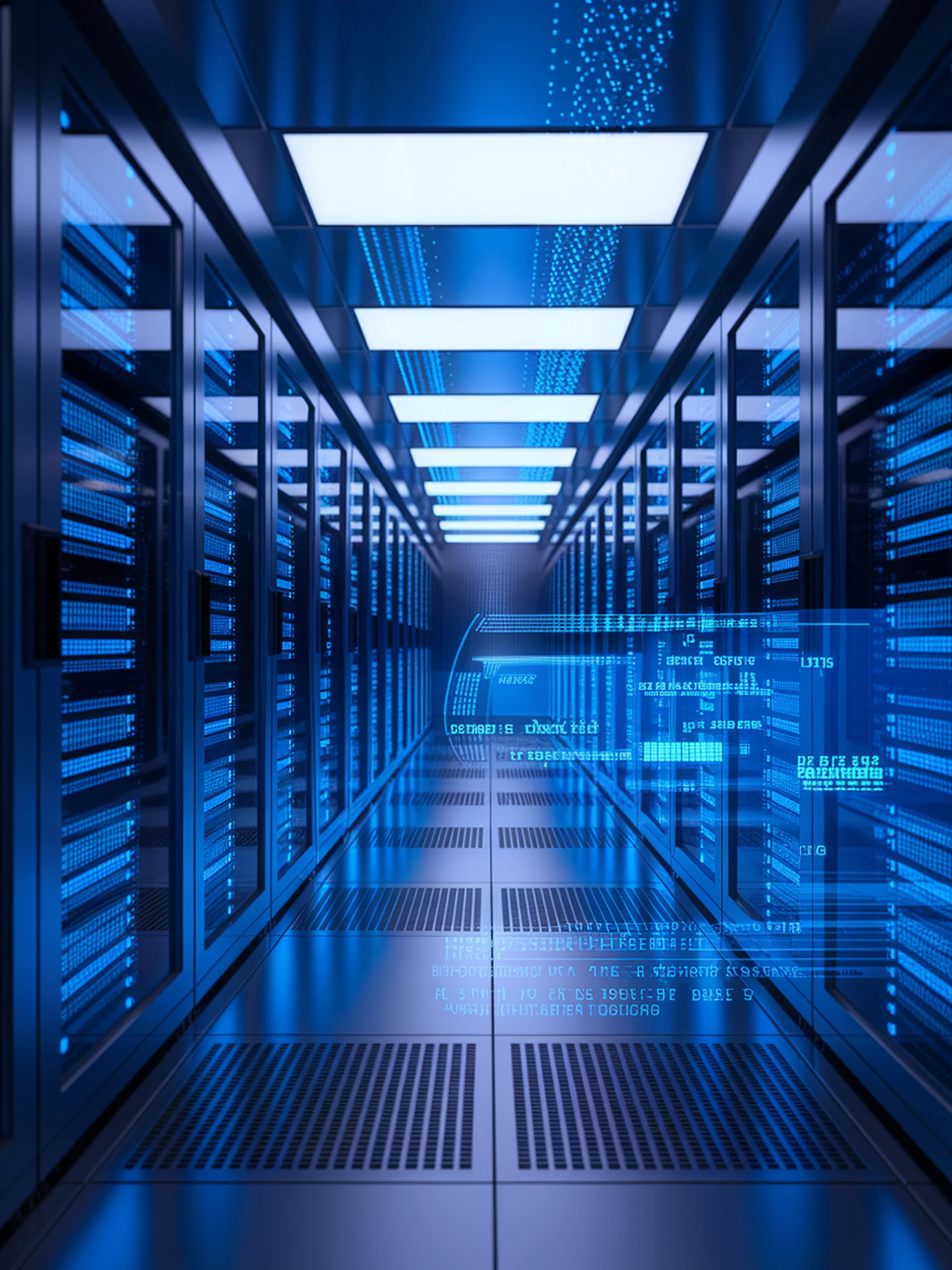
Fast-Track for Long-Context LLMs
Optimizing LLM serving with unified sparse attention
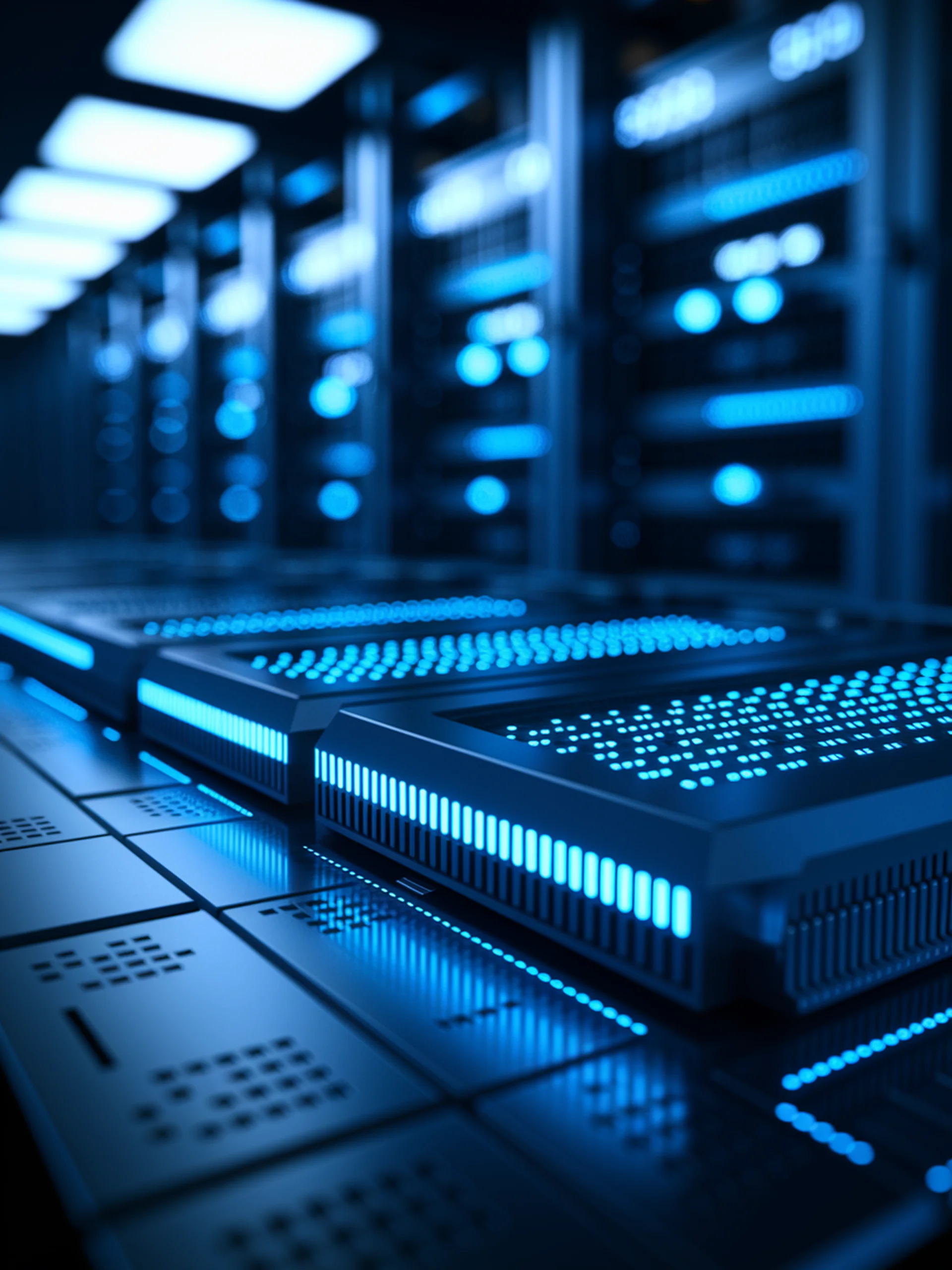
1-Bit KV Cache Quantization
Revolutionizing memory efficiency in multimodal LLMs
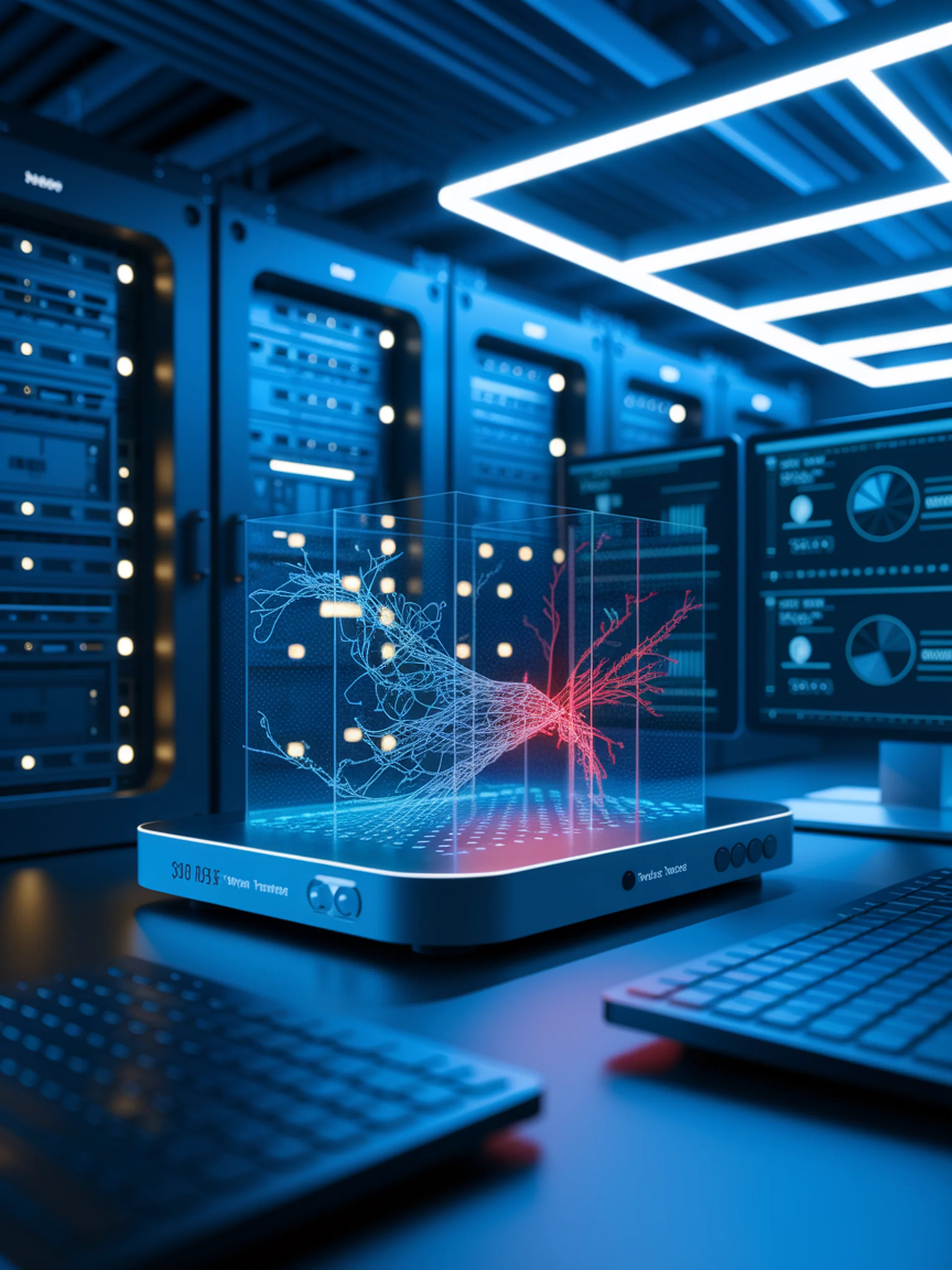
Evolutionary Pruning for Efficient LLMs
A novel approach to optimize LLMs for resource-constrained environments
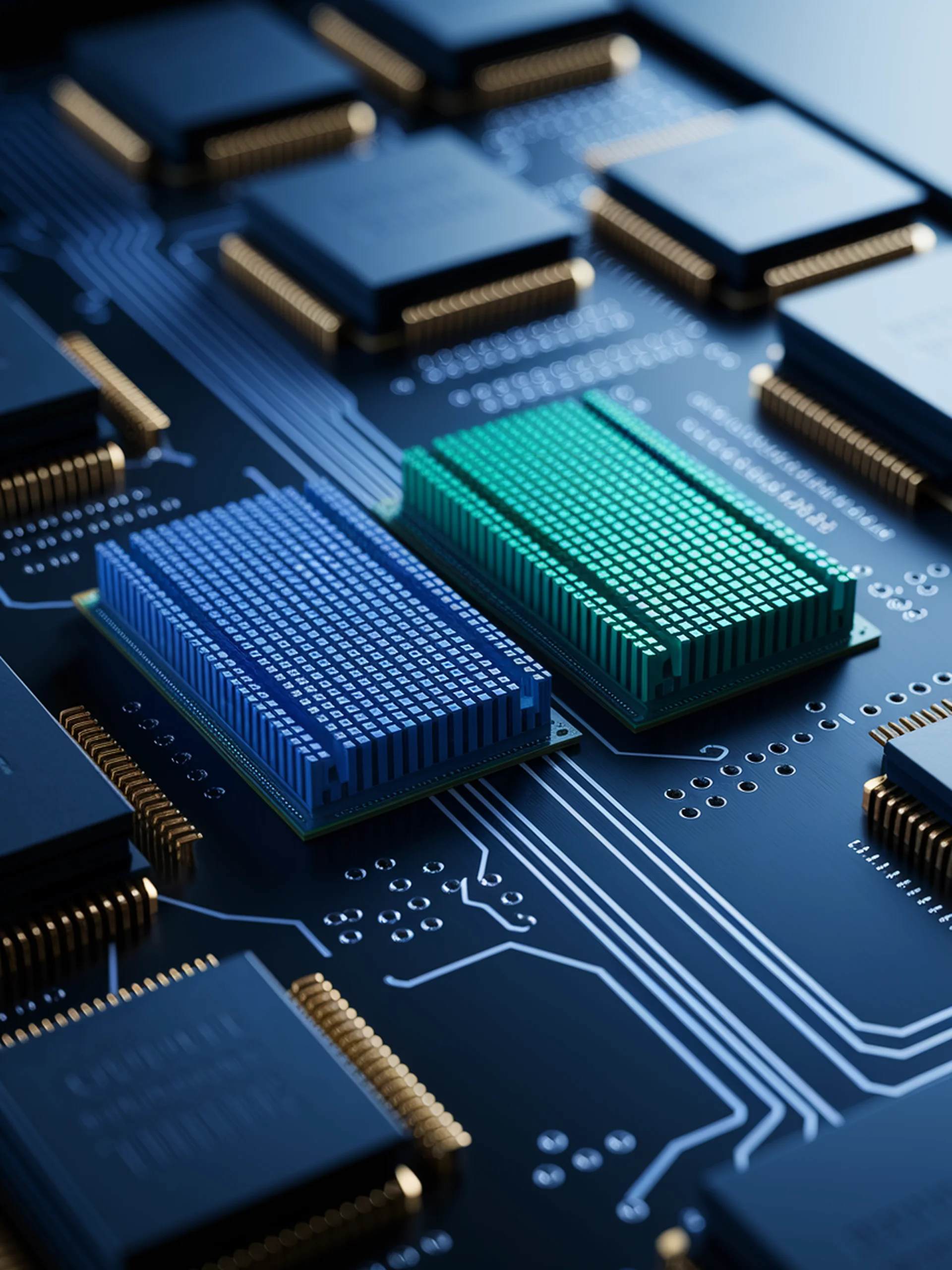
Smart Memory Compression for LLMs
Keys need more bits, values need fewer
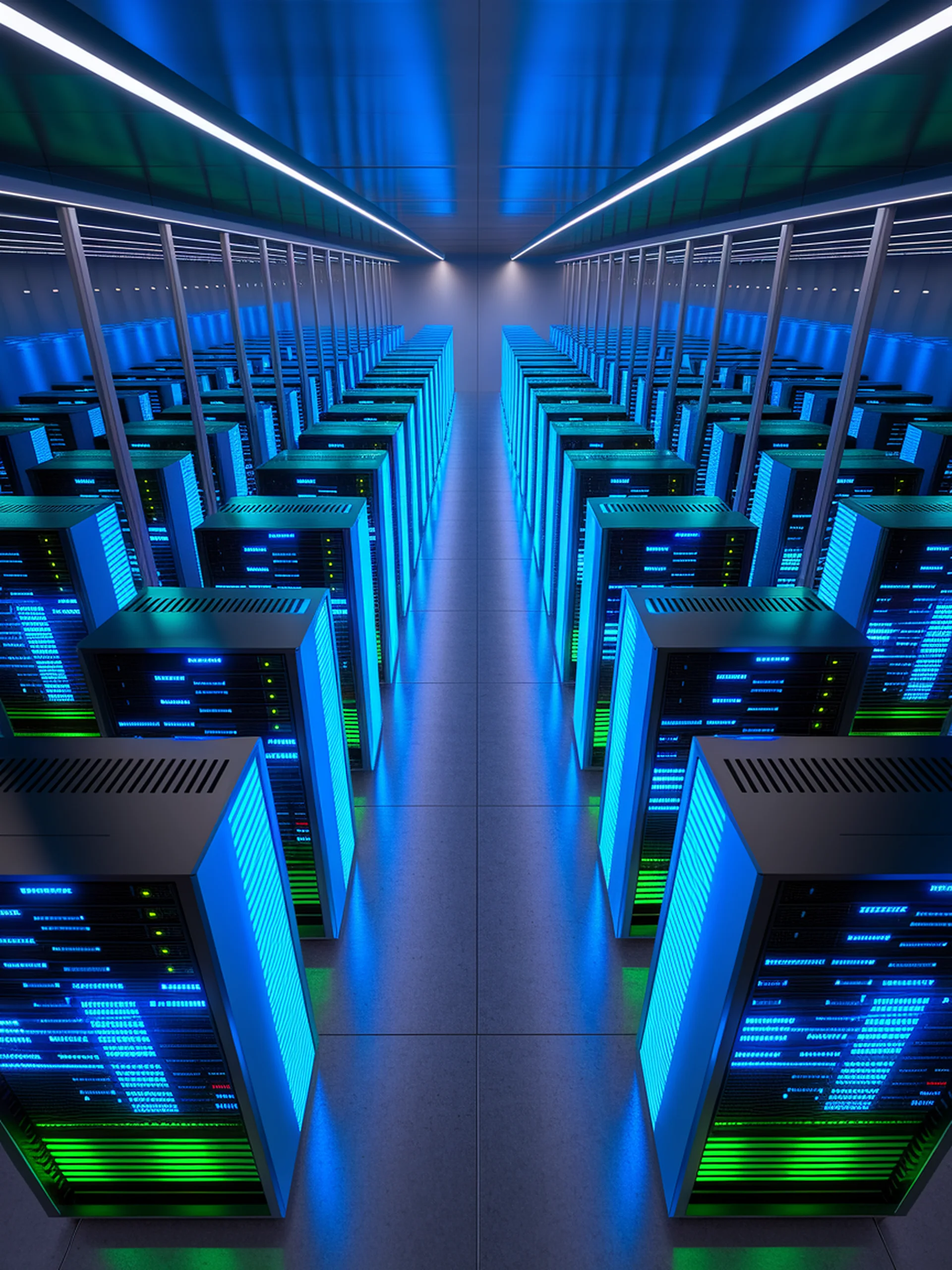
Optimizing LLM Throughput with TETRIS
Intelligent token selection for faster, more efficient inference

Accelerating Mamba Models for AI Applications
Hardware-efficient implementation for state space models
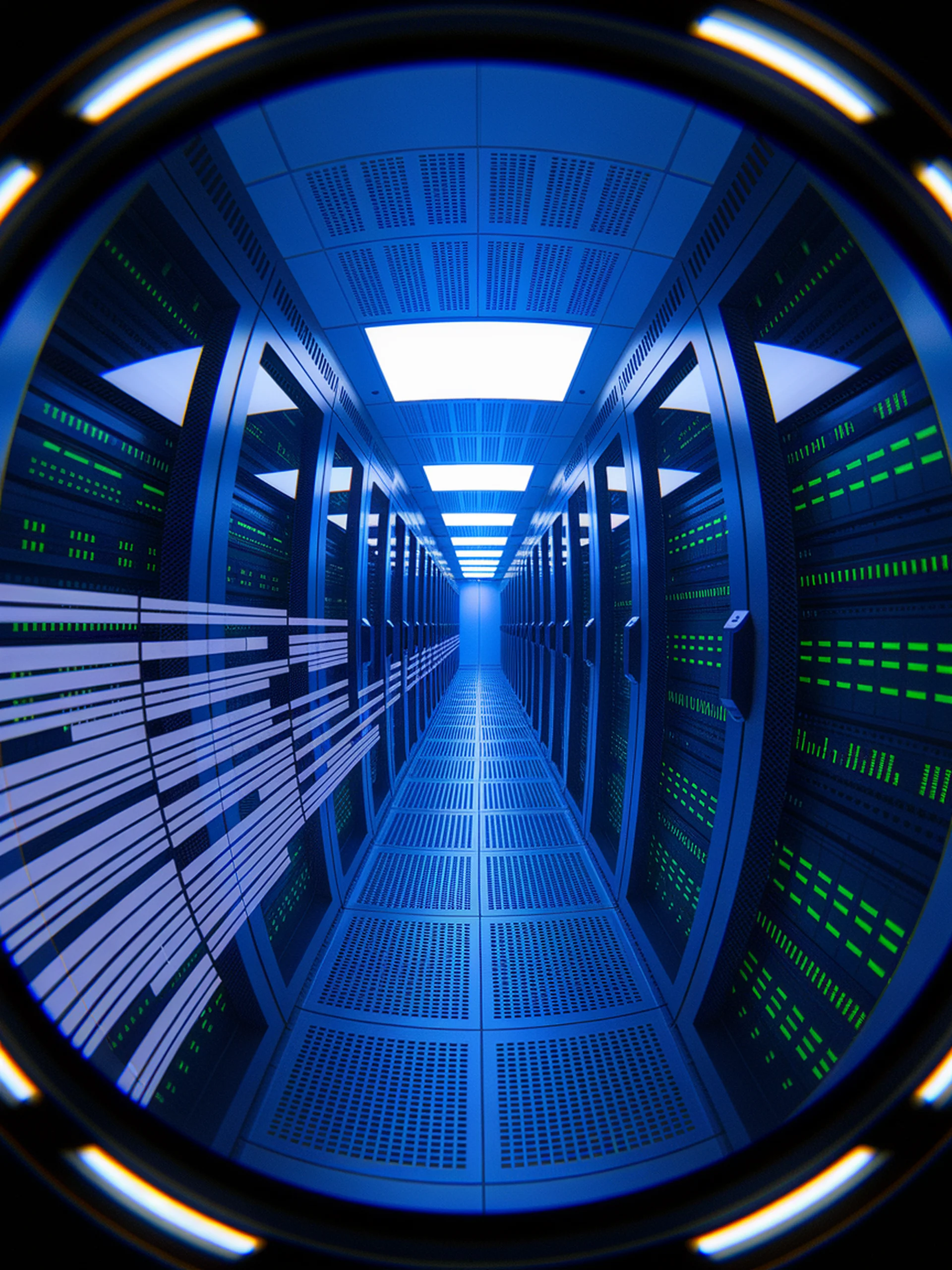
Accelerating LLM Conversations with Round Attention
A targeted approach to reduce memory overhead in multi-turn dialogues
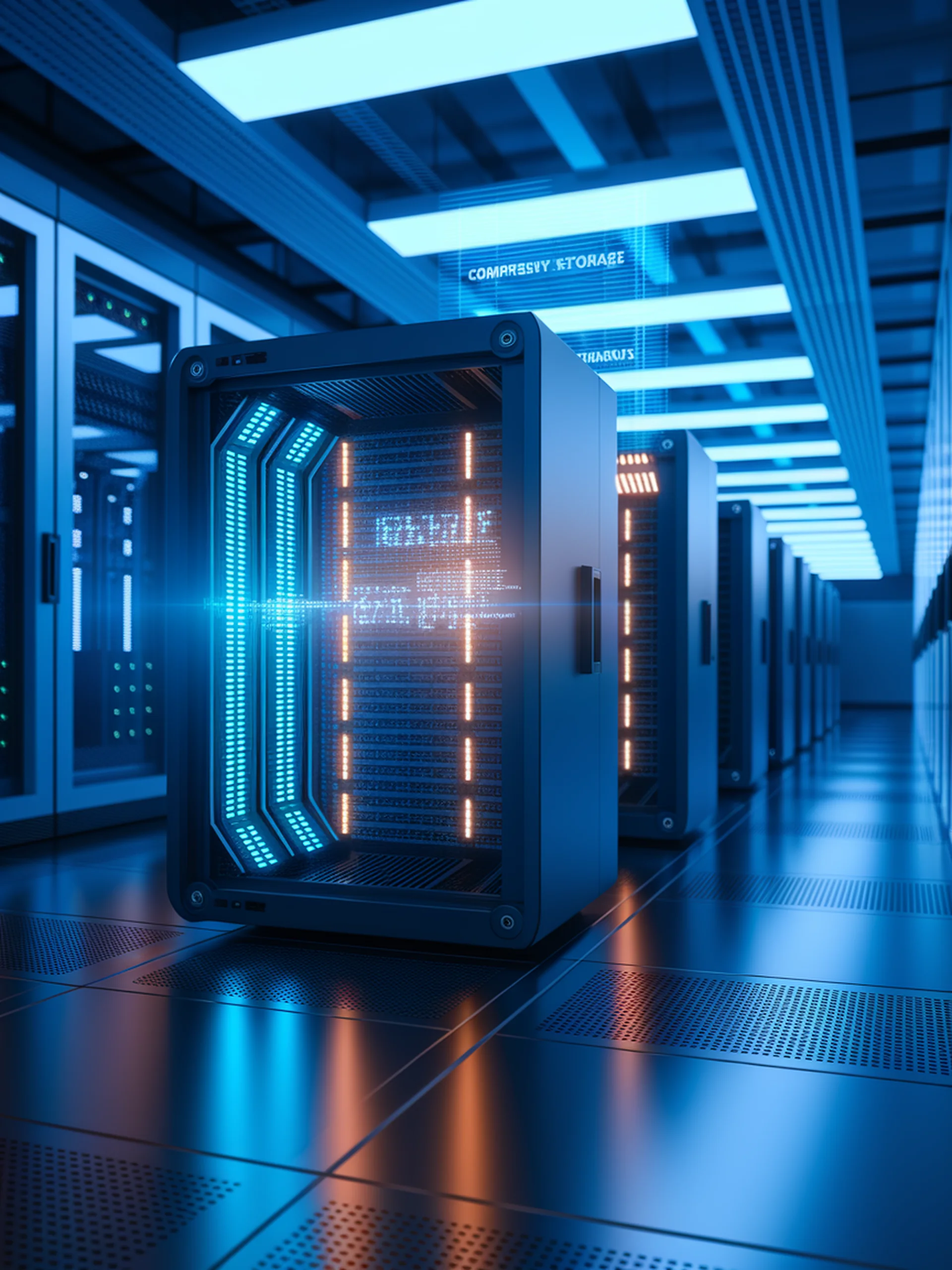
Revolutionizing LLM Memory Efficiency
SVDq: Achieving 410x Key Cache Compression with 1.25-bit Precision
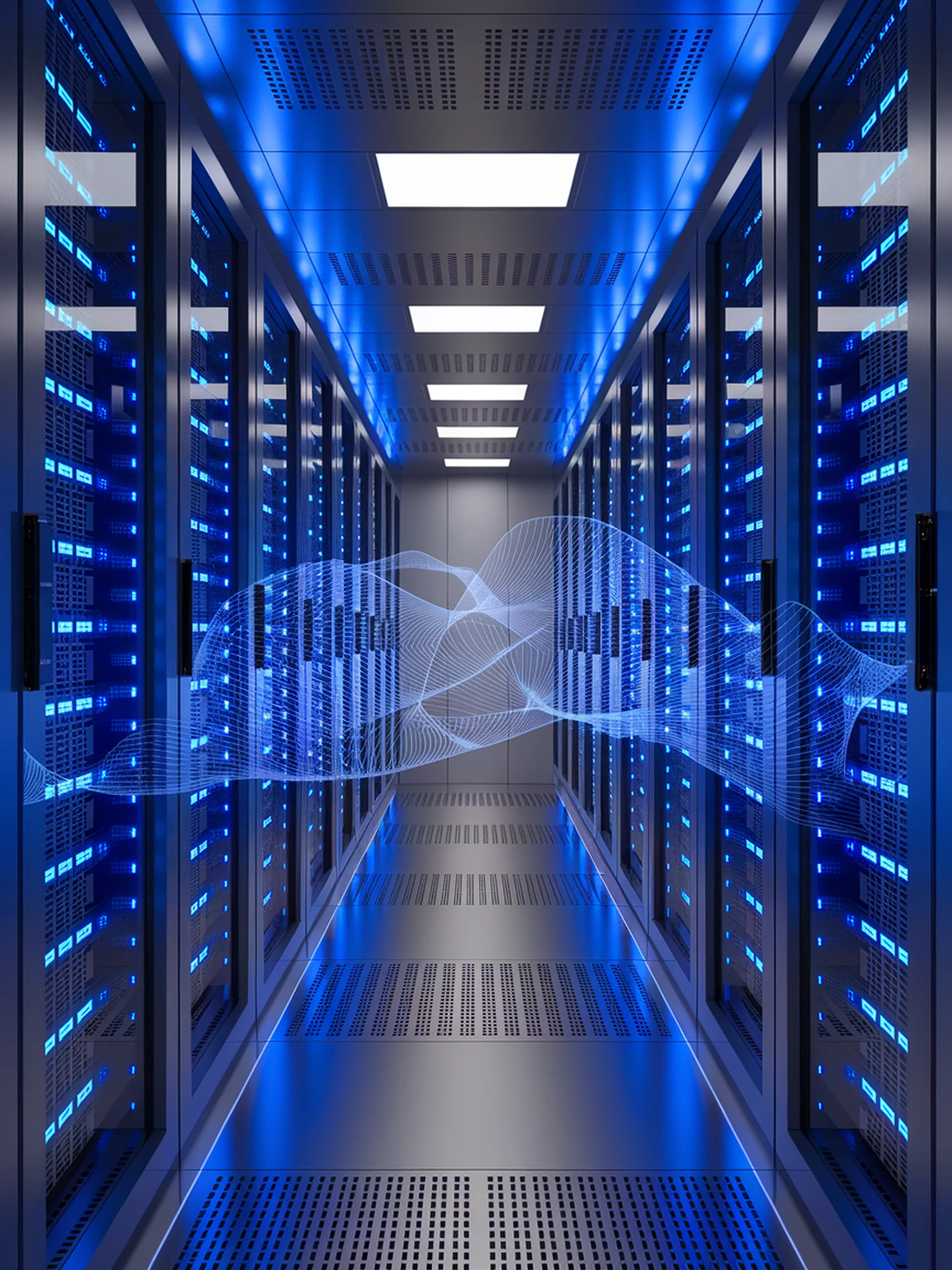
Optimizing Attention Mechanisms Across Hardware
A versatile framework for efficient LLM deployment
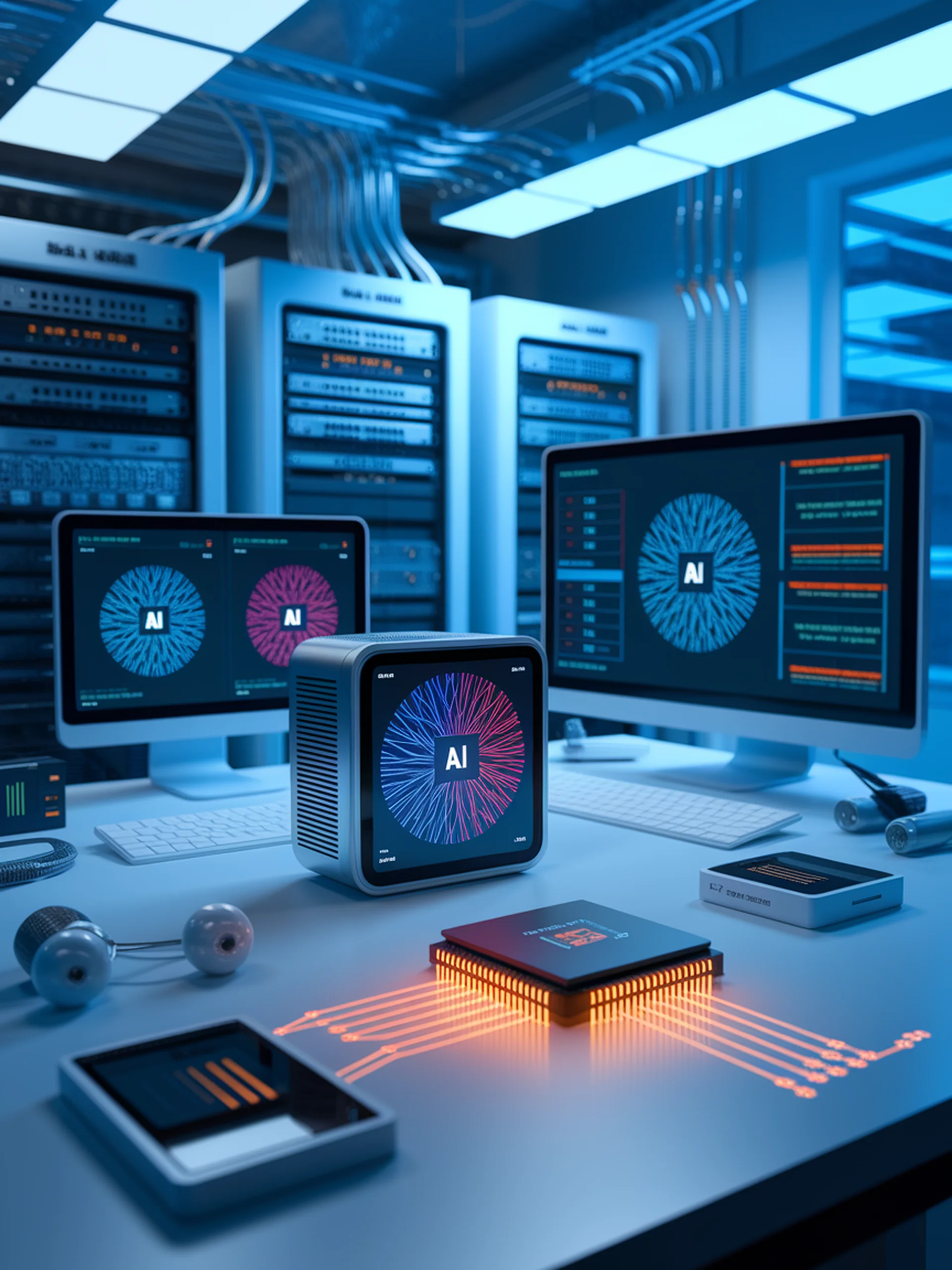
Double Compression for Memory-Efficient LLMs
Enabling LLM deployment on memory-limited devices
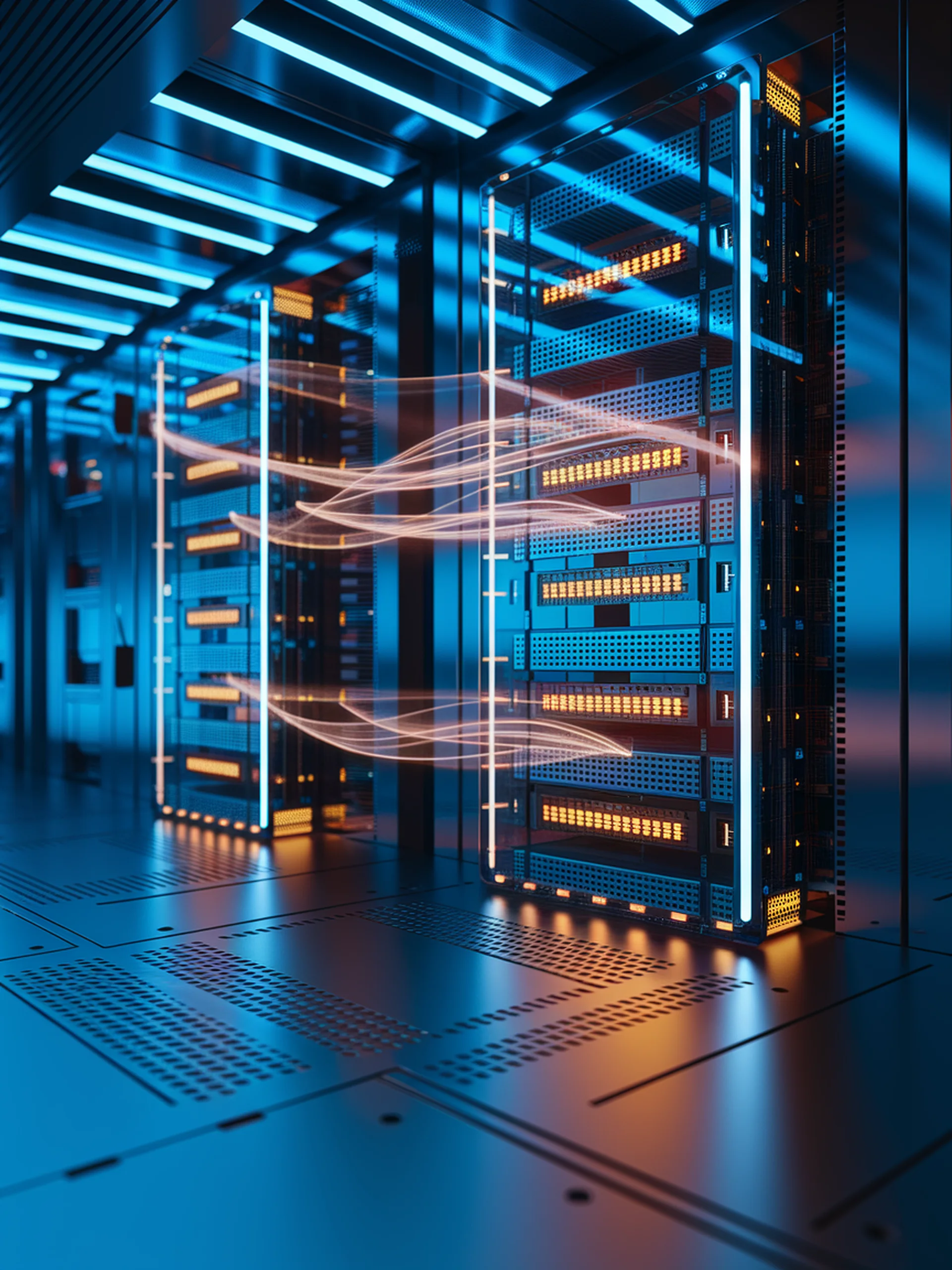
Accelerating LLM Decoding with PAPI
A Dynamic PIM-Enabled Architecture for Efficient Token Generation

Slashing LLM Cold Start Delays
How ParaServe accelerates serverless LLM deployment
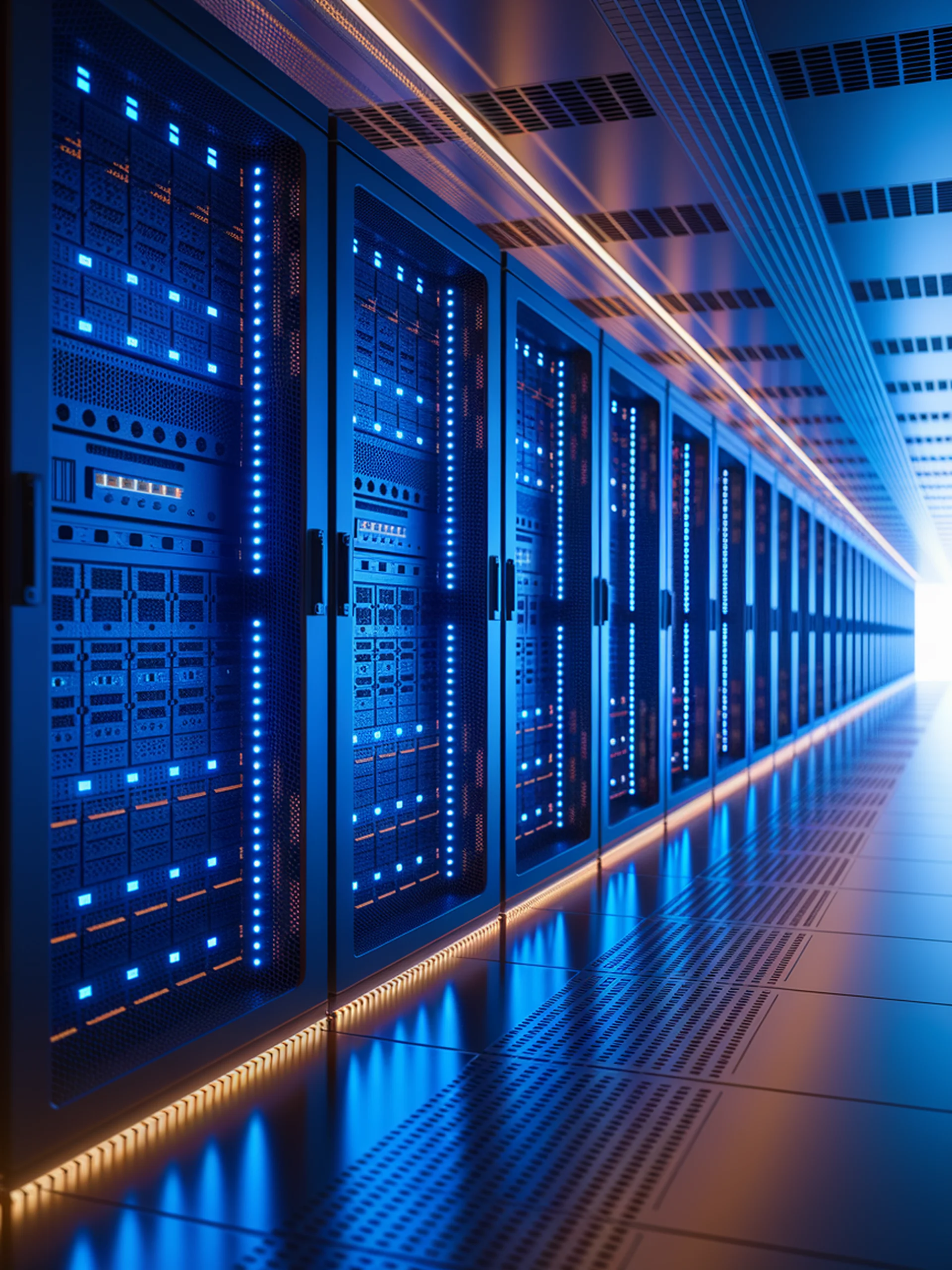
Dynamic Pruning for Faster LLMs
Accelerating large language models through intelligent token-based pruning
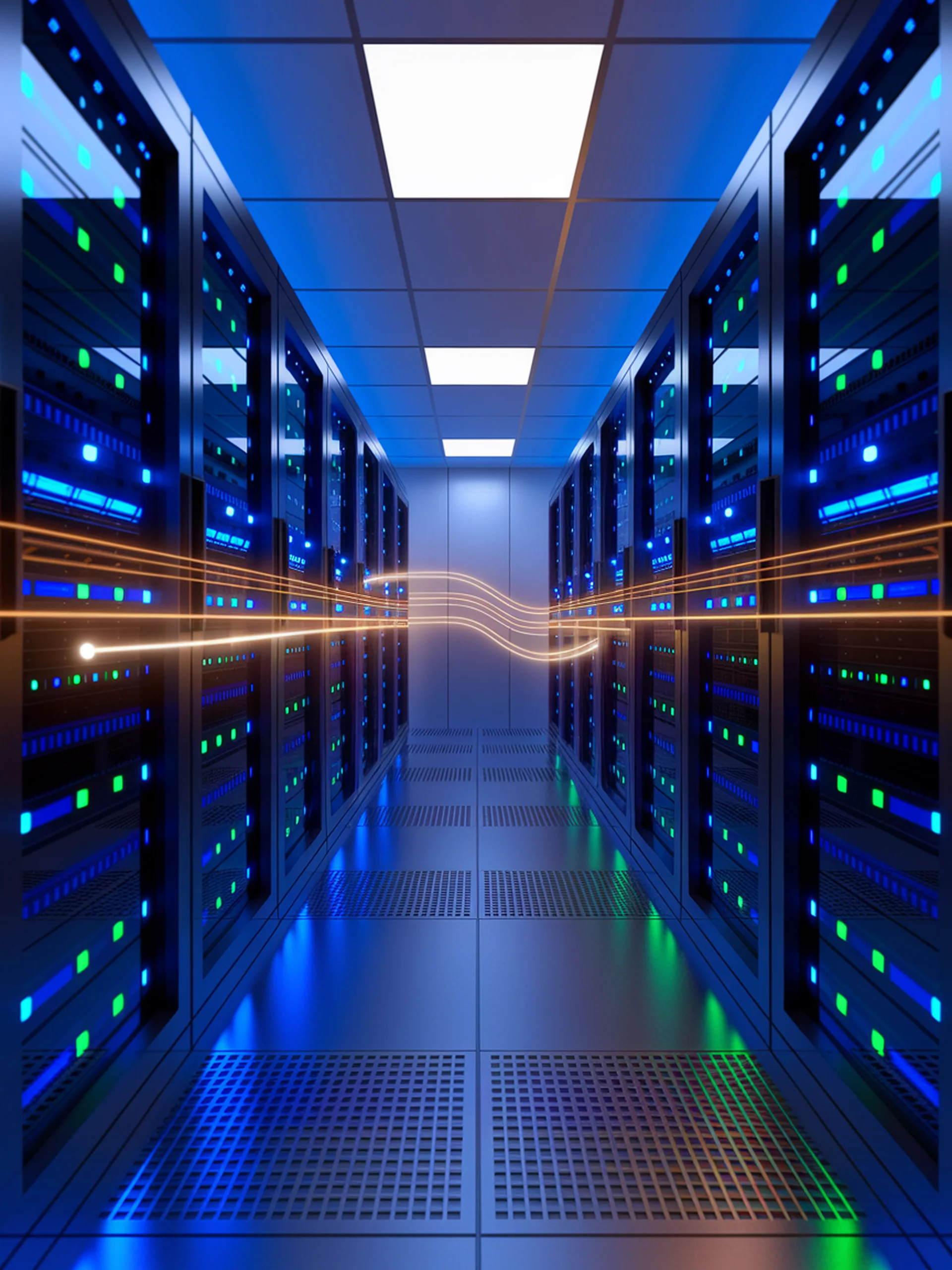
Boosting RAG Performance with Smart Caching
How Cache-Craft optimizes LLM processing for repeated content
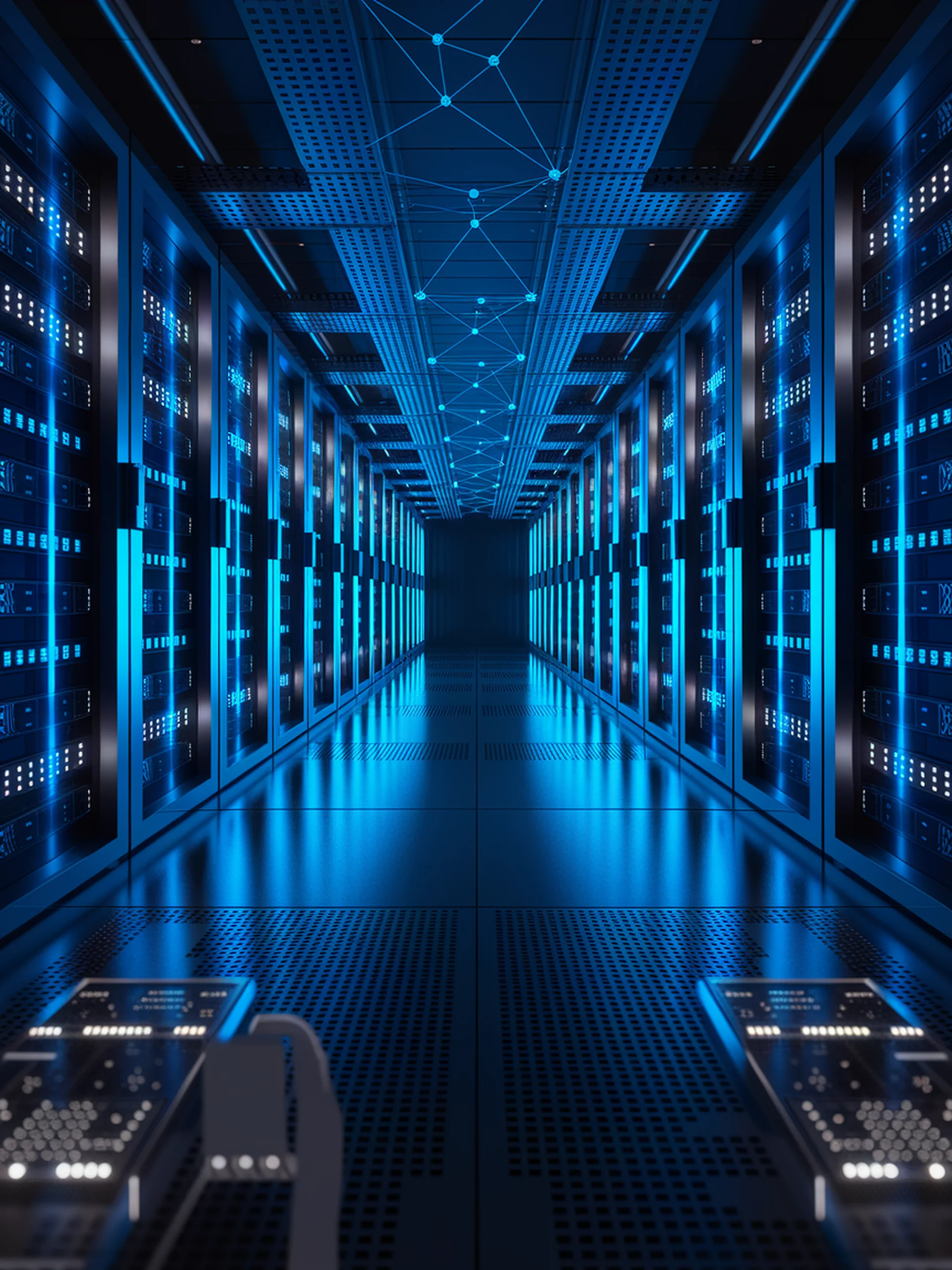
Optimizing LLM Inference at Scale
A hybrid offline-online scheduling approach for maximizing throughput

Efficient Edge Computing for LLMs
Transforming LLM Deployment with Ternary Quantization on FPGAs
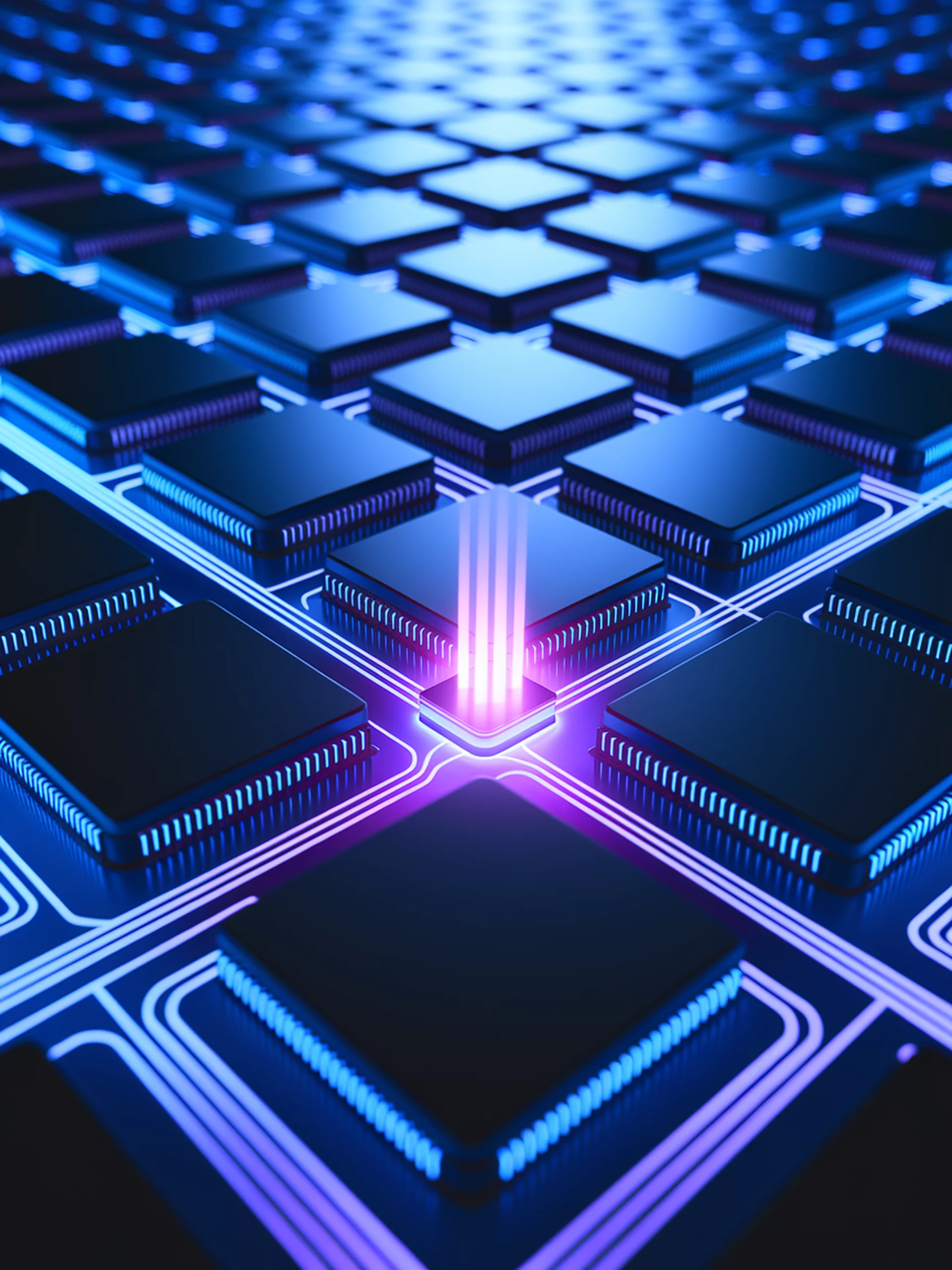
Accelerating LLM Inference with CORAL
Consistent Representation Learning for More Efficient Speculative Decoding

Smart Memory Management for LLMs
Dynamic KV Cache Compression for Optimal Performance
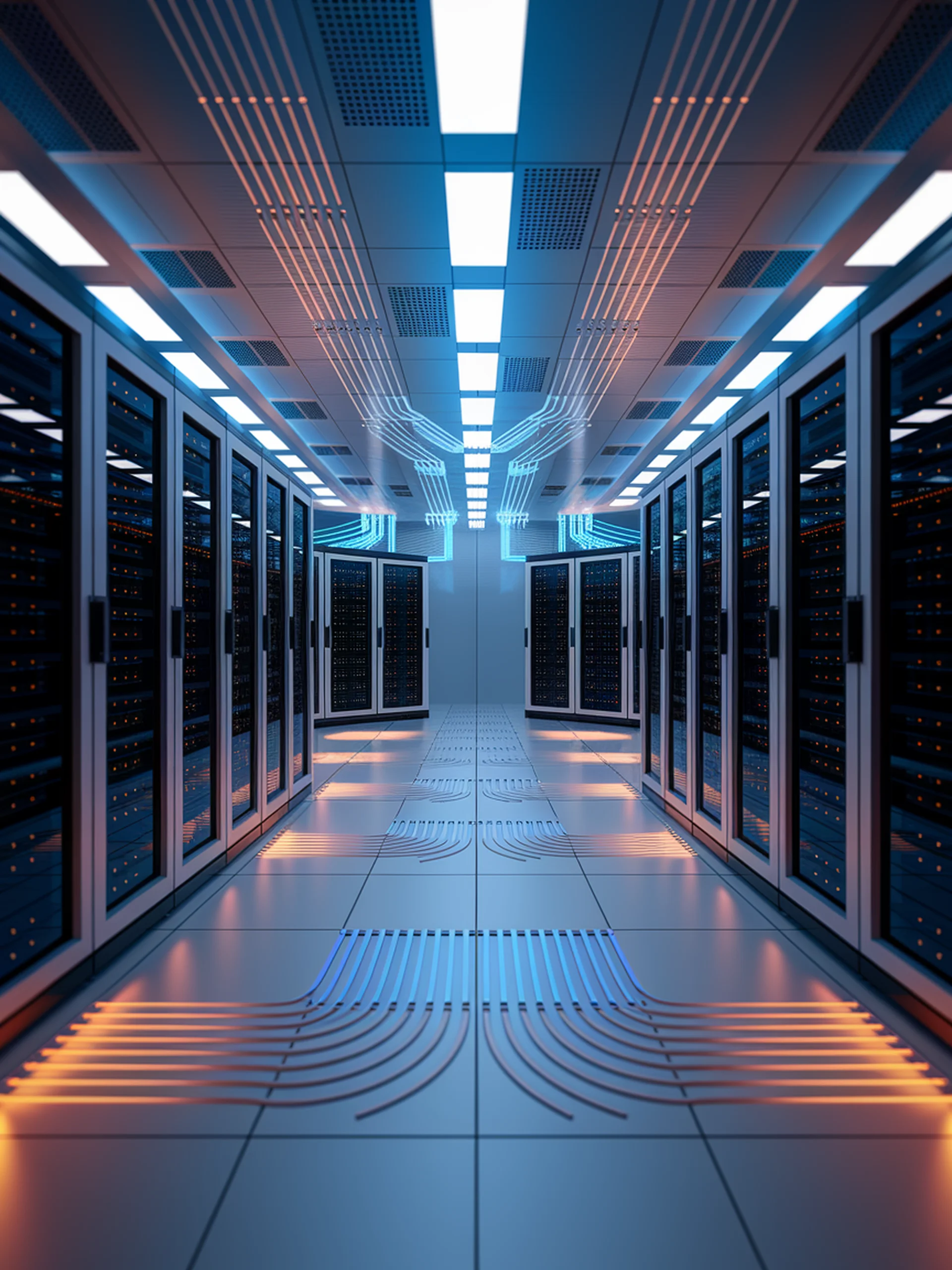
BigMac: Optimizing Communication in LLM Architecture
A breakthrough in reducing AI training and inference bottlenecks
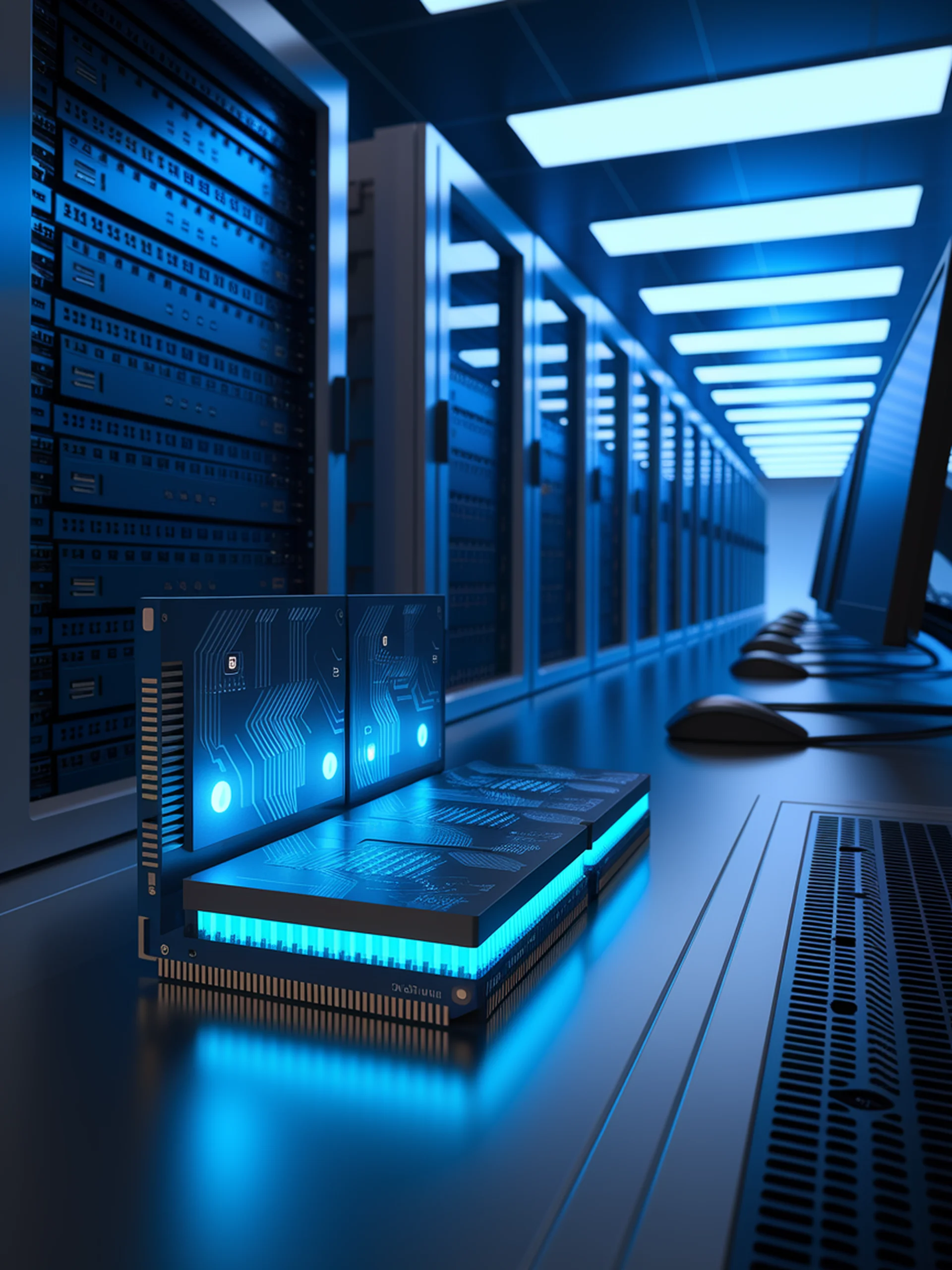
Democratizing LLM Inference
Bridging the GPU Memory Gap with Near-Data Processing
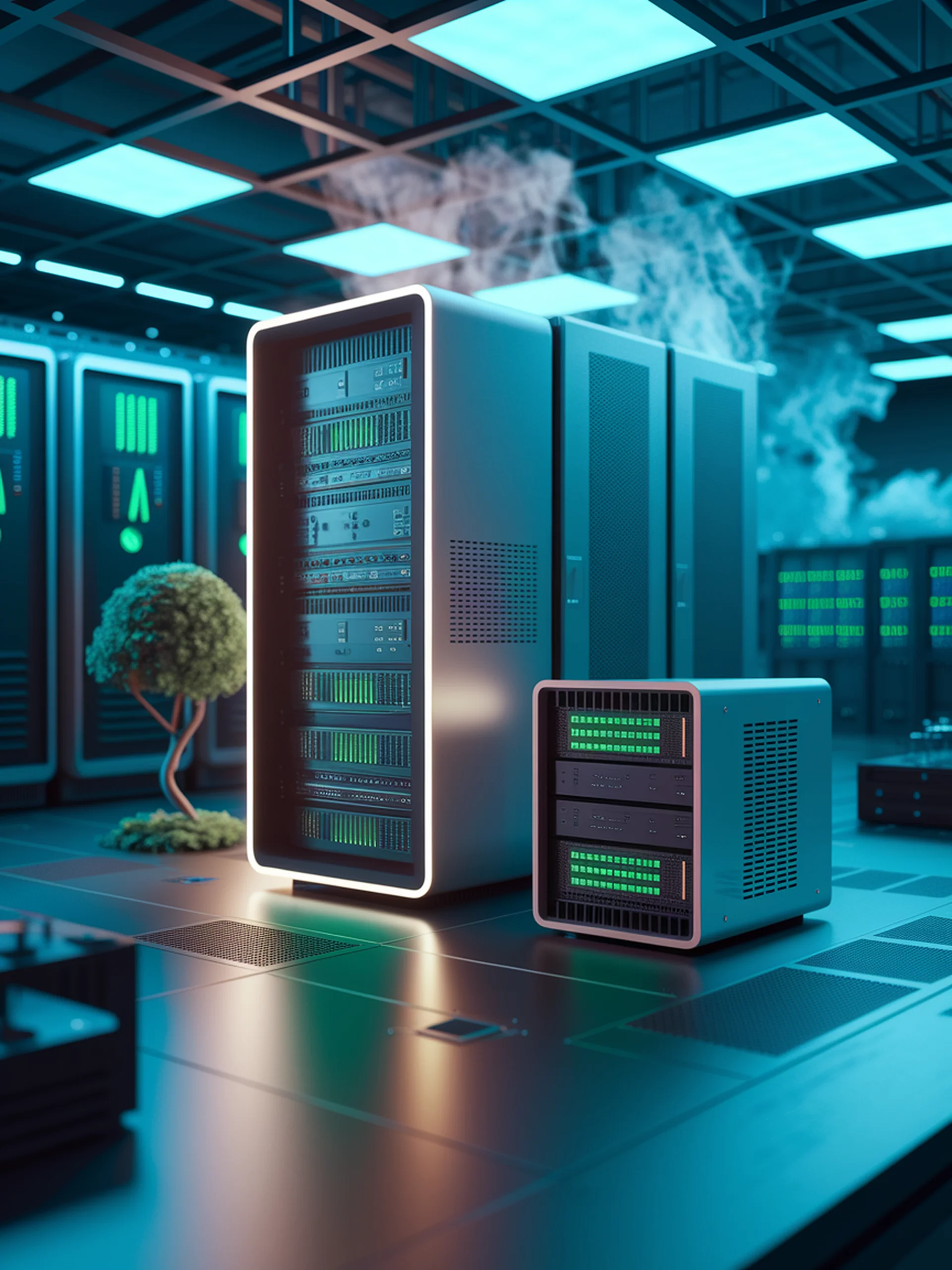
Smarter LLM Pruning for Greener AI
Reducing computational footprint while maintaining performance
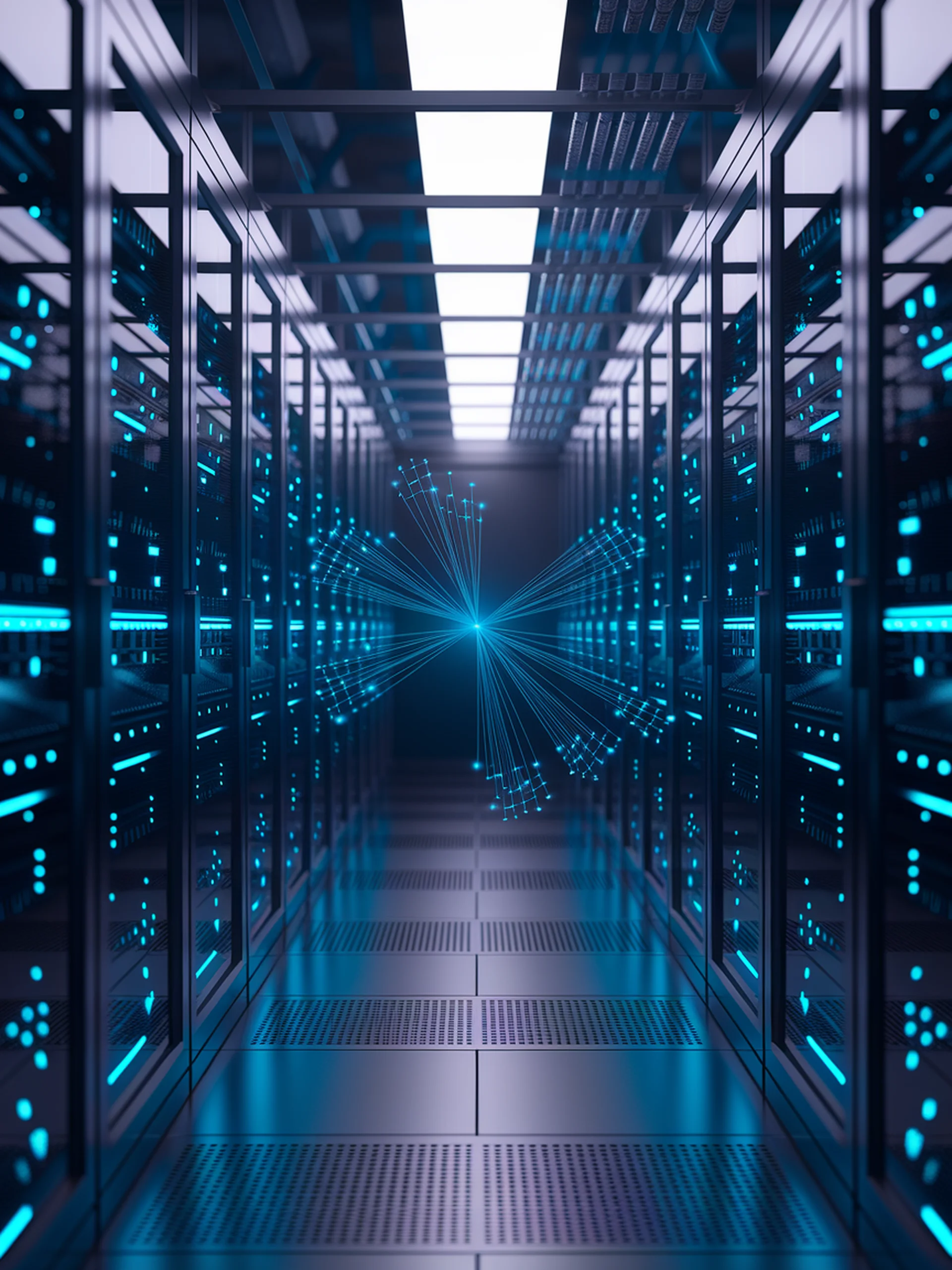
Shrinking MoE Language Models
Innovative compression through delta decompression technique
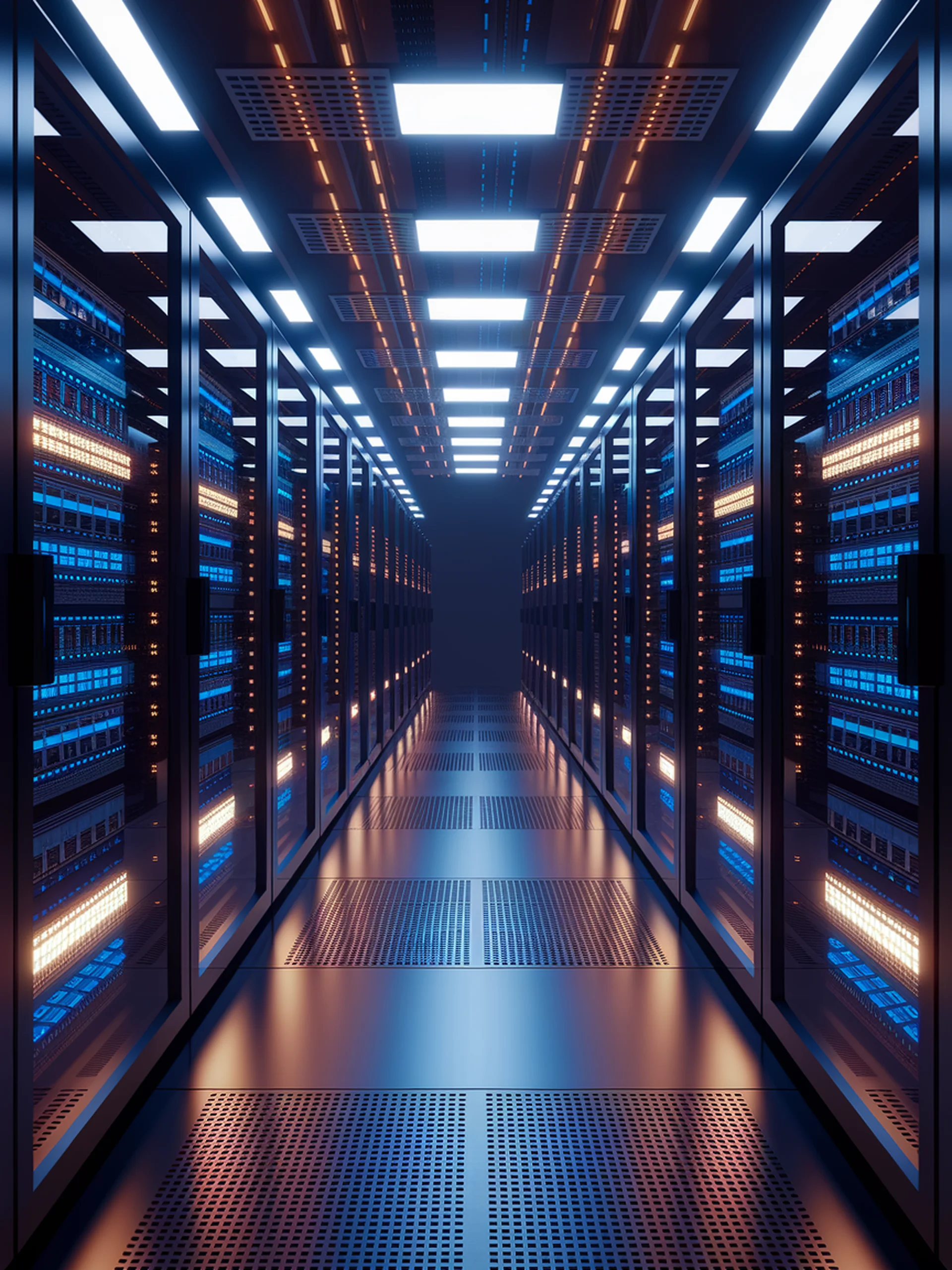
COSMOS: Revolutionizing LLM Optimization
A memory-efficient hybrid approach for training large language models

Accelerating LLMs for Long Contexts
A novel approach to speculative decoding that reduces inference bottlenecks
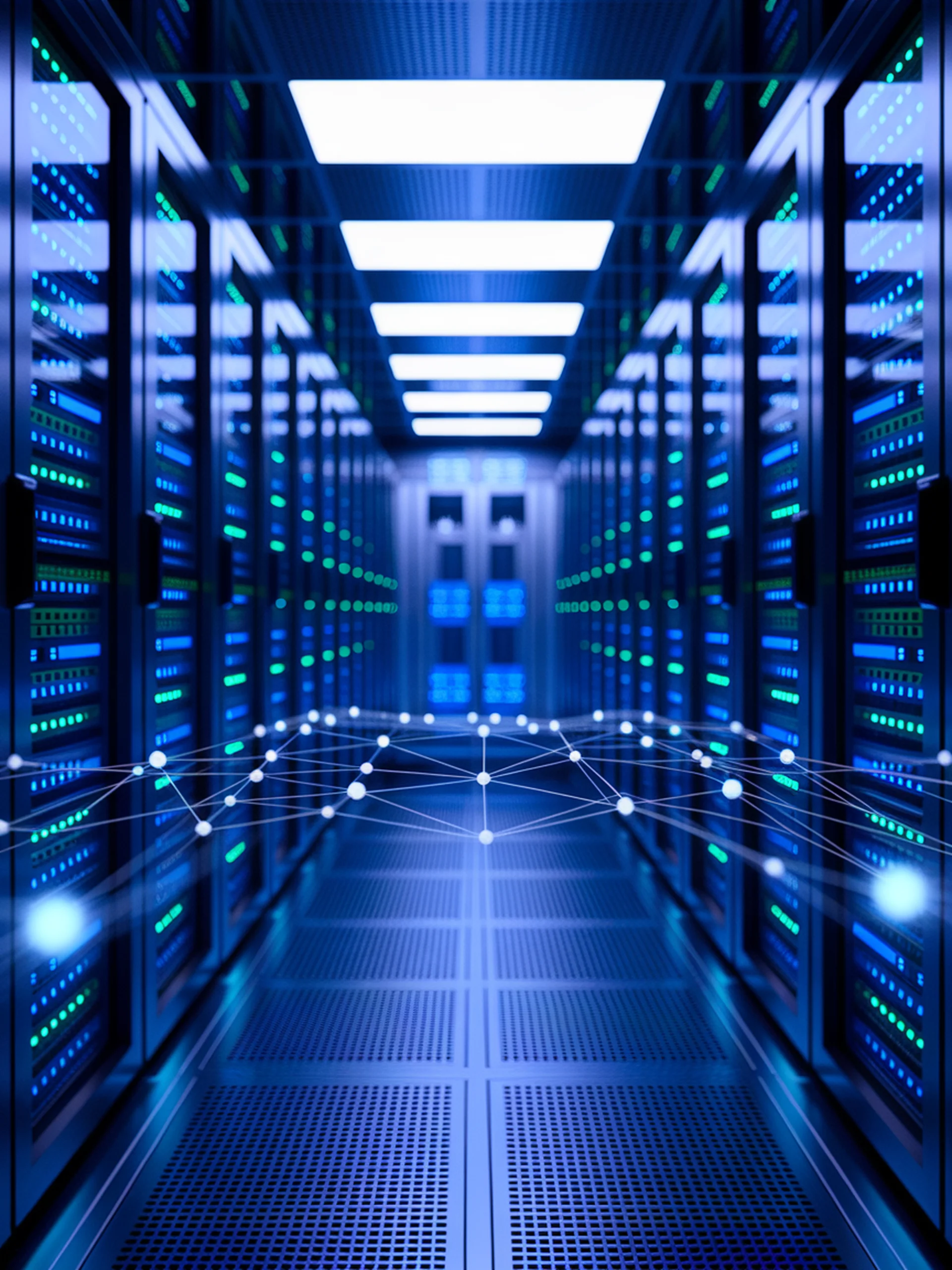
Smarter Memory Management for LLMs
A Game Theory Approach to Optimizing KV Cache Allocation
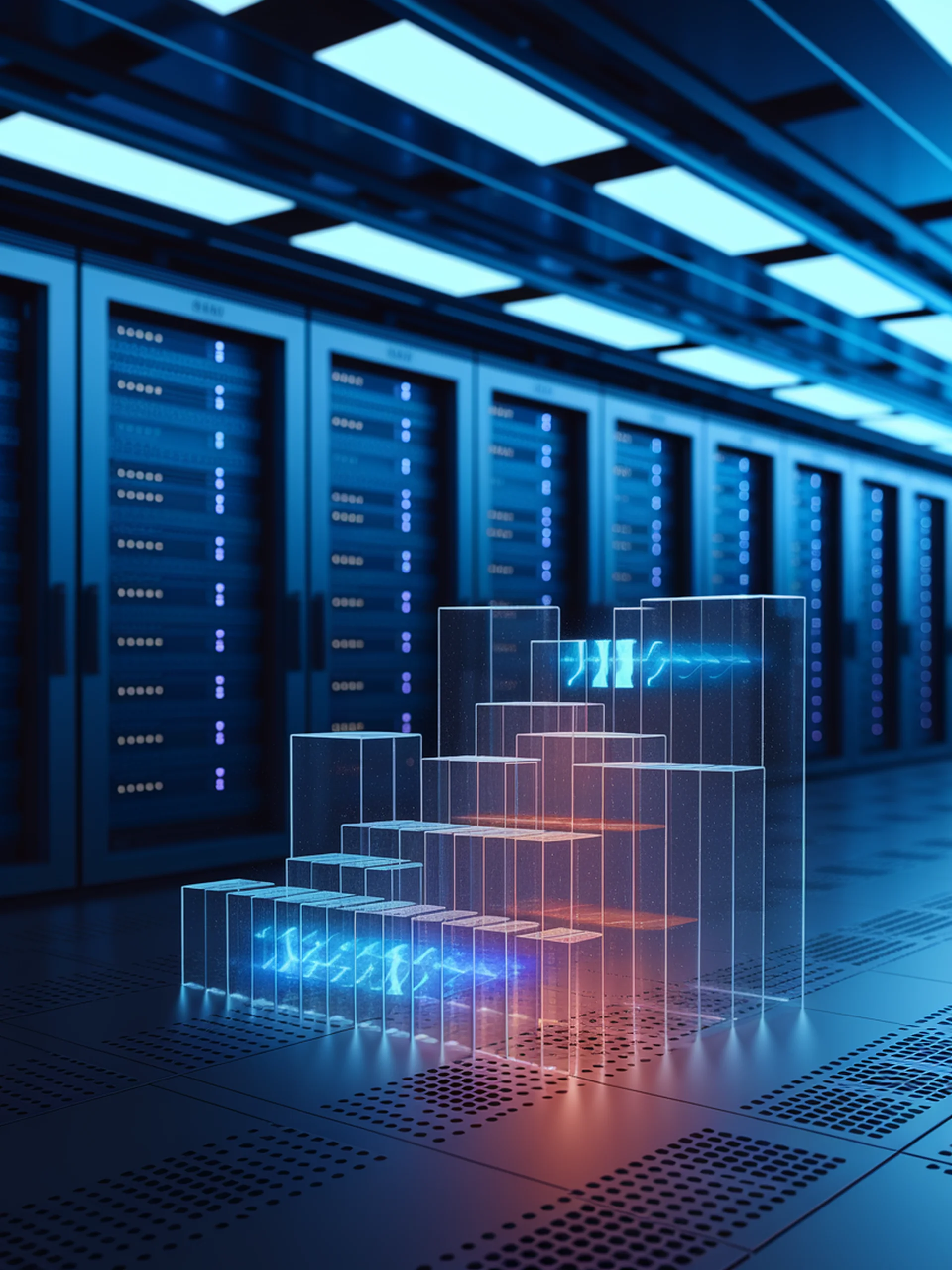
Smarter Memory for Multimodal AI
Dynamic optimization for long-context AI processing
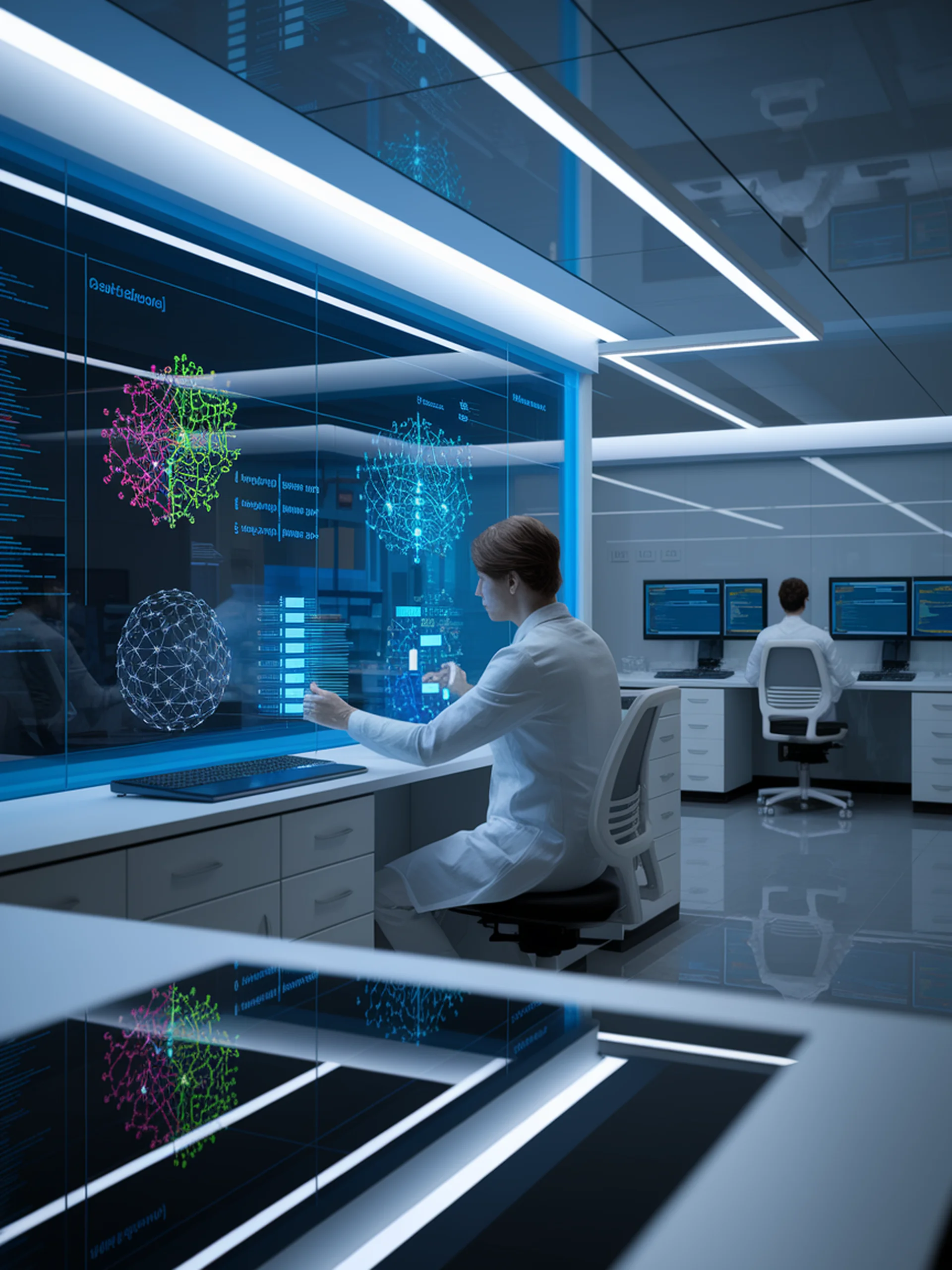
Making LLMs More Predictable
Representation Engineering: A New Approach to Control AI Behavior
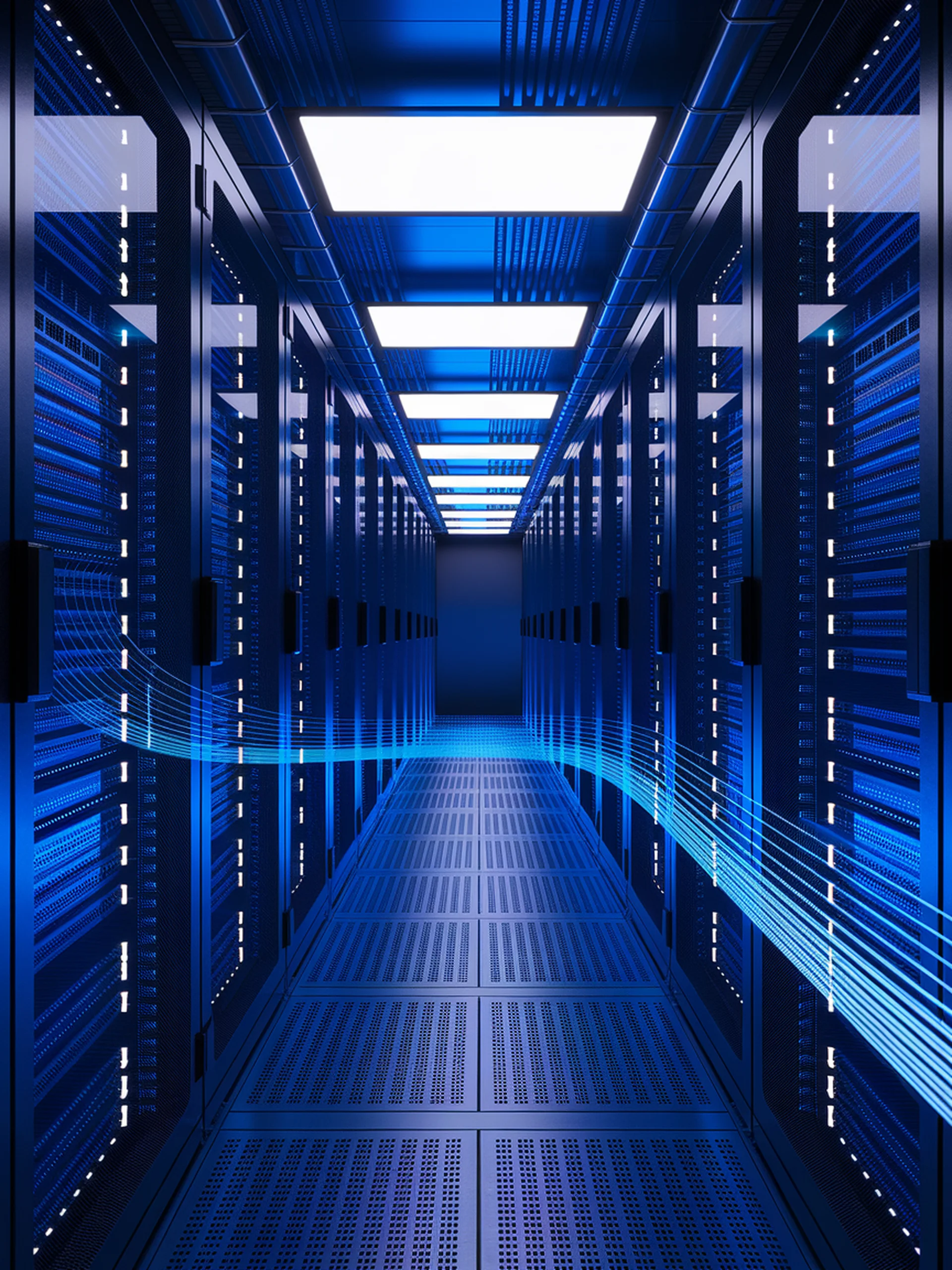
Speeding Up LLM Inference
Efficiency gains through smart operation fusion techniques
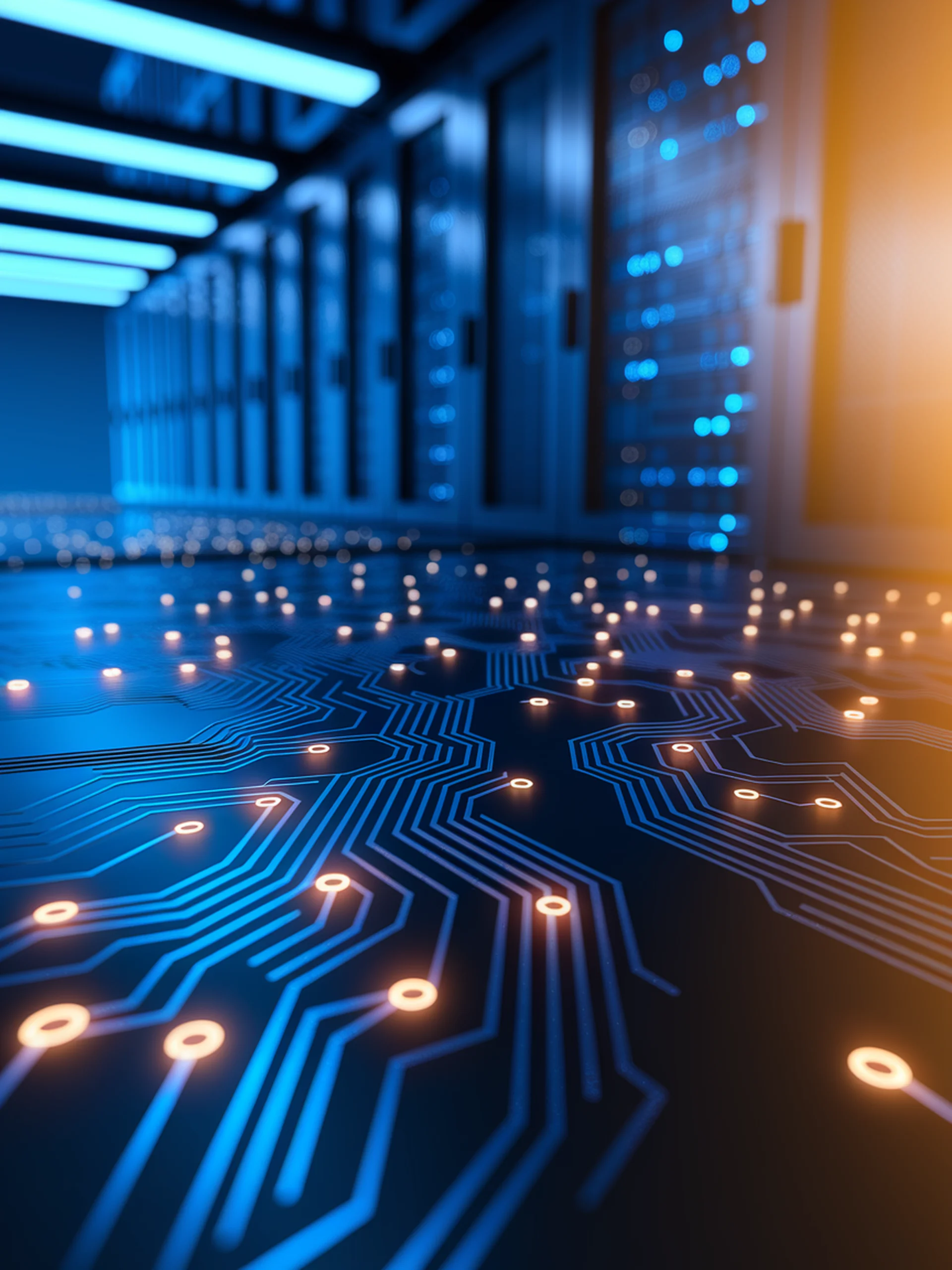
Accelerating LLMs with Sparse Attention
A universal approach to optimizing attention for any model

LevelRAG: Multi-Layered Knowledge Retrieval
Decoupling Query Logic from Retrieval for Enhanced LLM Accuracy
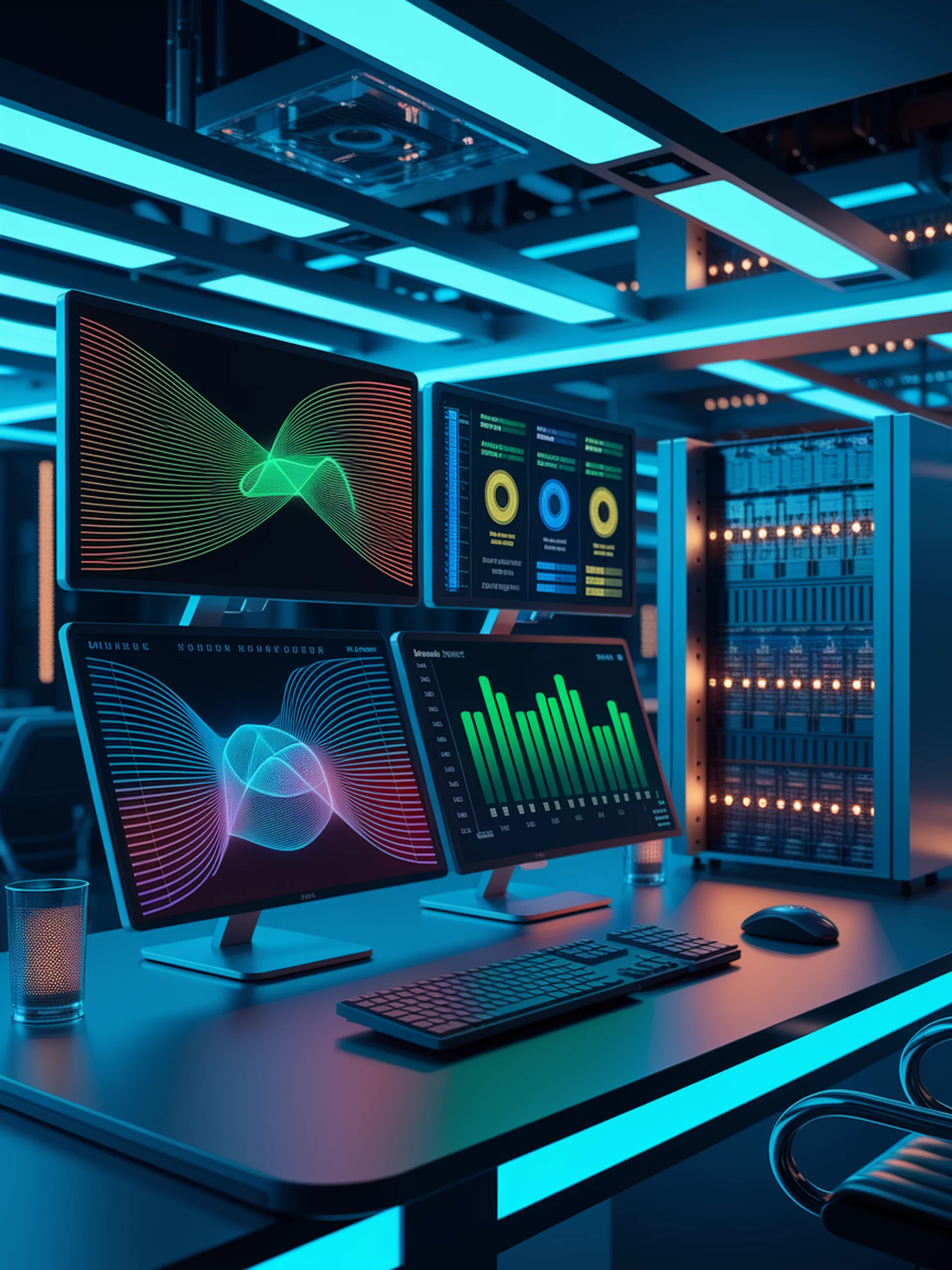
Smart LLM Routing for Maximum Efficiency
Dynamically selecting the optimal model for each query
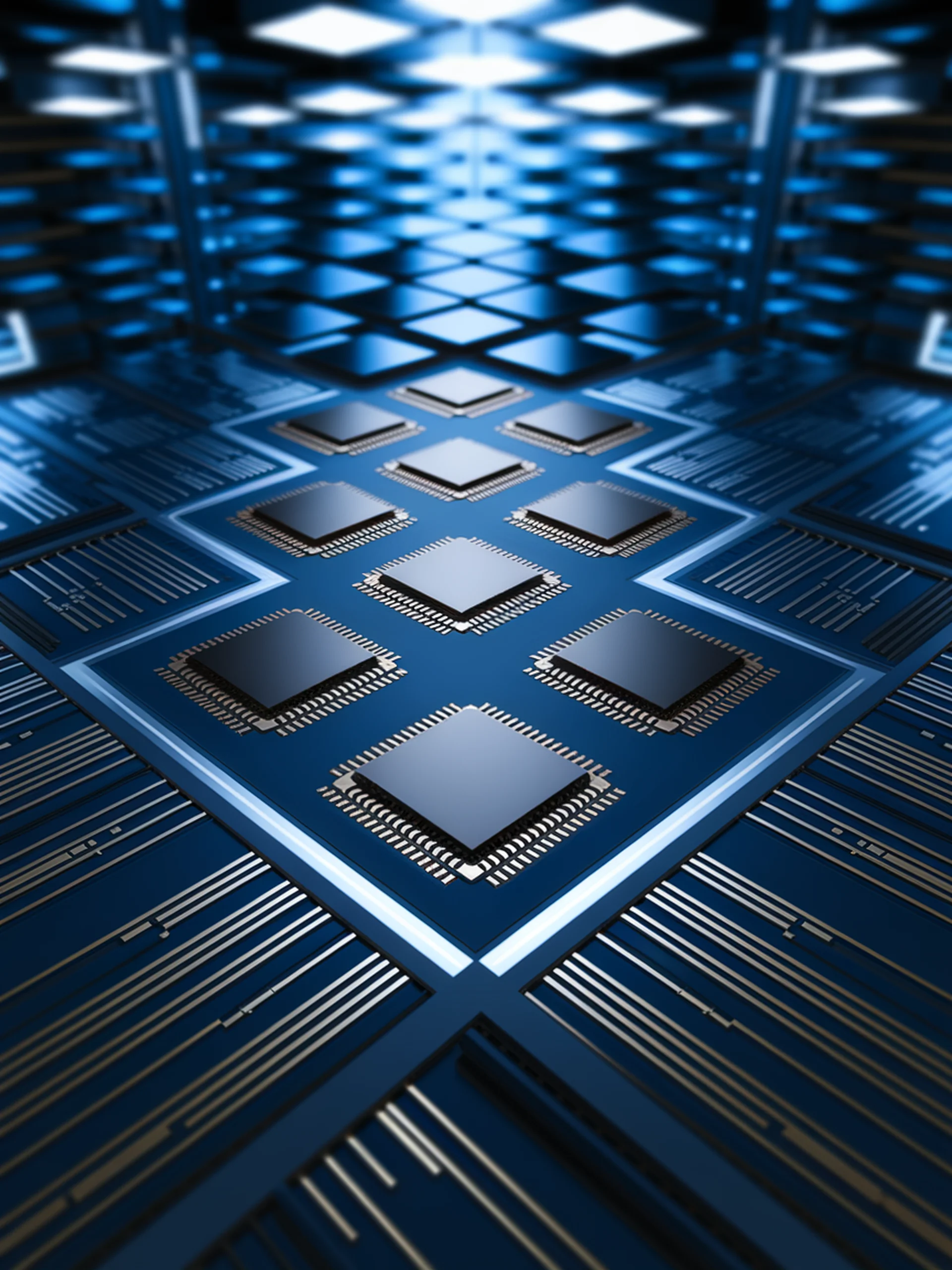
Smart Compression for LLMs
Boosting Efficiency with Adaptive Data Types

Beyond Flat Geometry in LLMs
Improving Model Performance with Curvature-Aware Expert Merging
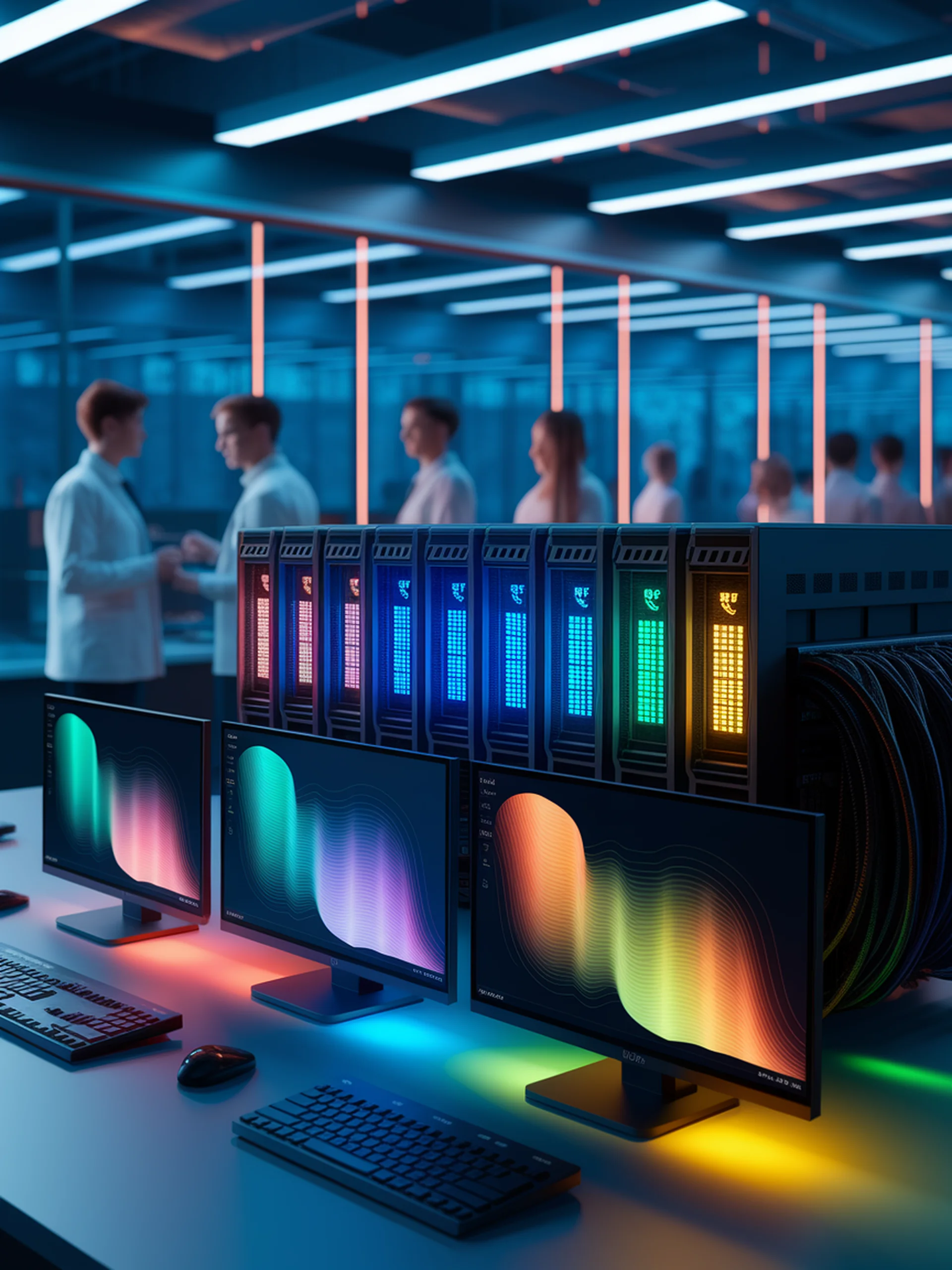
Optimizing Transformer Training Speed
Leveraging Sharpness Disparity for Faster LLM Pre-training
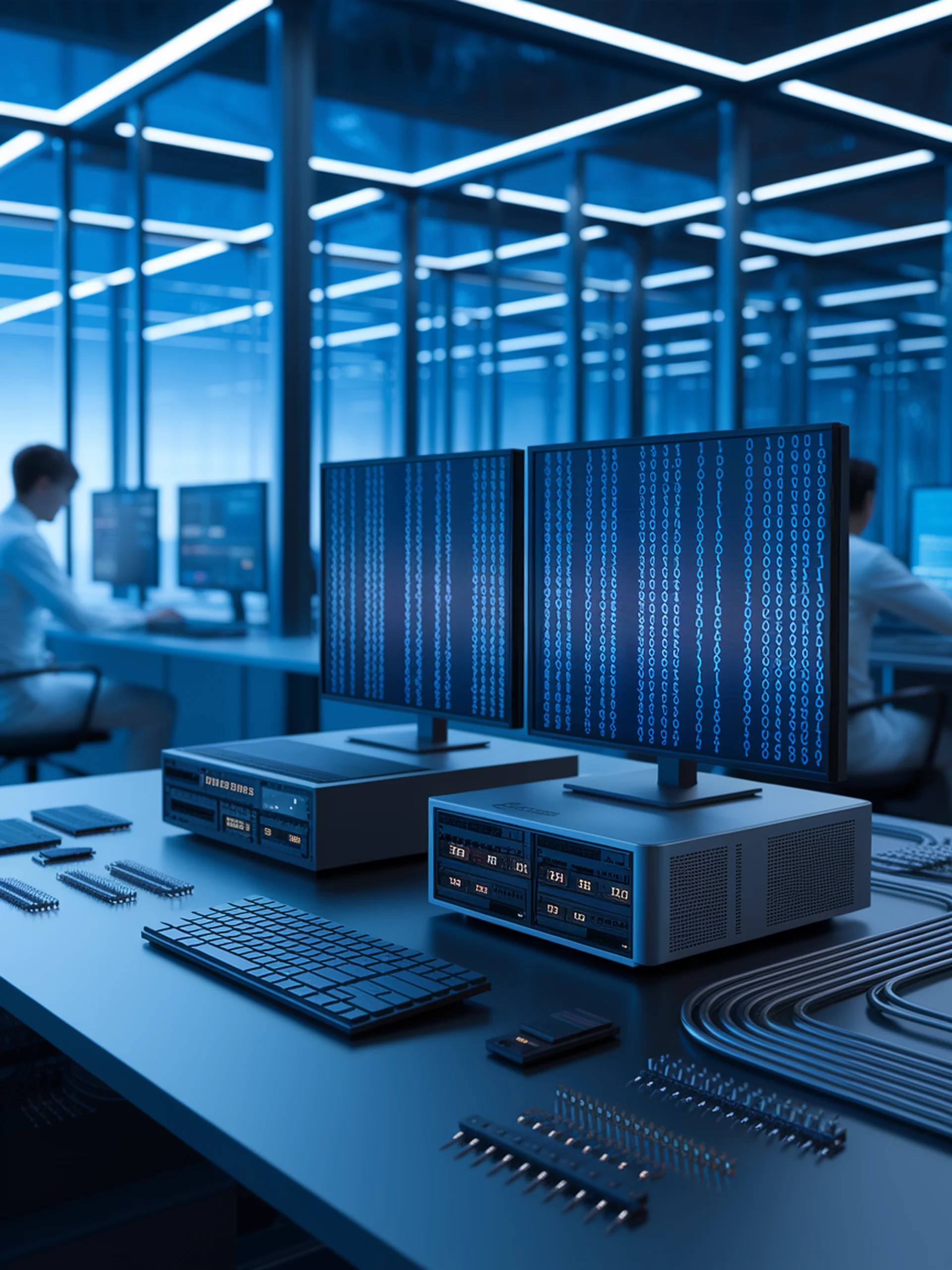
Binary Neural Networks: Shrinking LLMs
Optimizing large language models through extreme quantization
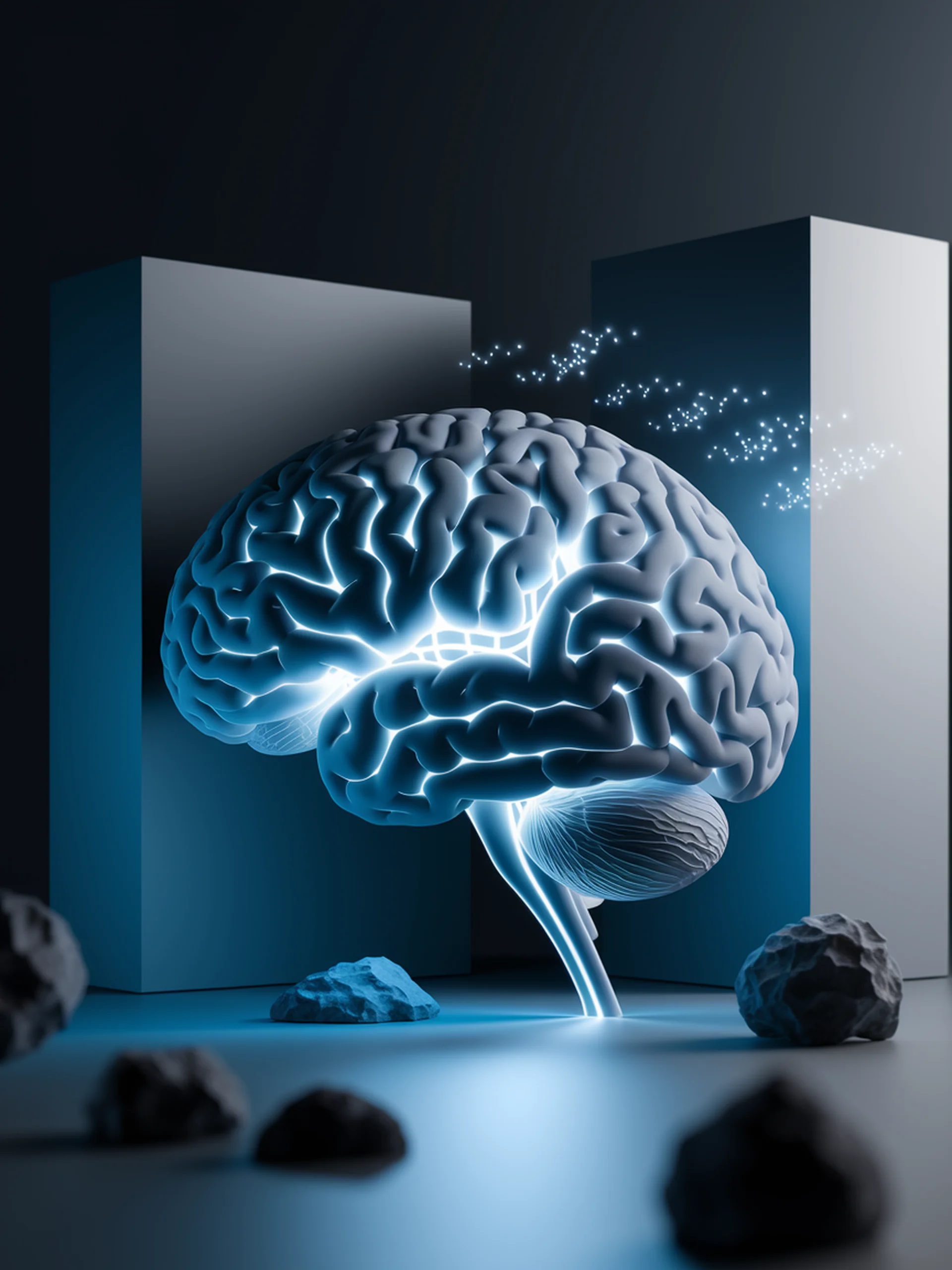
Brain-Inspired LLM Efficiency
How Cognitive Load-Aware Dynamic Activation Makes Language Models Smarter
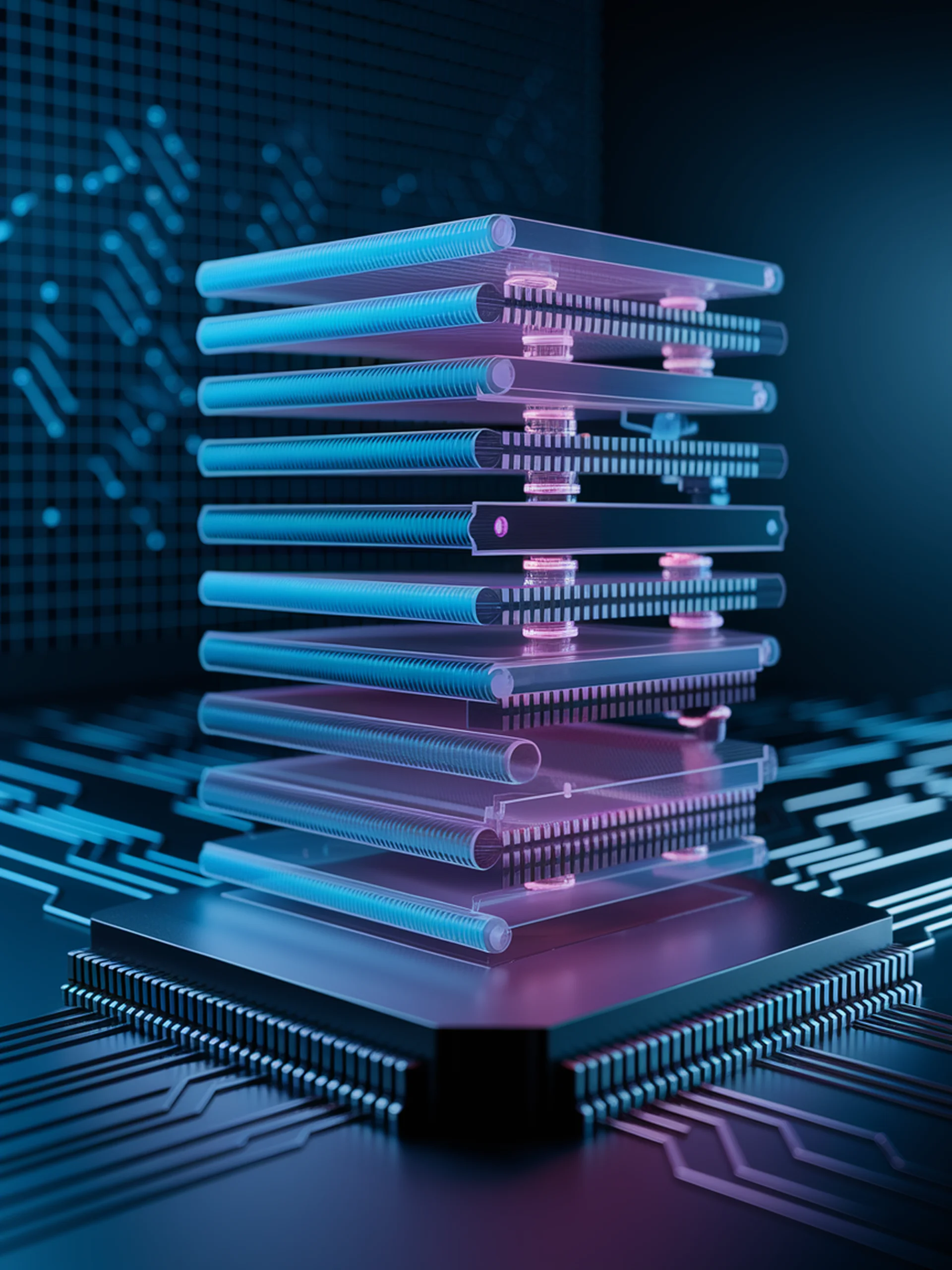
Smarter Layer Pruning for Efficient LLMs
A novel sliding approach to merge layers rather than removing them entirely
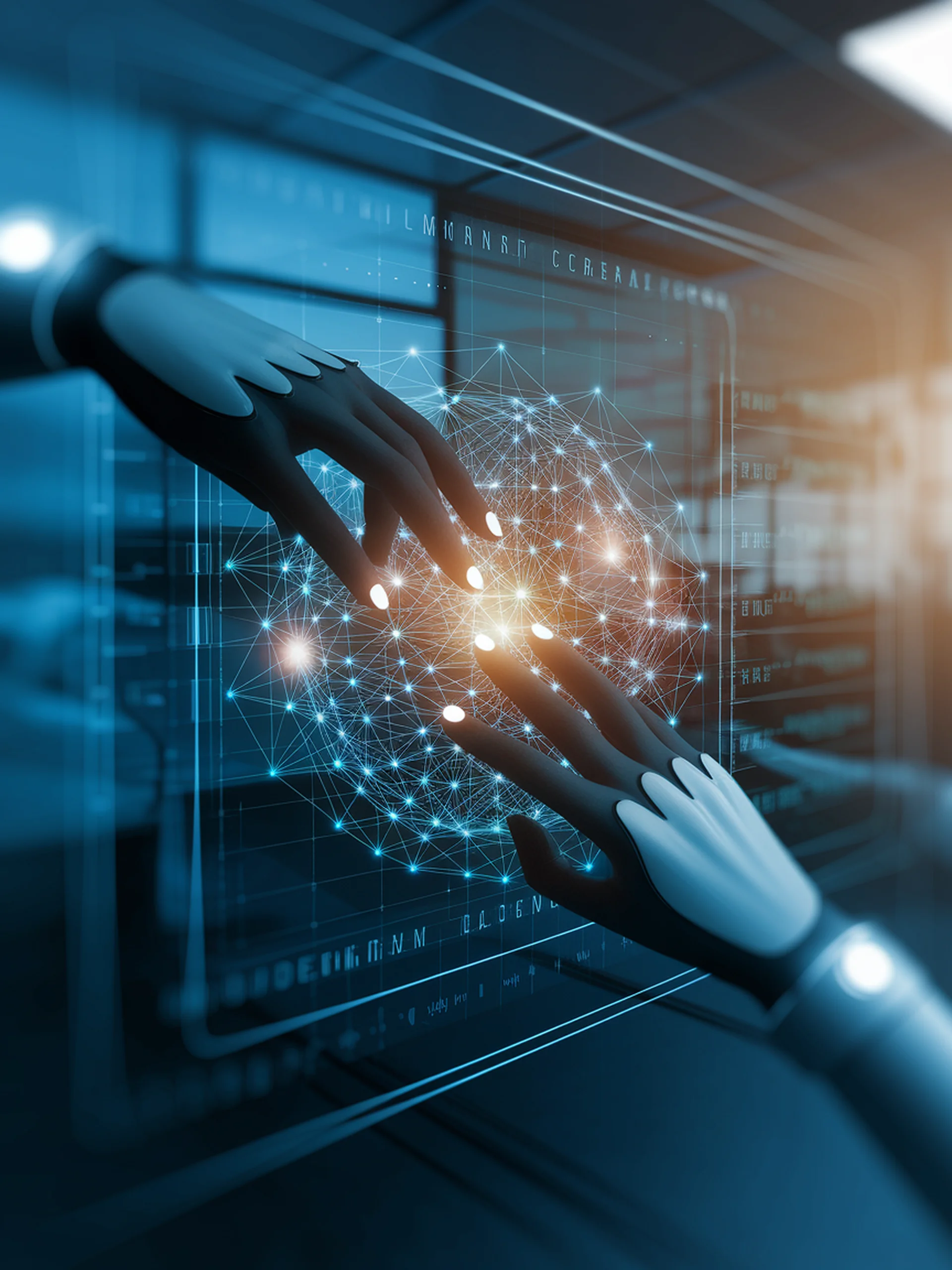
Controlling LLMs from the Inside Out
Representation Engineering: A New Paradigm for LLM Control

Hardware-Optimized LLM Adaptation
Making LoRA models resilient to hardware noise in memory architectures

COMET: Accelerating AI with Efficient MoE Communication
Solving the communication bottleneck in trillion-parameter AI models
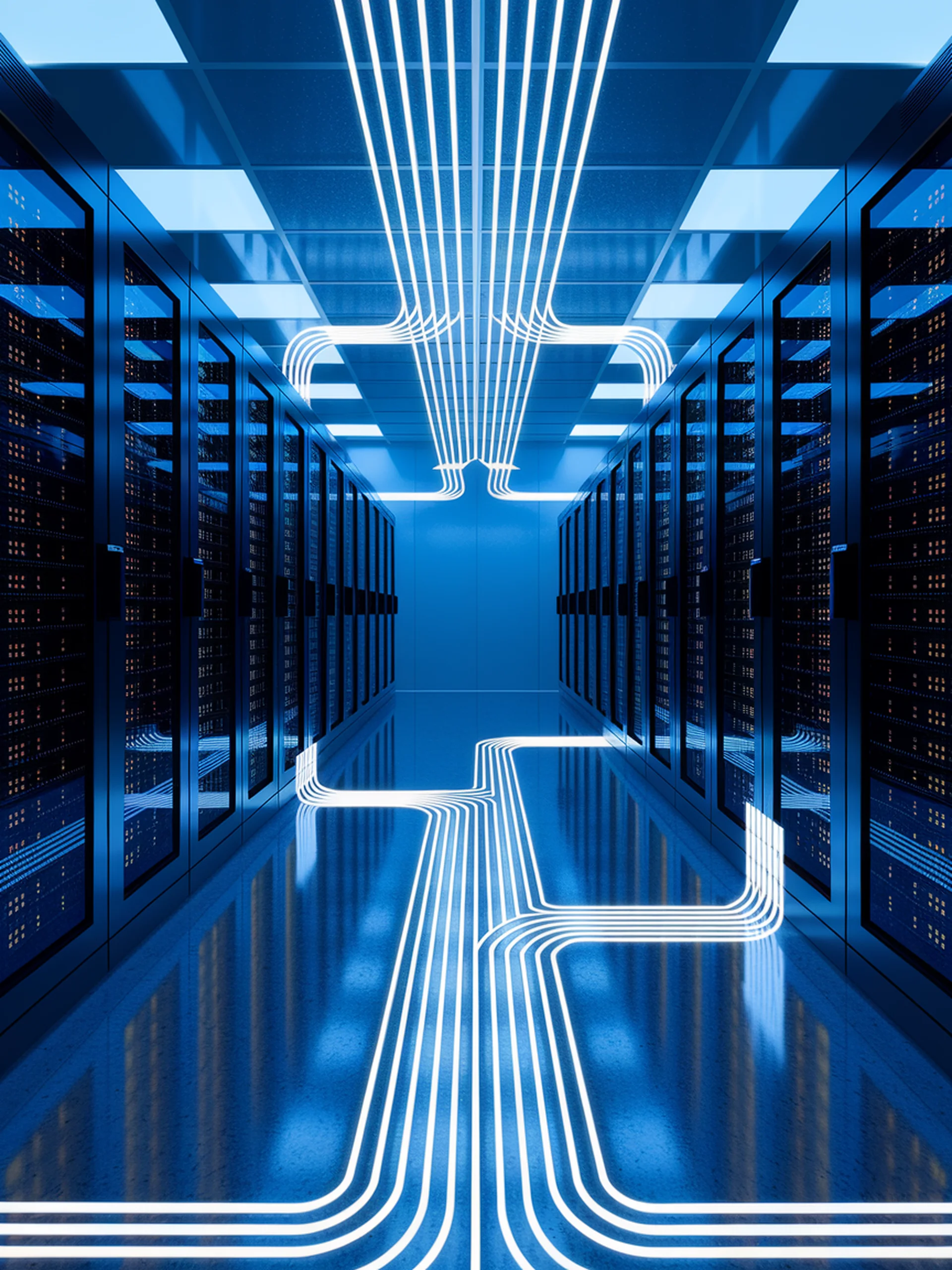
Optimizing LLM Training with SkipPipe
Breaking the sequential pipeline paradigm for faster, cost-effective LLM training

SEKI: Automating AI Design with AI
Using LLMs to Design Neural Networks Through Self-Evolution
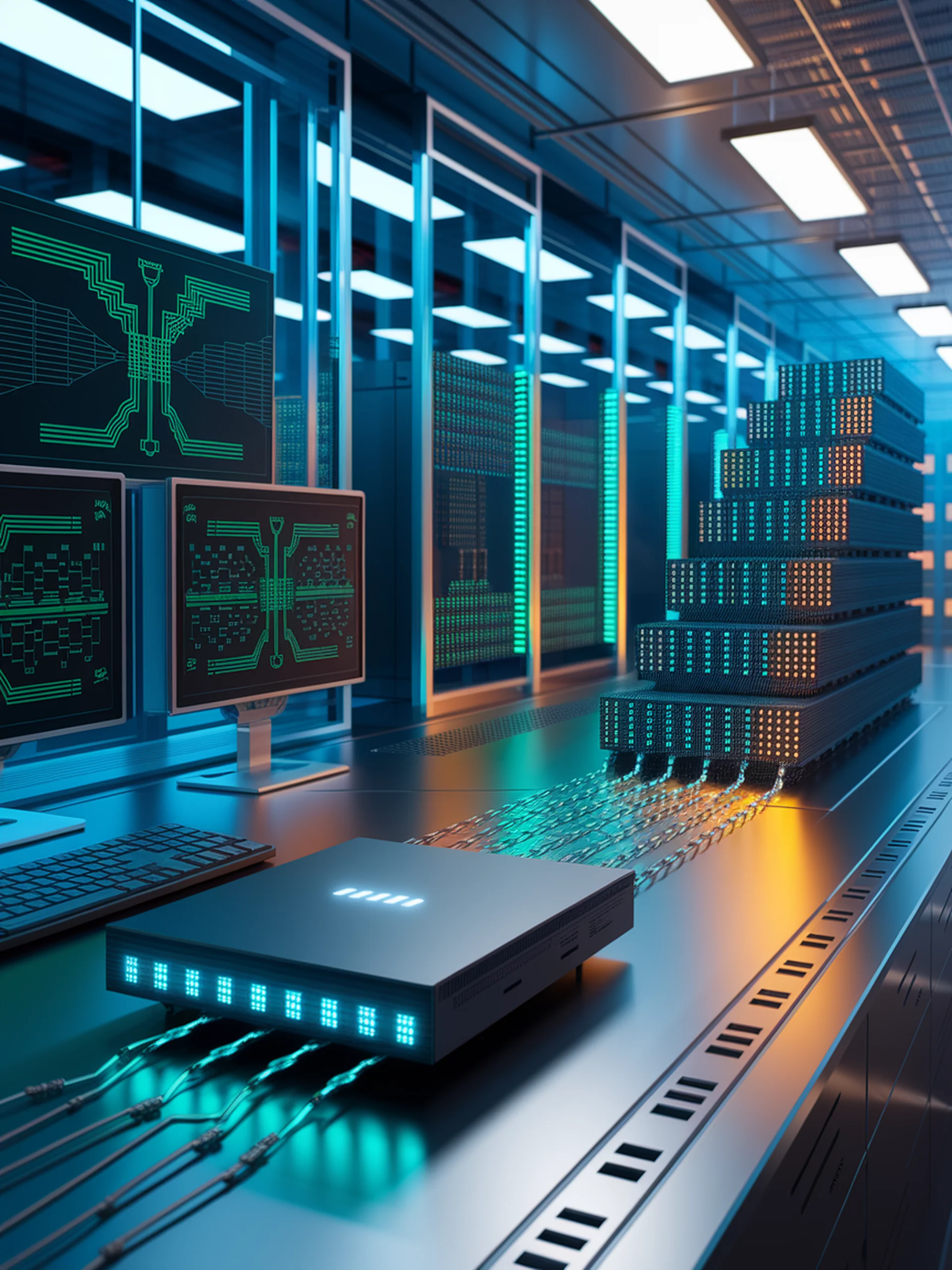
Smart LLM Routing
Balancing Capability and Cost in Multi-LLM Systems
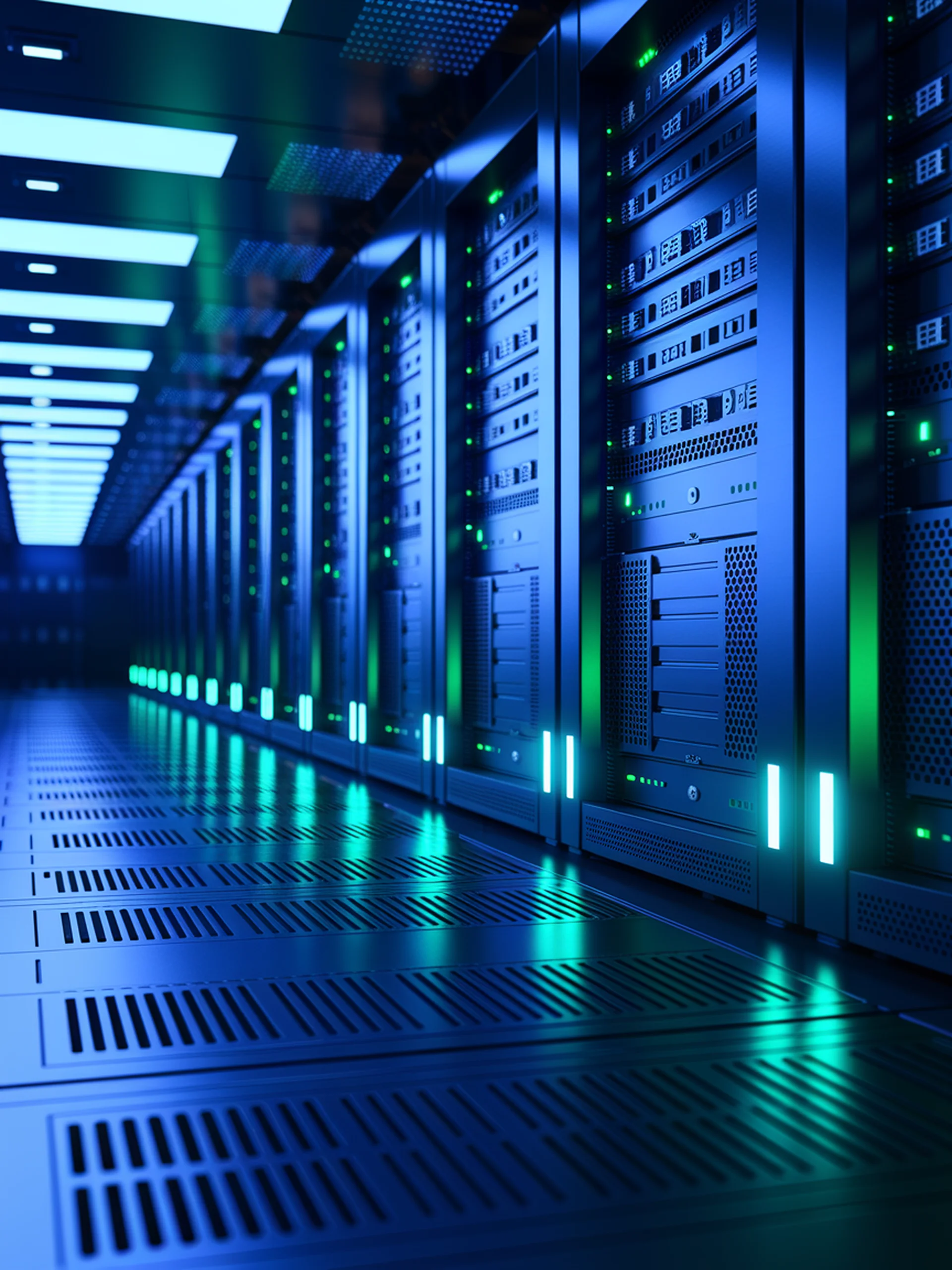
Optimizing LLM Inference Across Multiple GPUs
Reducing Communication Bottlenecks in Tensor Parallelism
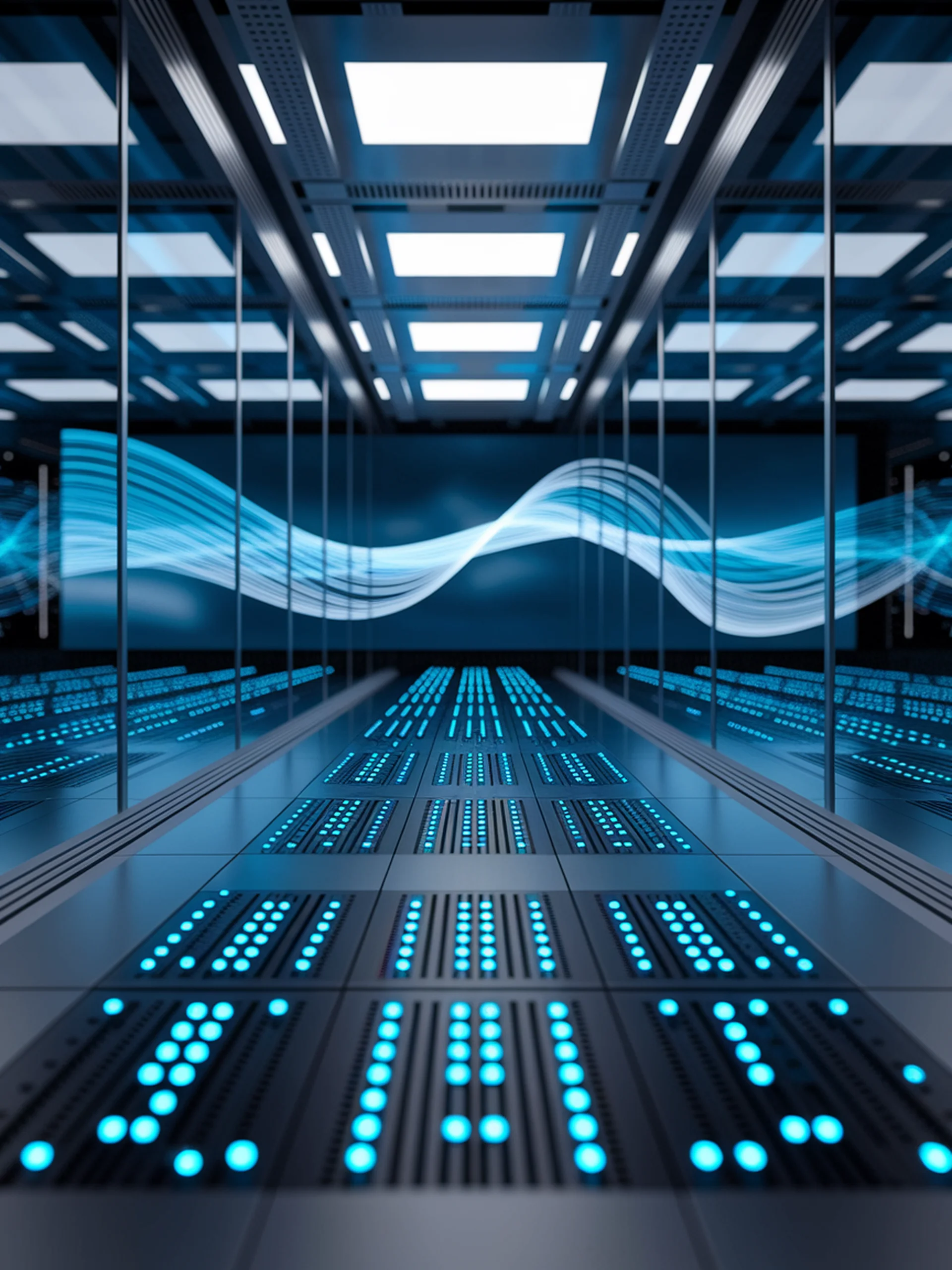
Accelerating RAG Systems
Reducing latency with innovative lookahead retrieval techniques
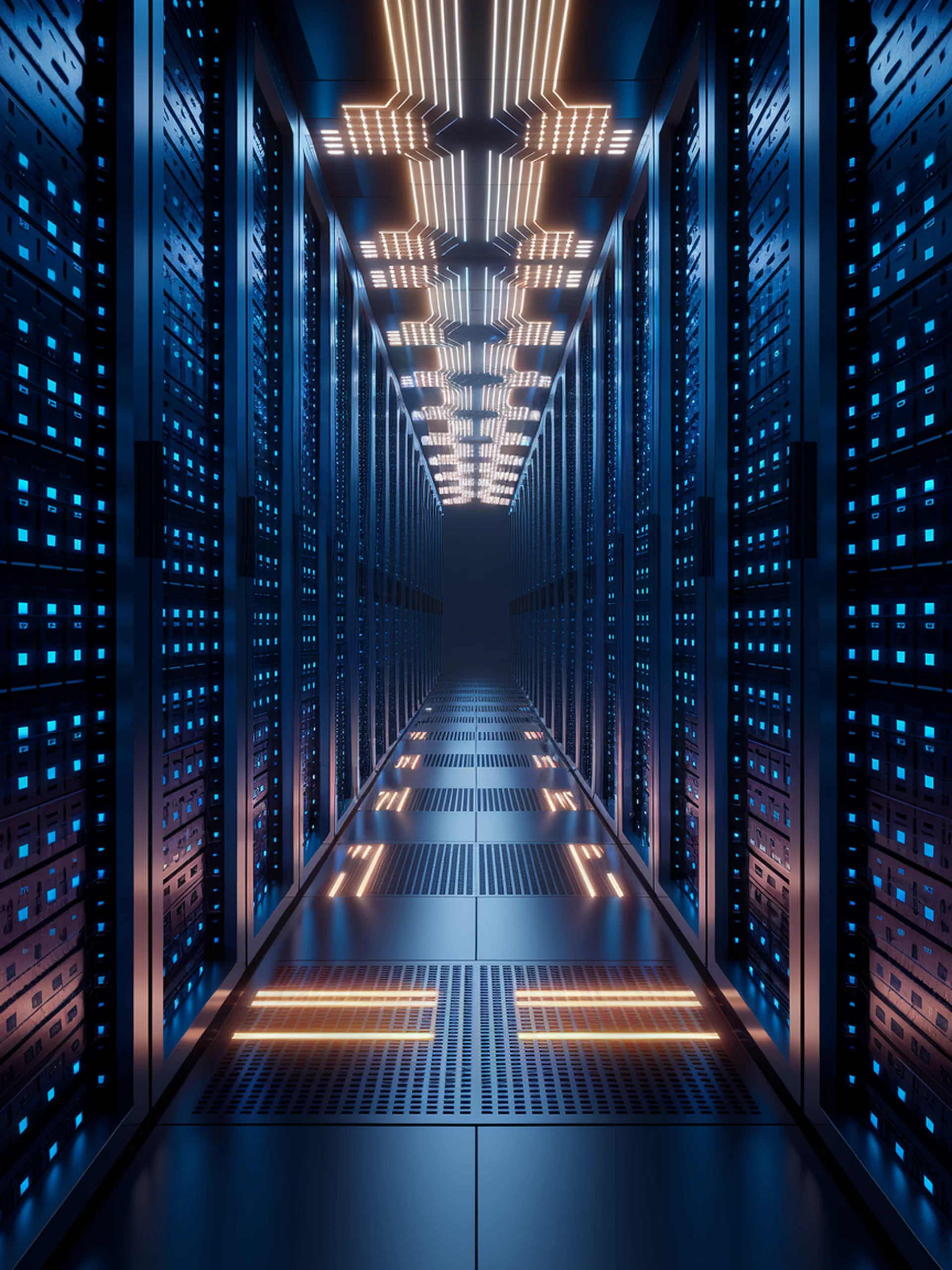
Breaking the Context Barrier: ByteScale
Efficient LLM Training with 2048K Context Length on 12,000+ GPUs
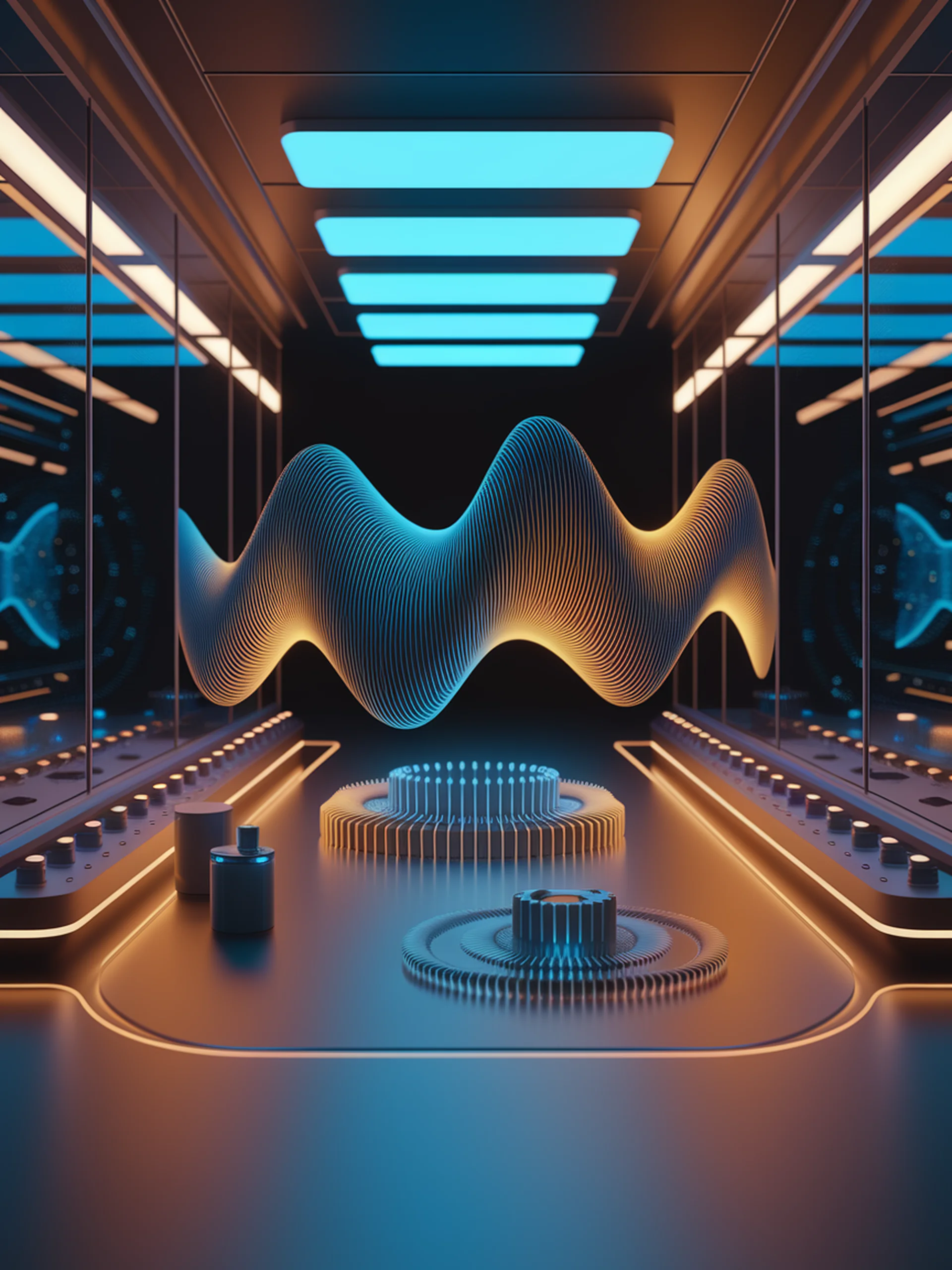
FANformer: Enhancing LLMs Through Periodicity
A novel architecture improving how language models recognize patterns

Shrinking Memory Footprints for LLMs
Optimizing Key-Value Caches to Scale Inference Efficiency
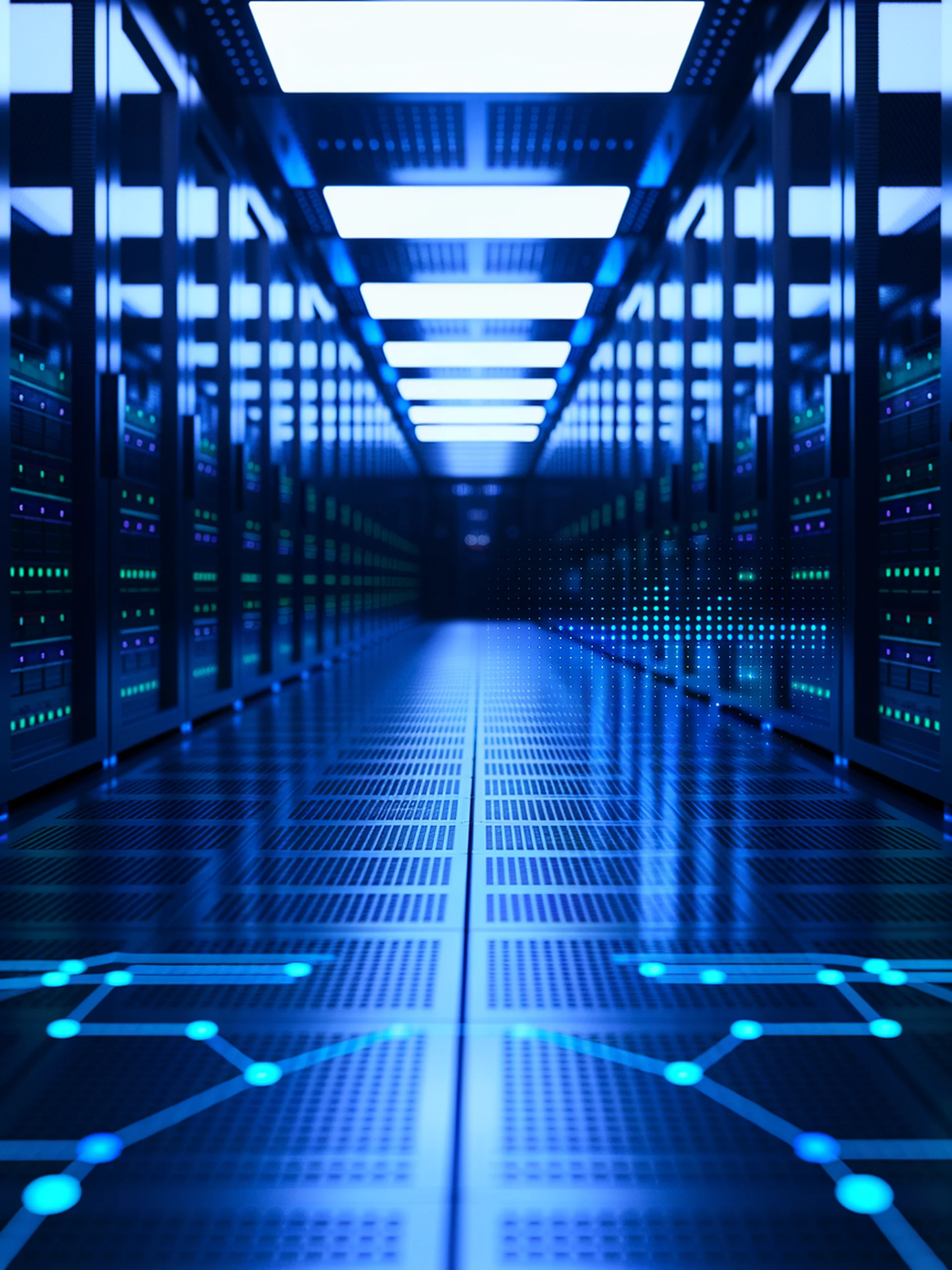
Progressive Sparse Attention
Optimizing LLM Performance for Long-Context Tasks
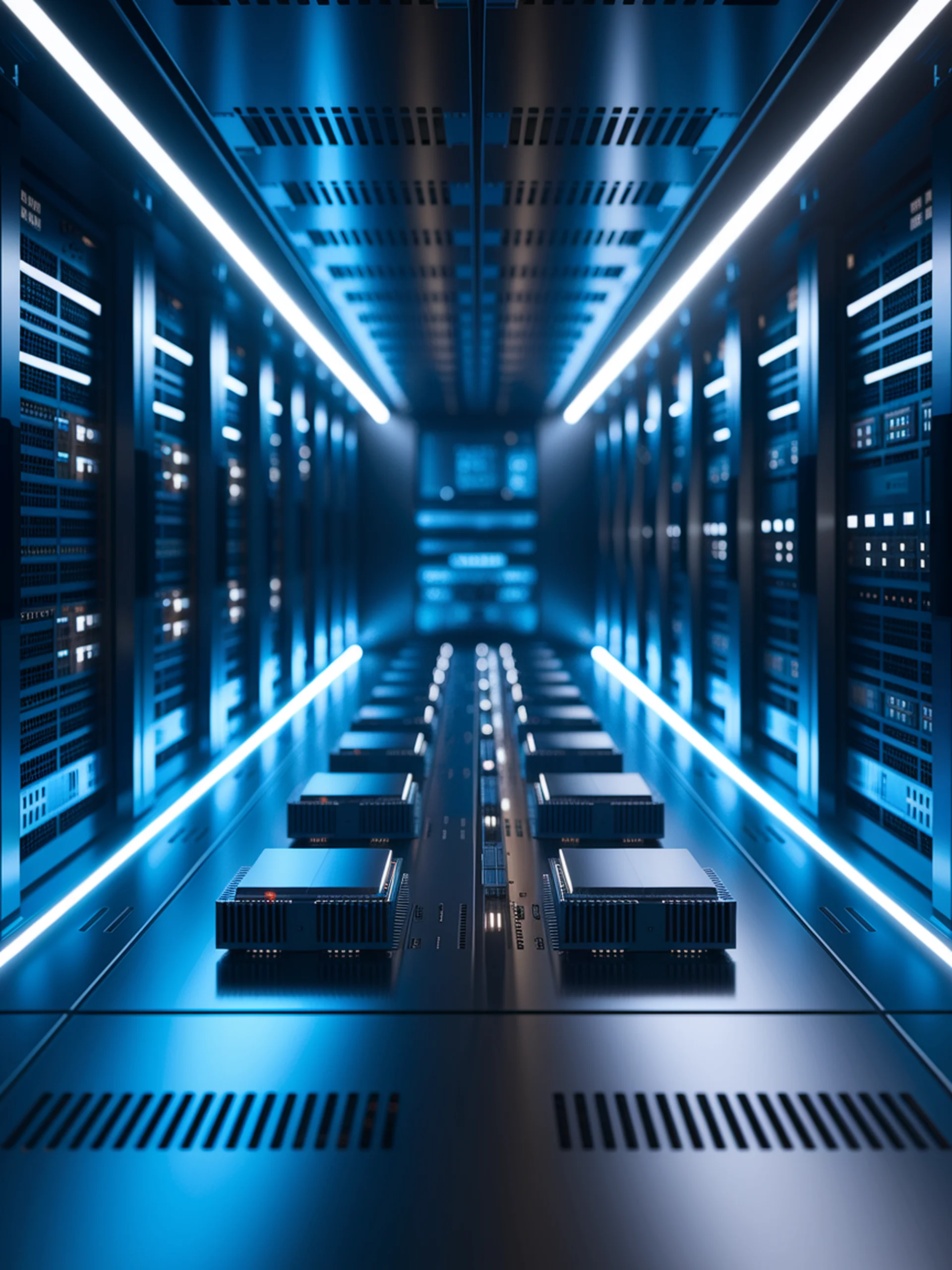
Accelerating LLM Inference with Smart Decoding
Hardware-aware optimization through heterogeneous speculative techniques
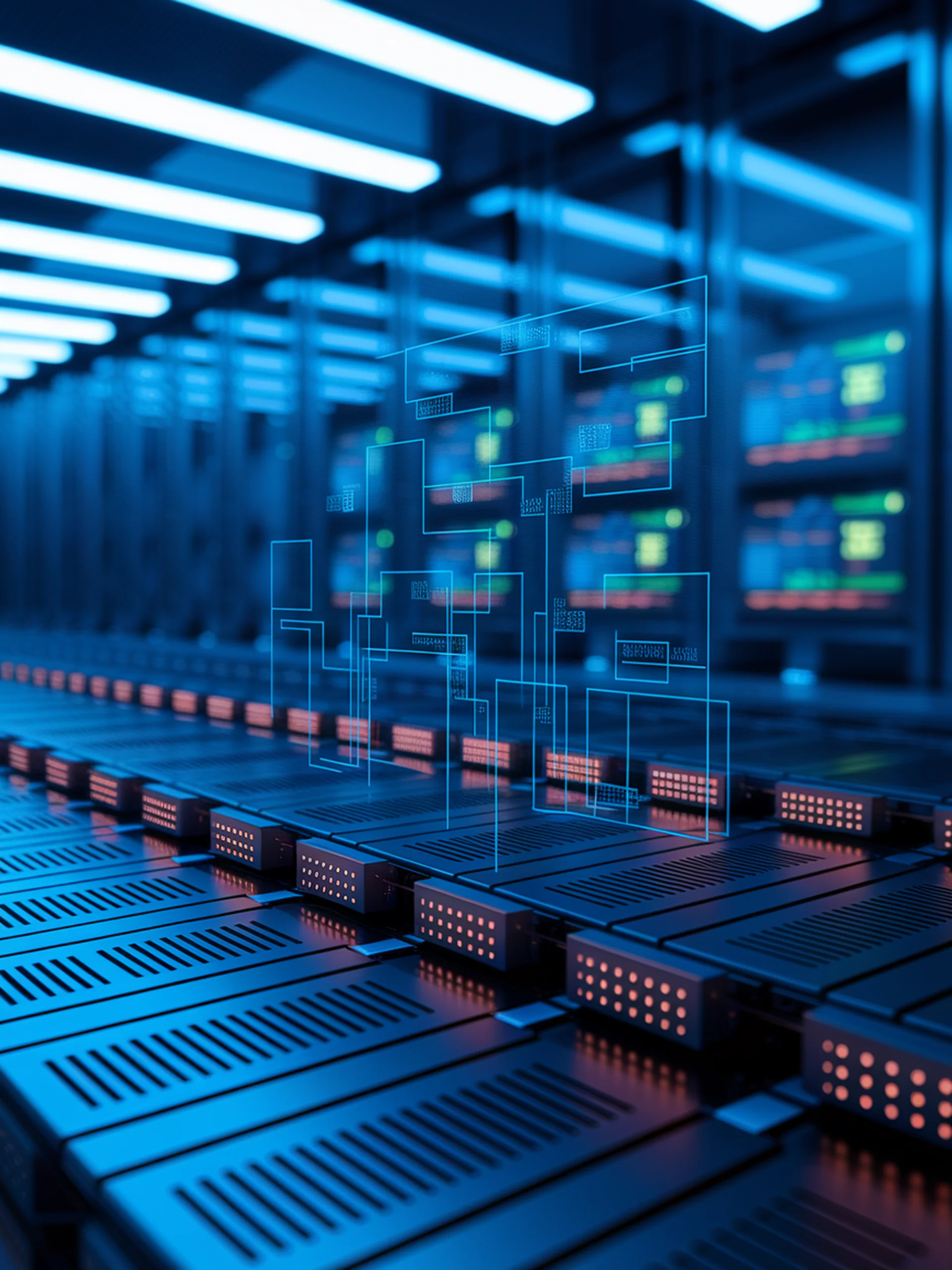
Making LLMs Efficient for CTR Prediction
A Novel Training Paradigm for Recommendation Systems
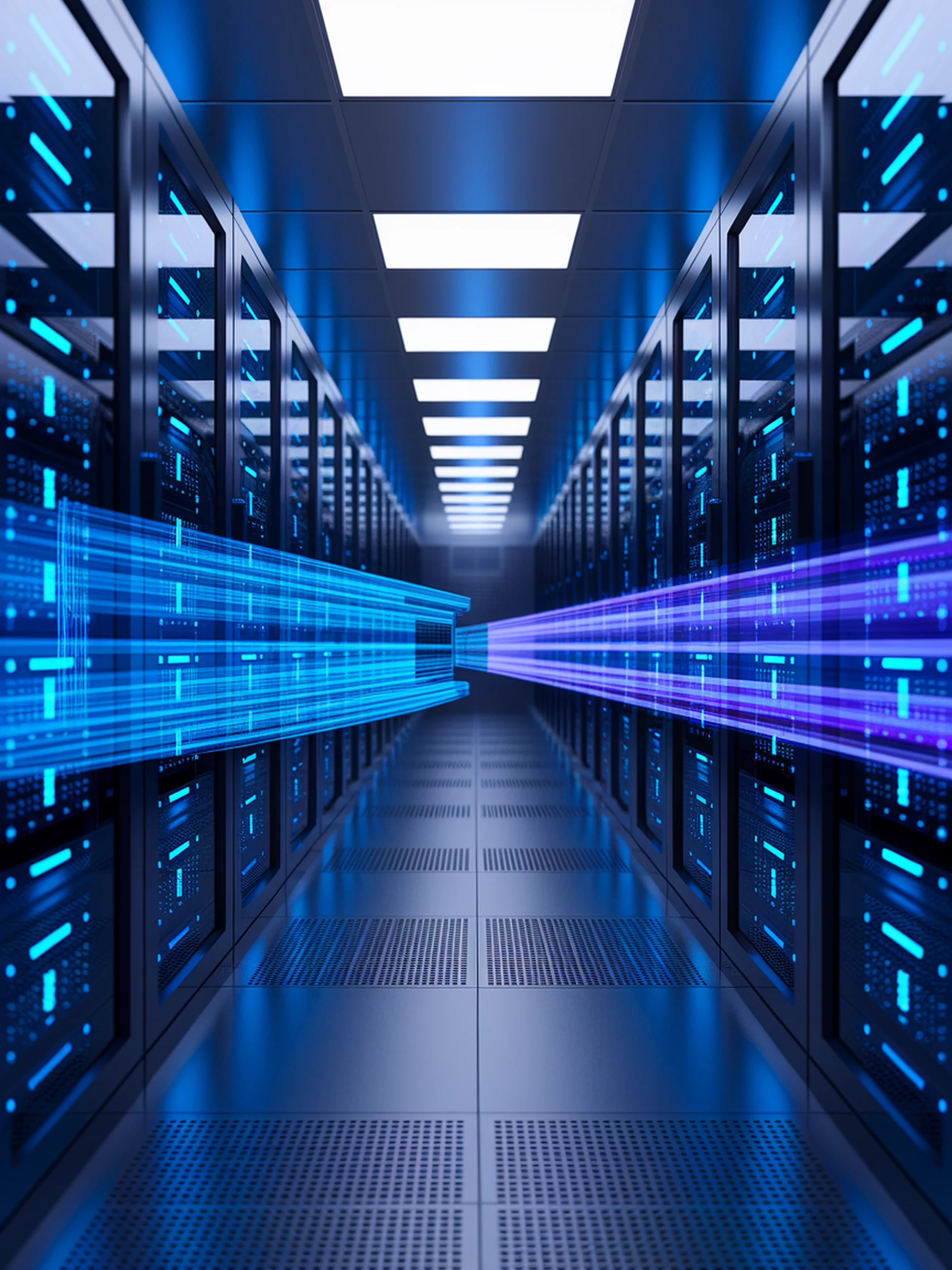
Memory Optimization for LLM Training
Enhancing Pipeline Parallelism with Strategic Memory Offloading
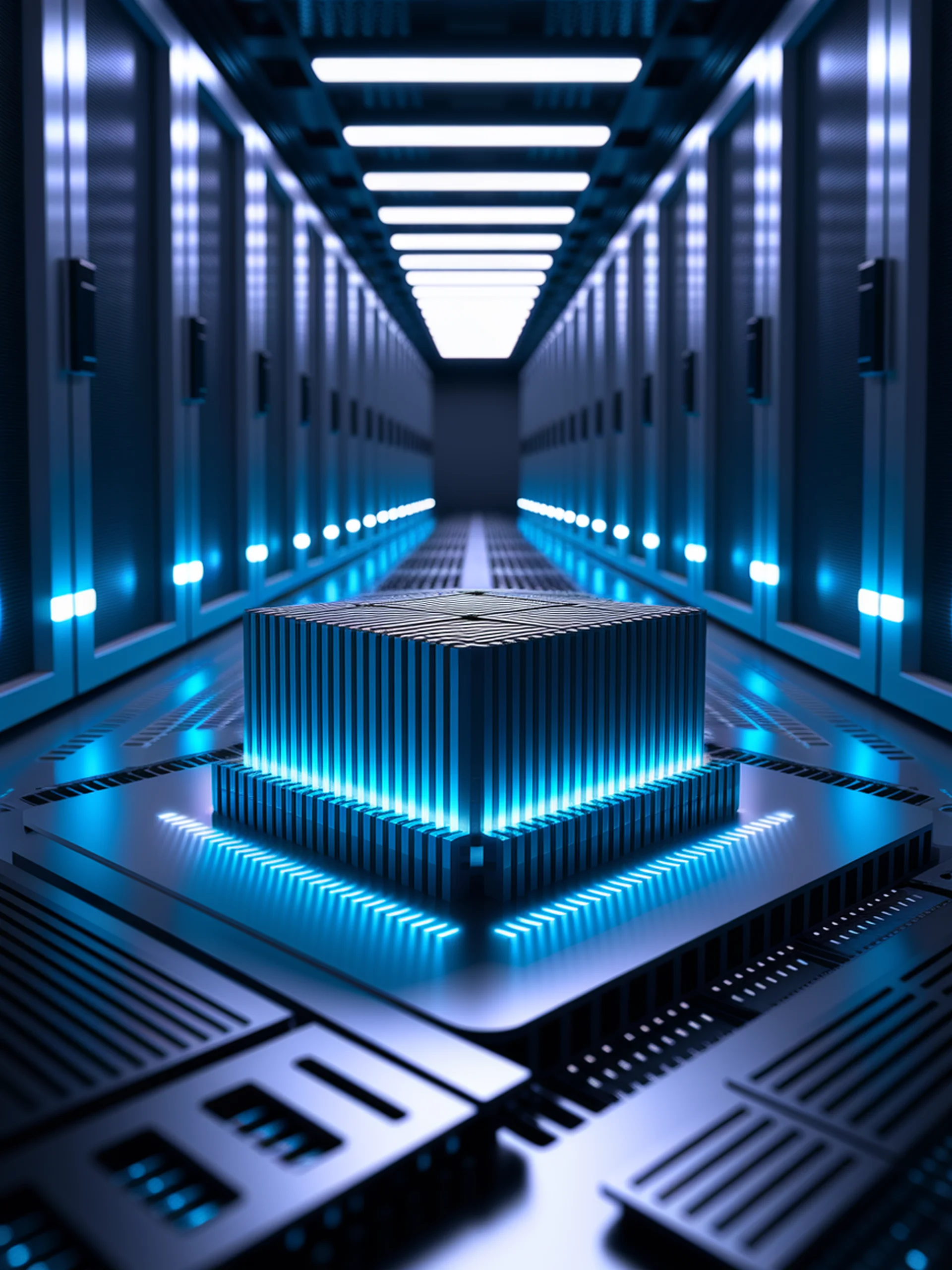
Smarter Memory for LLMs
Optimizing KV cache for efficient text generation
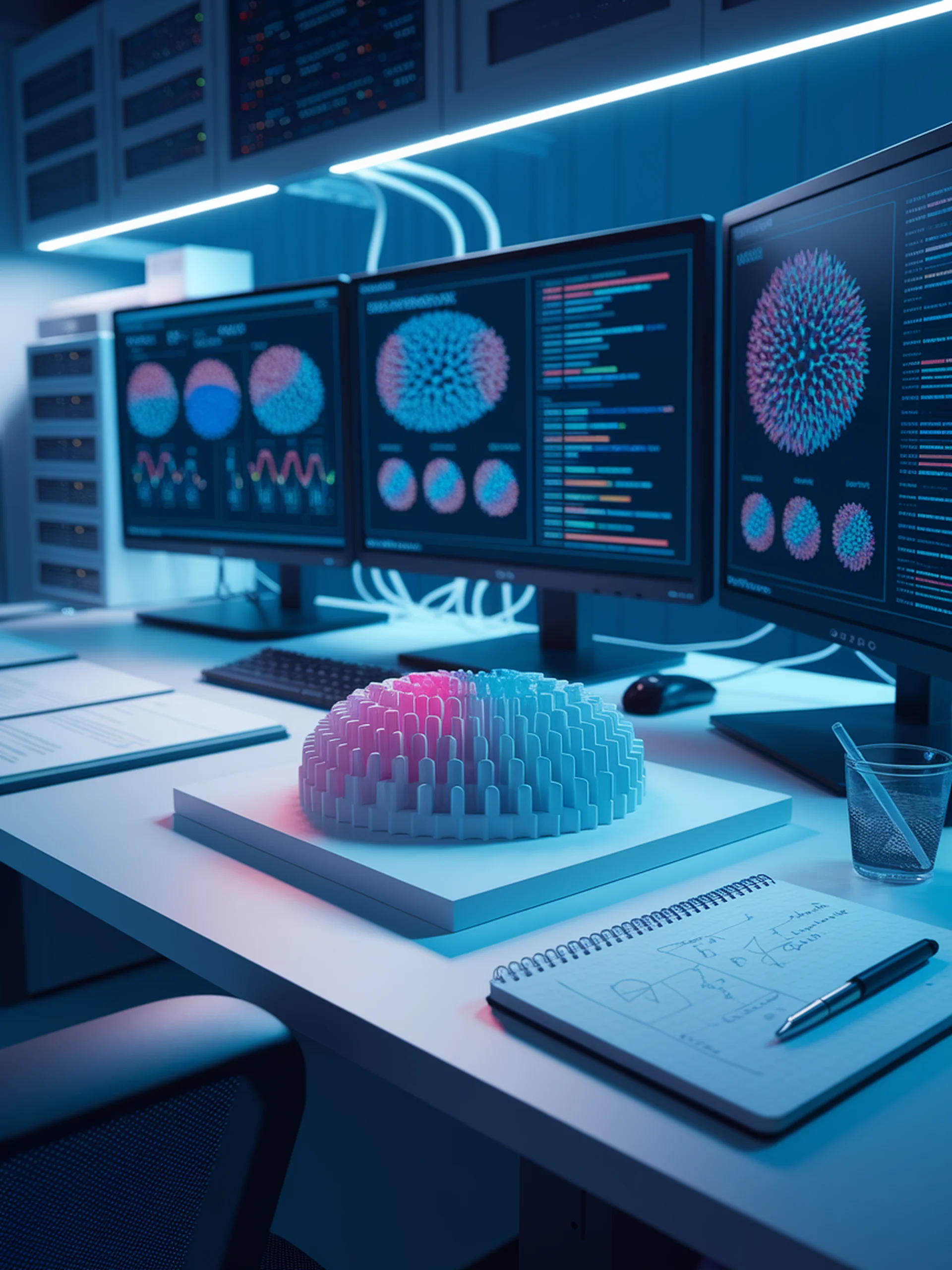
LLM Quantization Breakthrough
Using Kurtosis to Tackle Outliers in Model Compression
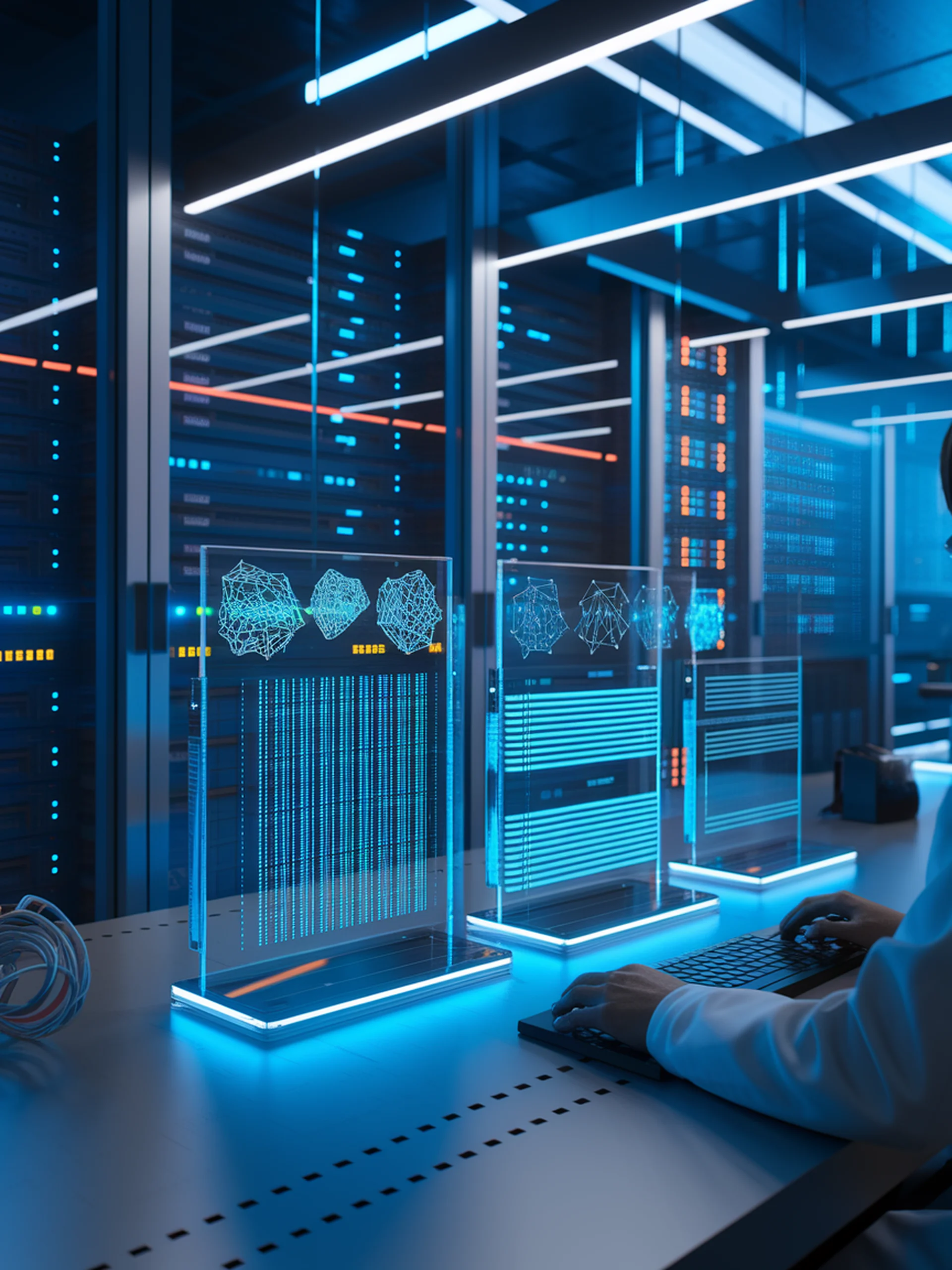
Streamlining LLMs with Linear Recurrence
Transforming standard models into more efficient structures without retraining
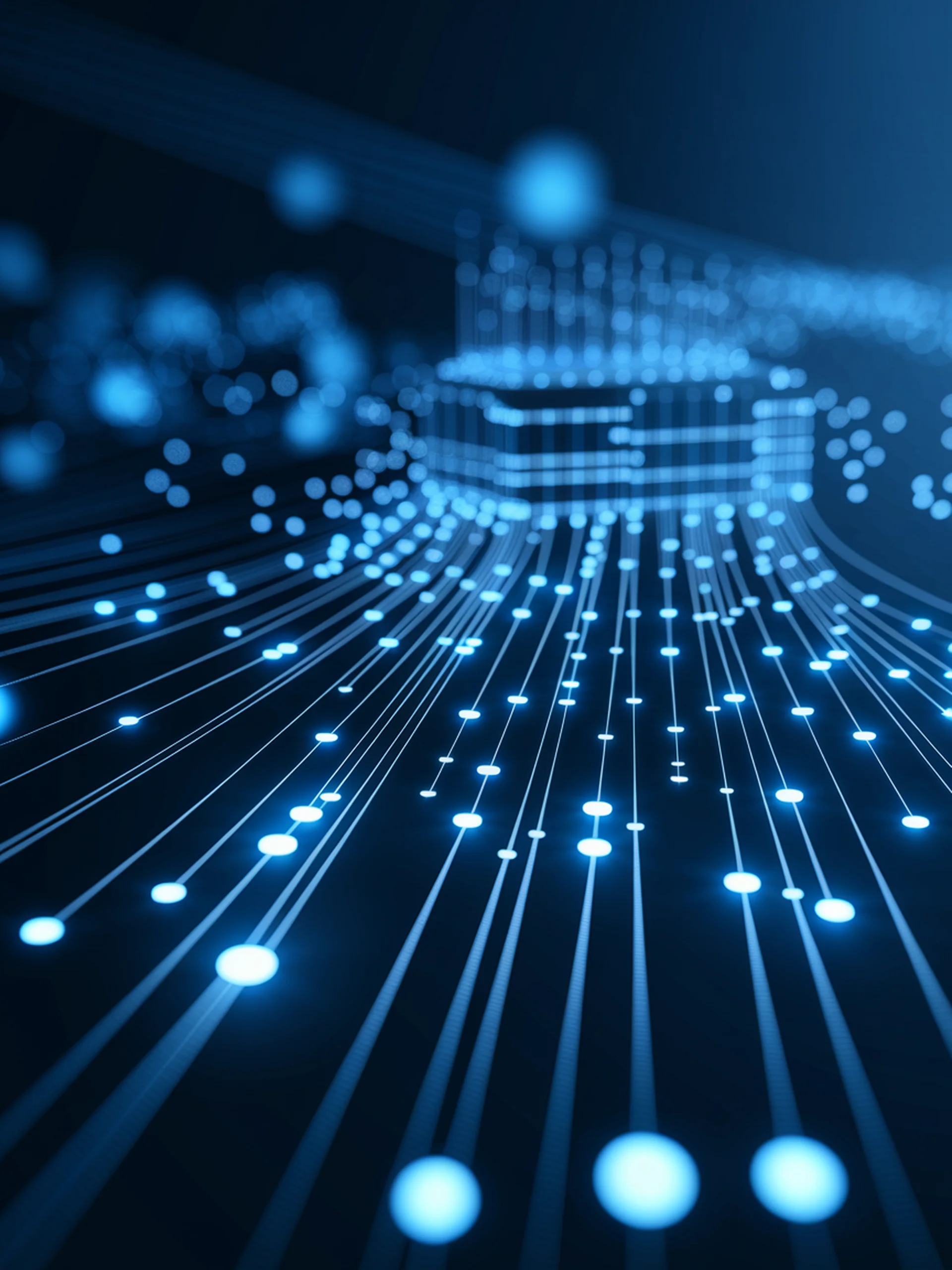
Speeding Up LLMs Through Smart Attention
Making large language models faster with attention sparsity
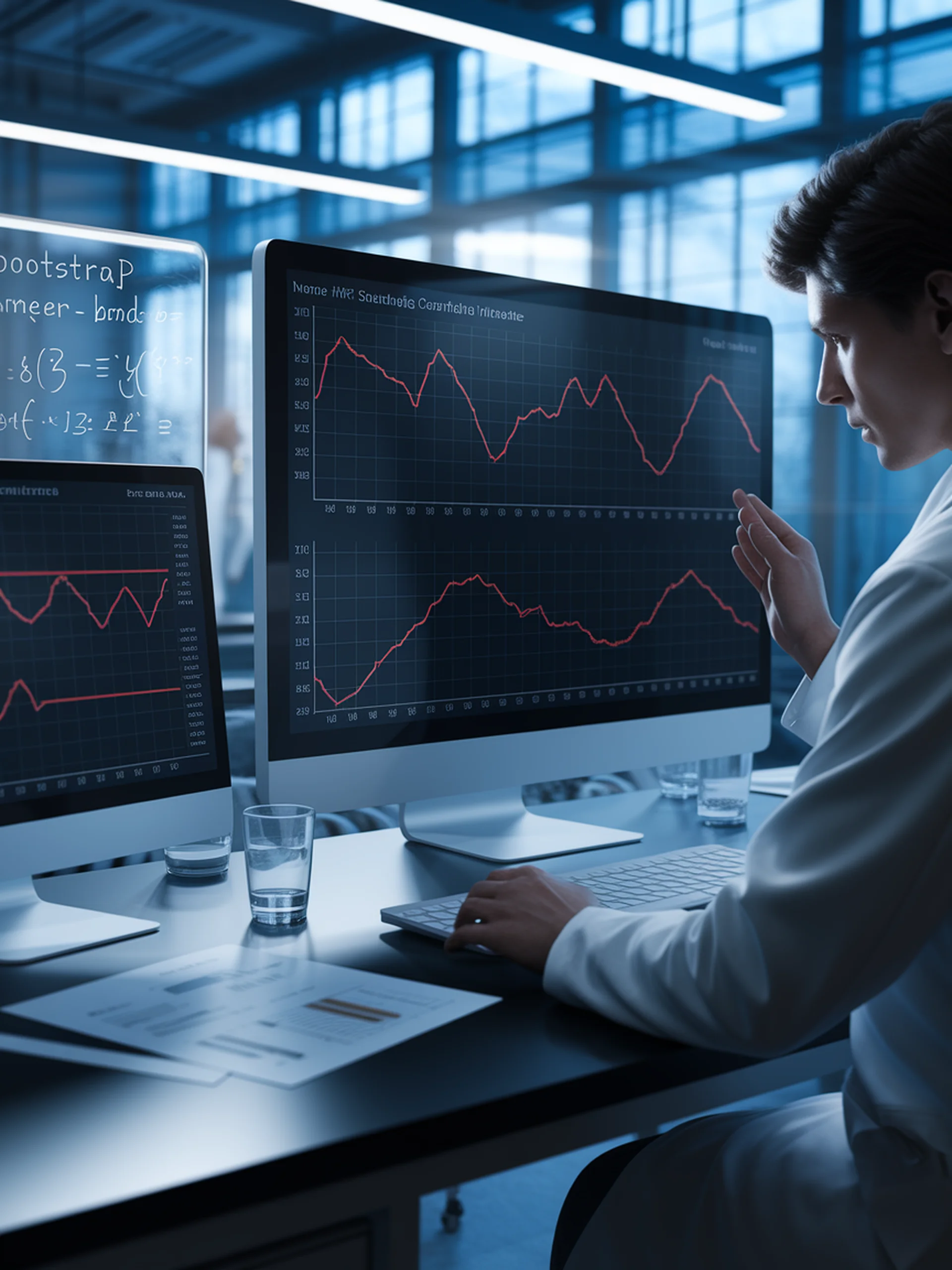
Statistical Pitfalls in LLM Evaluation
Why the Central Limit Theorem fails for small datasets
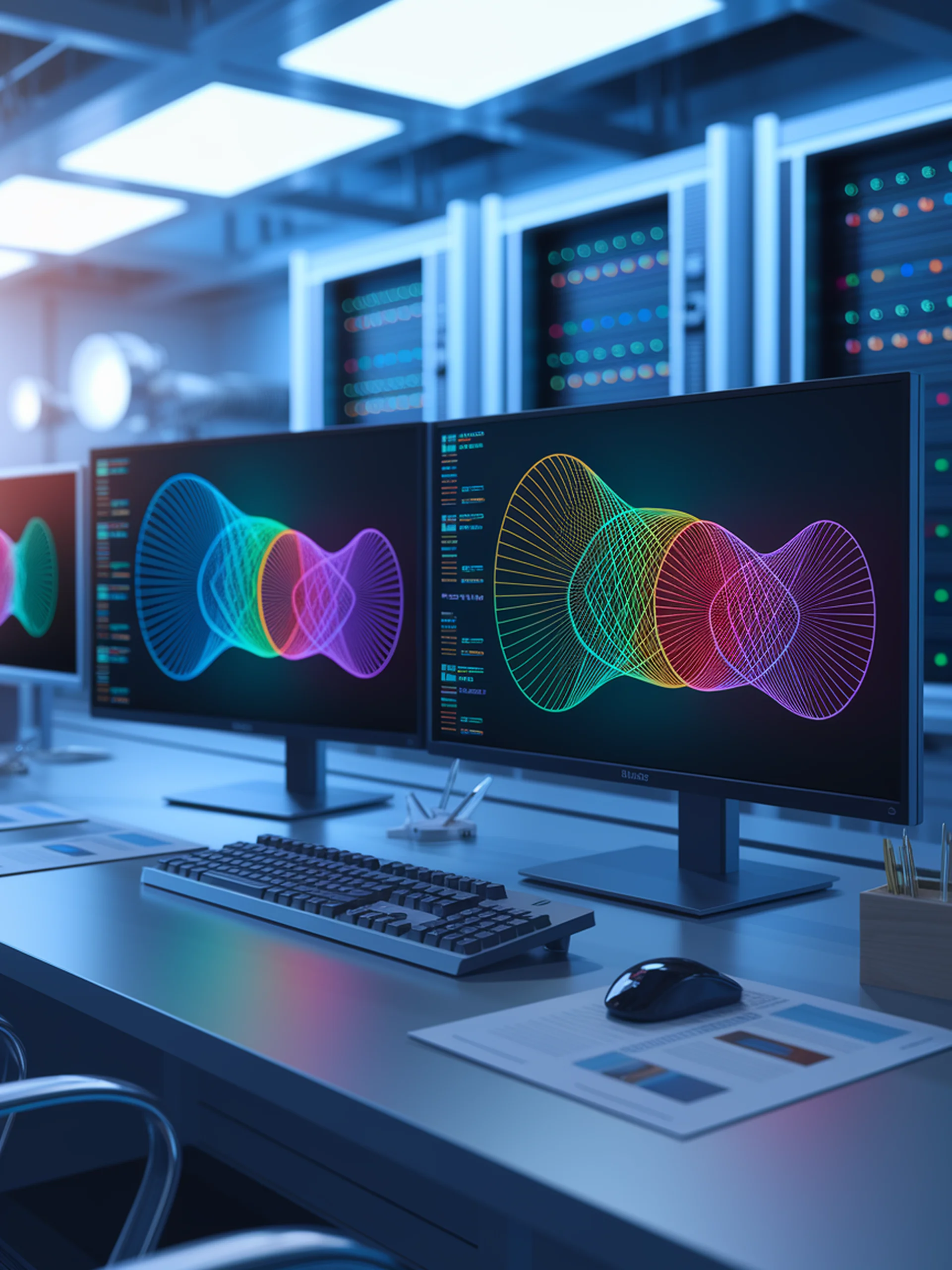
Unlocking the Secrets of Rotary Embeddings in LLMs
Revealing hidden patterns in positional encoding mechanisms

EAGLE-3: Scaling up Inference Acceleration of Large Language...
By Yuhui Li, Fangyun Wei...
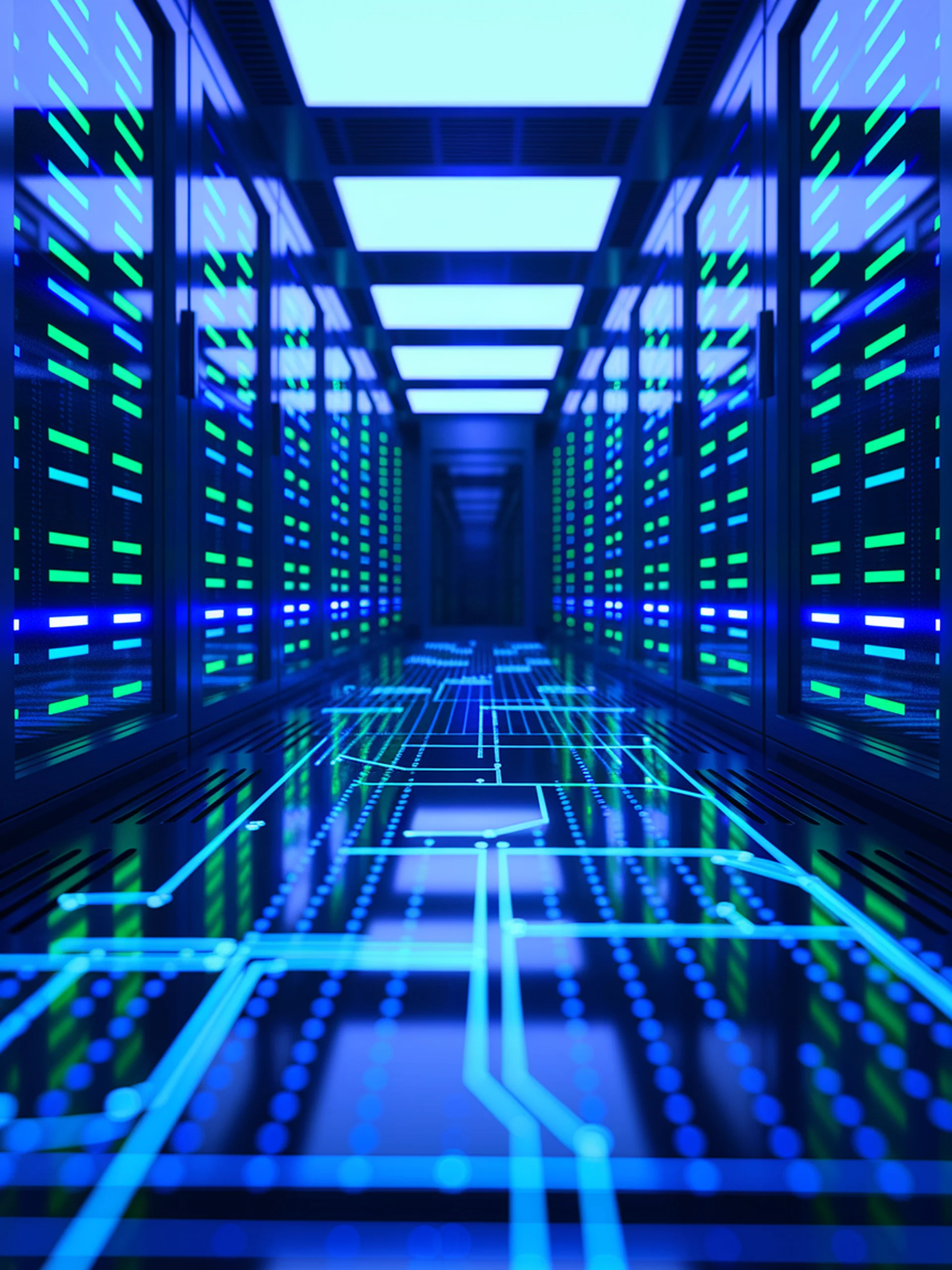
Accelerating LLM Inference with PASA
A robust low-precision attention algorithm that eliminates overflow issues
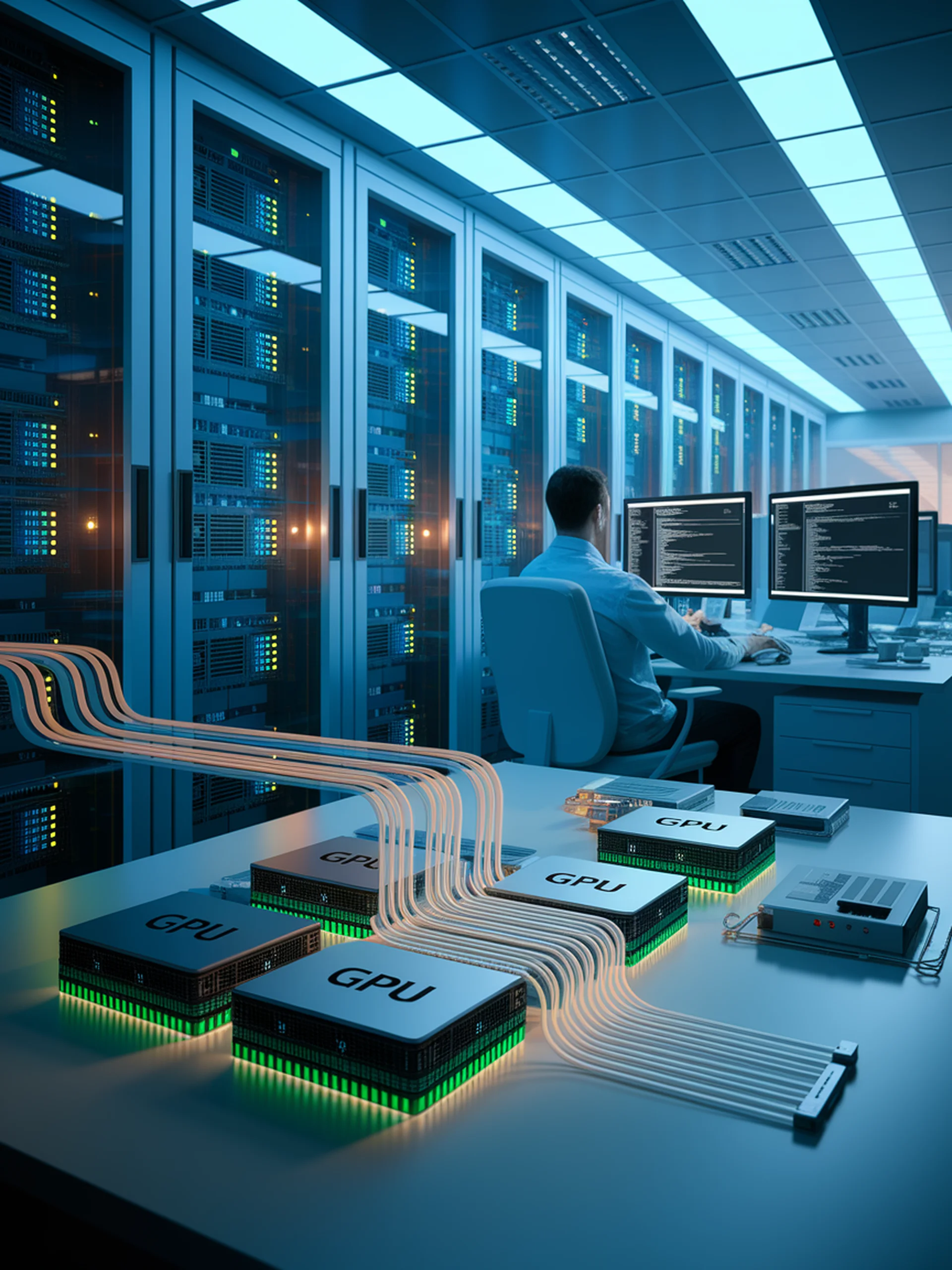
Breaking GPU Memory Barriers
Automating Efficient Heterogeneous Training for Large Language Models

Optimizing LLMs Through Smart Weight Quantization
A novel approach to identify and preserve critical model weights
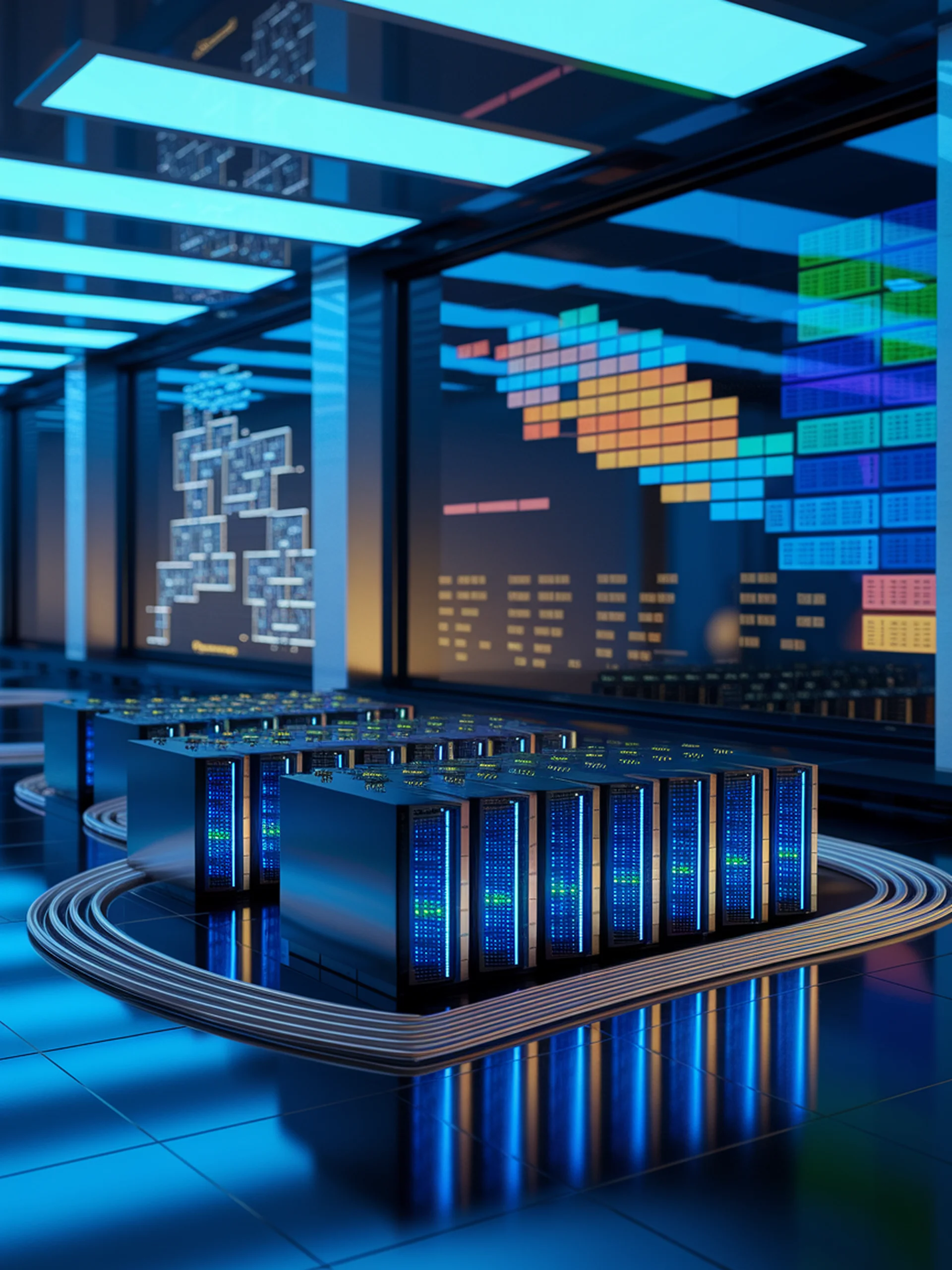
ChunkFlow: Solving the Long Context Challenge
A more efficient approach to fine-tuning LLMs on variable-length sequences
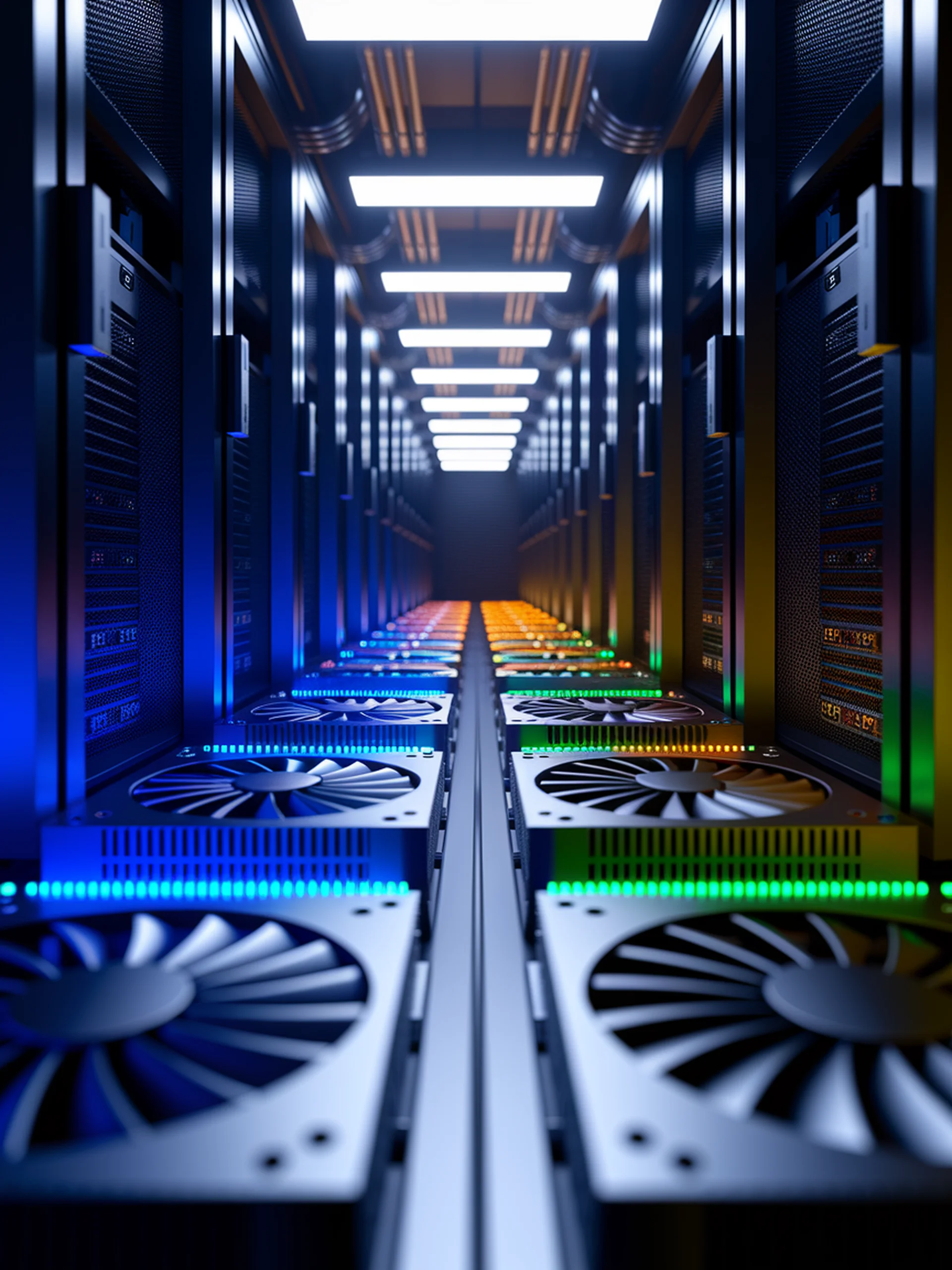
Maximizing GPU Efficiency in AI Training
Using Idle Resources for Inference During Training
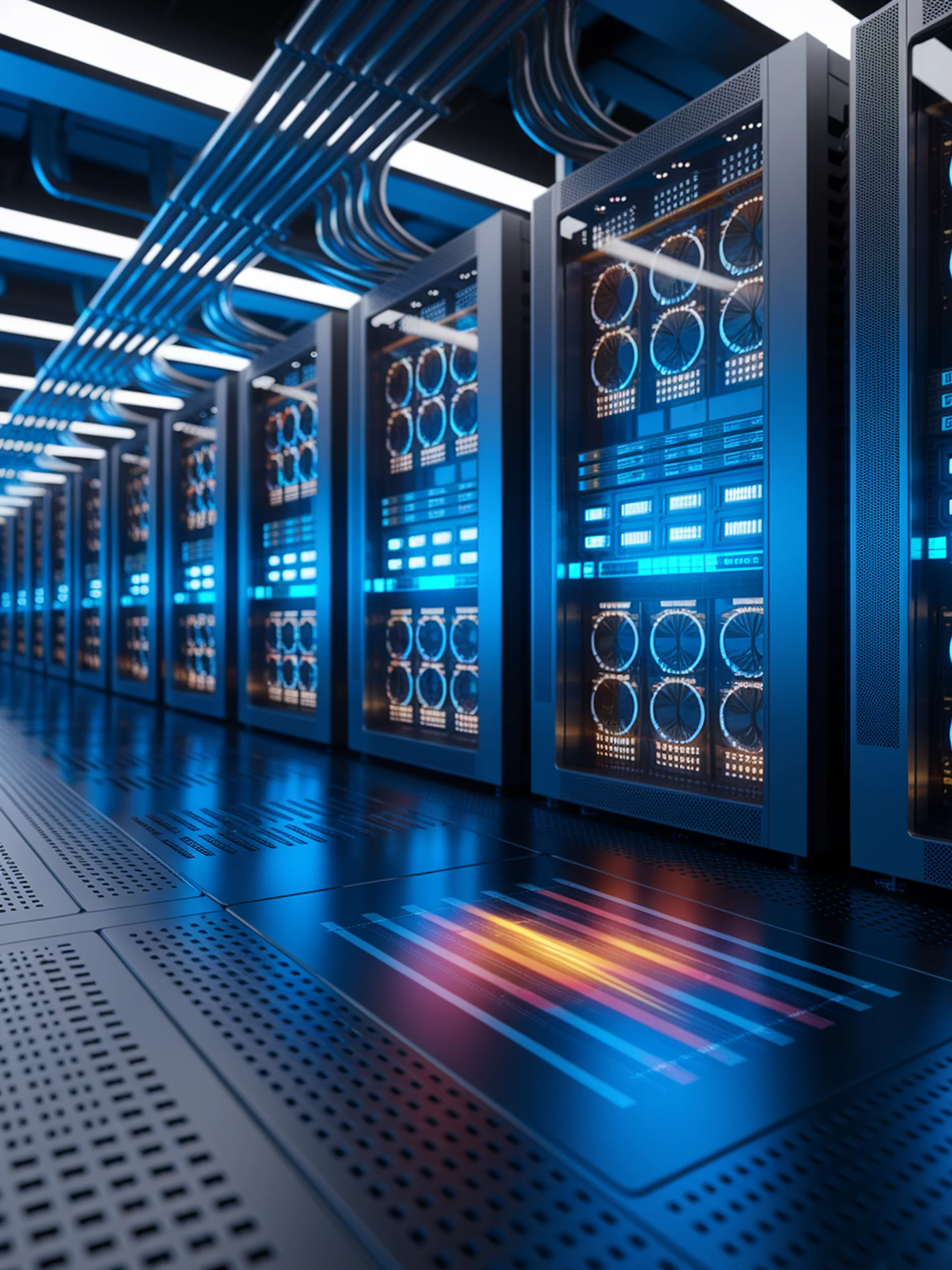
Optimizing Memory for LLM Training
Chronos-aware Pipeline Parallelism for Efficient Resource Utilization
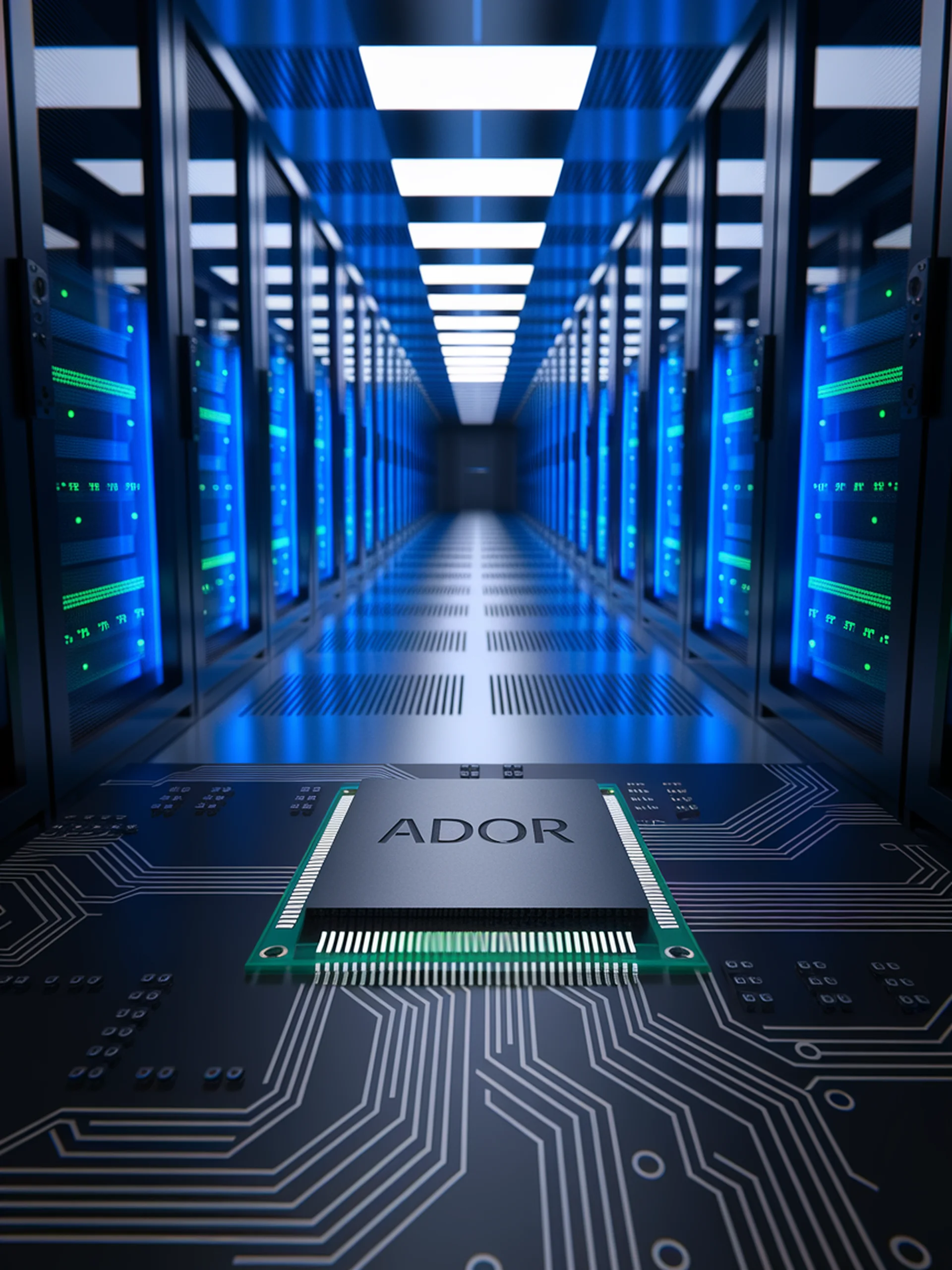
Optimizing LLM Deployment: The ADOR Framework
A novel hardware design approach for faster, more efficient LLM serving
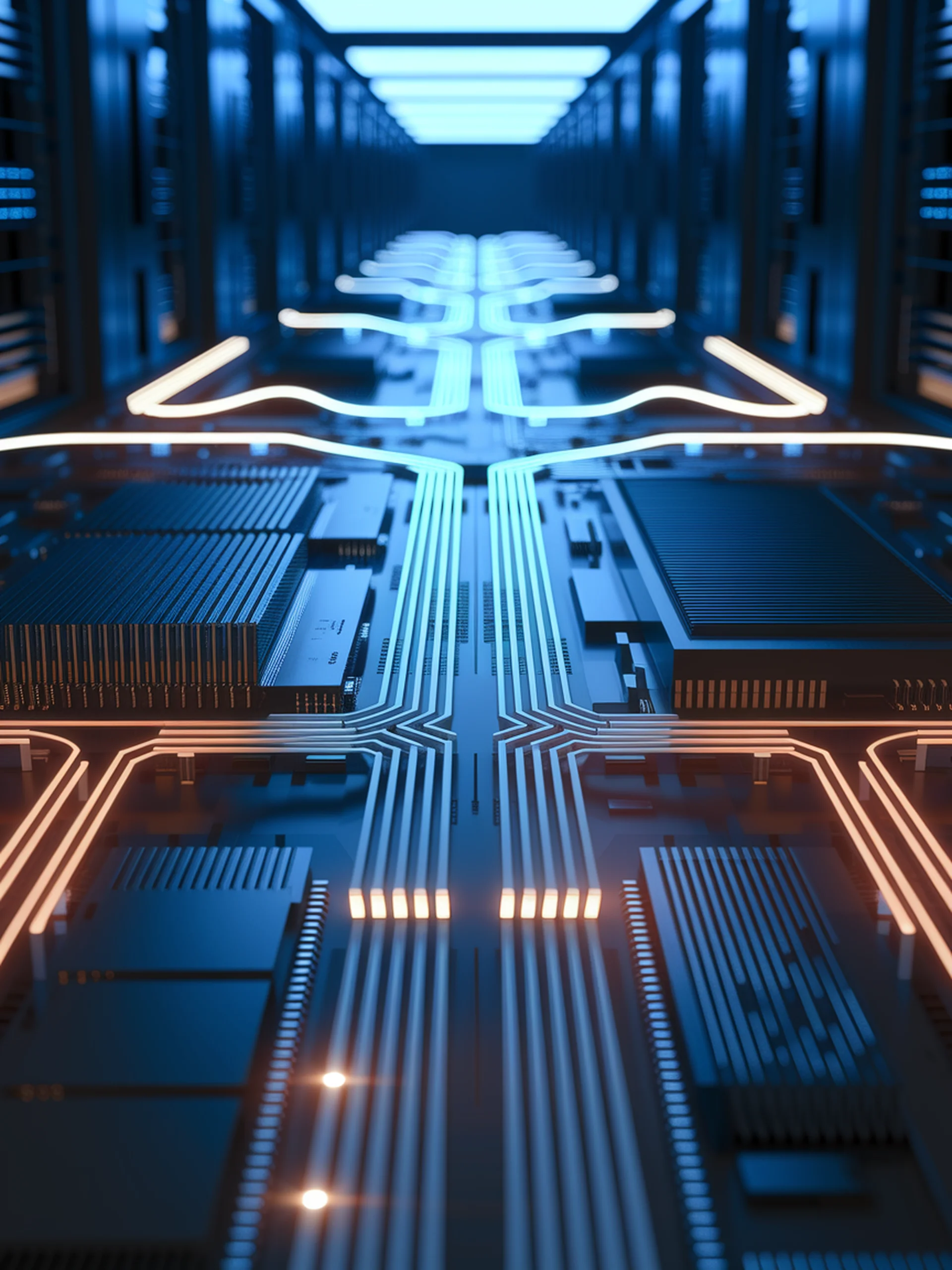
Accelerating LLM Inference with Smart MoE Parallelism
Boosting MoE efficiency through speculative token processing
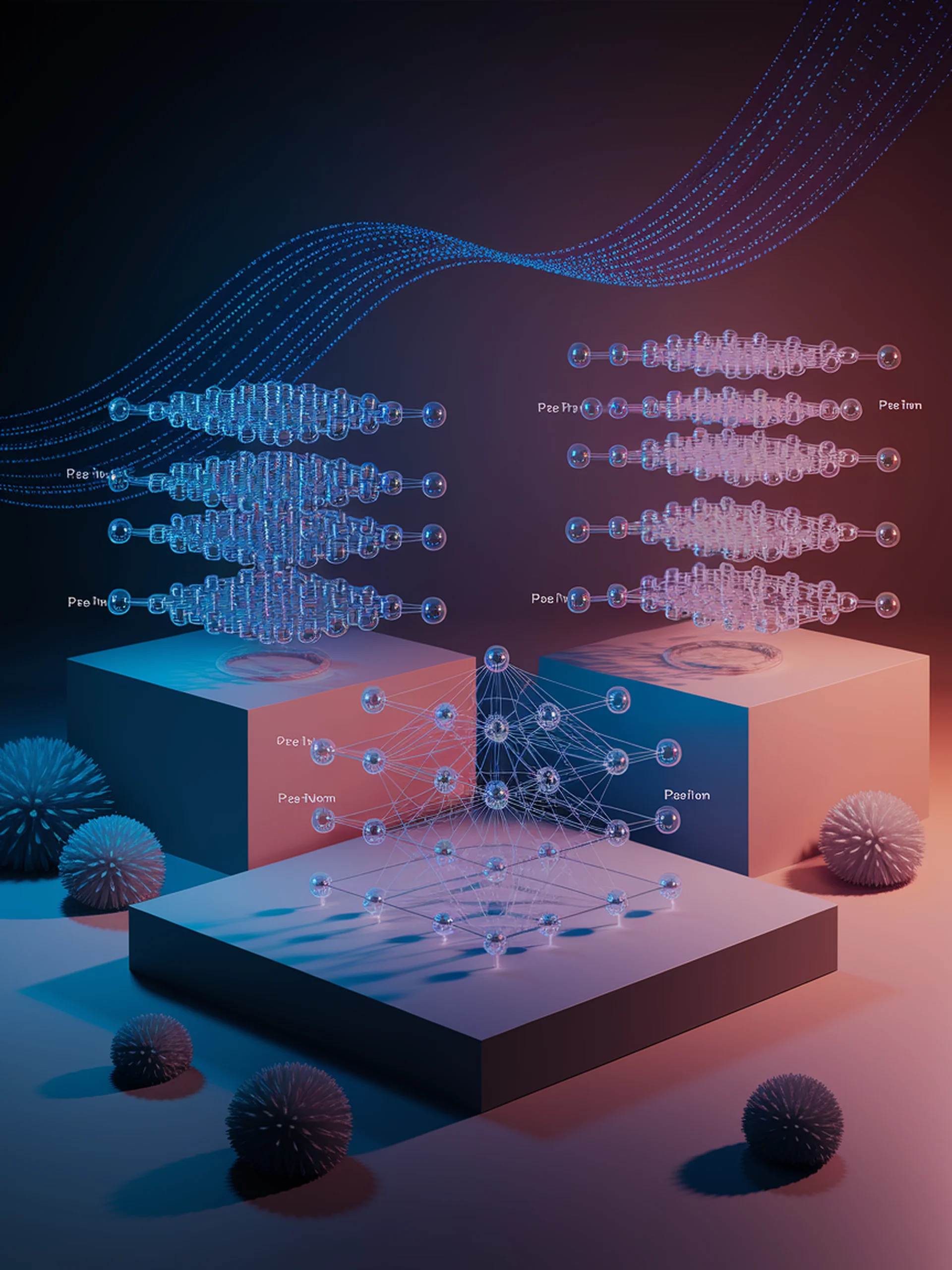
HybridNorm: Stabilizing Transformer Training
A novel normalization approach that combines the best of Pre-Norm and Post-Norm architectures
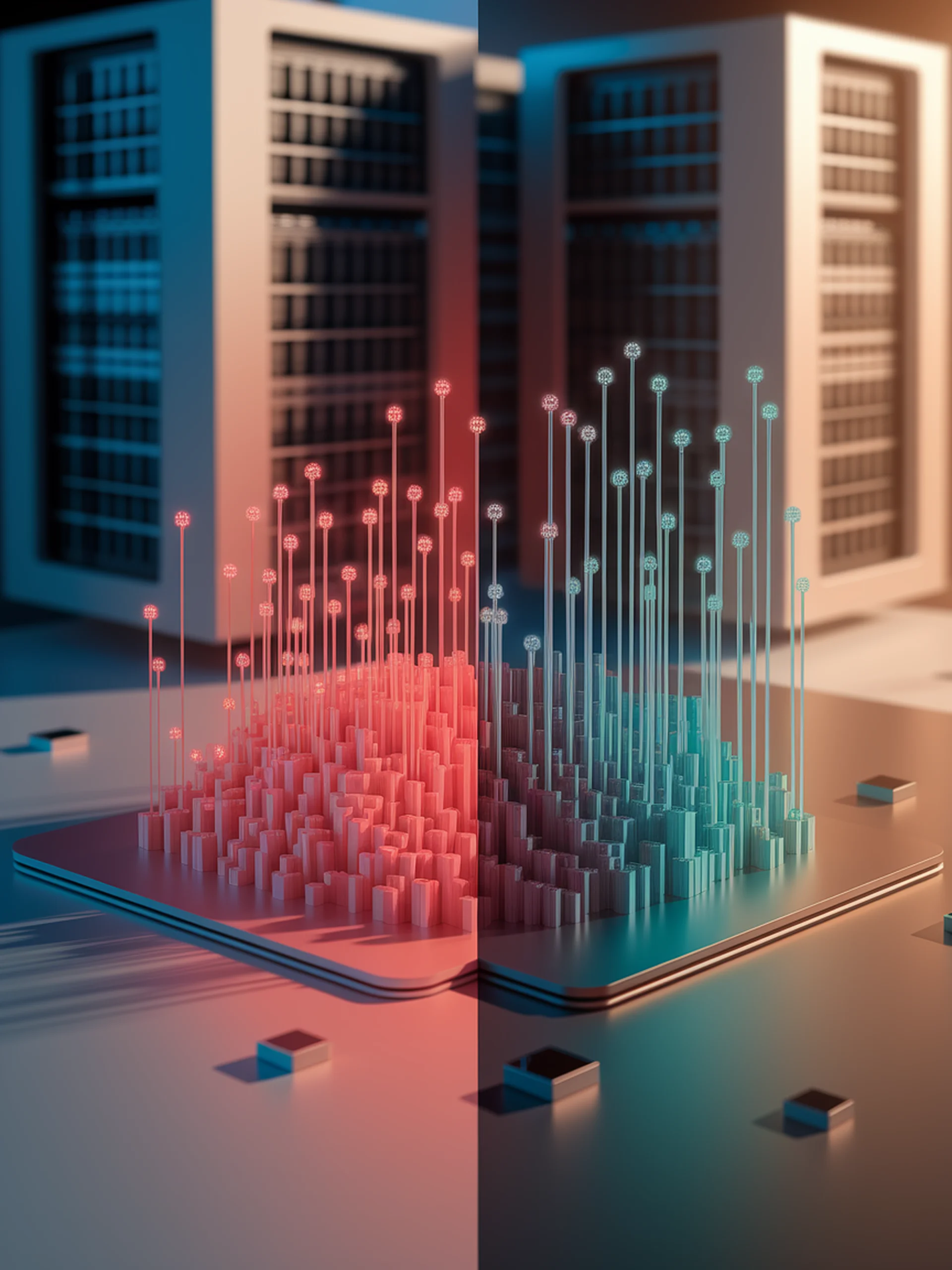
Smarter LLM Compression
Using entropy to selectively quantize models across architectures
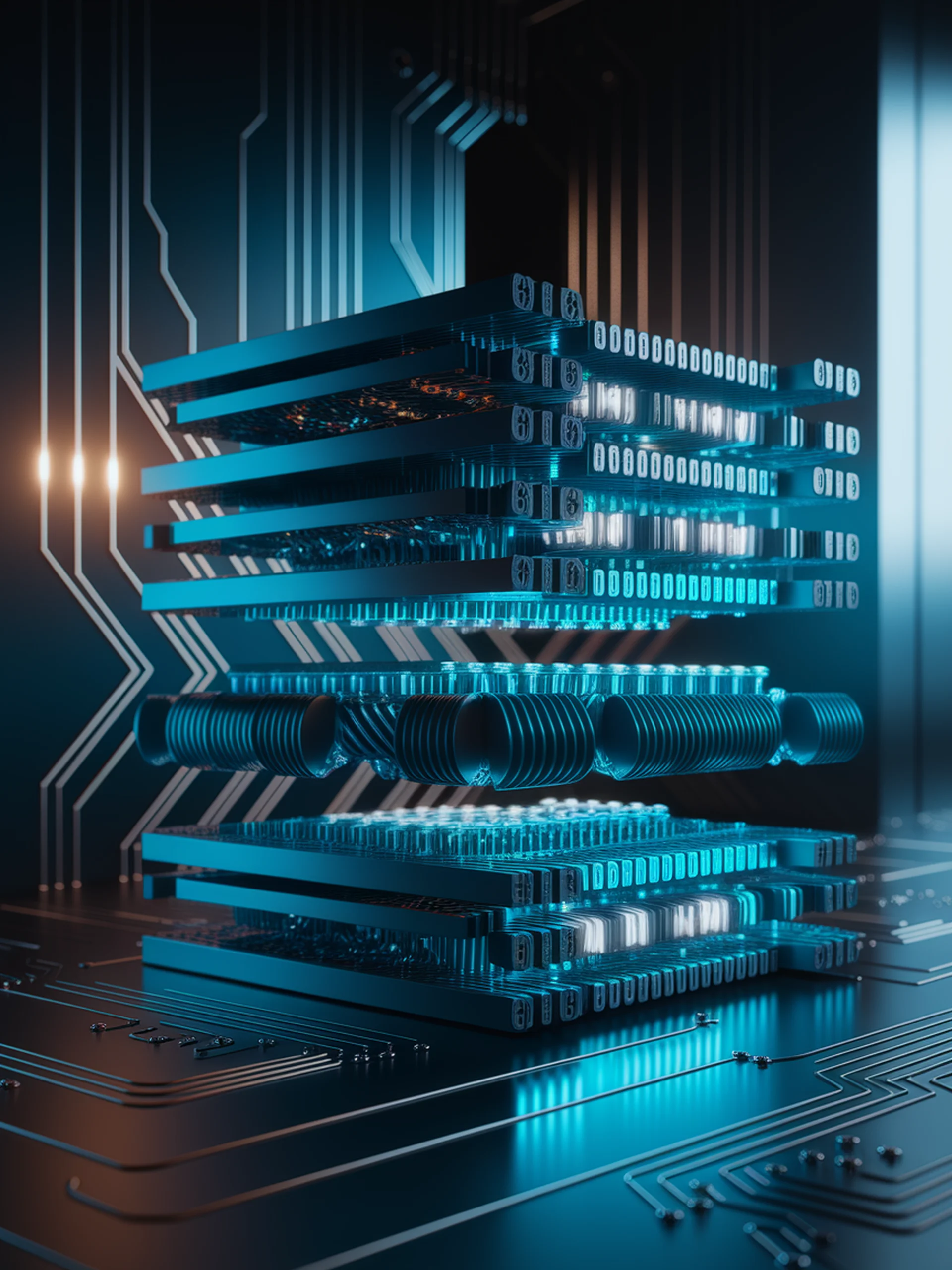
TransformerX: Reimagining LLM Architecture
Enhancing LLM efficiency with multi-scale convolution and adaptive mechanisms
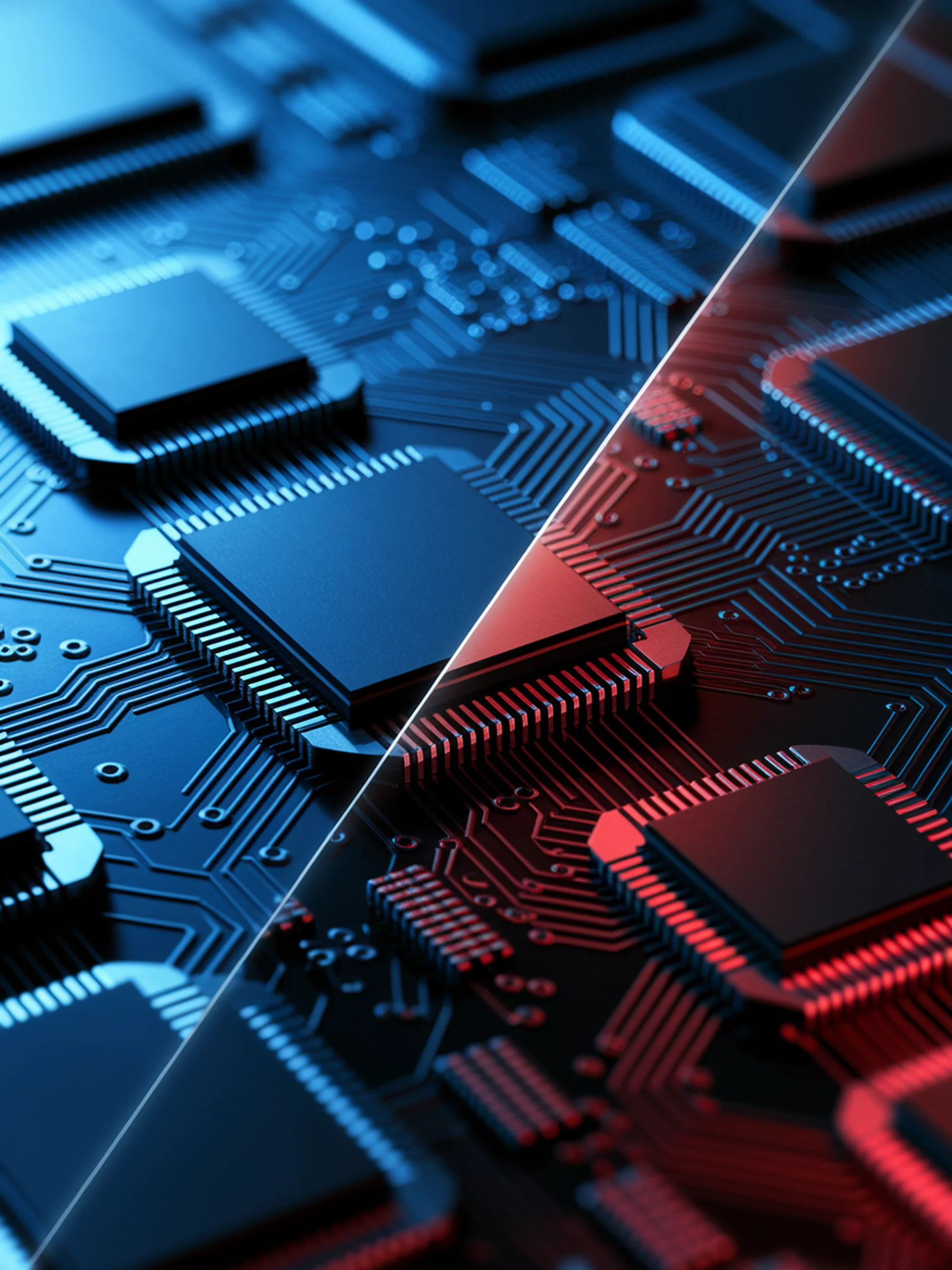
Security Implications of LVLM Compression
How model compression affects vision-language models' trustworthiness
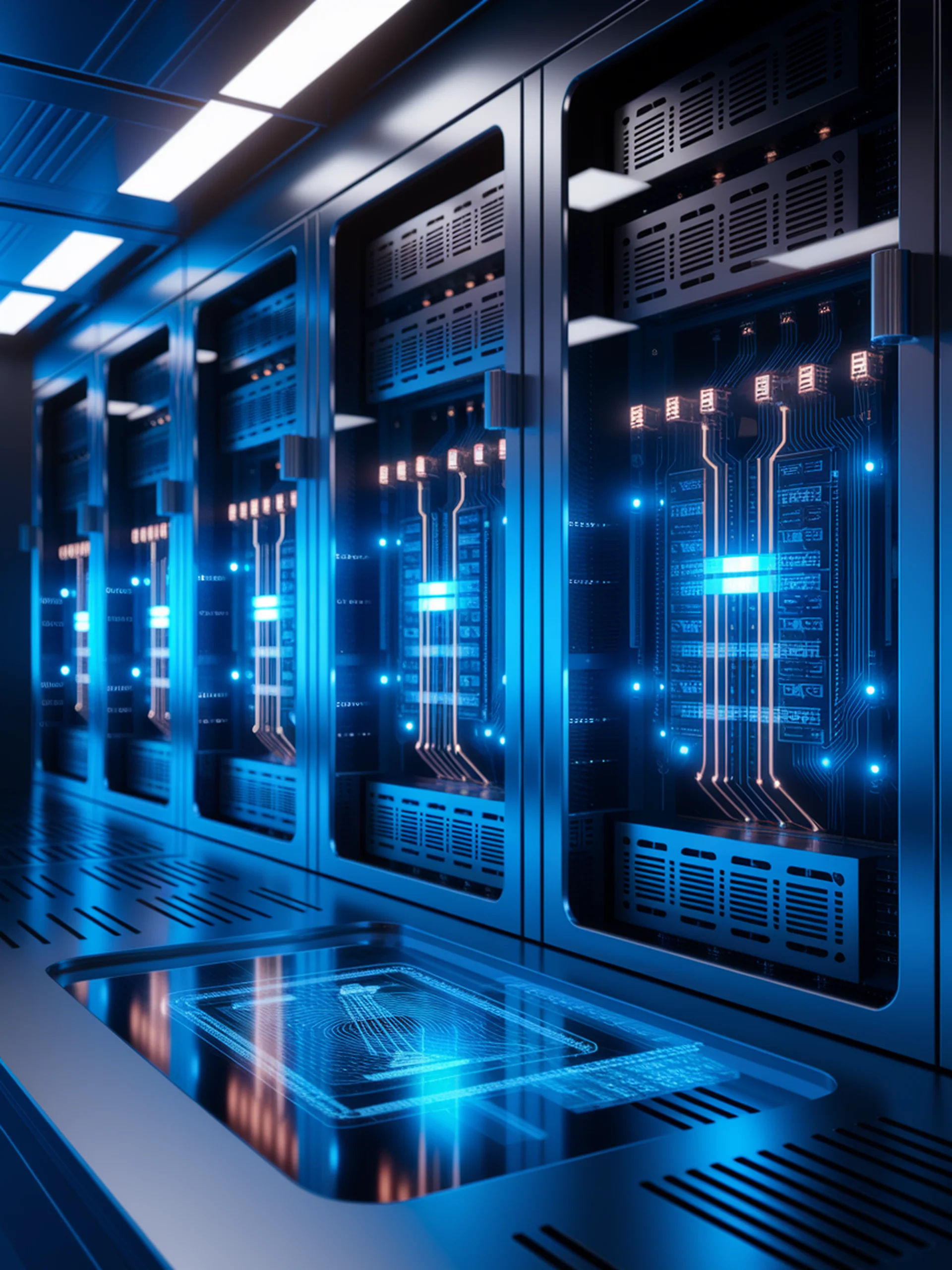
Smarter LLM Pruning with Wanda++
Accelerating inference speed while preserving performance
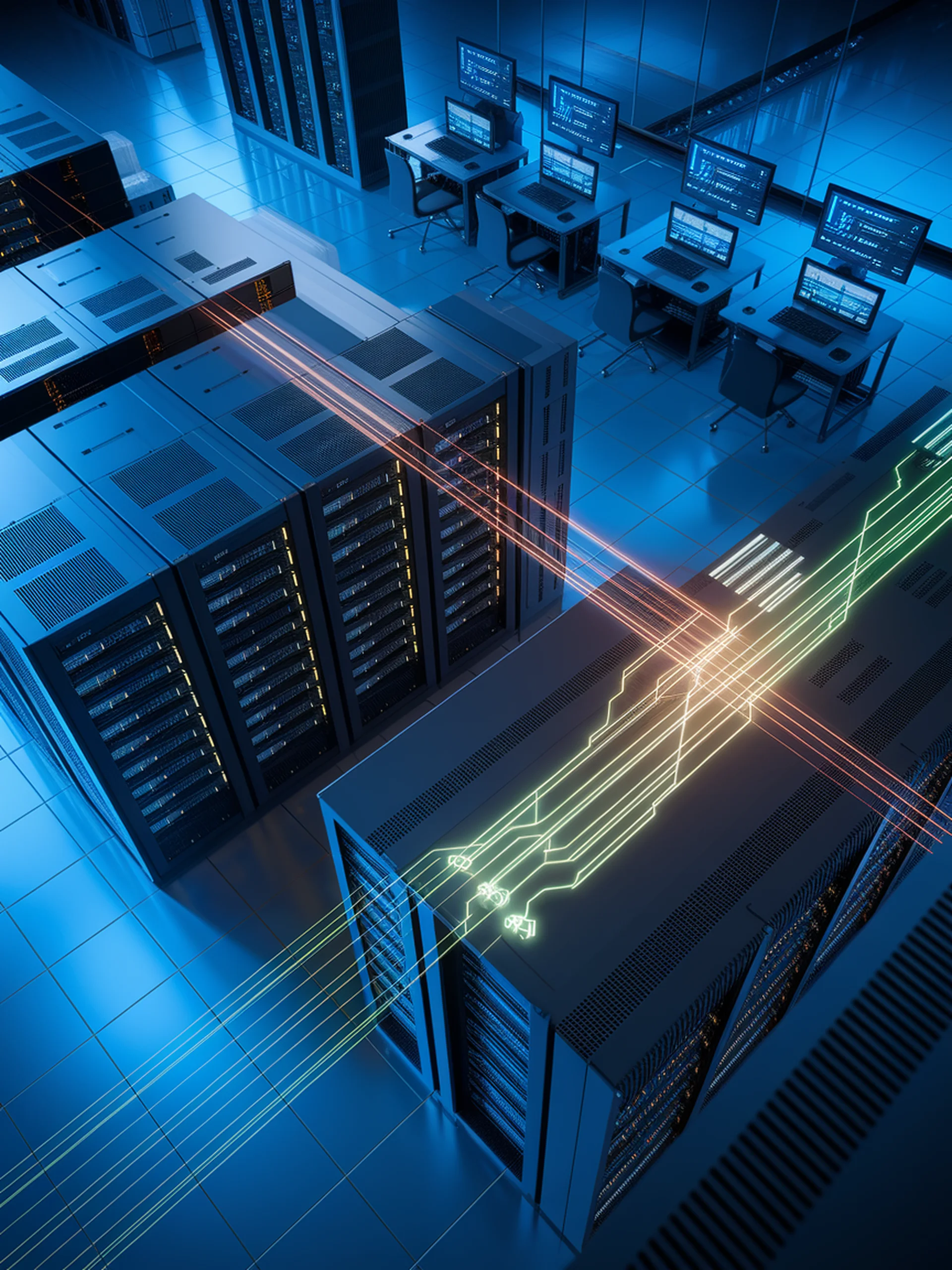
Balcony: Efficient LLM Deployment Made Simple
A lightweight approach for dynamic inference that balances performance and efficiency
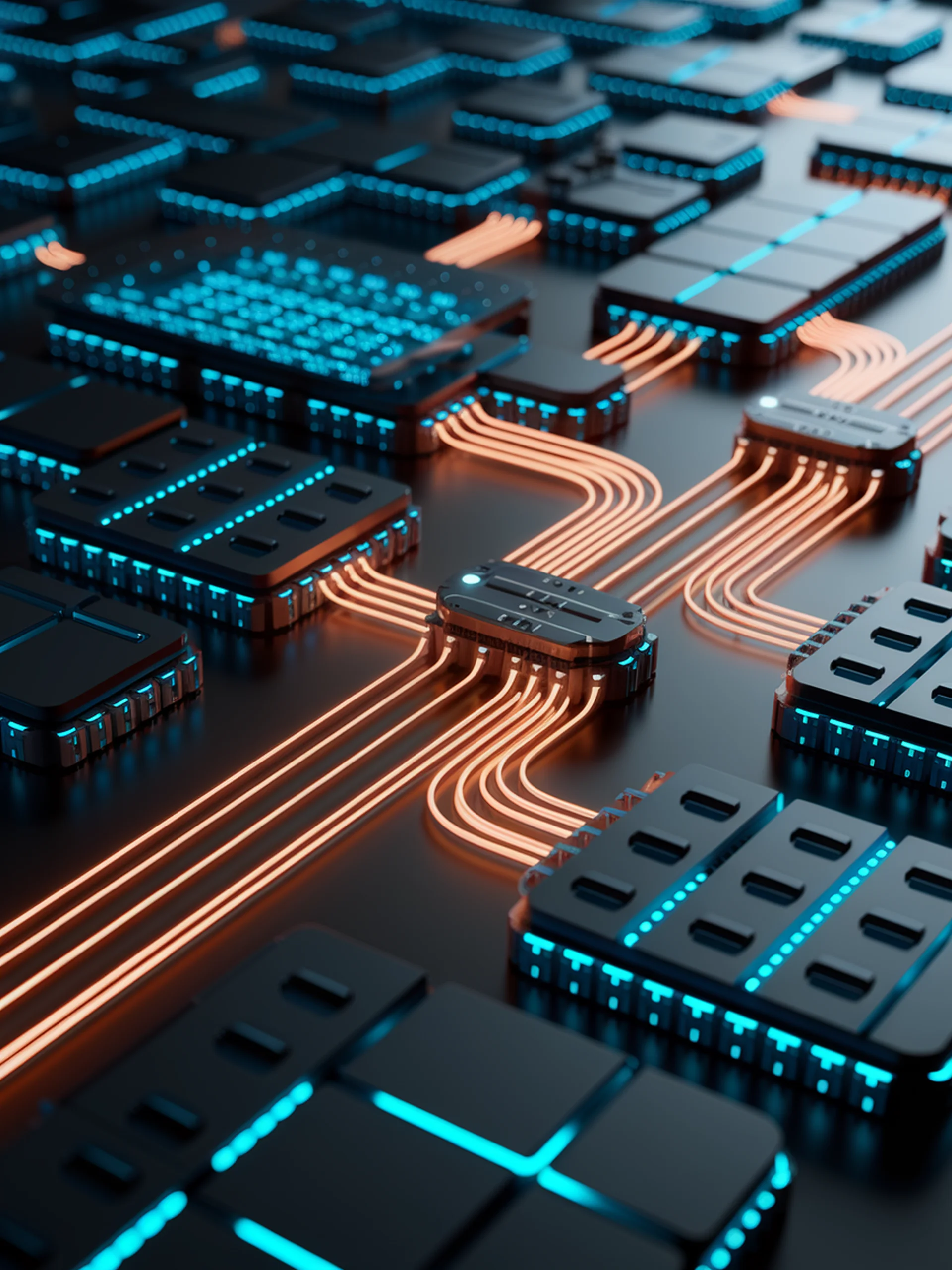
Solving the MoE Bottleneck
Intelligent token routing for faster LLM inference
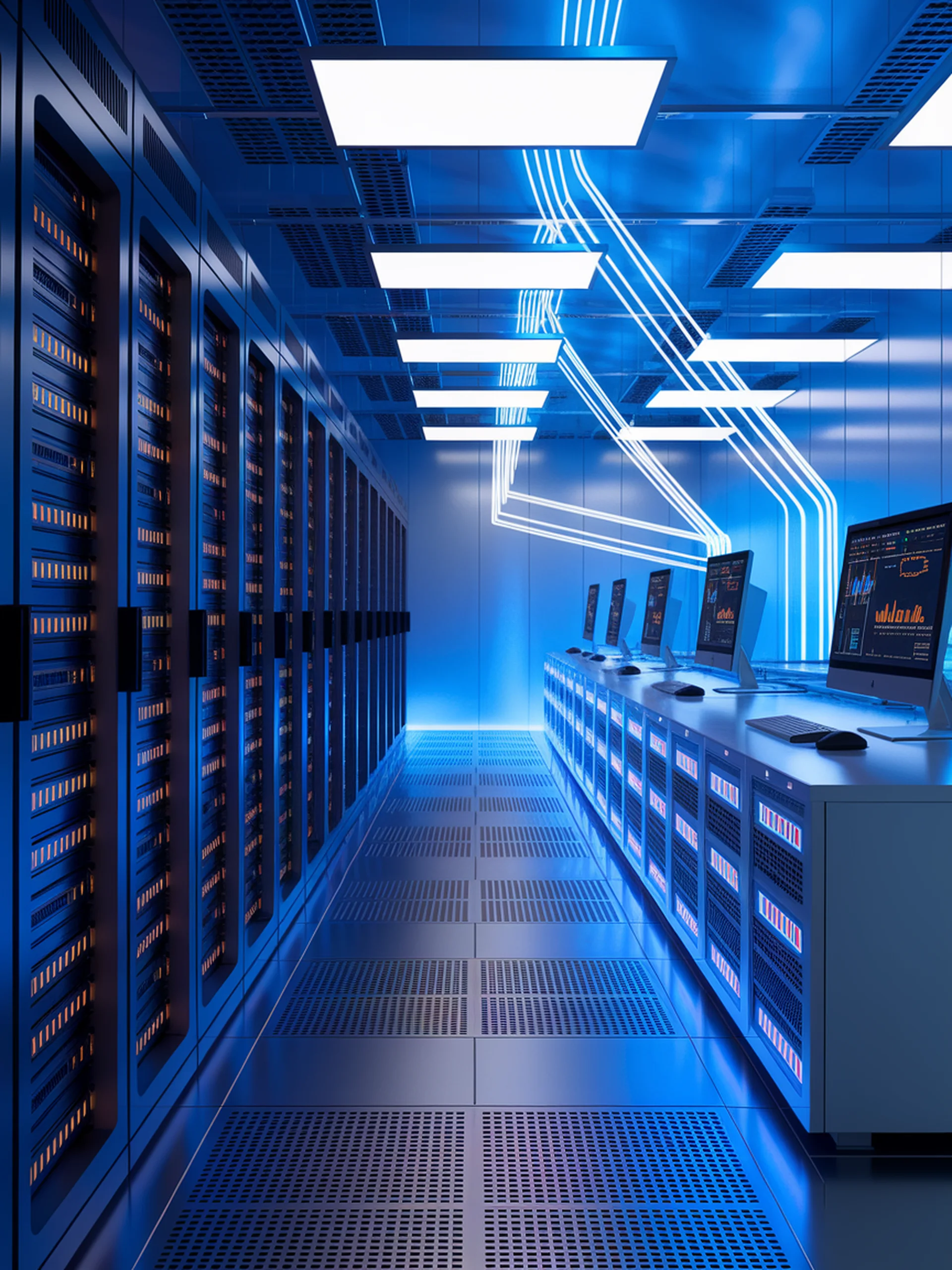
Accelerating LLM Inference with Adaptive Speculation
Meeting SLOs through intelligent workload adaptation
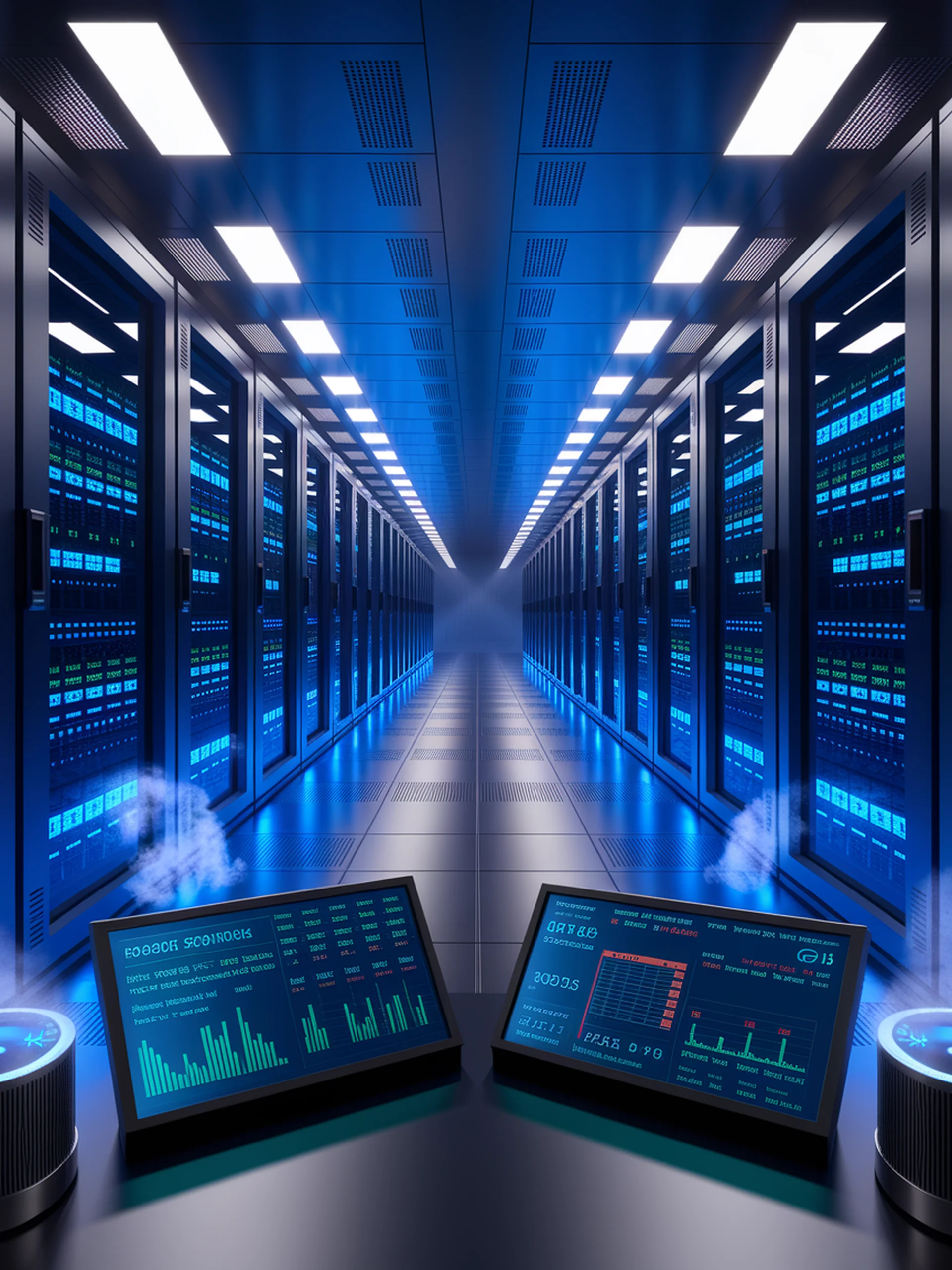
Optimizing GPU Usage for Serverless AI
A Dynamic Resource Allocation System for Large Language Models
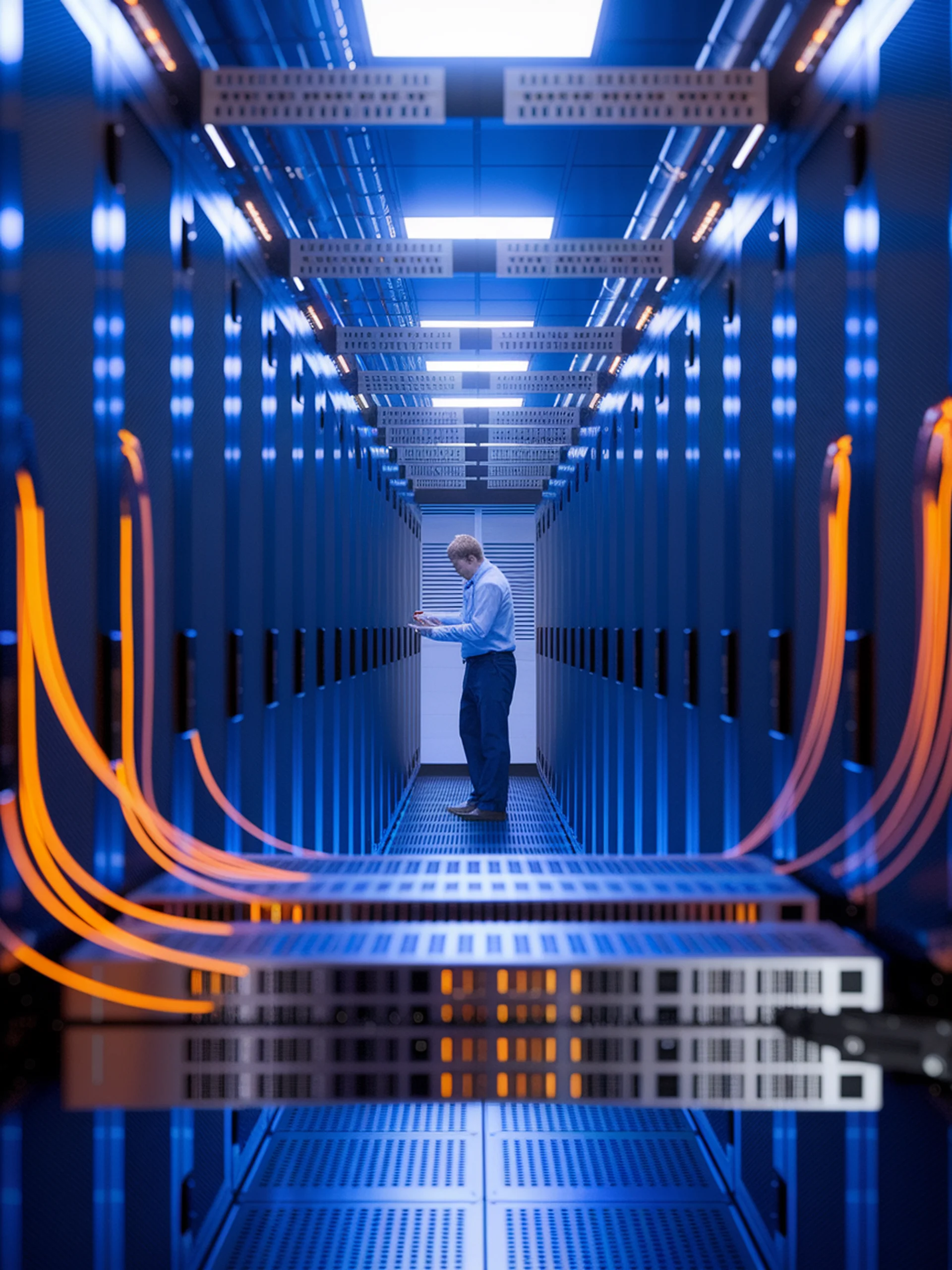
Making LLMs More Efficient
Scaling 300B parameter models without premium hardware
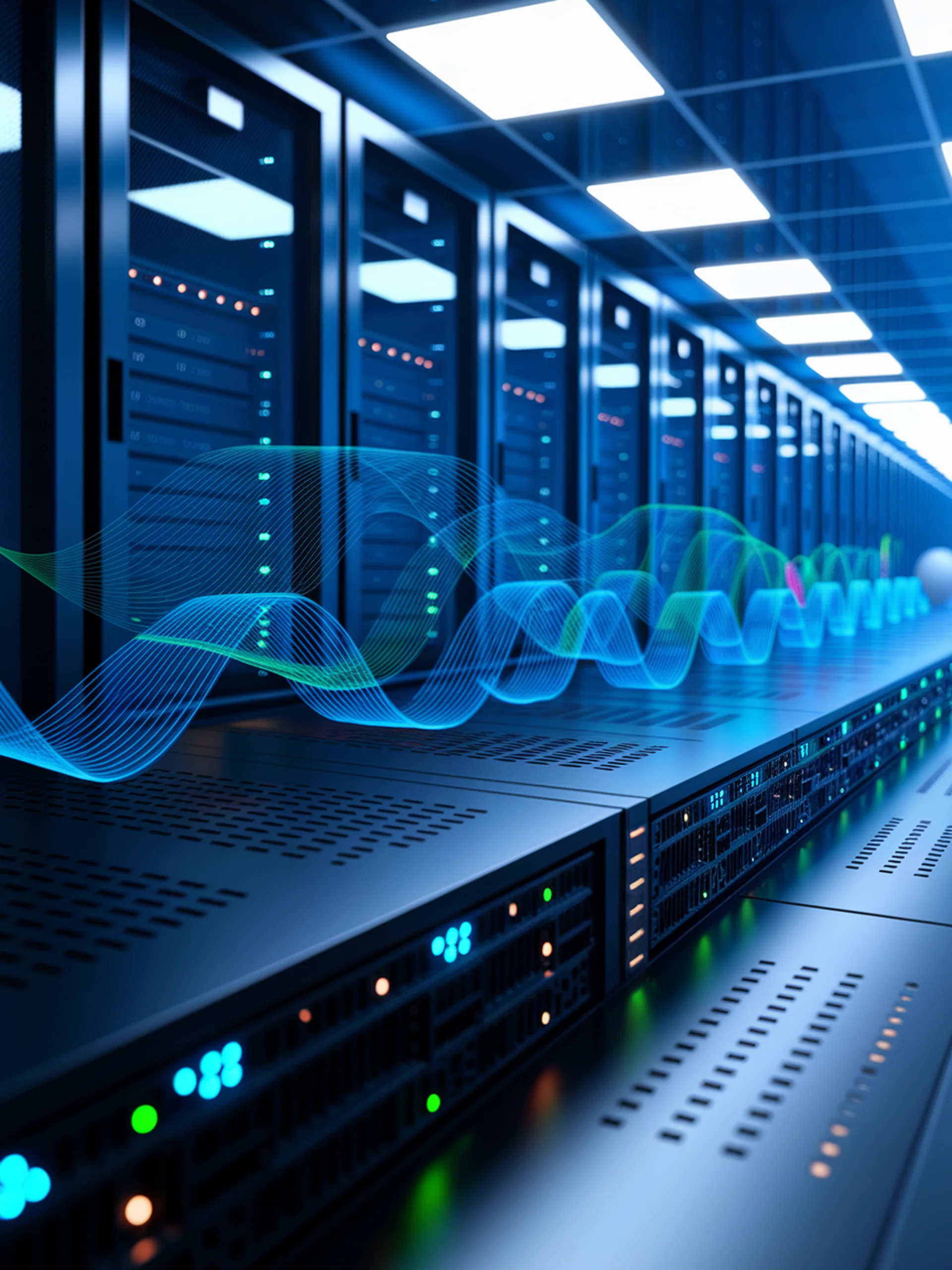
Boosting LLM Performance: Dynamic Batch Optimization
Memory-aware, SLA-constrained approach to maximize inference throughput
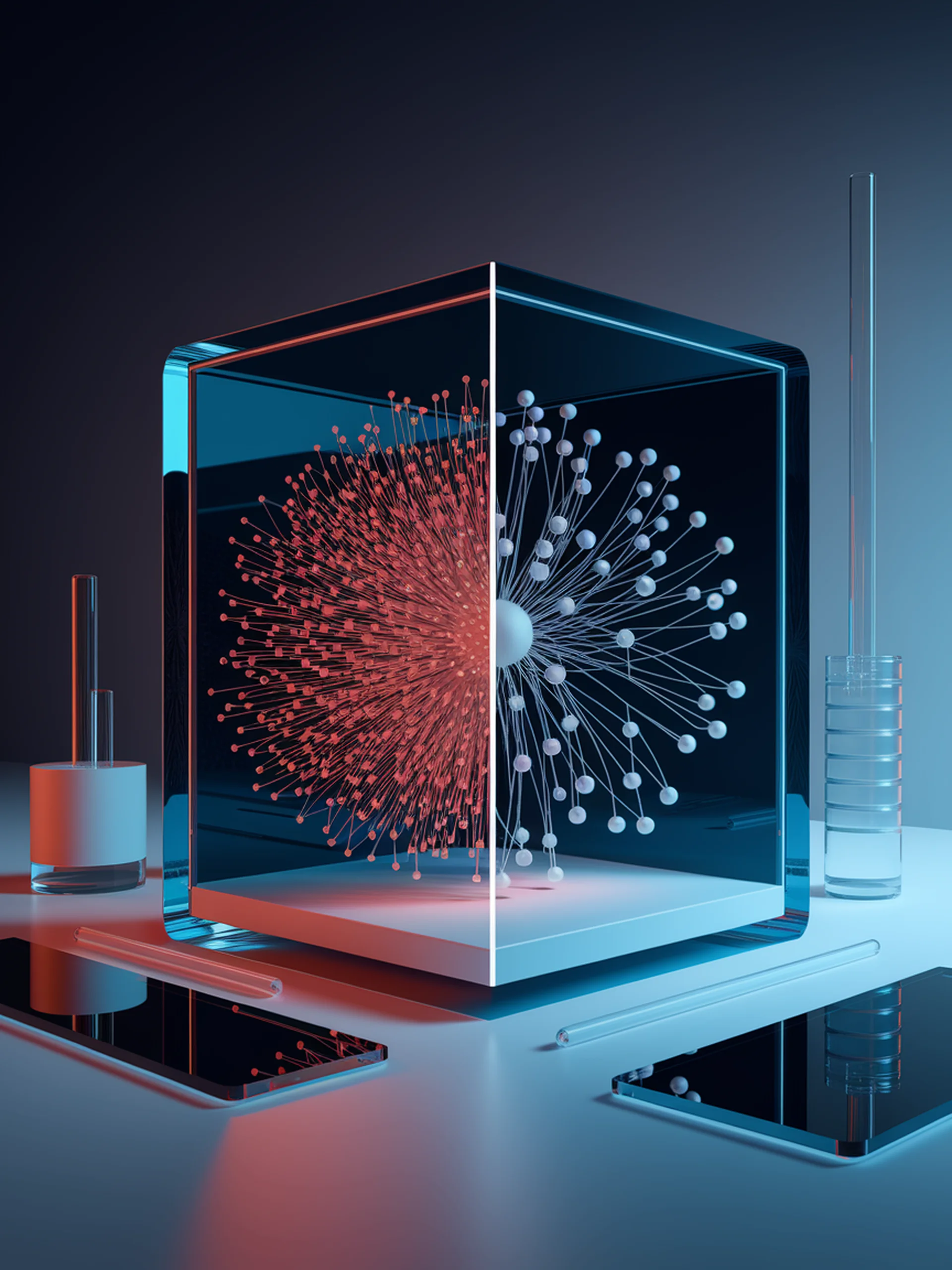
Shrinking Multimodal Models Without Losing Power
Leveraging Attention Sparsity for Extreme Model Compression
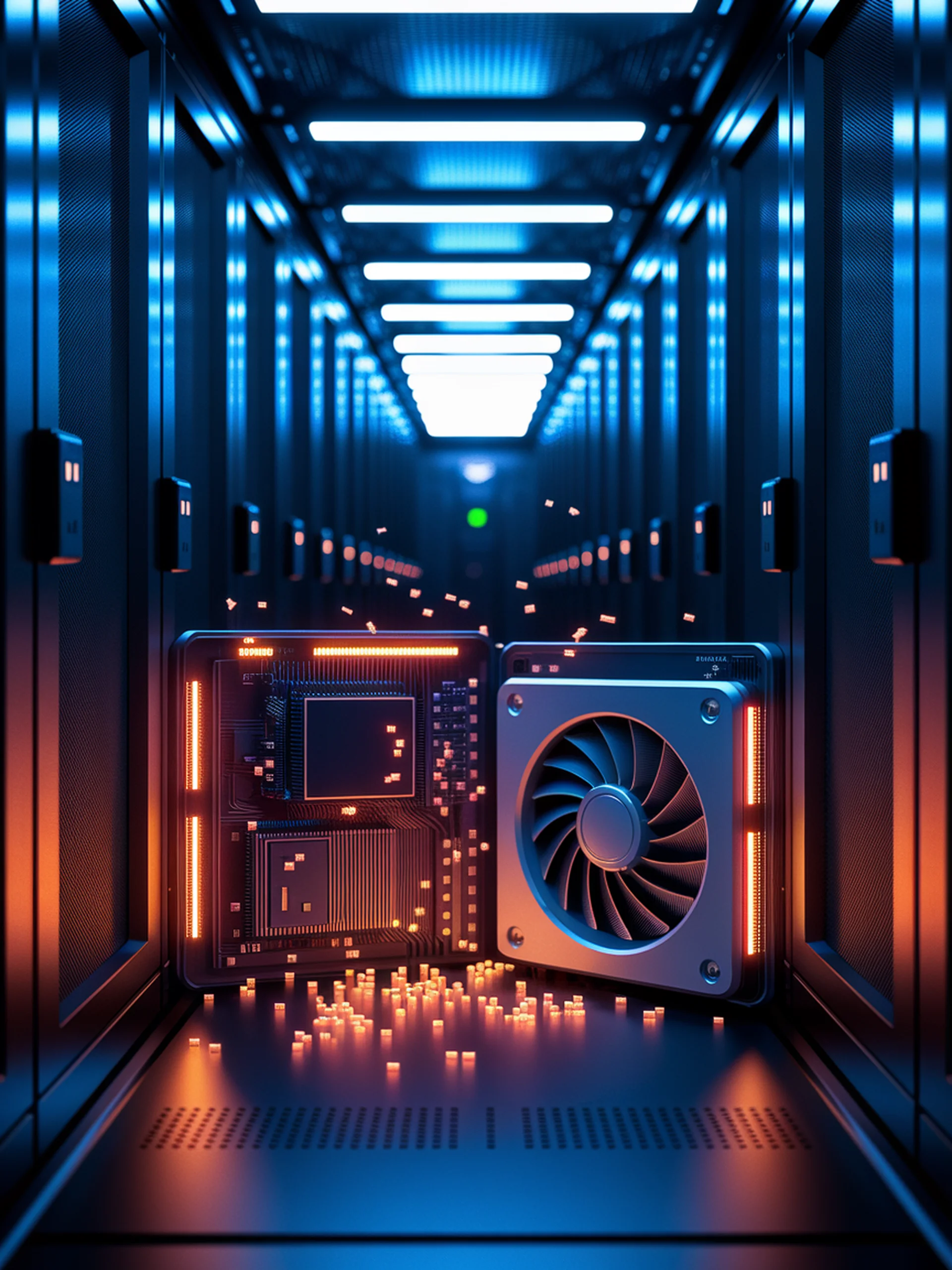
Unlocking LLM Inference Bottlenecks
How Memory Management Constraints GPU Performance in Large-Batch Processing
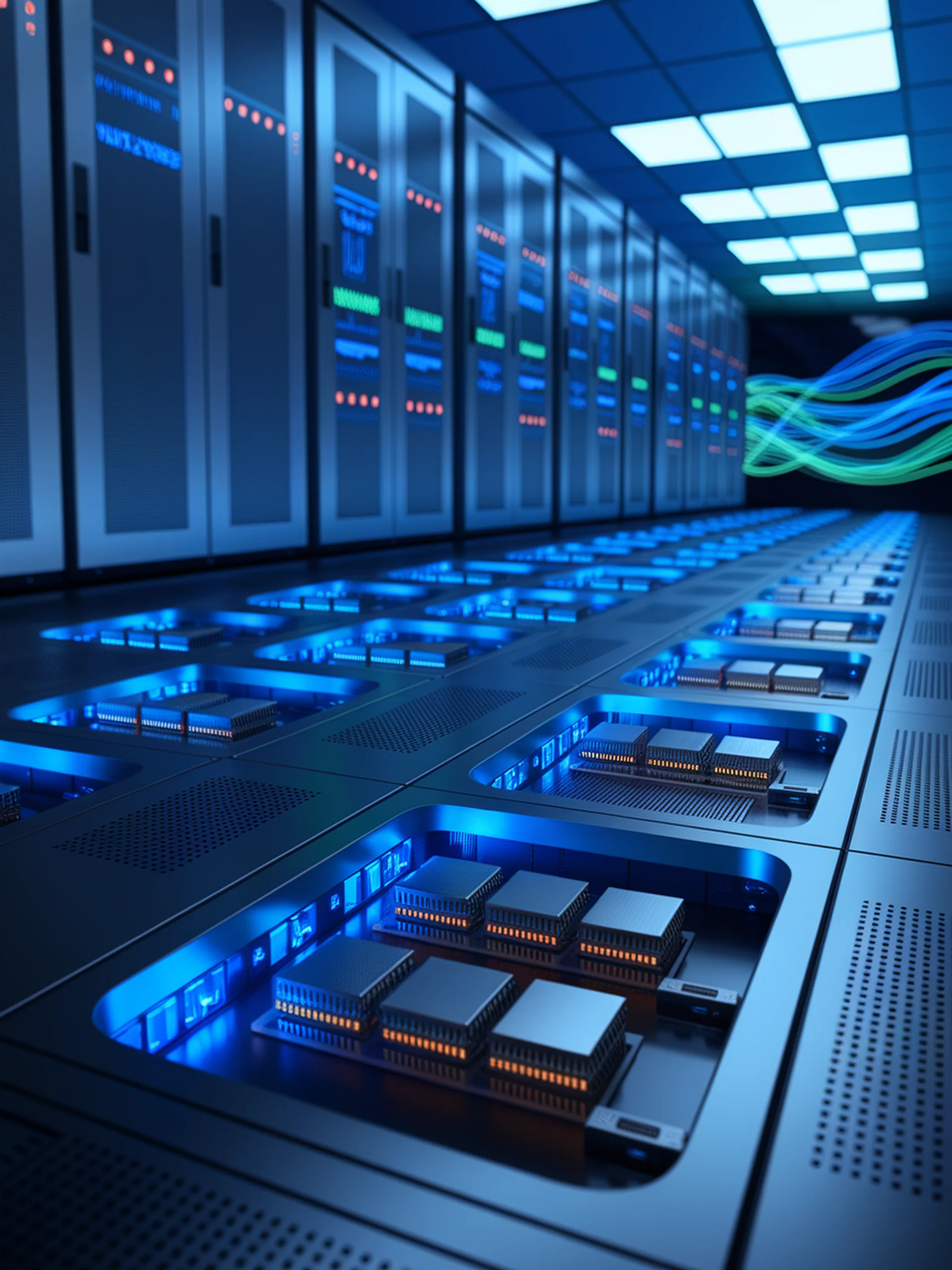
FastCache: Accelerating MLLM Performance
A lightweight framework for optimizing multi-modal LLM serving
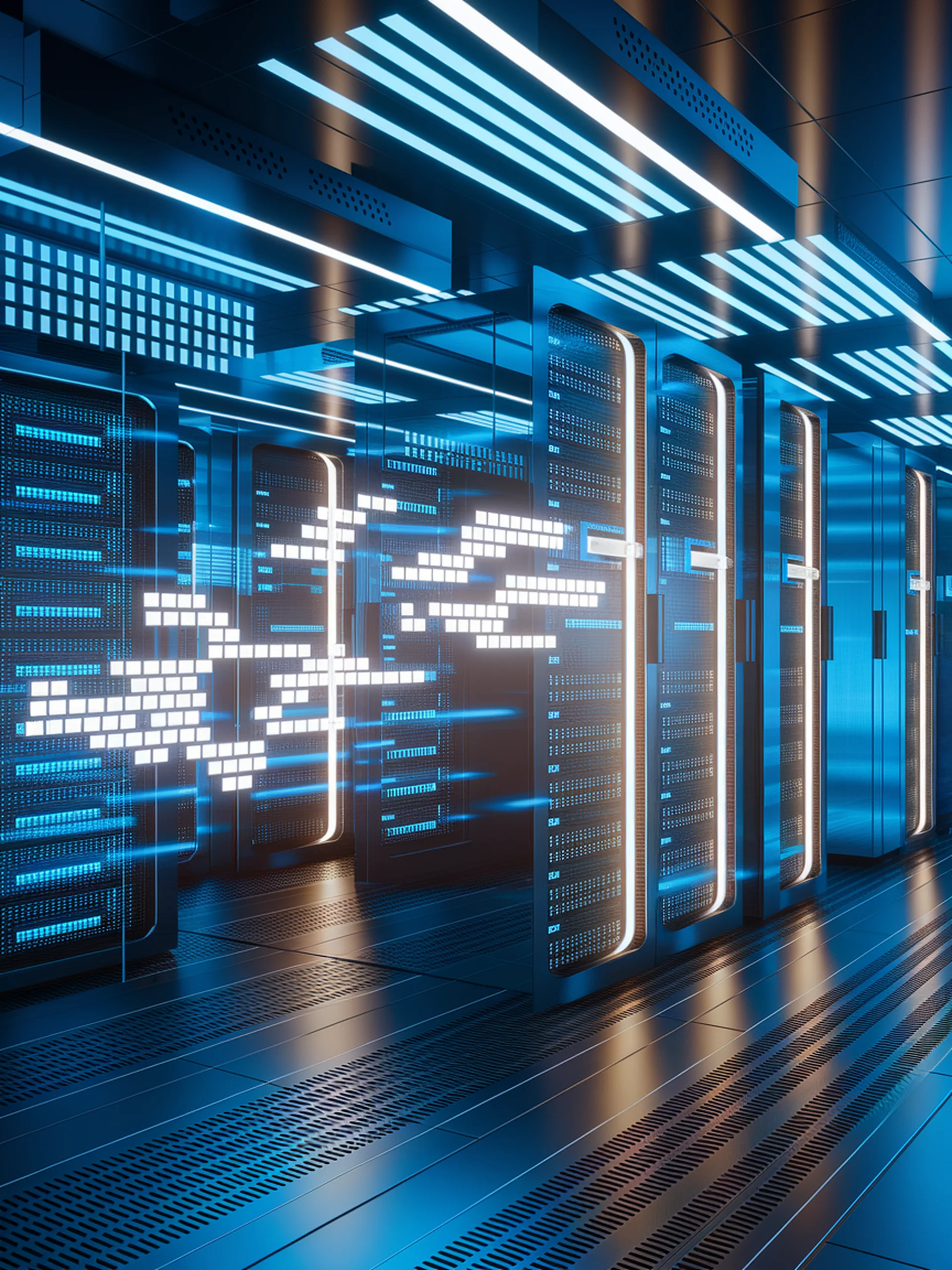
Boosting LLM Efficiency with Dynamic Depth
A computational breakthrough that saves resources without retraining
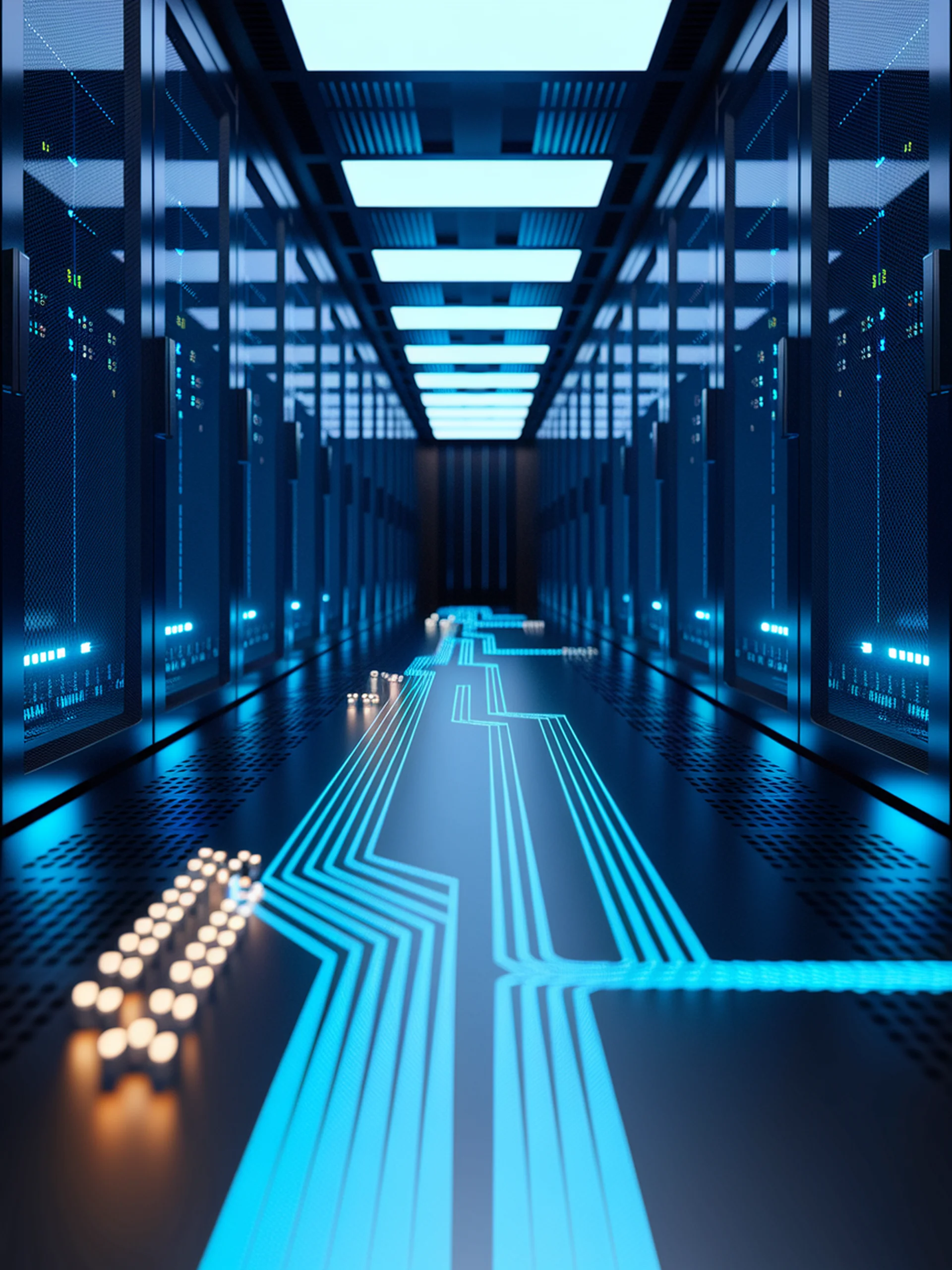
Smarter Memory Management for LLMs
How models can self-optimize their memory use for long contexts
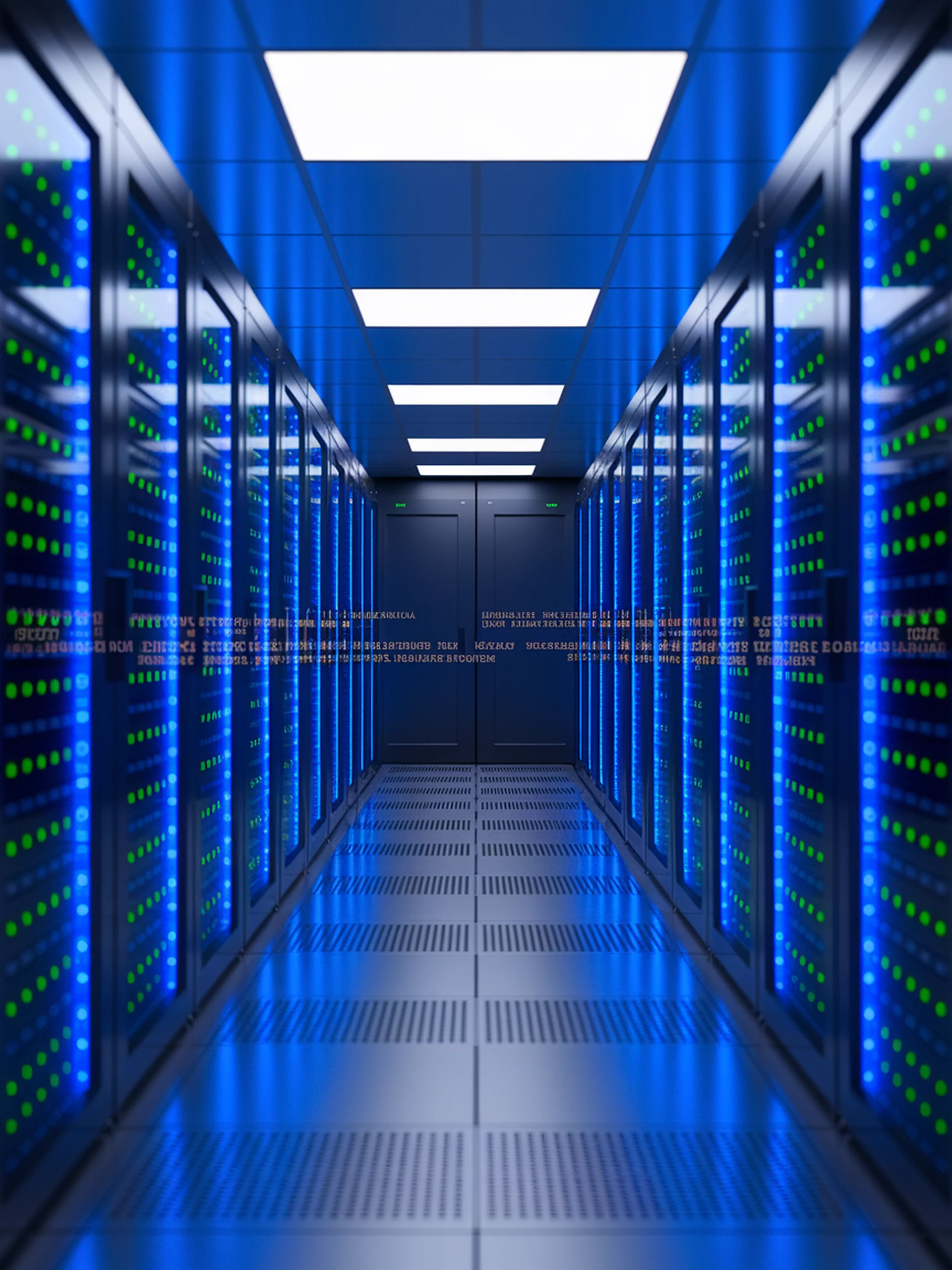
Smarter LLM Scheduling for Mixed Workloads
Preemptive prioritization for MoE models improves service quality
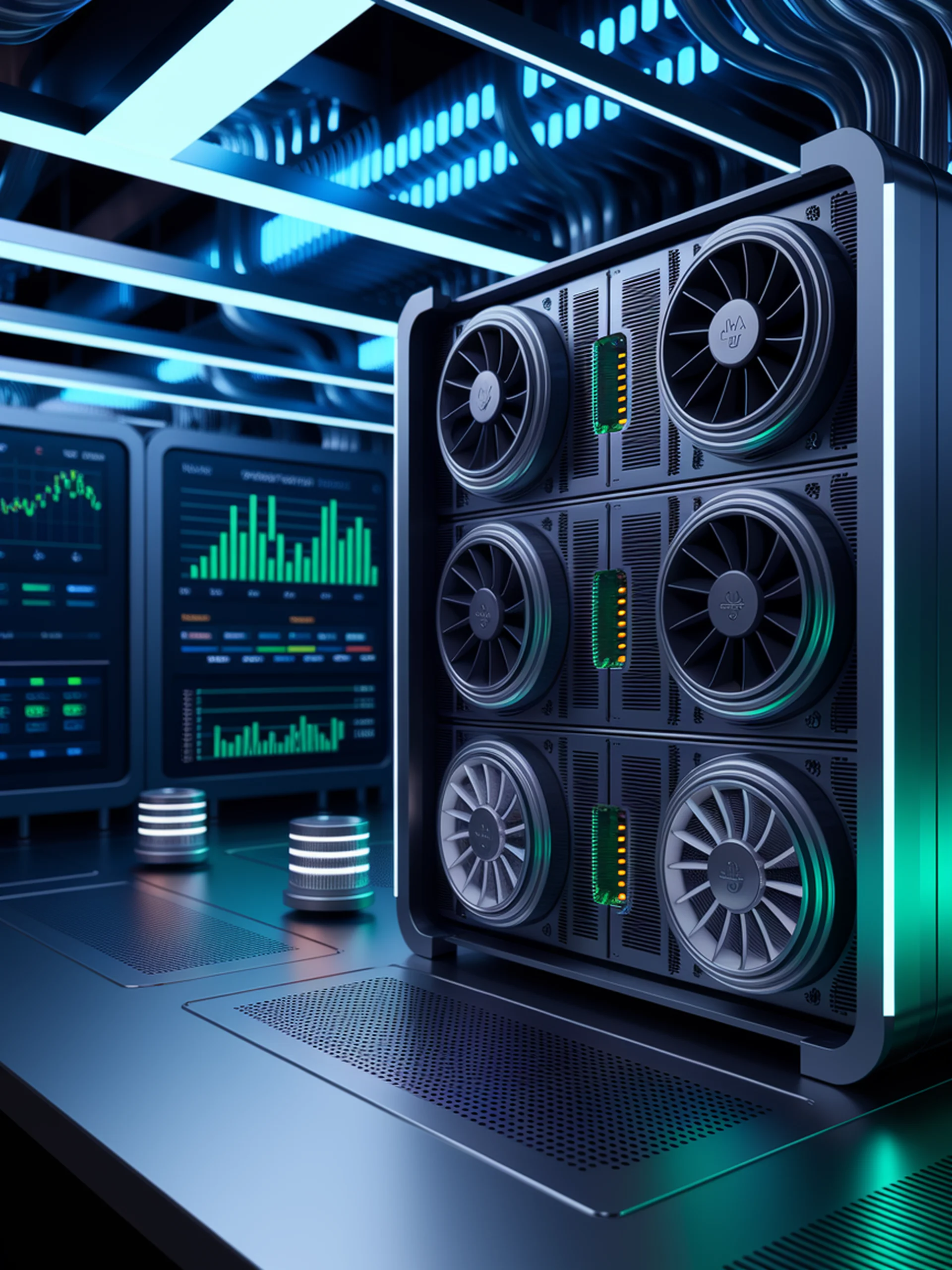
Smarter LLM Pruning
Achieving 50% Compression with Minimal Performance Loss
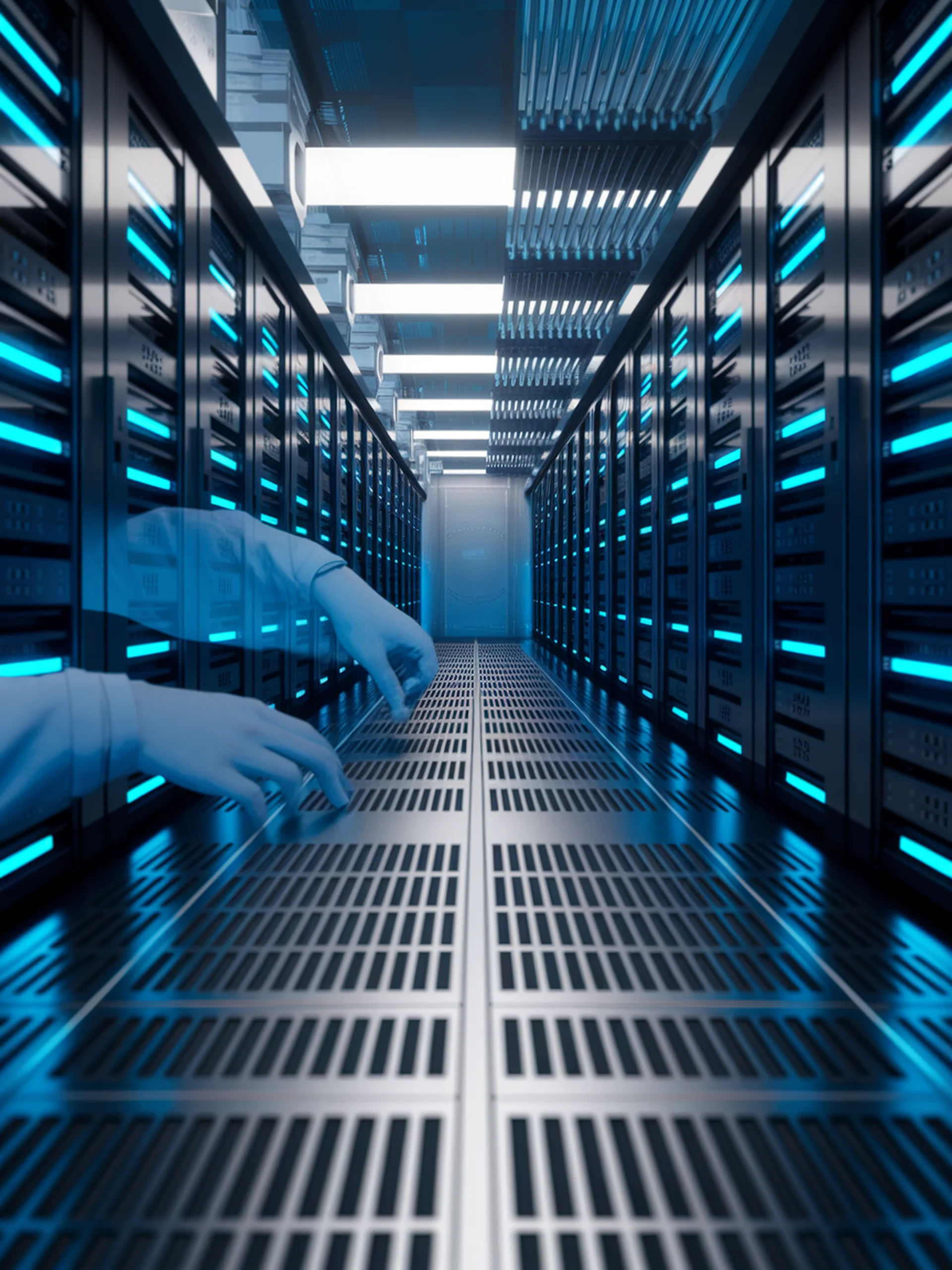
Accelerating LLMs with Smarter Token Prioritization
Gumiho: A hybrid approach to optimize speculative decoding
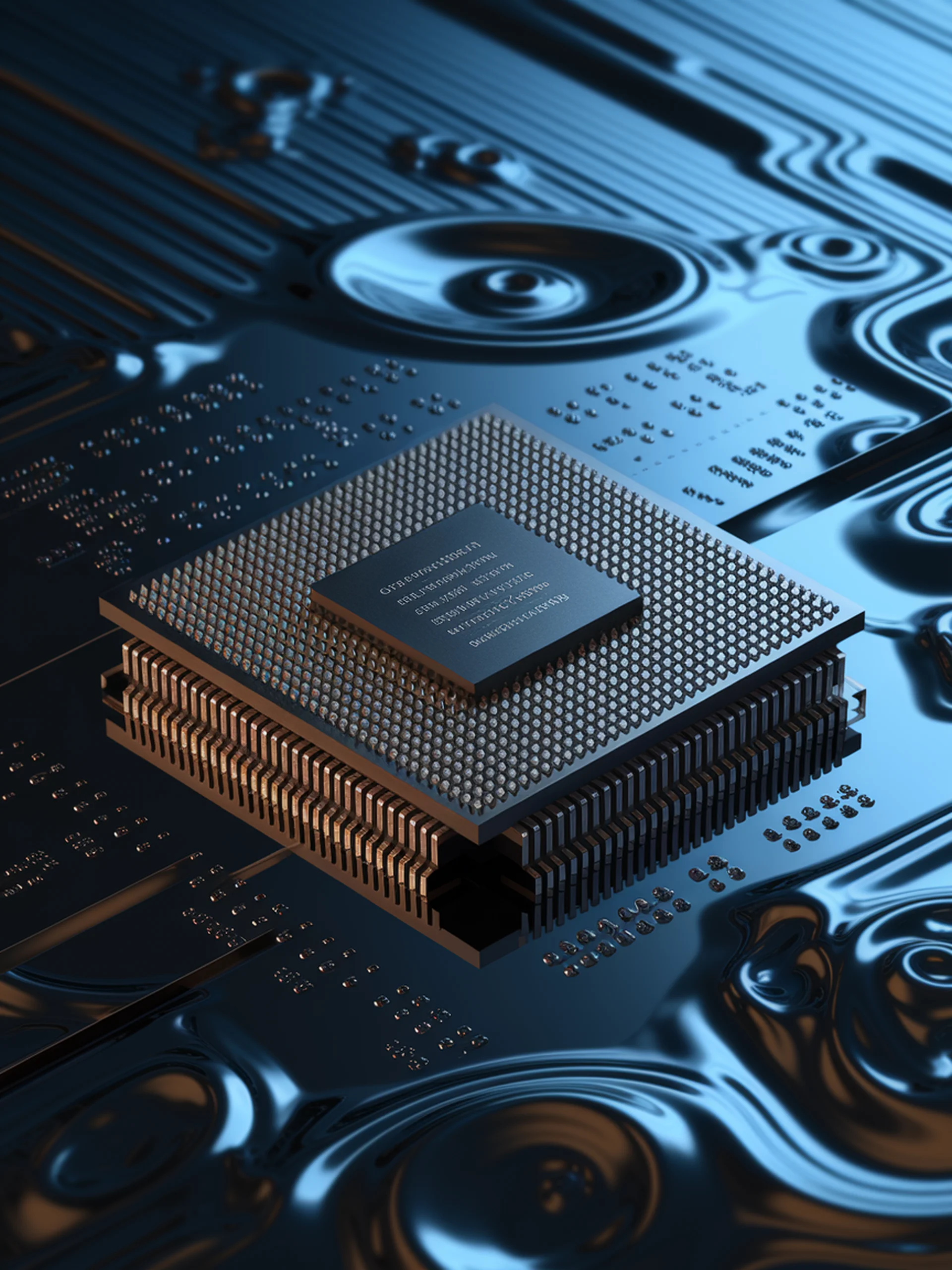
Precision Matters: Numerical Errors in LLMs
Understanding how finite-precision computations impact transformer performance
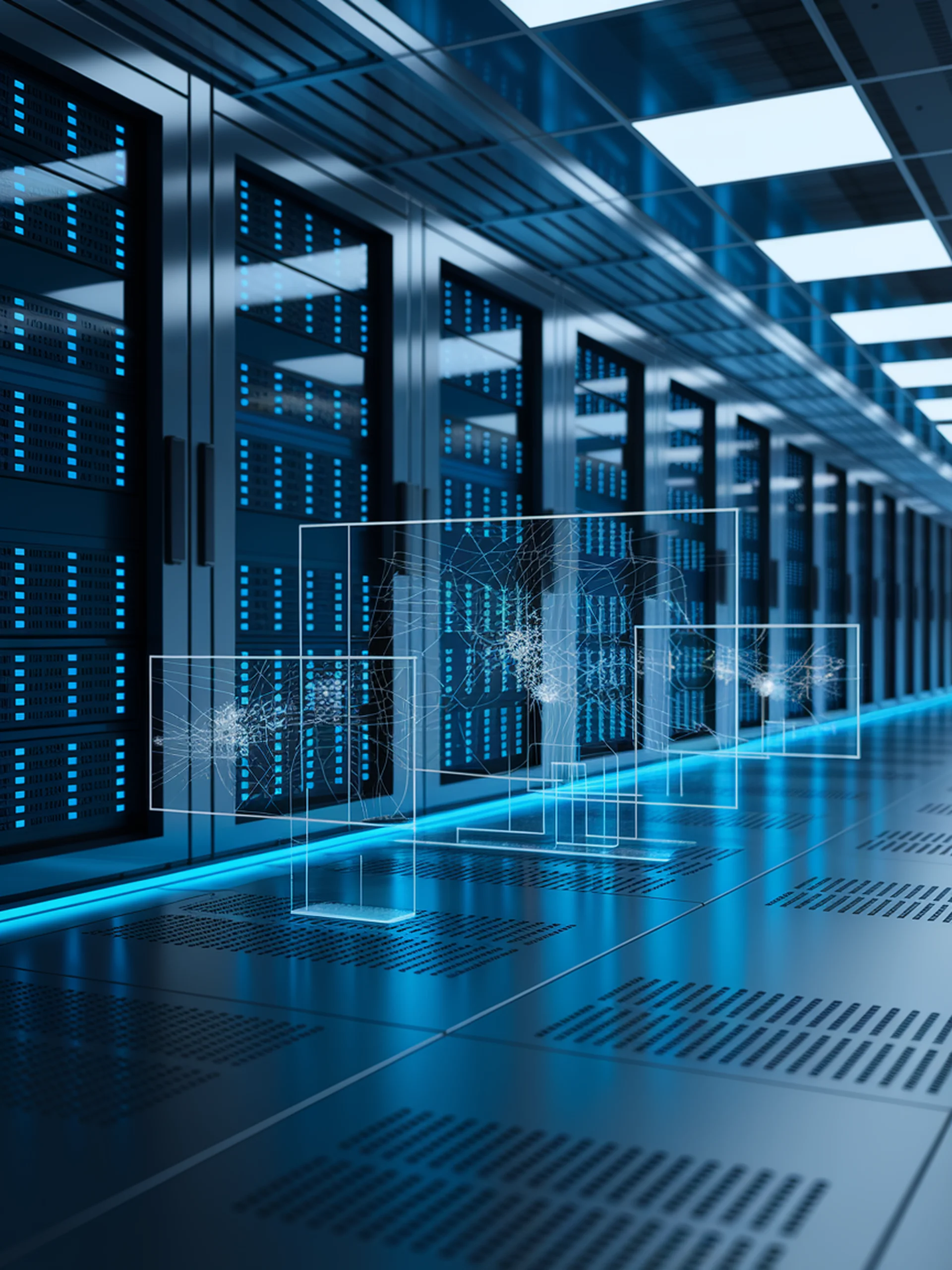
Accelerating LLM Inference with Collaborative Speculation
A novel architecture for more efficient language model serving
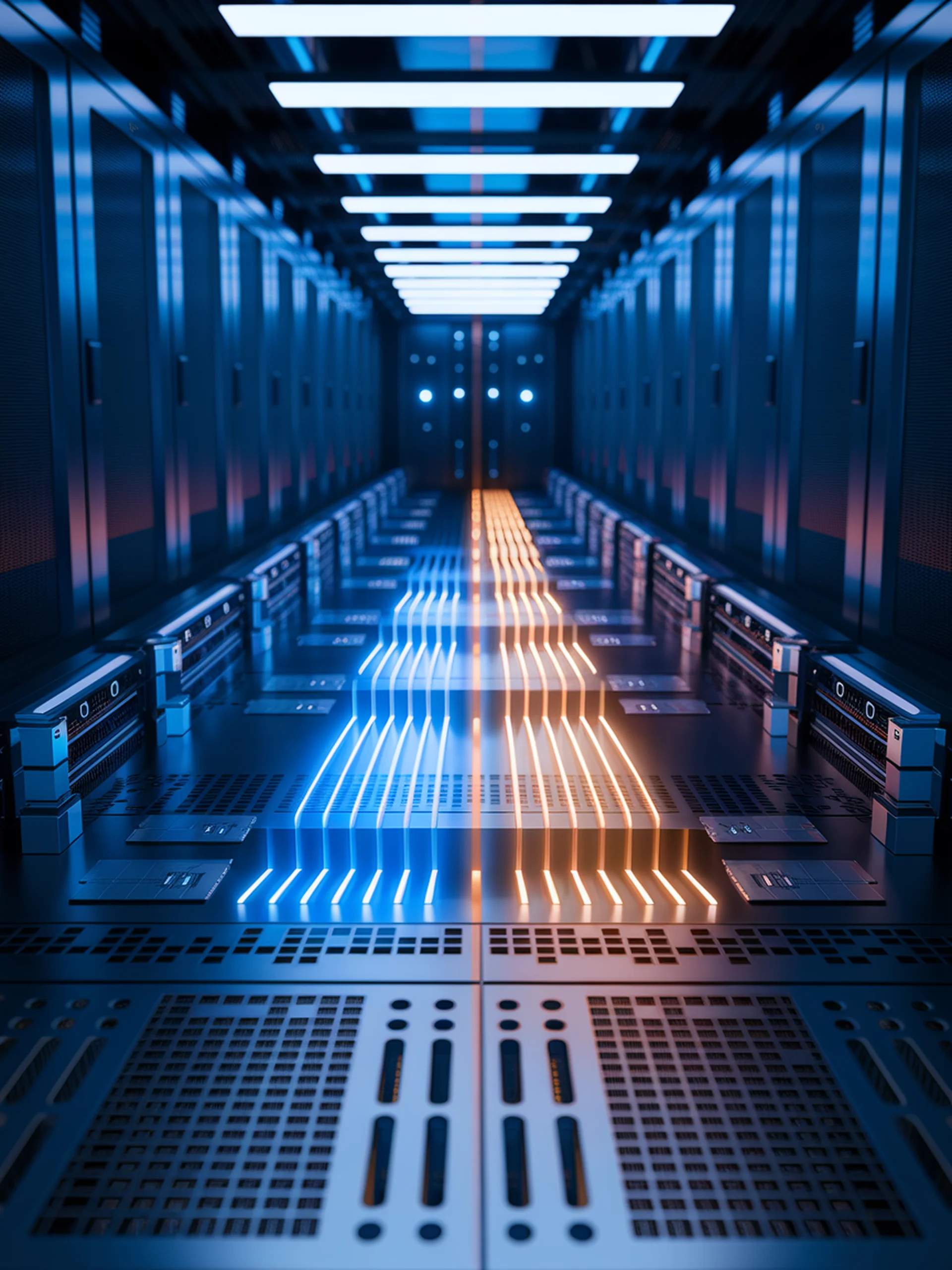
Optimizing LLM Training for Long Sequences
A Novel Pipeline Approach to Memory Management

Smarter Token Compression for Multimodal AI
Reducing computational costs without performance loss
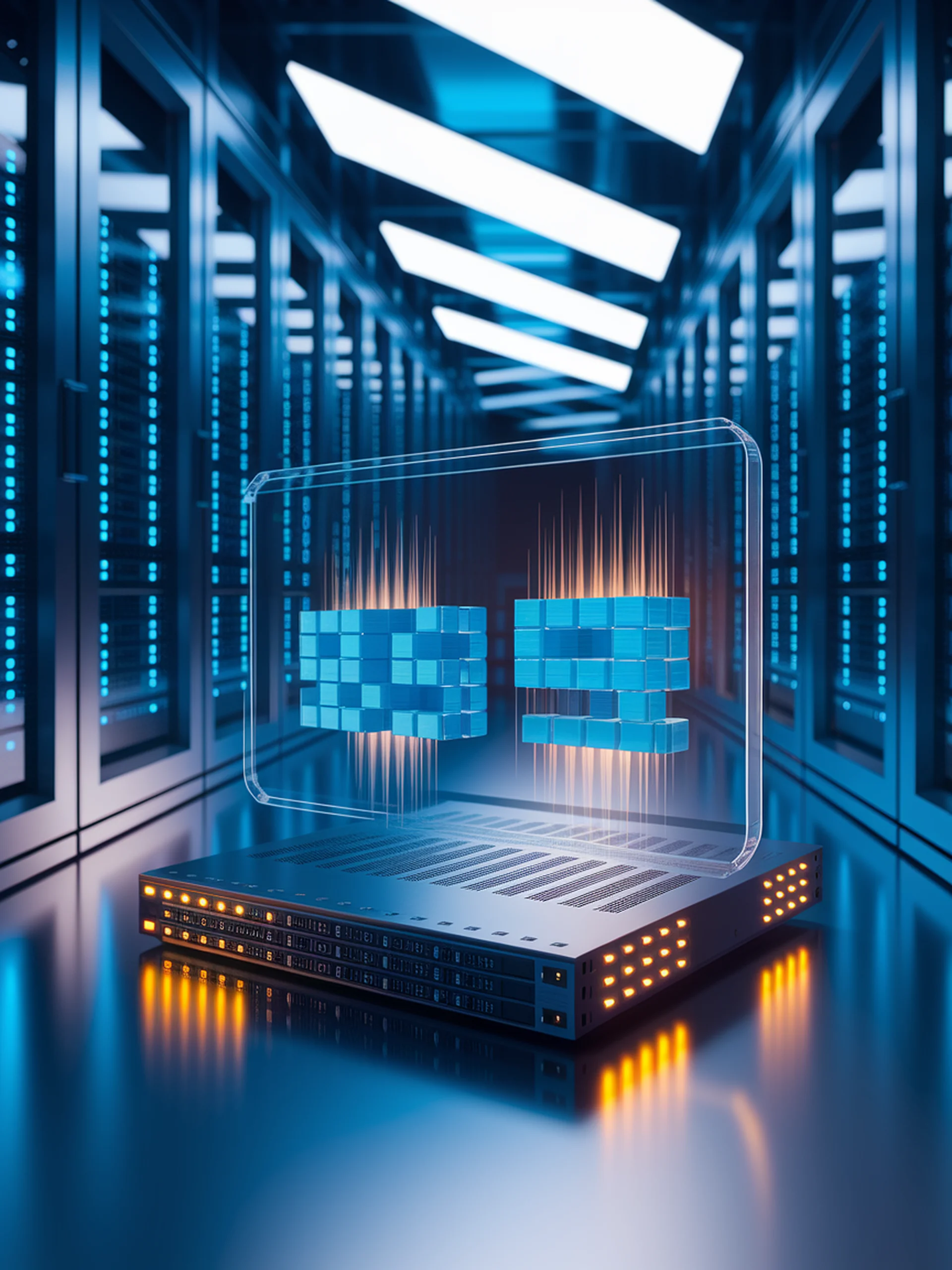
Memory-Efficient LLMs for Long Contexts
Zero-shot compression technique for KV caches without retraining
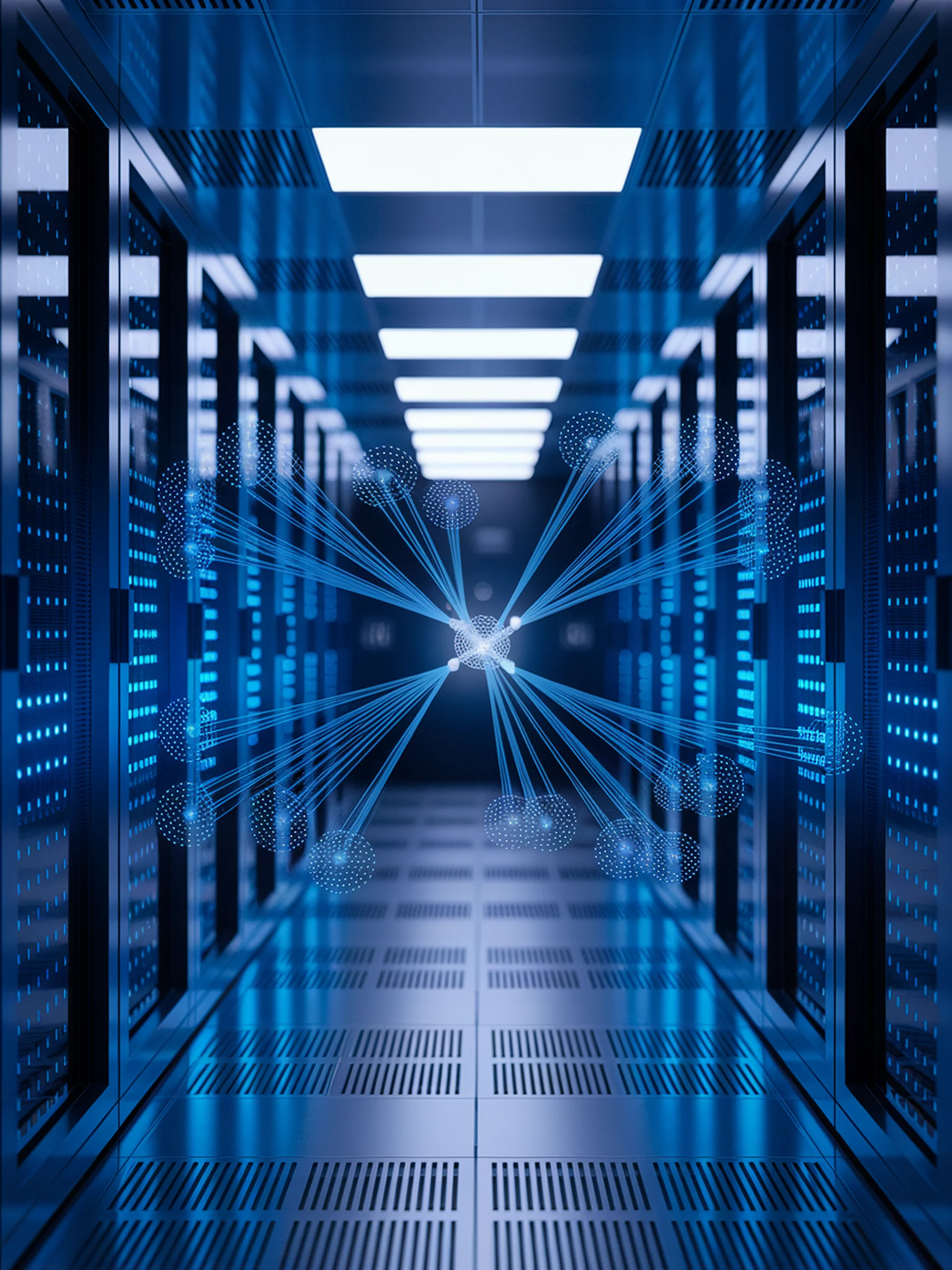
Making LLMs More Reliable Through Efficient Ensembling
A scalable framework for improving consistency across multiple language models
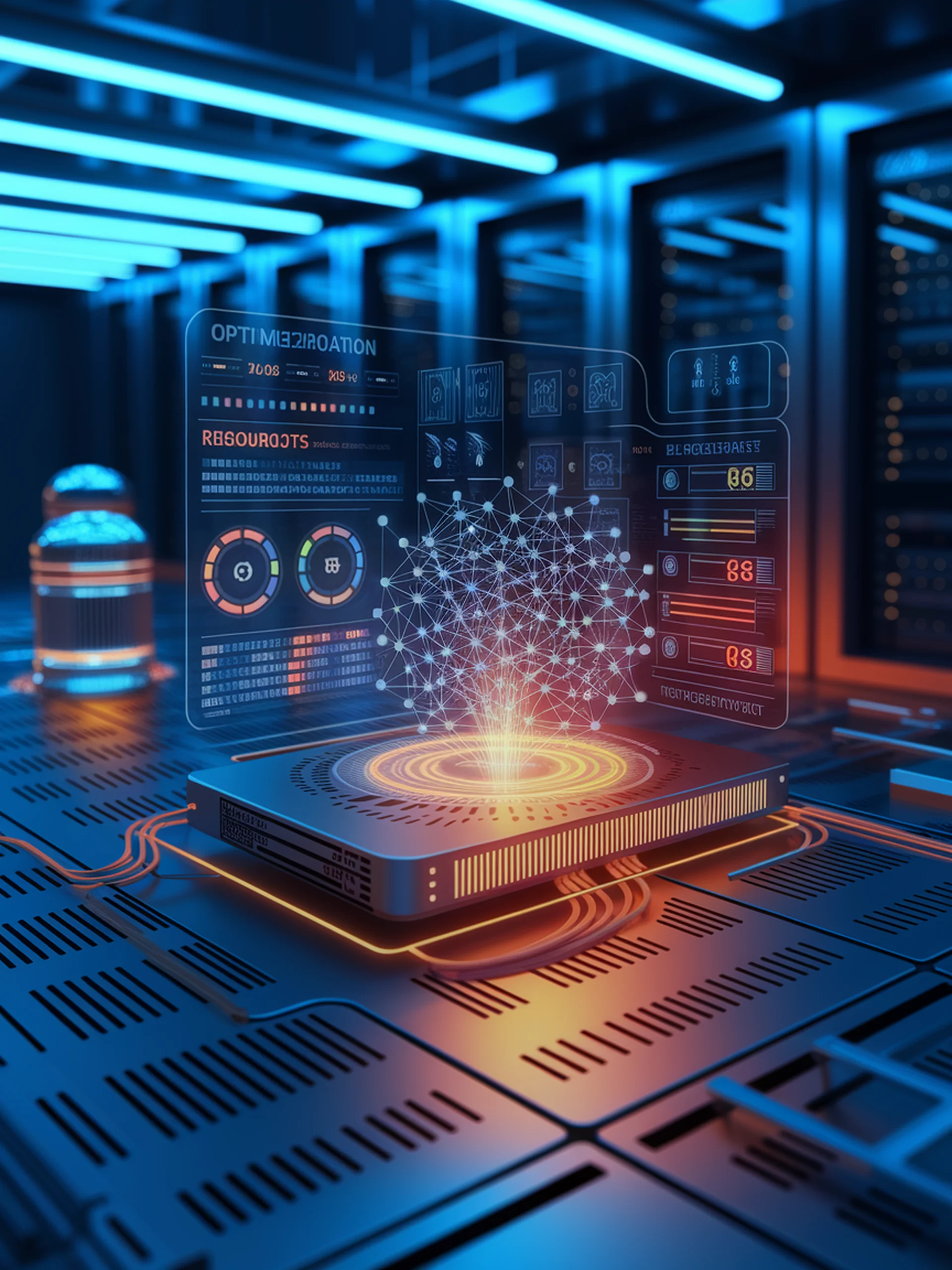
Adaptive Multimodal AI: Doing More with Less
Intelligent resource management for multimodal language models
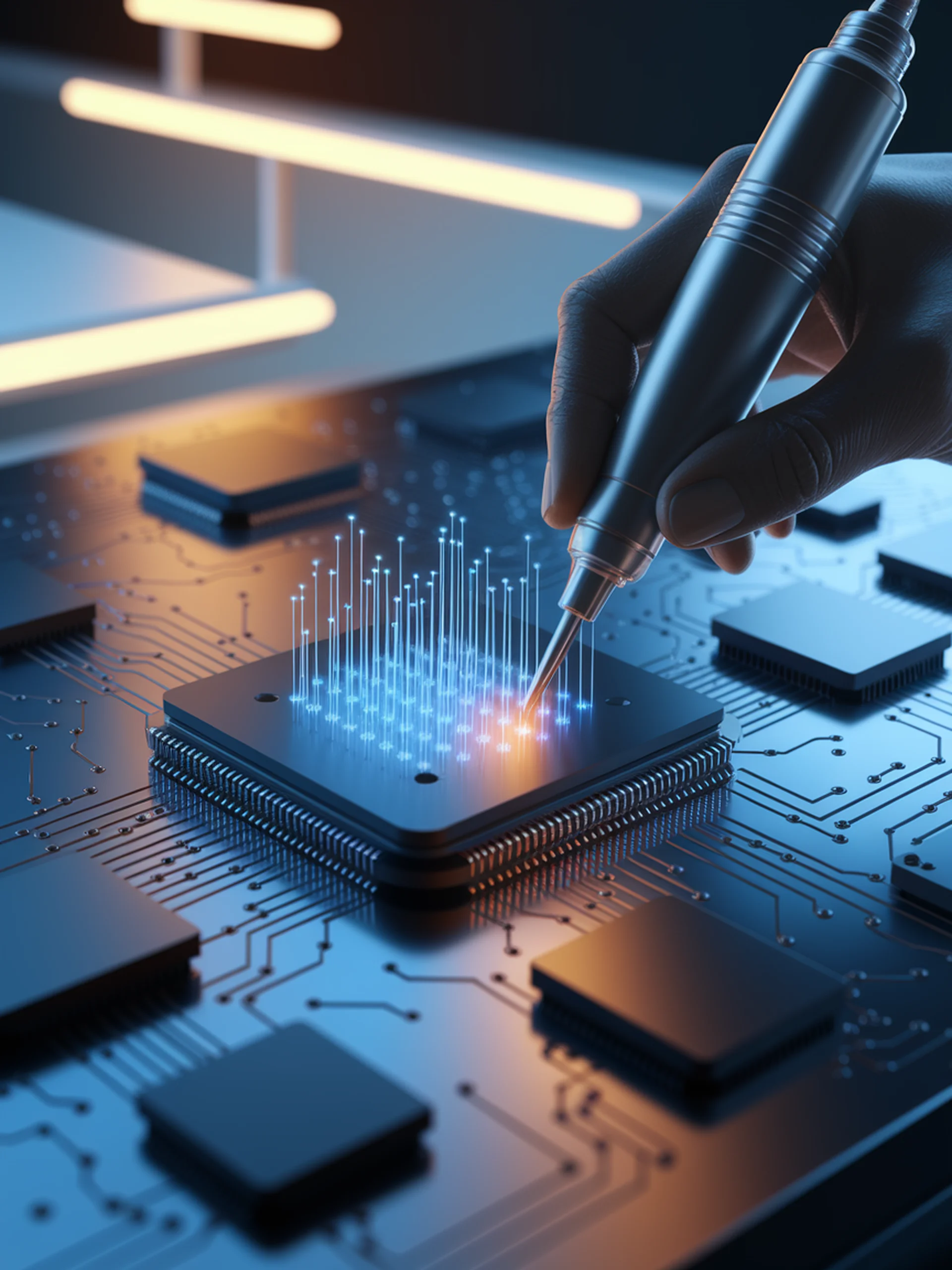
Smart Pruning for Leaner LLMs
Tailoring Sparsity Patterns for Optimal Performance
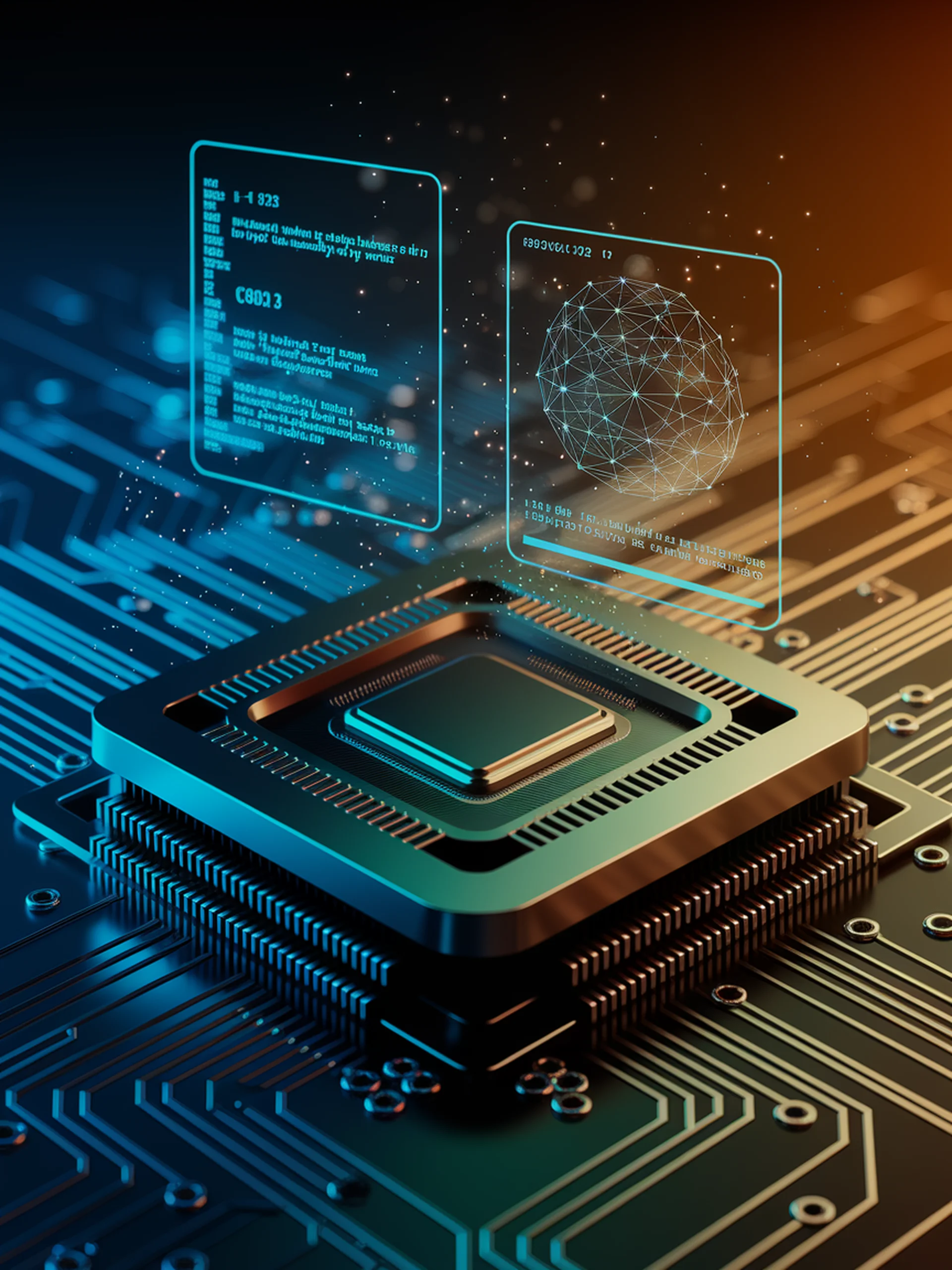
GPU Performance Modeling with LLMs
Harnessing AI to predict GPU program performance
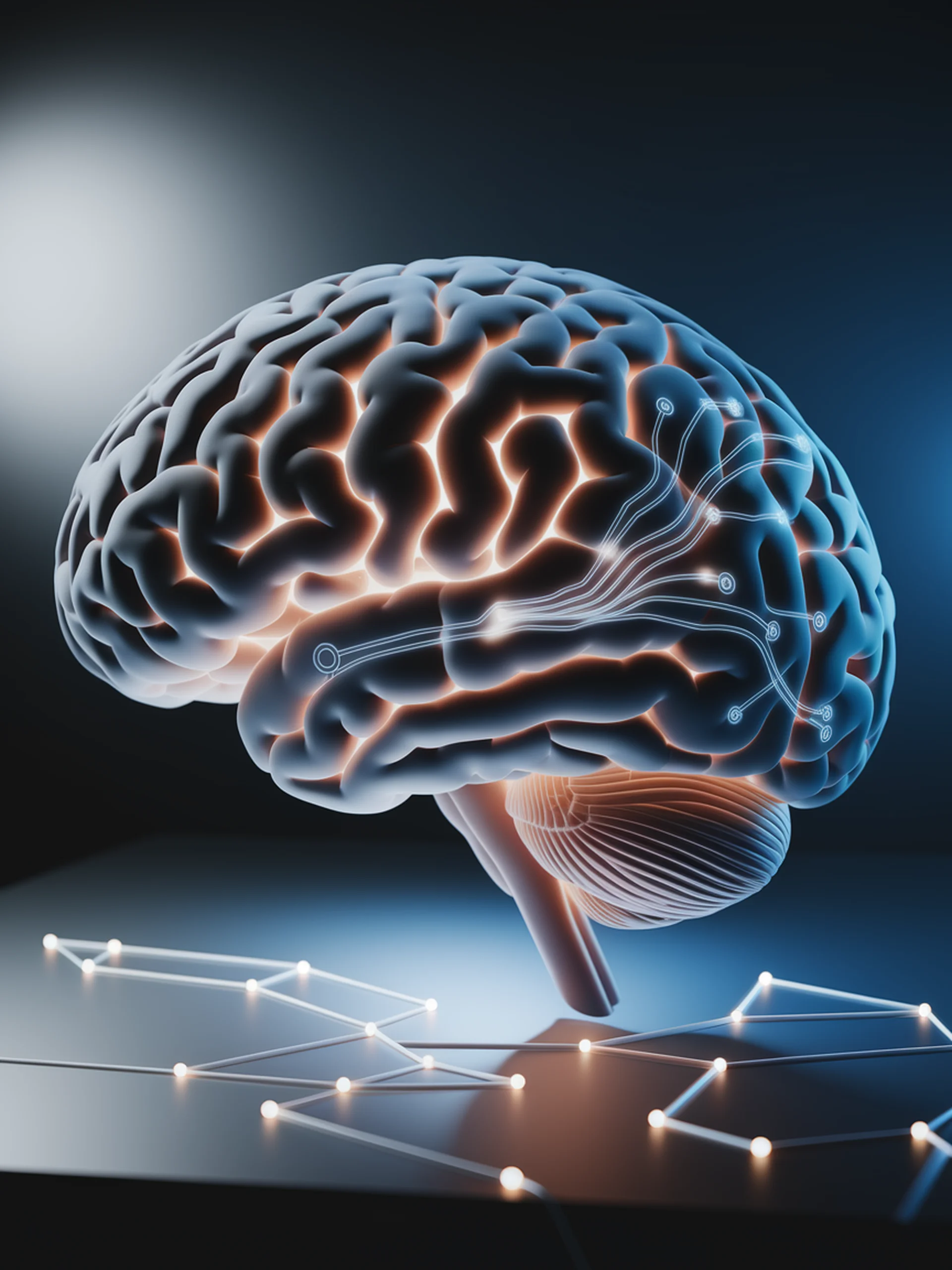
Beyond Transformers: A New Brain-Inspired AI Architecture
Reimagining language models with neural network interpretability
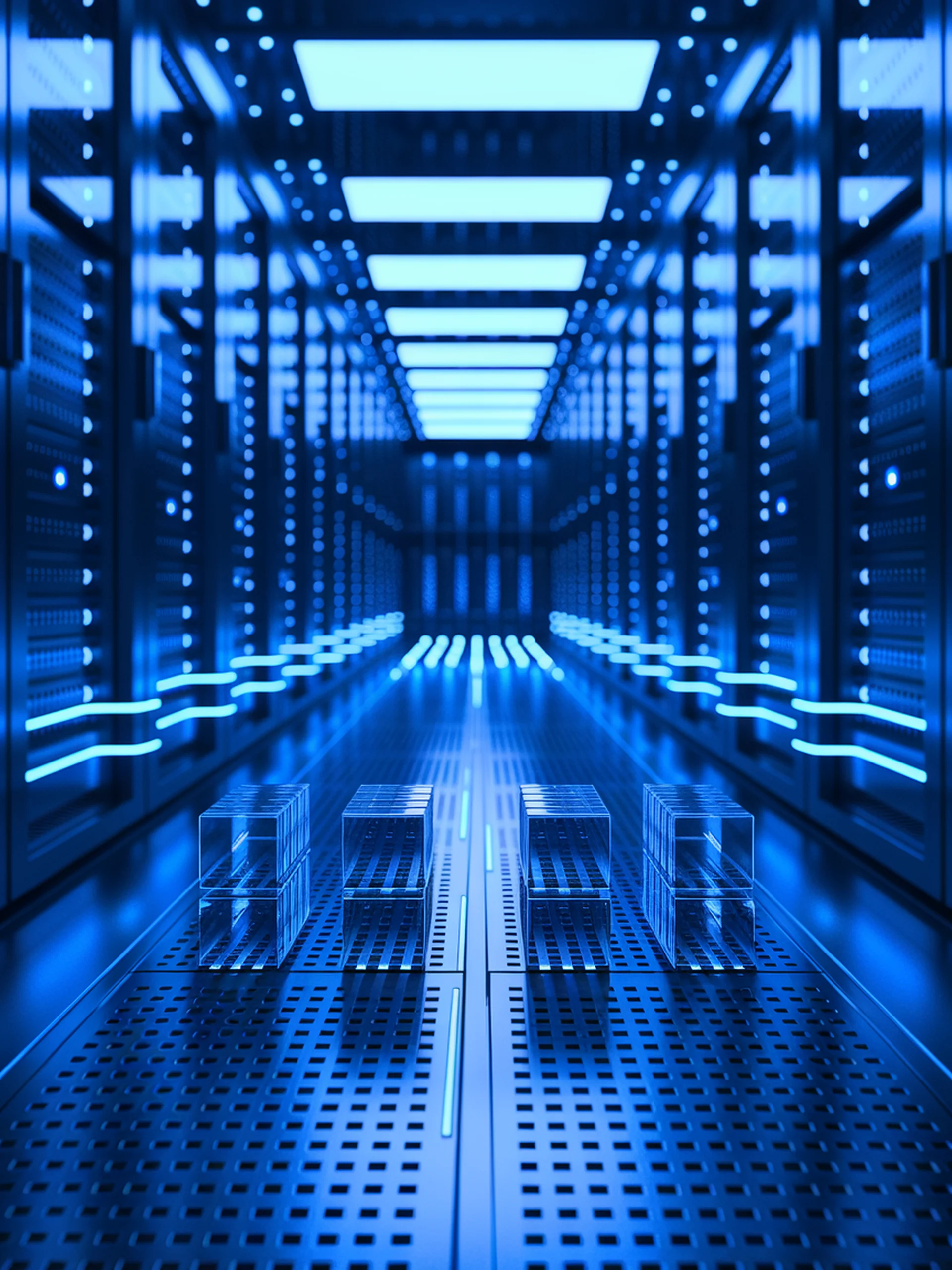
Optimizing LLM Memory Efficiency
Systematic analysis of KV cache compression techniques
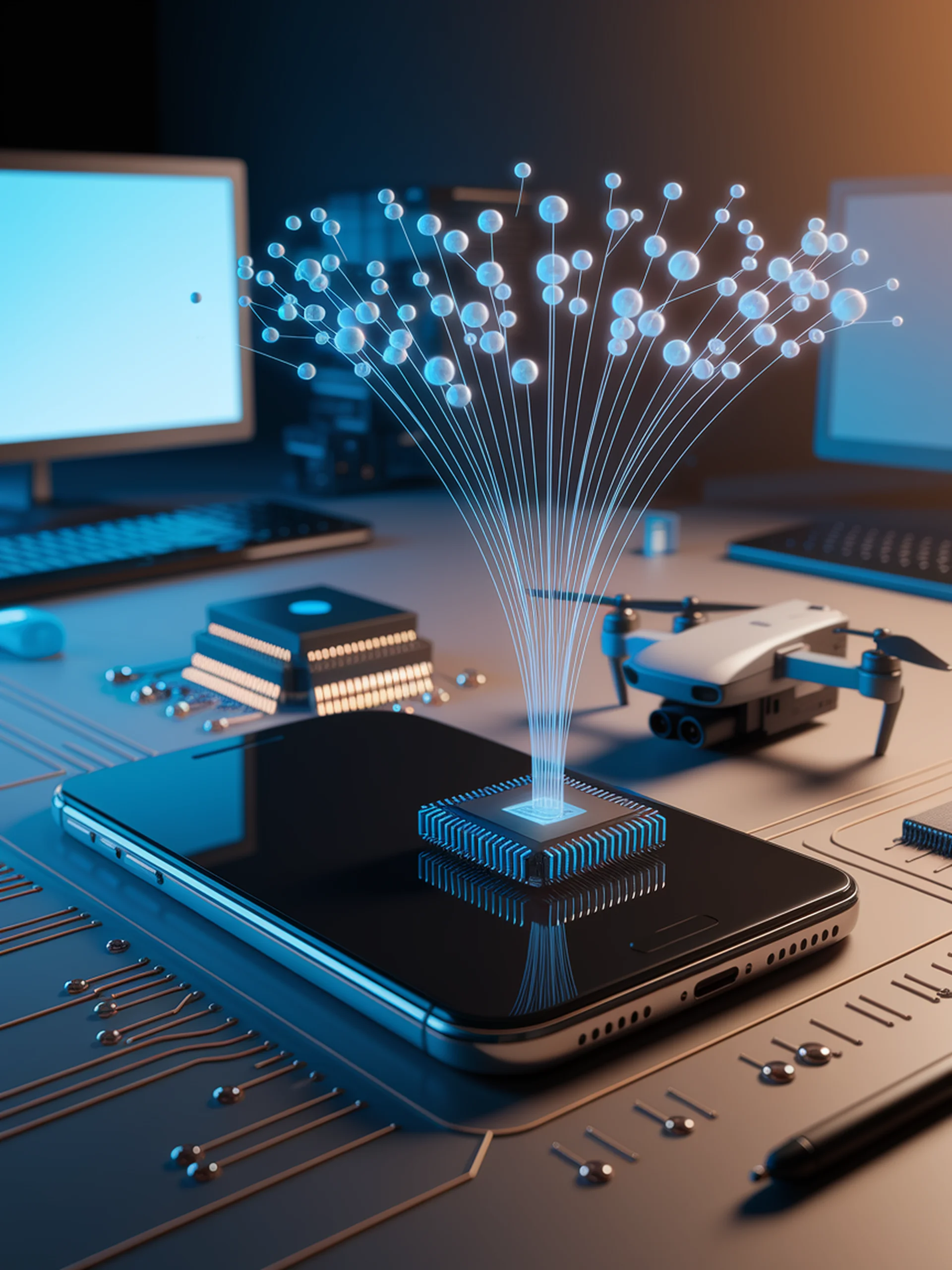
Shrinking AI Giants for Edge Devices
Knowledge Distillation to Deploy Large Models on Resource-Constrained Devices
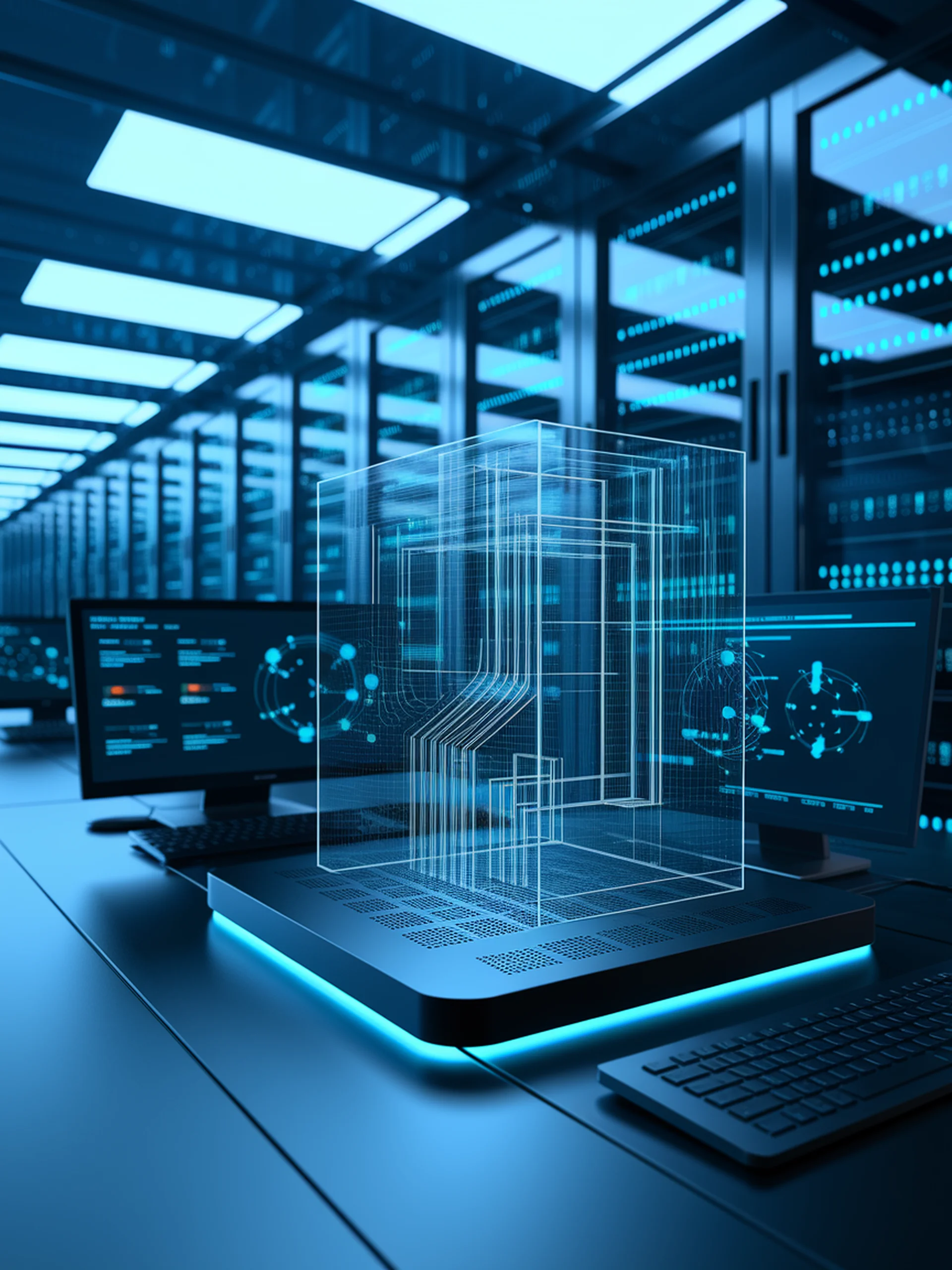
Smarter Model Compression with SVD-LLM V2
Optimizing LLM efficiency through advanced matrix factorization
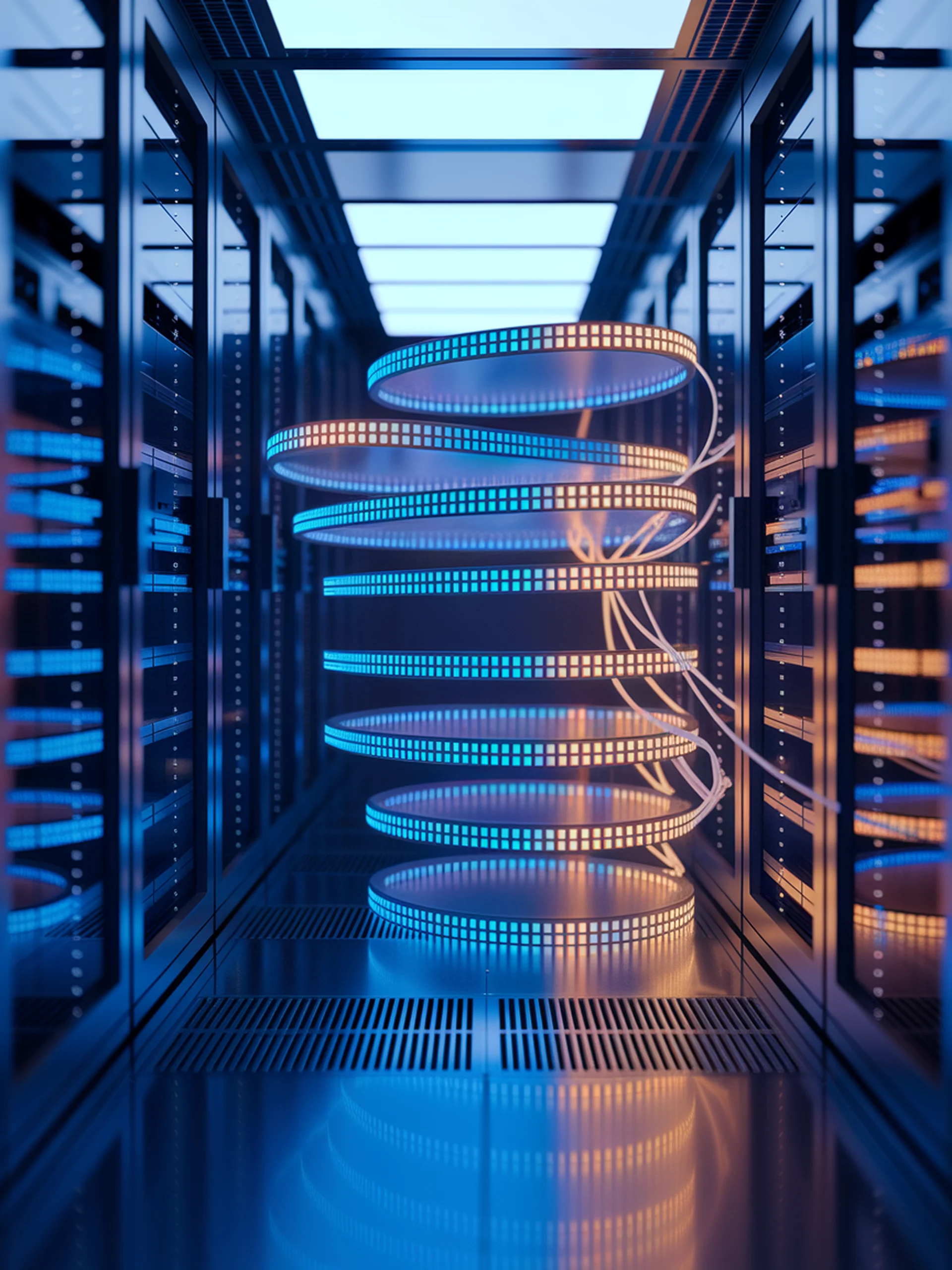
Smarter Memory for Faster LLMs
Introducing CAKE: A Layer-Aware Approach to KV Cache Management
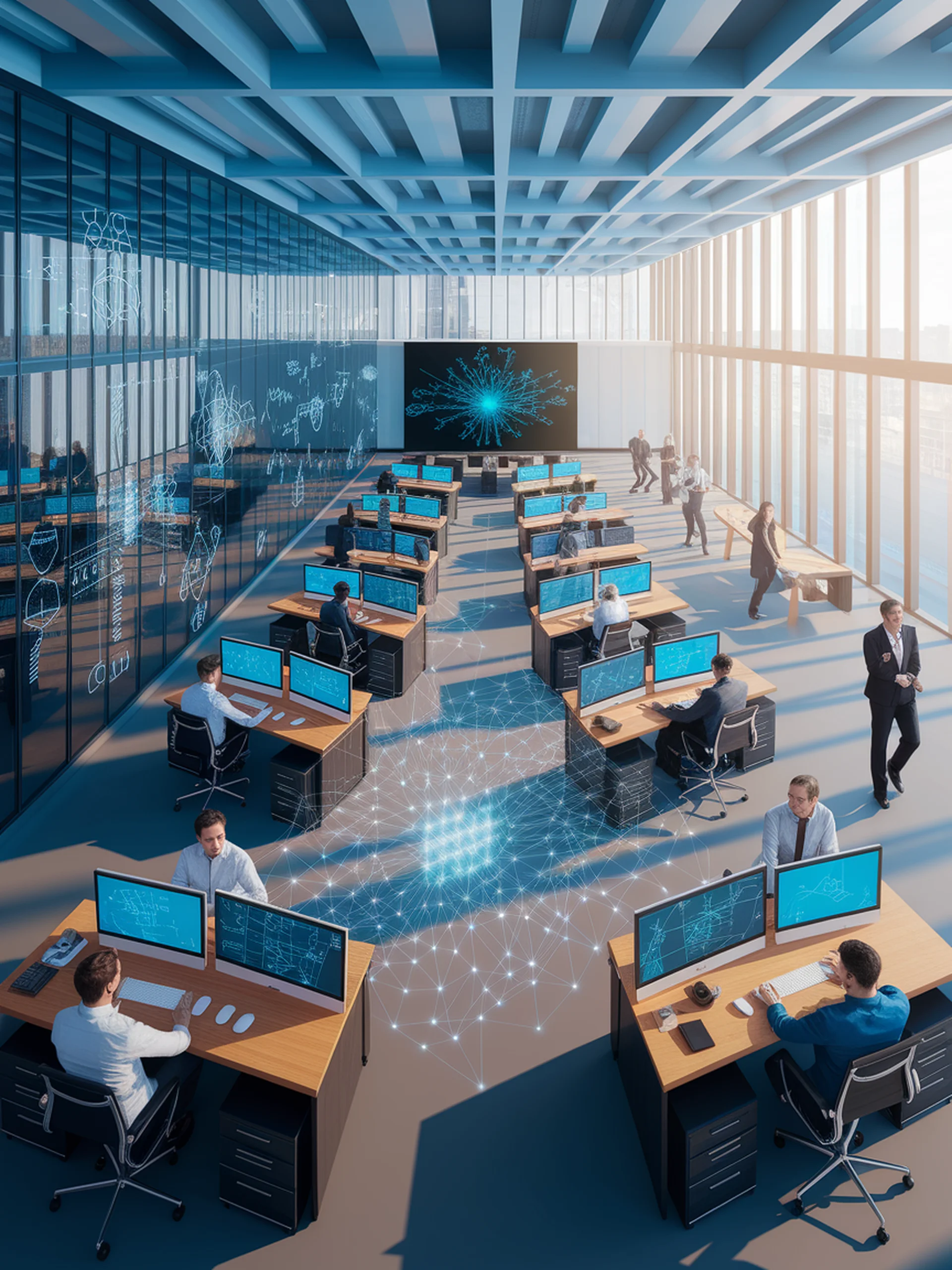
Democratizing LLM Development
Breaking down barriers with collaborative AI expertise
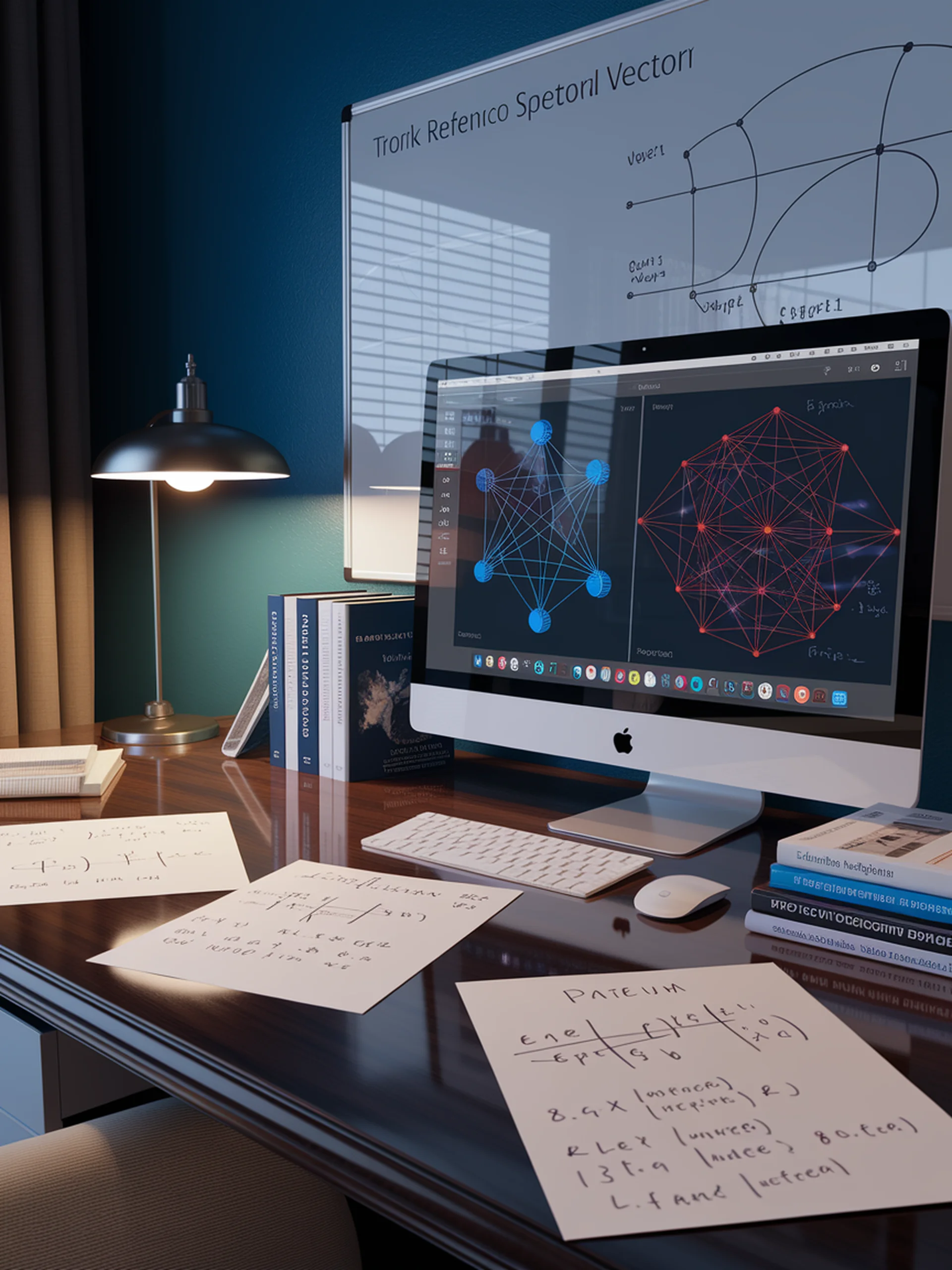
Rethinking Gradient Methods in Deep Learning
A Trust-Region Perspective on Gradient Orthogonalization
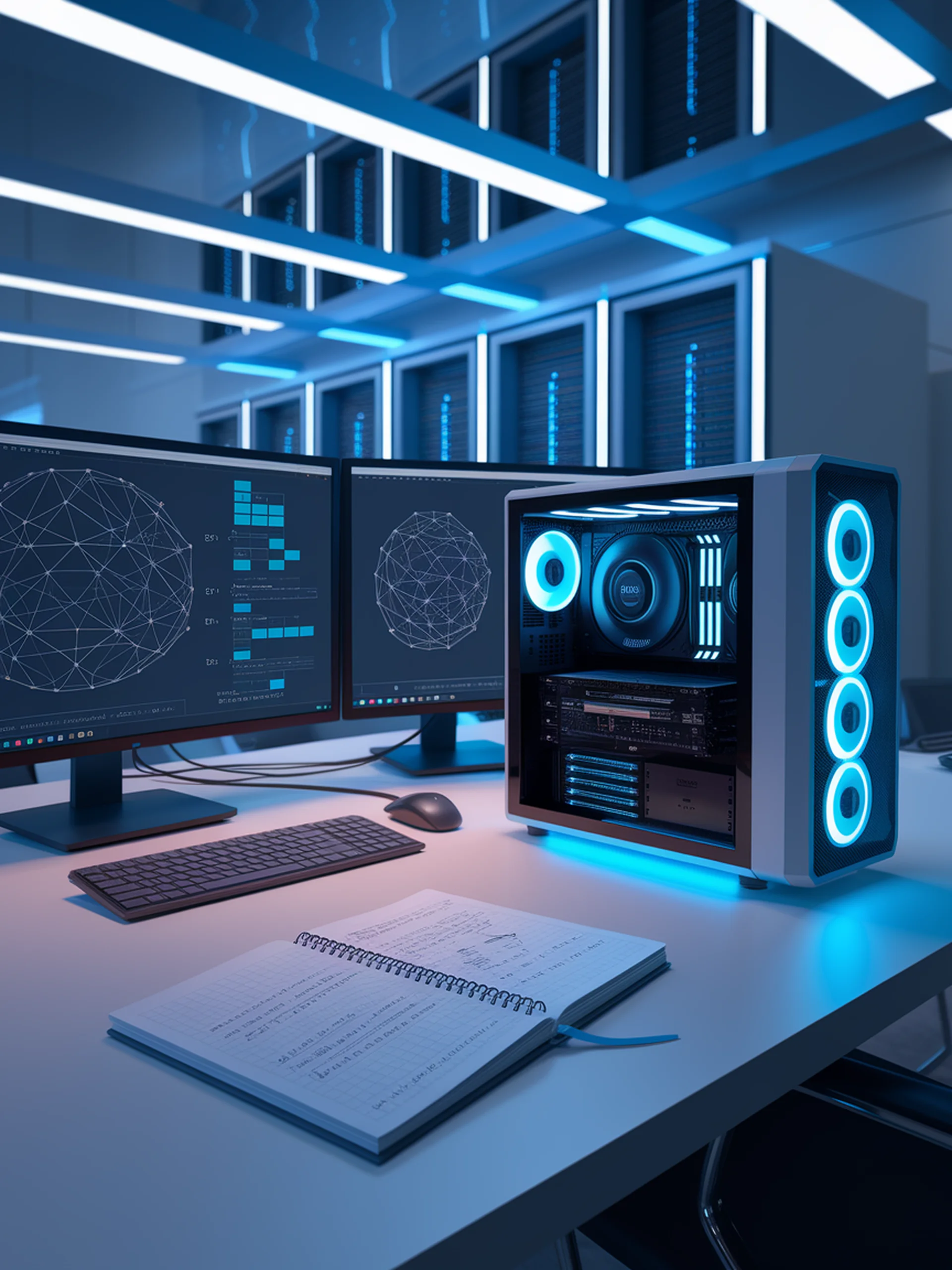
Breaking Memory Barriers for LLM Fine-Tuning
A Zeroth-Order Approach for Training Giant Models With Limited GPU Resources
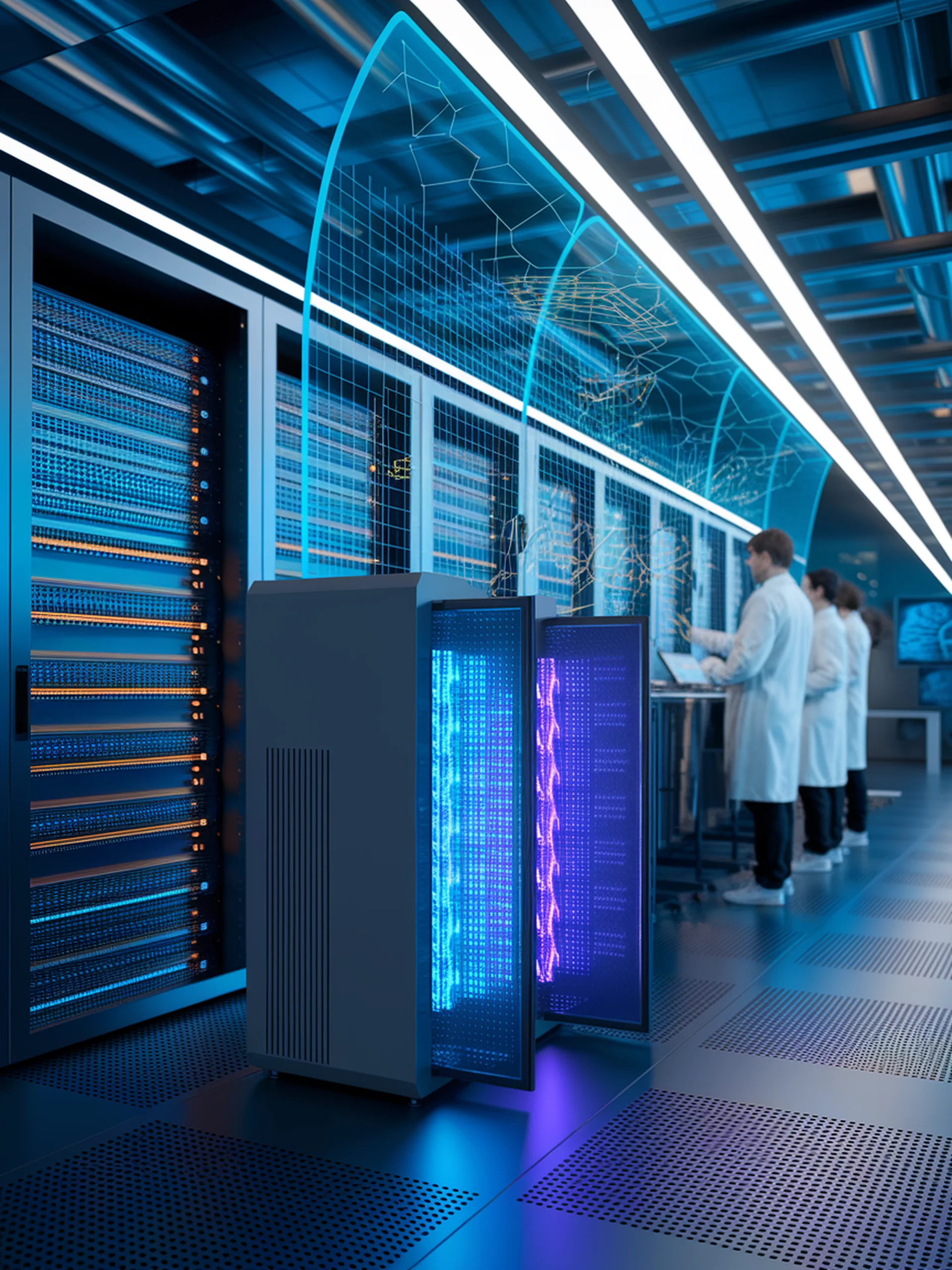
Quantum Computing Meets LLMs
Breaking the Low-Rank Bottleneck in Fine-Tuning
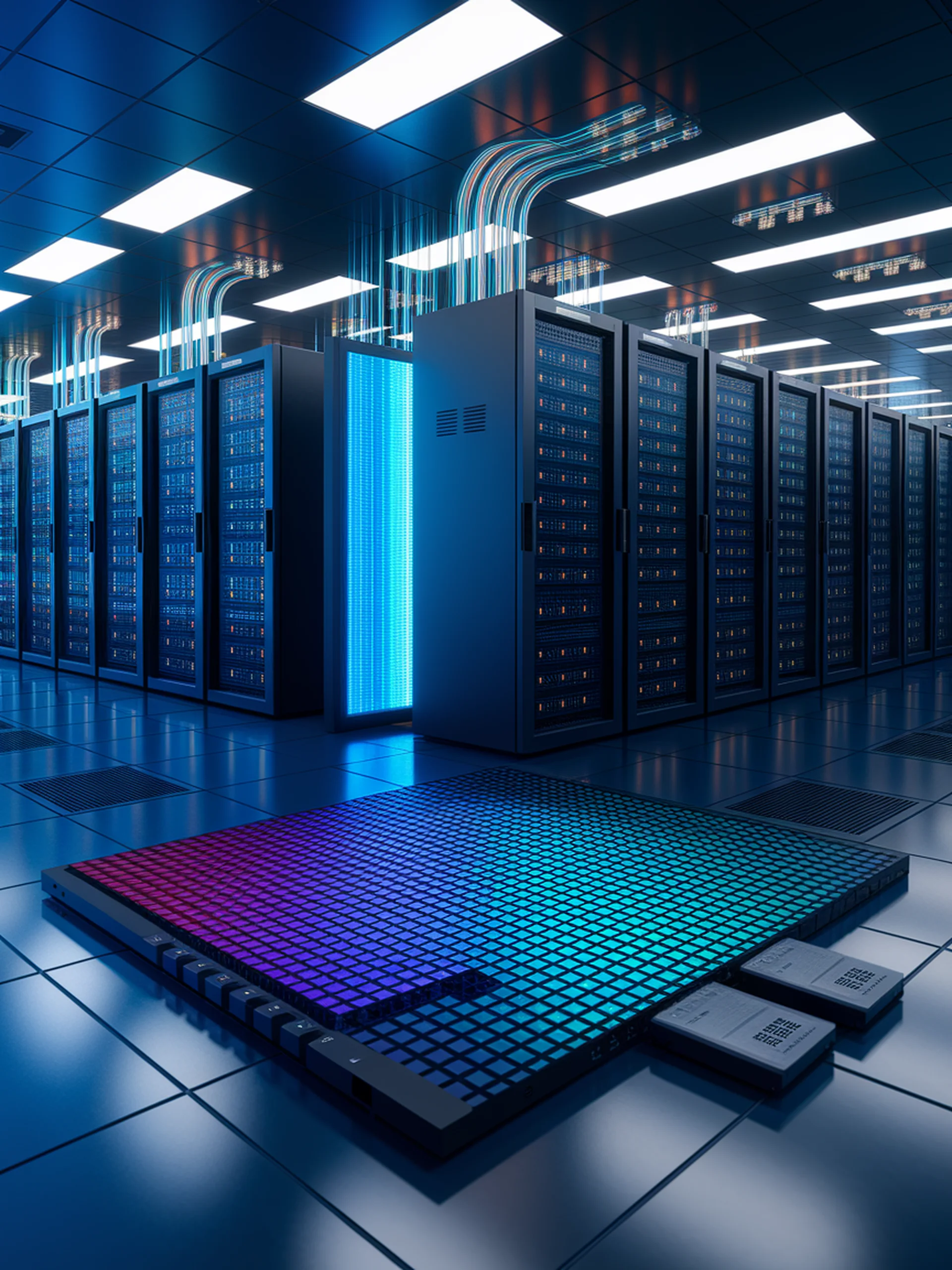
Efficient LLM Compression
How ClusComp Makes Large Models Smaller and Faster to Finetune
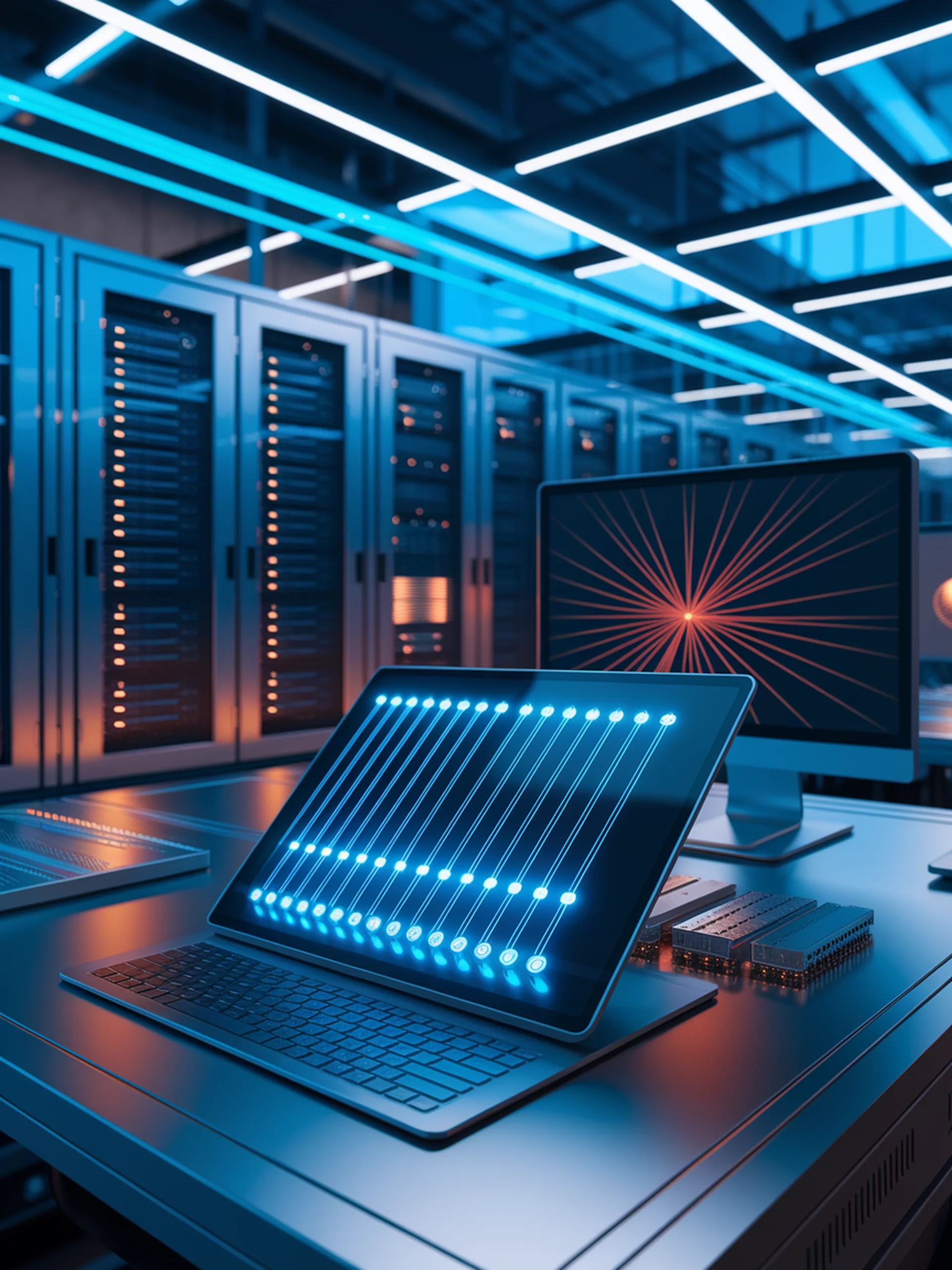
Recurrent LLMs: The New Speed Champions
How xLSTM architecture delivers faster, more efficient inference
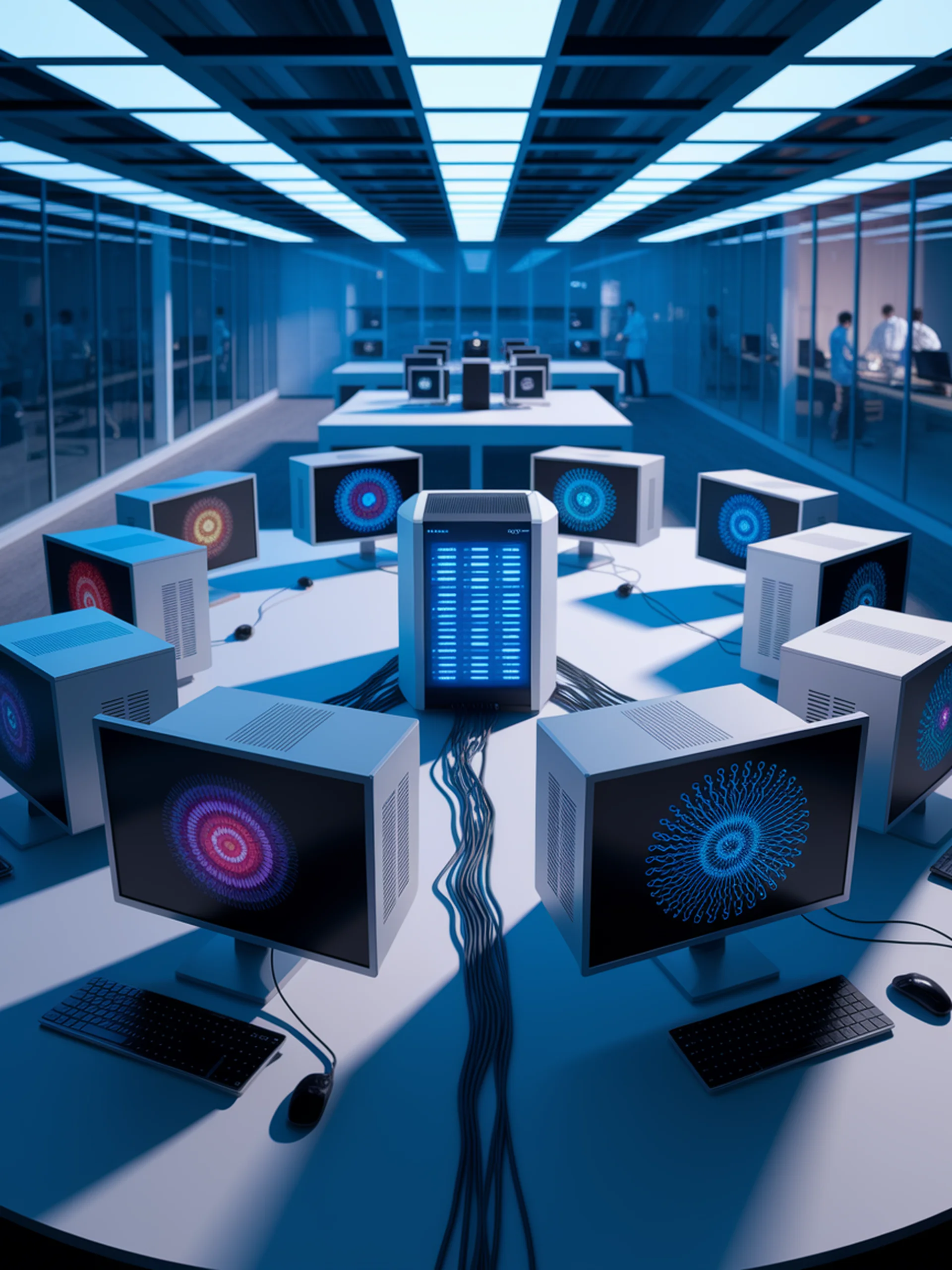
Boosting LLM Performance Through Ensemble Learning
Overcoming limitations of individual models for better text and code generation
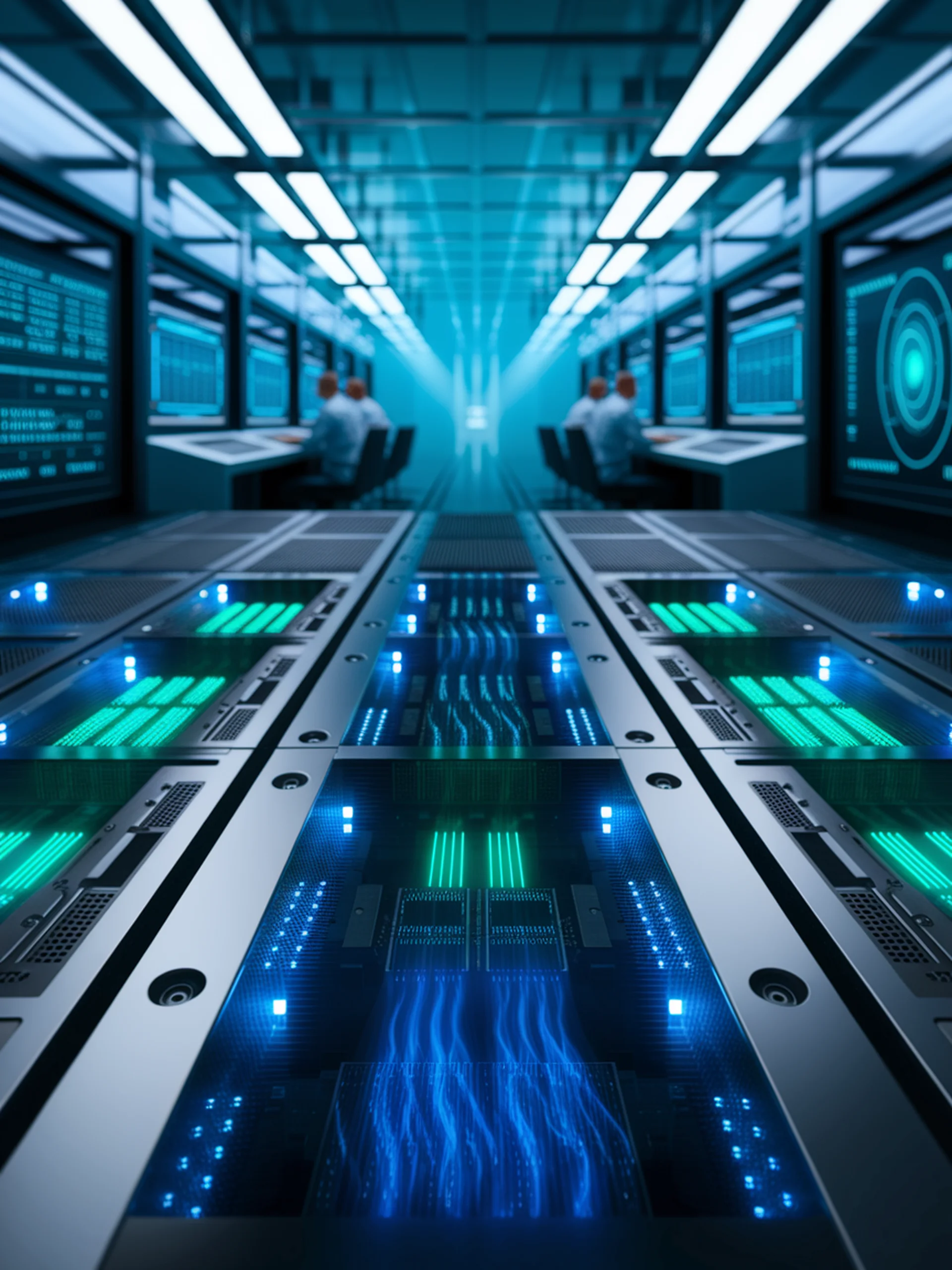
Accelerating LLM Inference
Multi-Level Speculative Decoding with Quantized Drafts
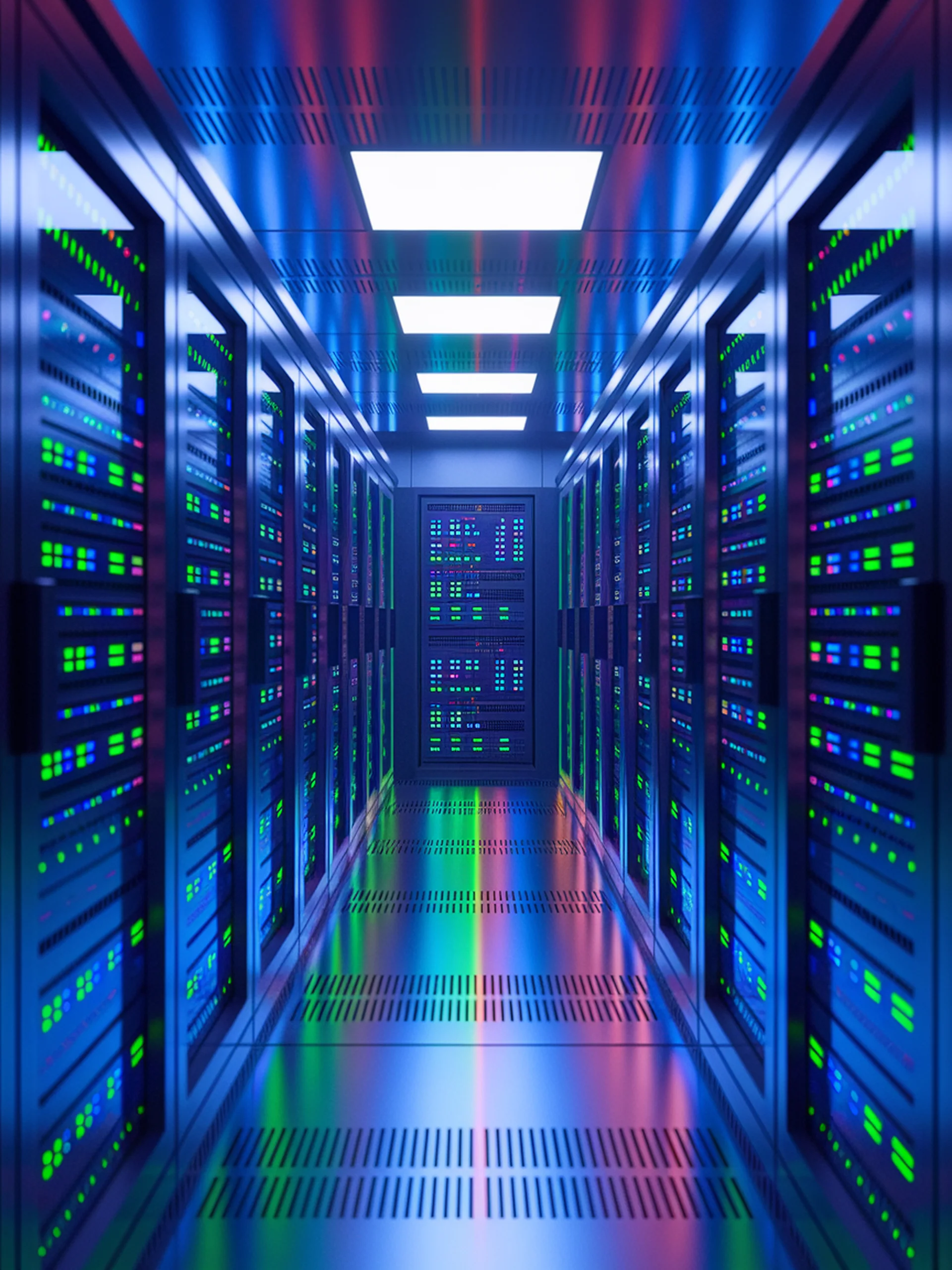
High-Throughput LLM Inference with SLO Guarantees
Optimizing mixed-prompt scenarios with differentiated service levels
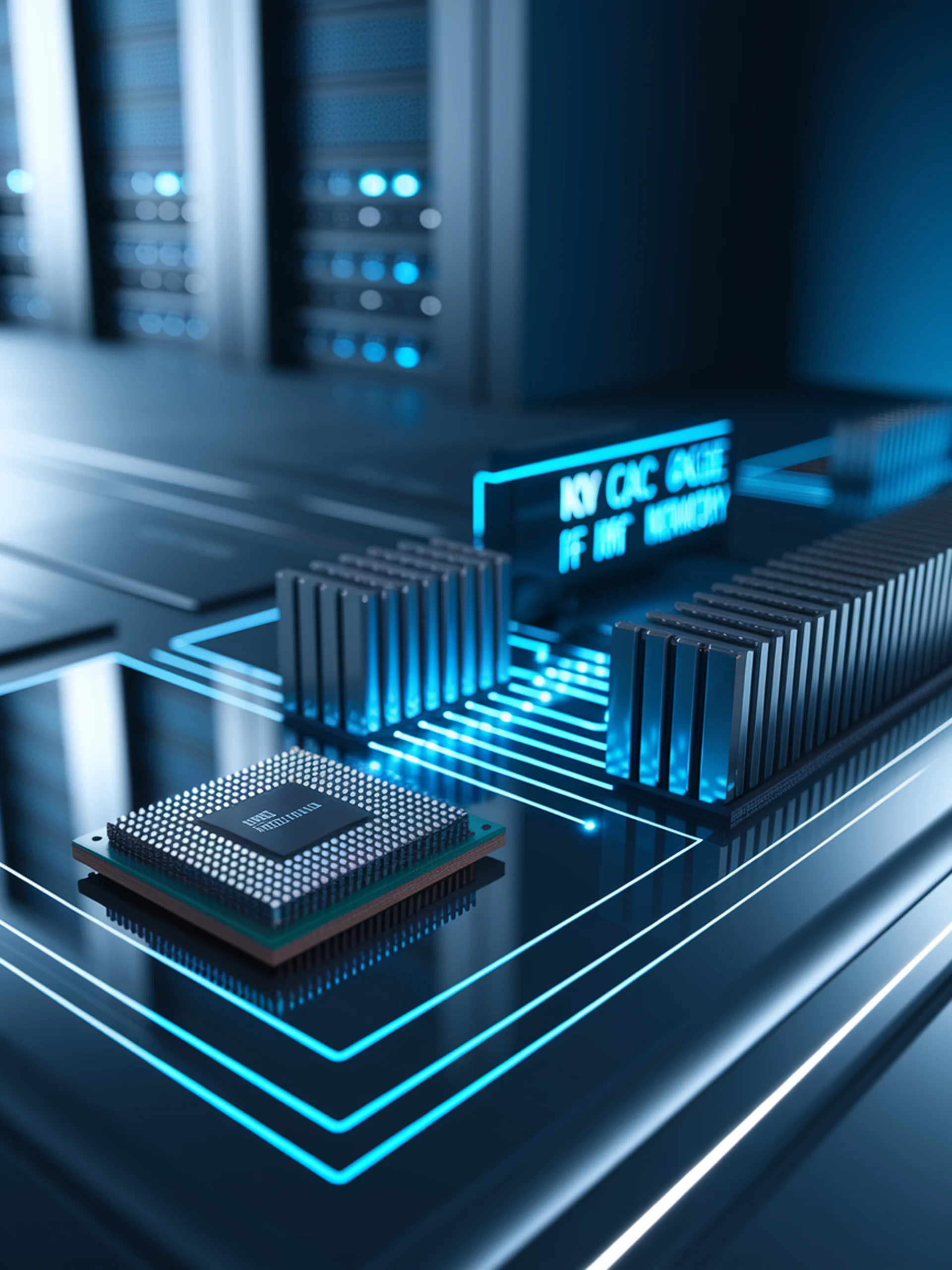
Enhancing LLM Responsiveness
Optimizing KV Cache for Better User Experience
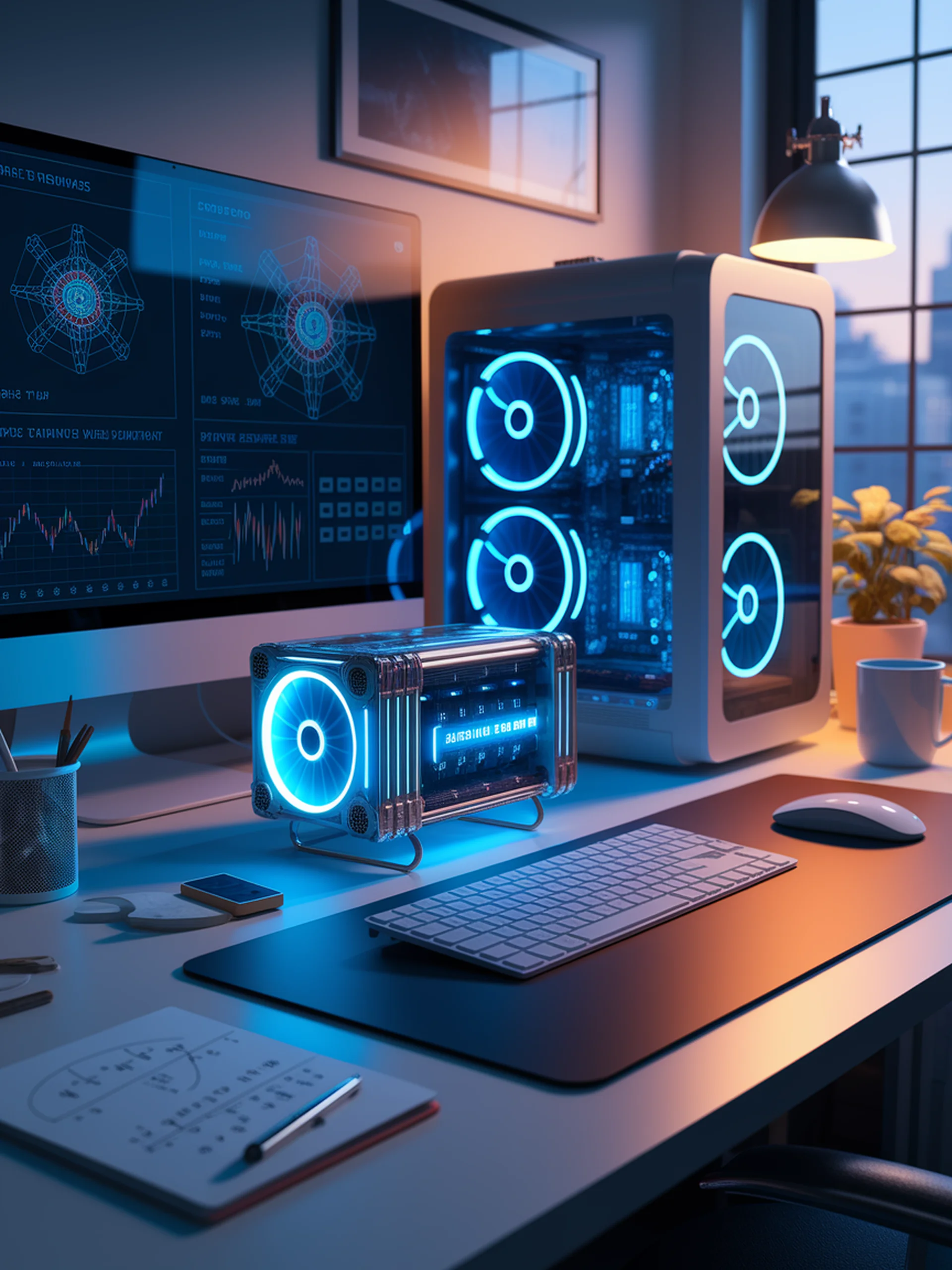
BurTorch: Rethinking DL Training Efficiency
A minimalist approach to high-performance deep learning
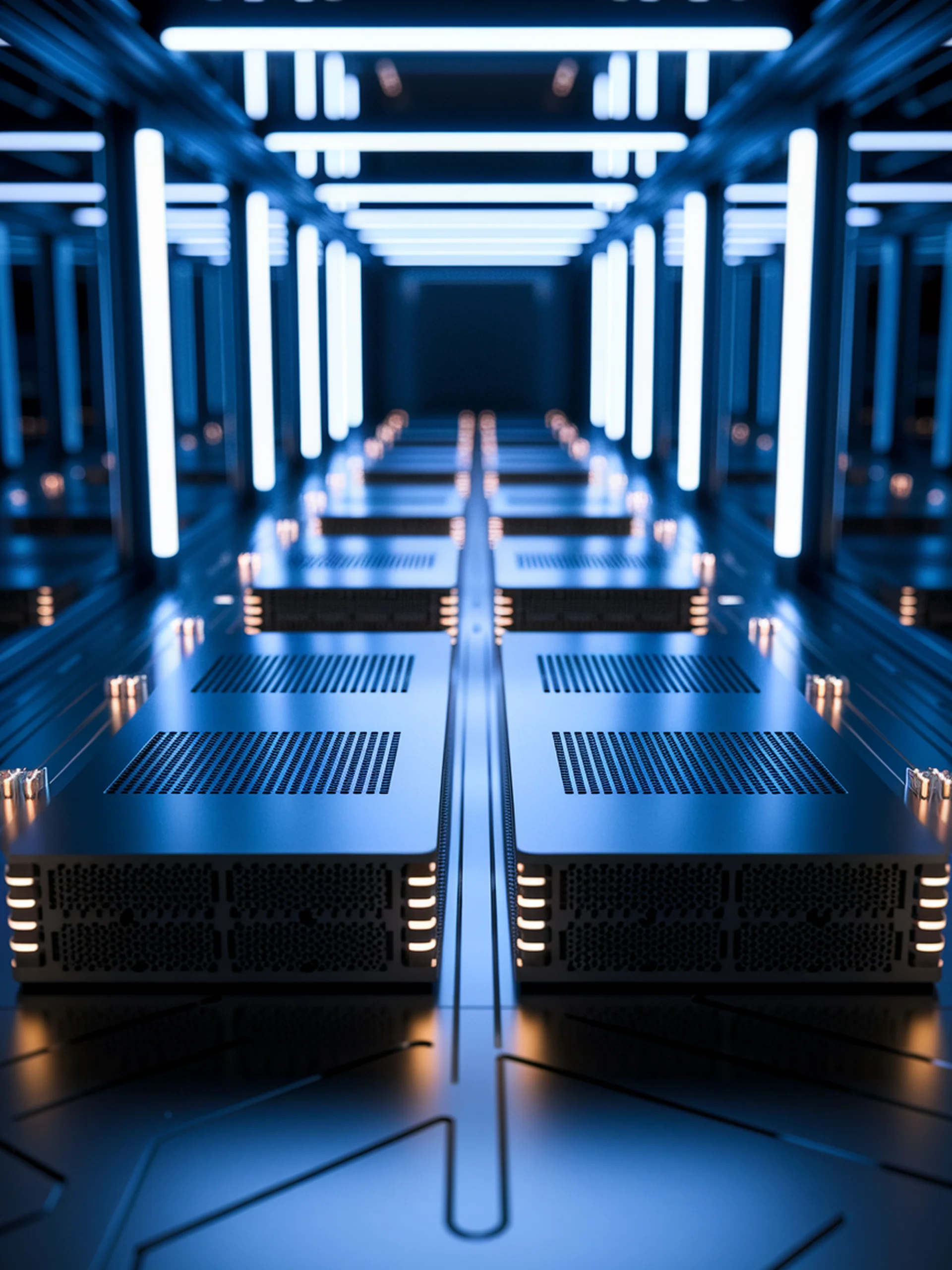
Accelerating Linear RNNs
New kernels for faster sequence processing with linear scaling
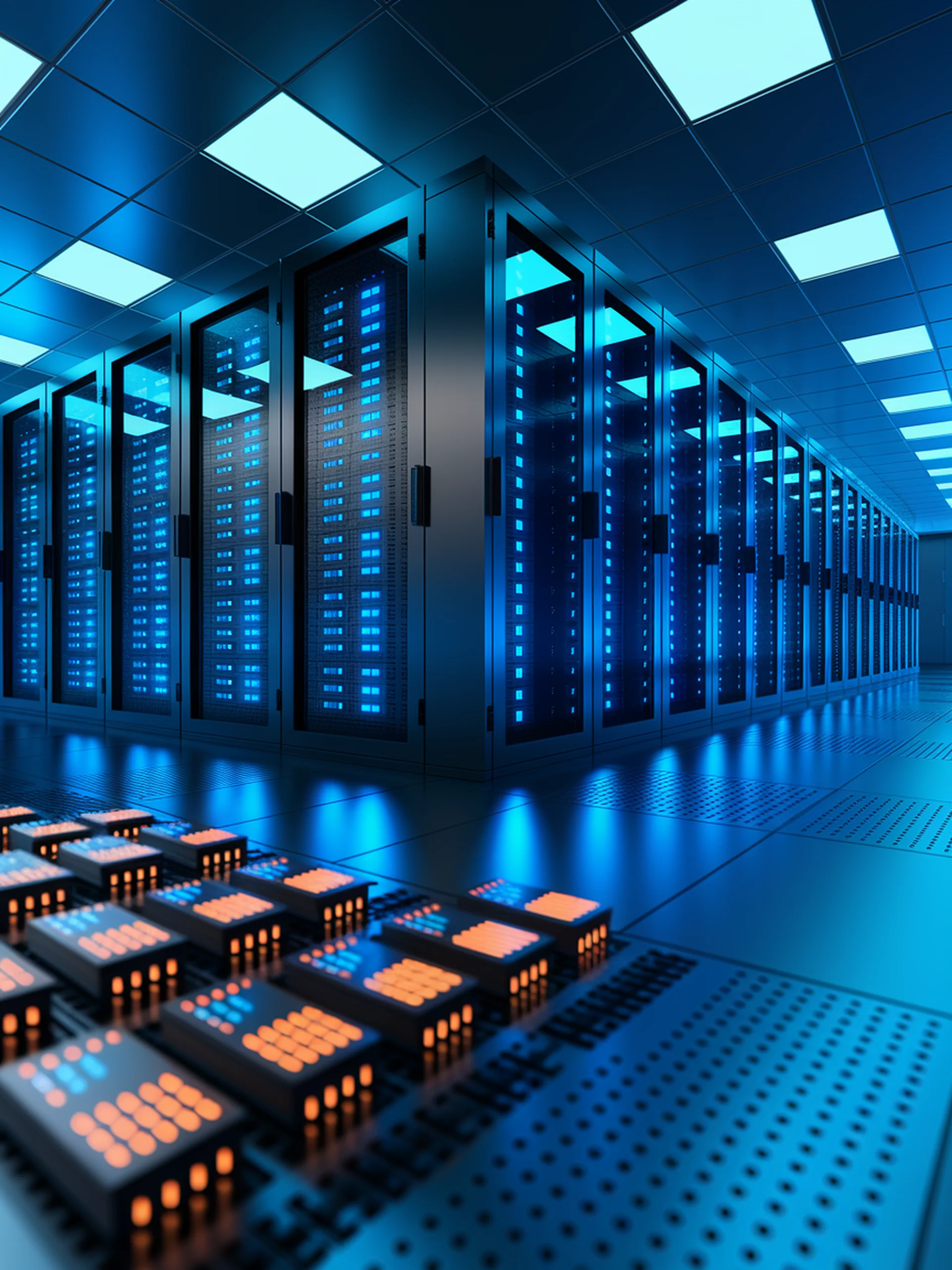
Optimizing LLM Economics with KV Cache Reuse
Making context-augmented LLMs more cost-efficient in the cloud
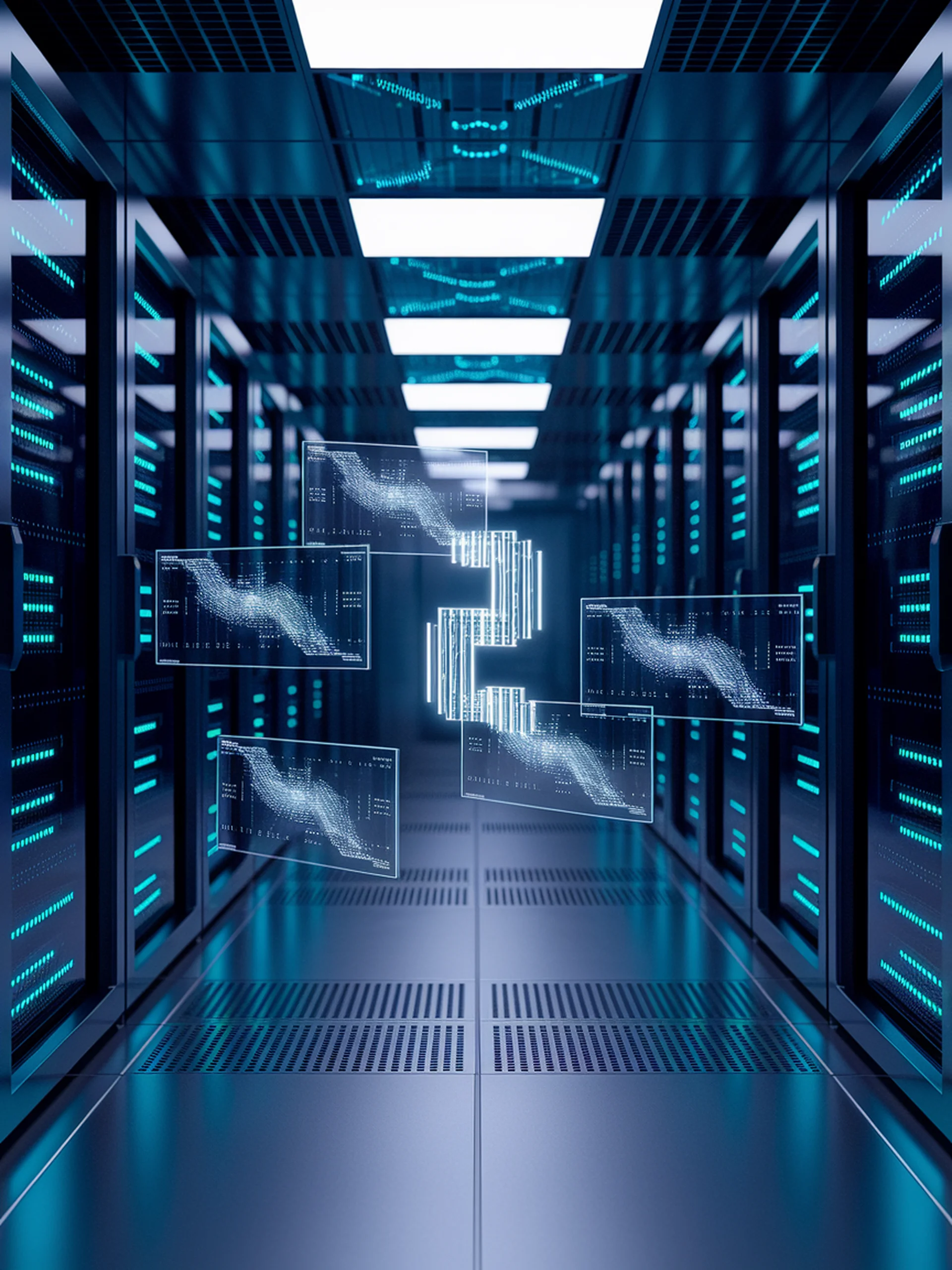
Optimizing RAG Performance
Systematic approach to enhancing retrieval-augmented generation efficiency
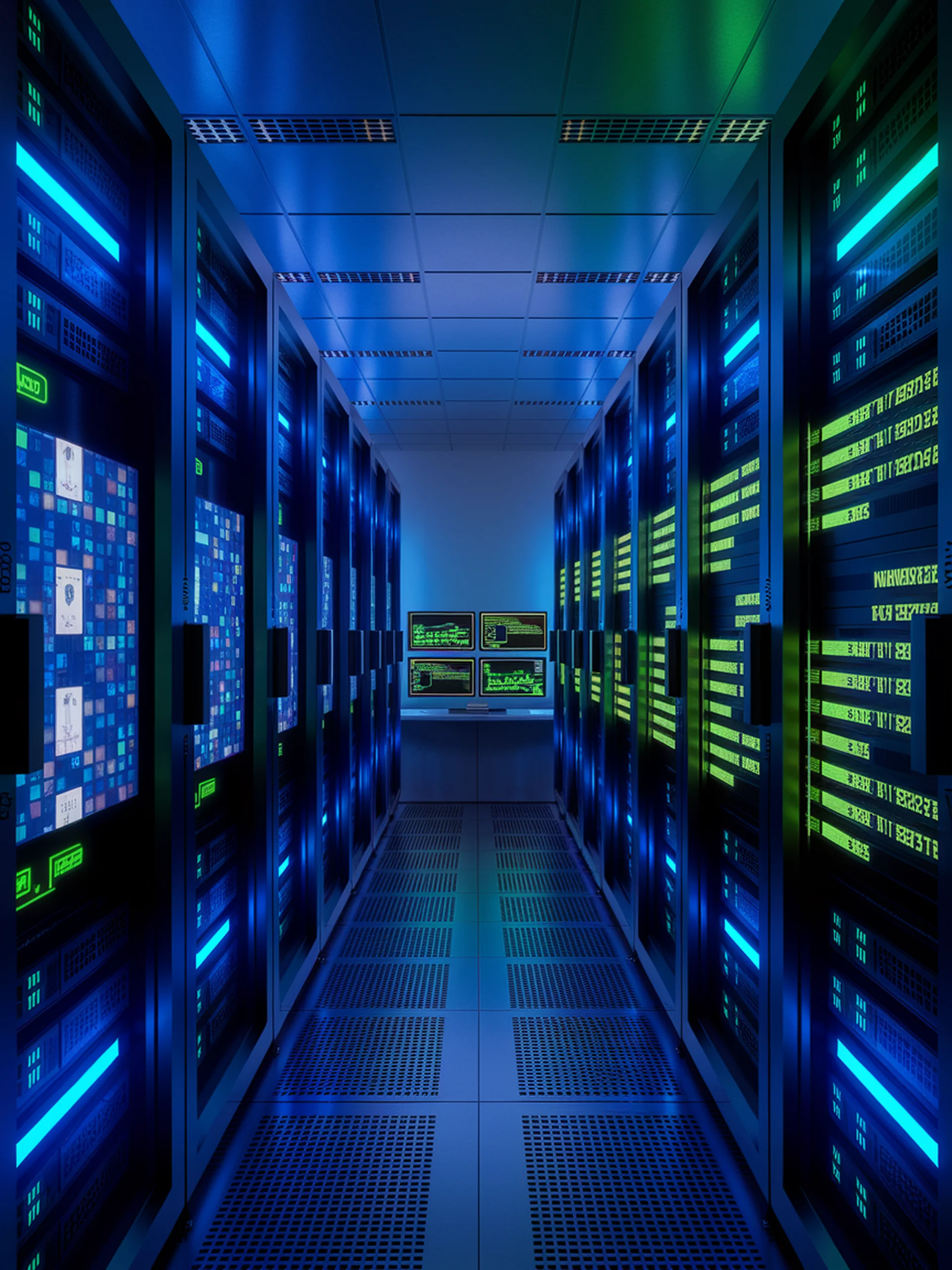
Optimizing LLM and Image Recognition Performance
Efficient task allocation strategies for multi-GPU systems
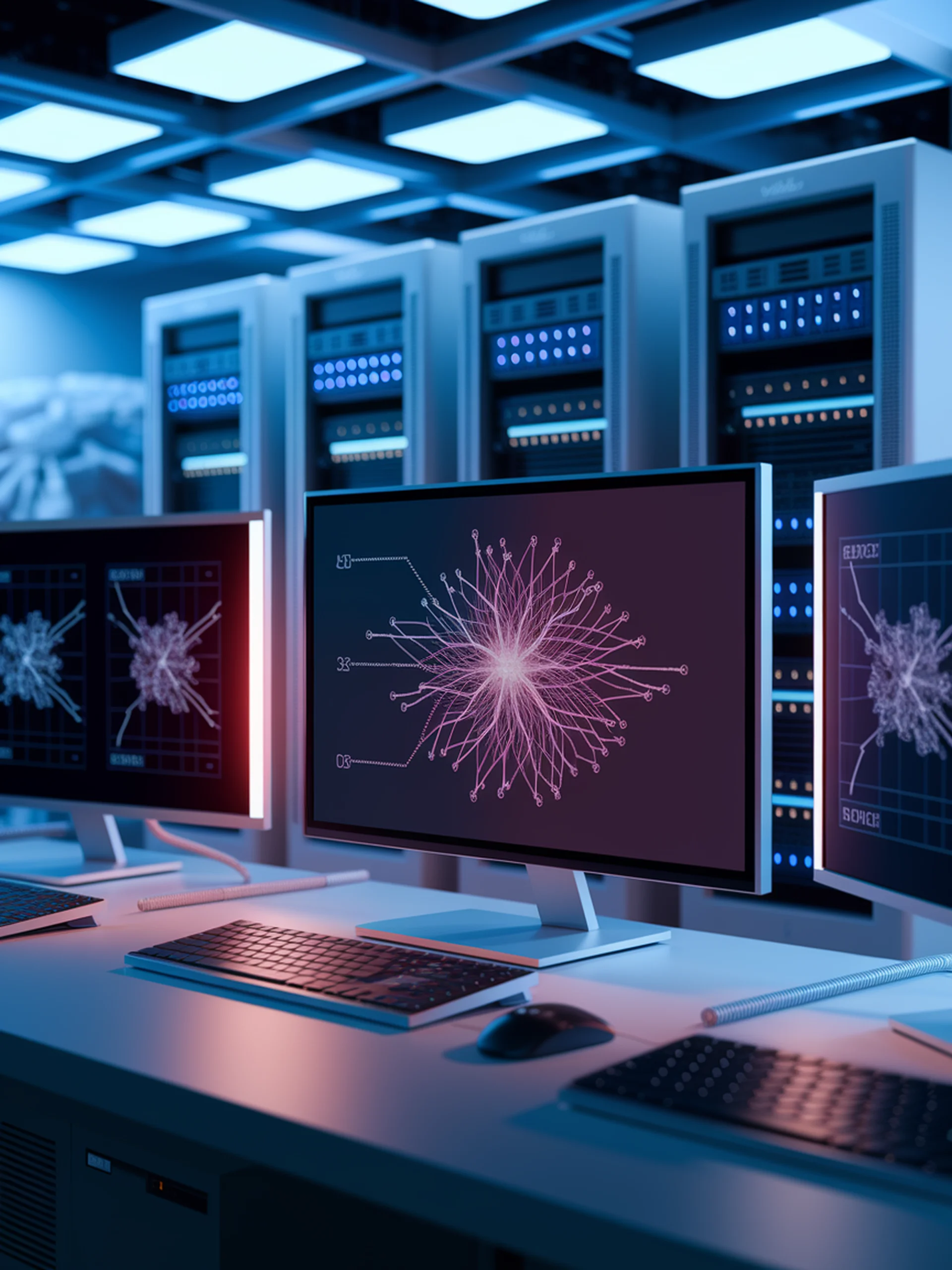
Smarter, Smaller Vision-Language Models
Automated pruning for efficient multimodal AI
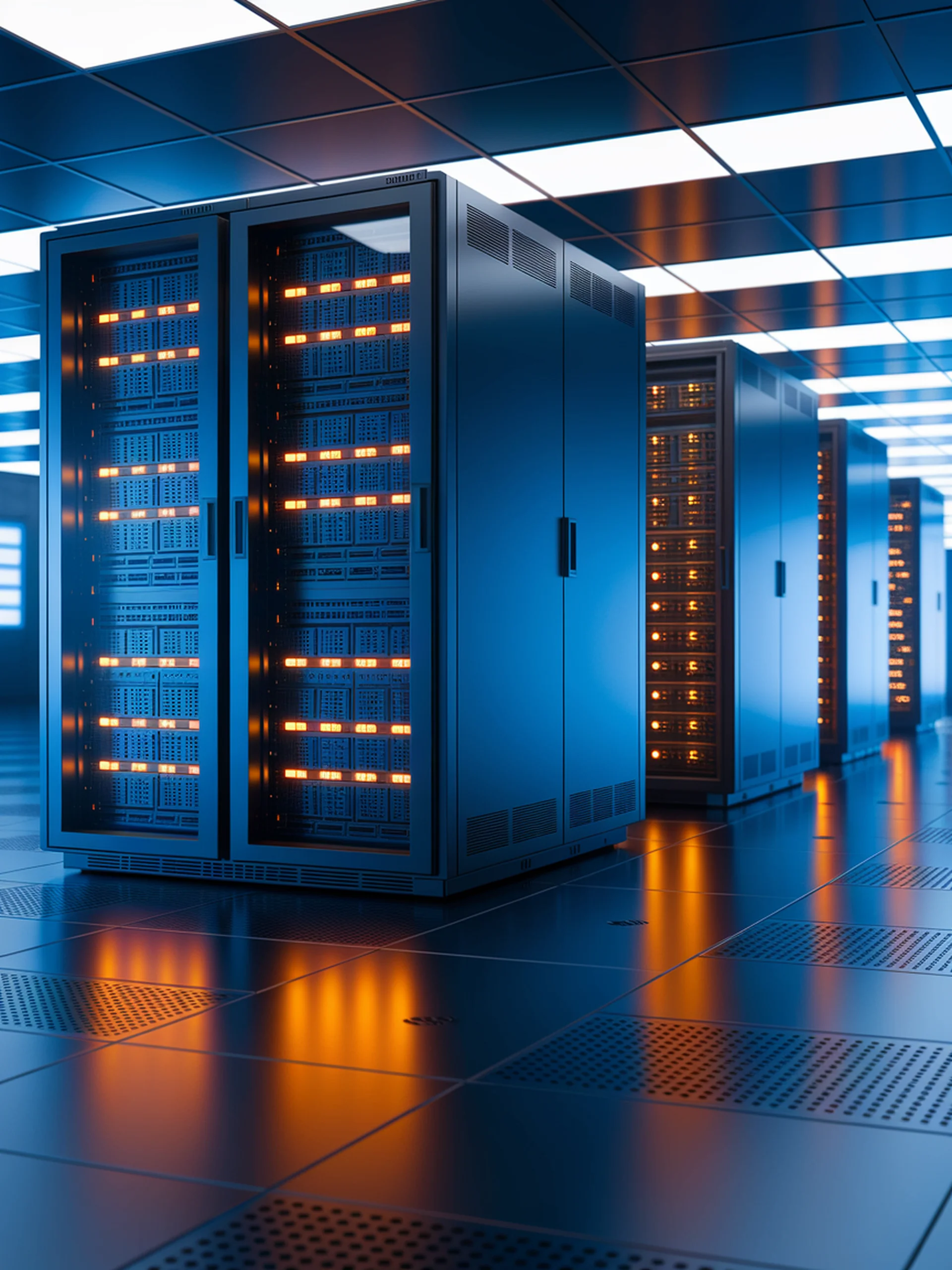
Memory-Efficient Expert Models
Lookup-based approach reduces VRAM requirements without sacrificing performance
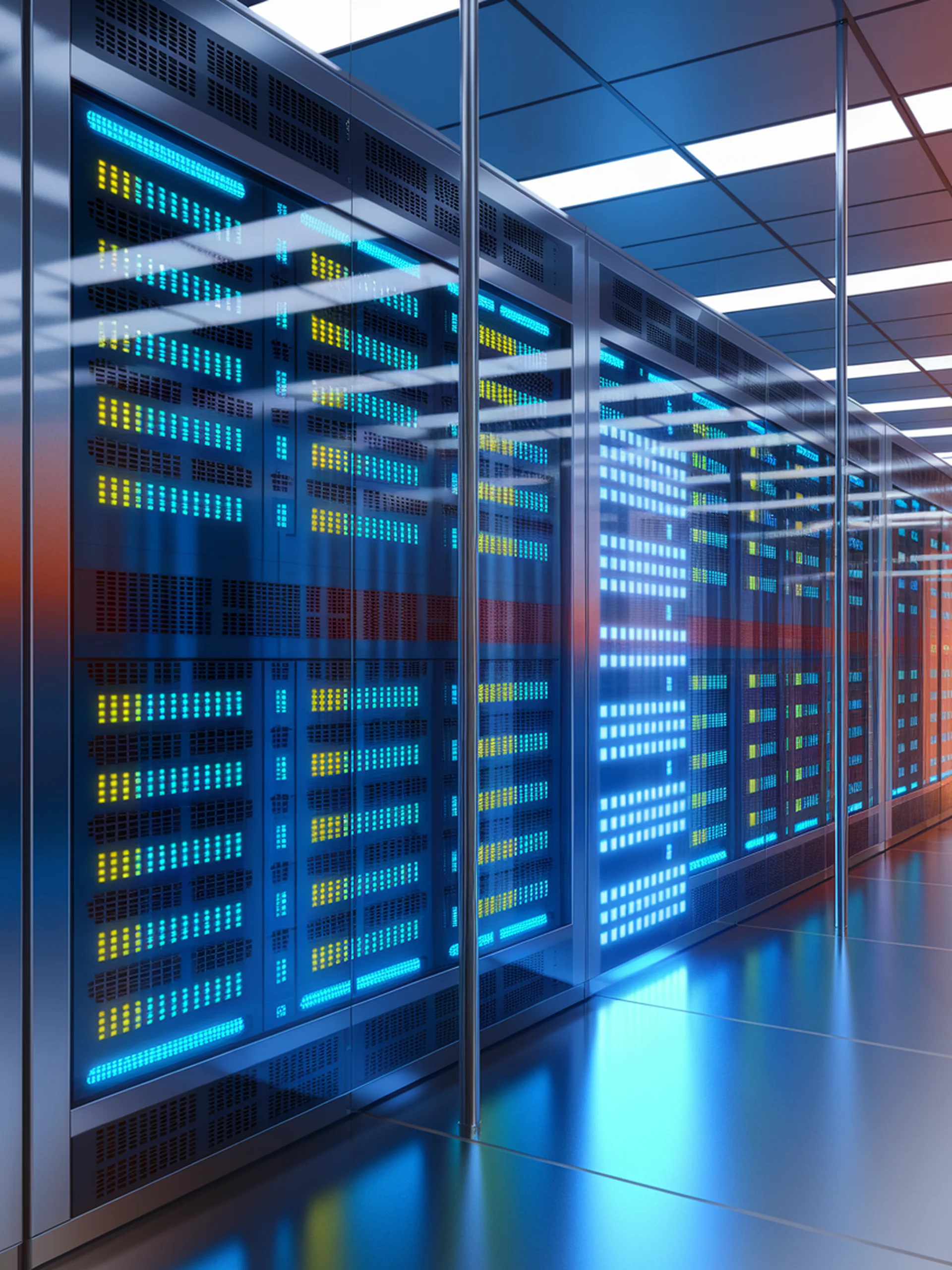
Accelerating LLM Inference with SPIN
Smart Speculative Decoding Using Heterogeneous Models

Breaking Memory Barriers in LLMs
Intelligent KV Cache Management for Efficient Long Sequence Processing
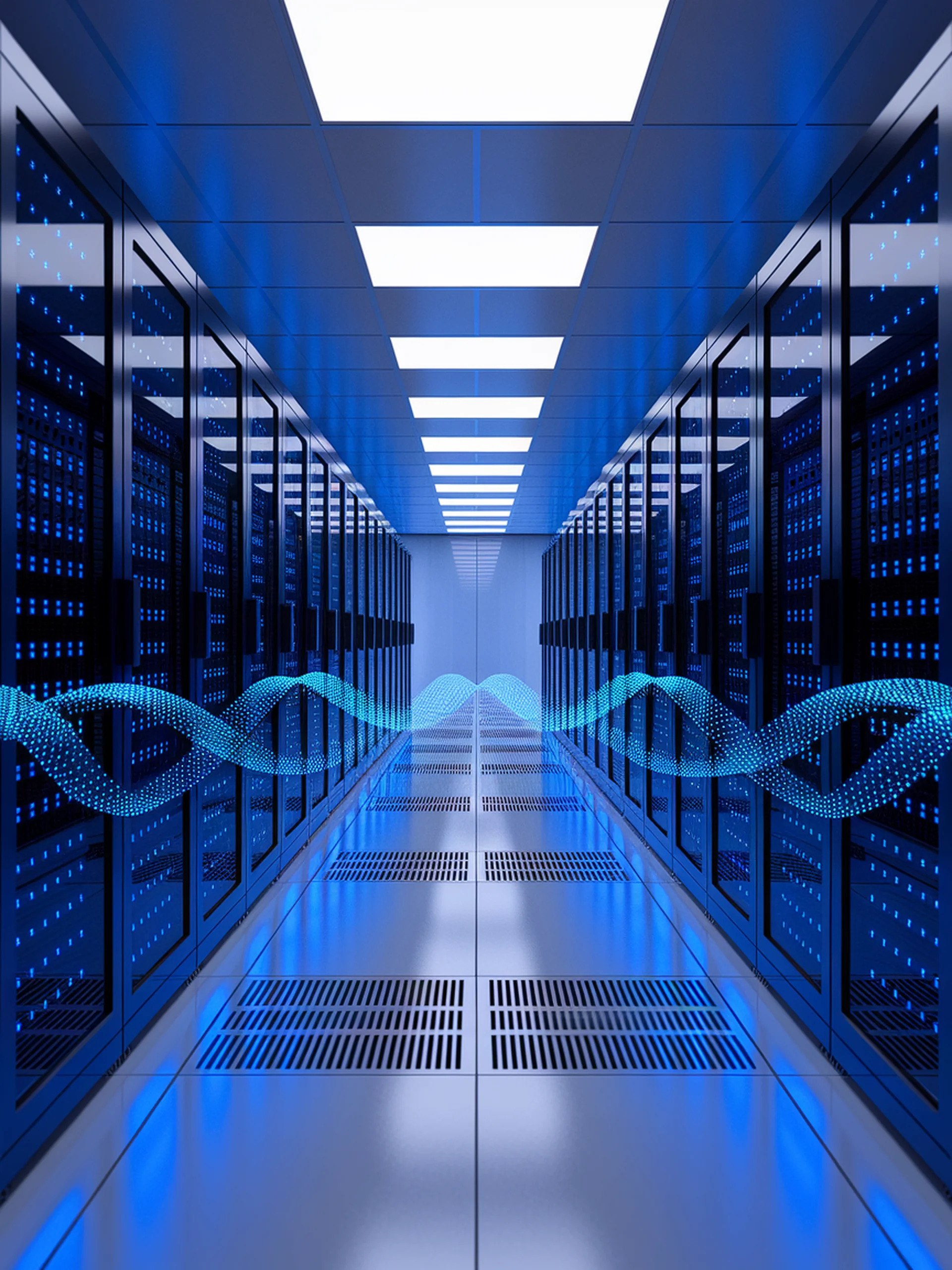
Smarter Cache Sharing for LLMs
Improving inference efficiency through semantic similarity

Turbocharging Large Language Models
Leveraging 2:4 activation sparsity to accelerate LLM performance
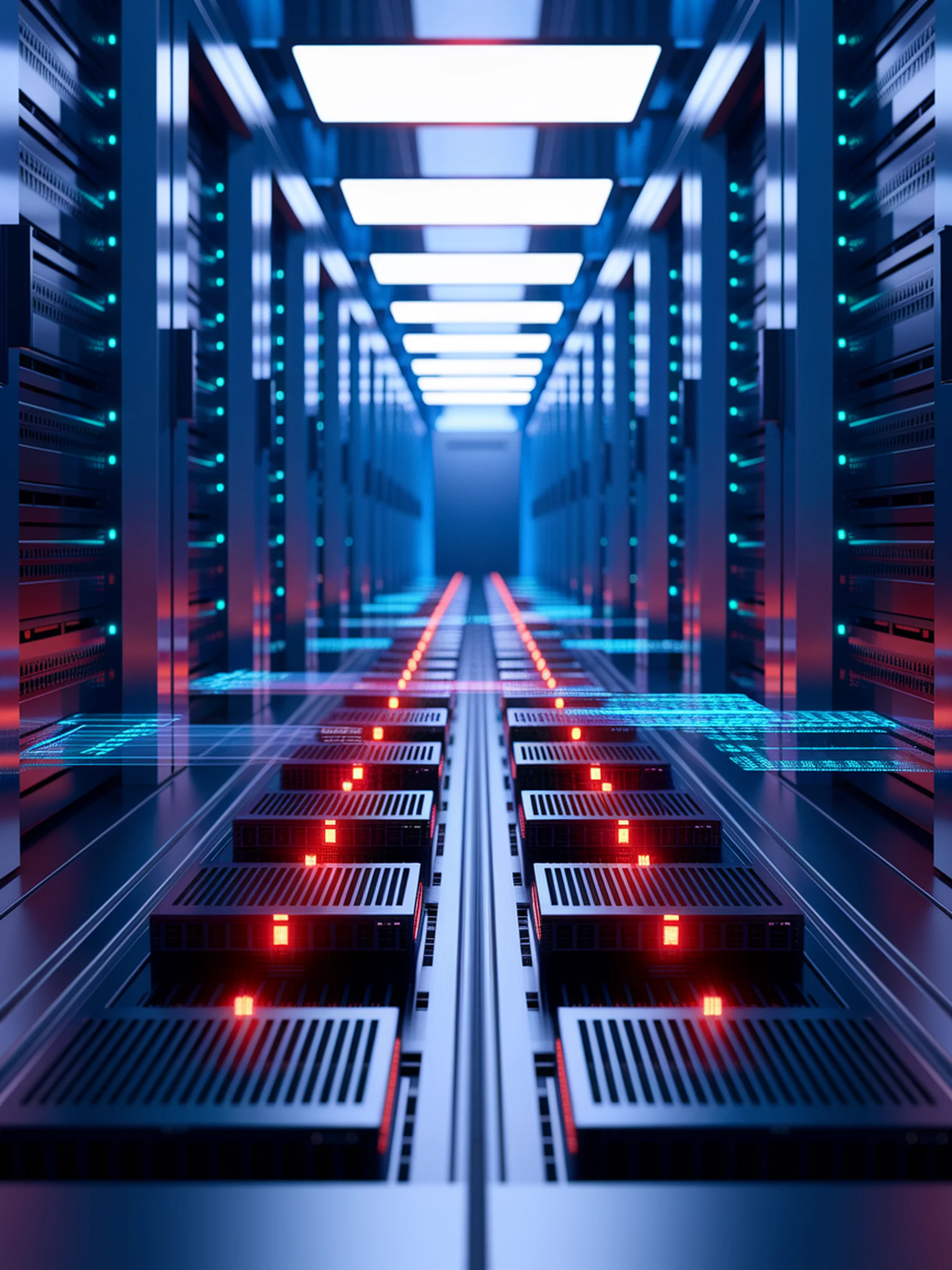
Optimizing Multi-LLM Workflows
Enhancing Efficiency for Complex AI Systems
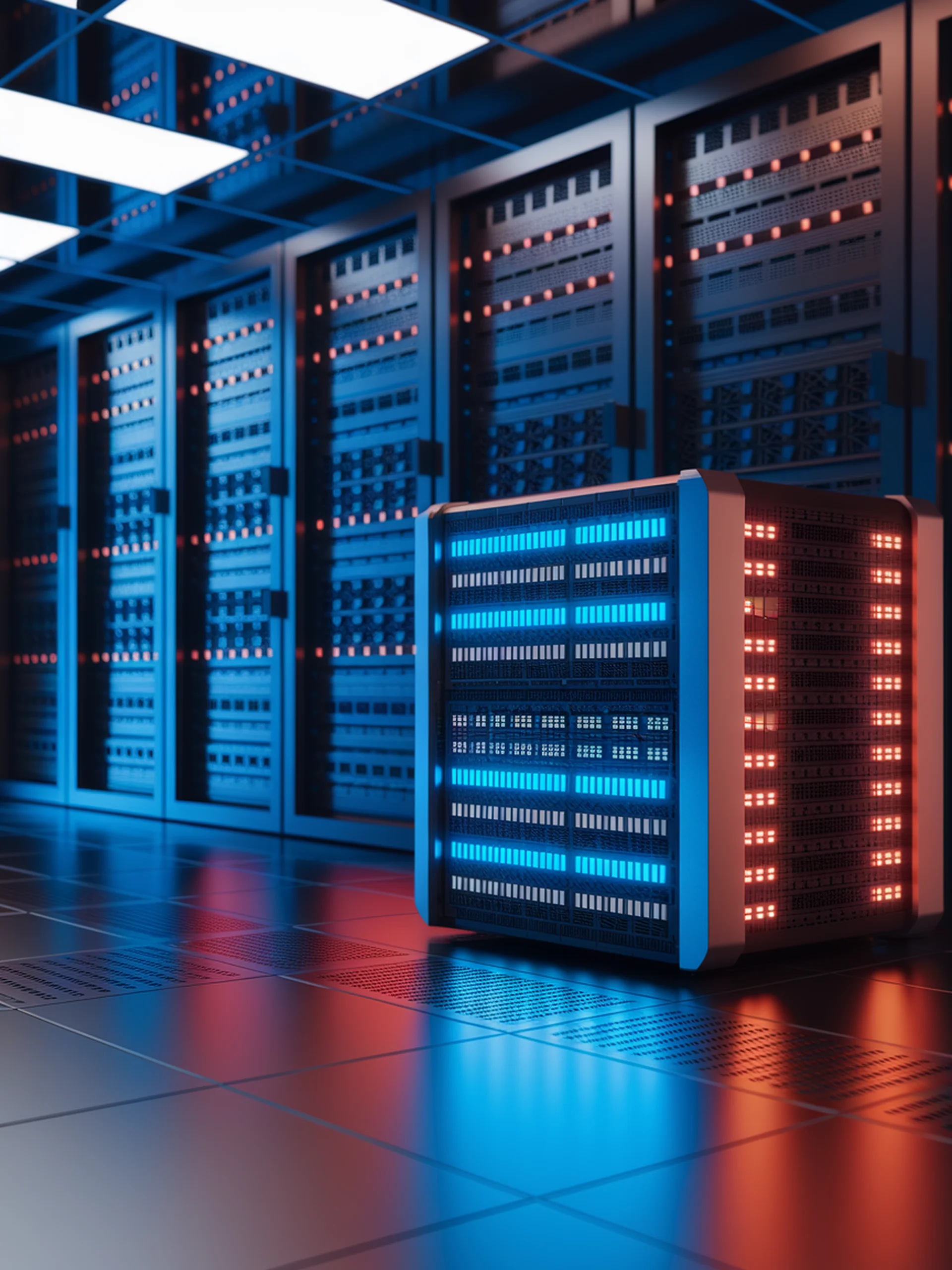
Smarter LLM Compression
Nested Activation-Aware Decomposition for Efficient AI Deployment
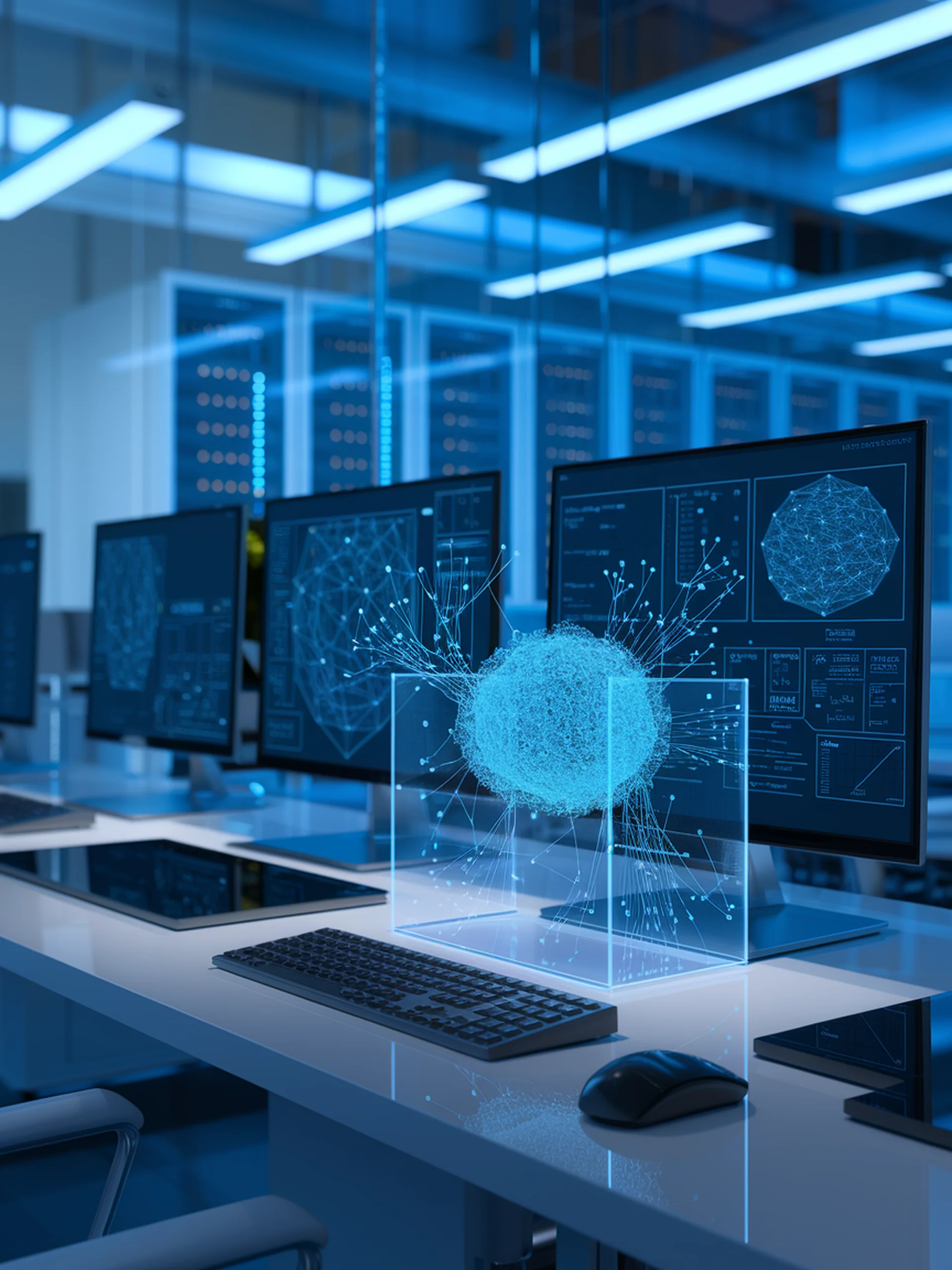
Expanding to Shrink: A New Approach to Model Efficiency
How post-training expansion can improve quantized LLMs
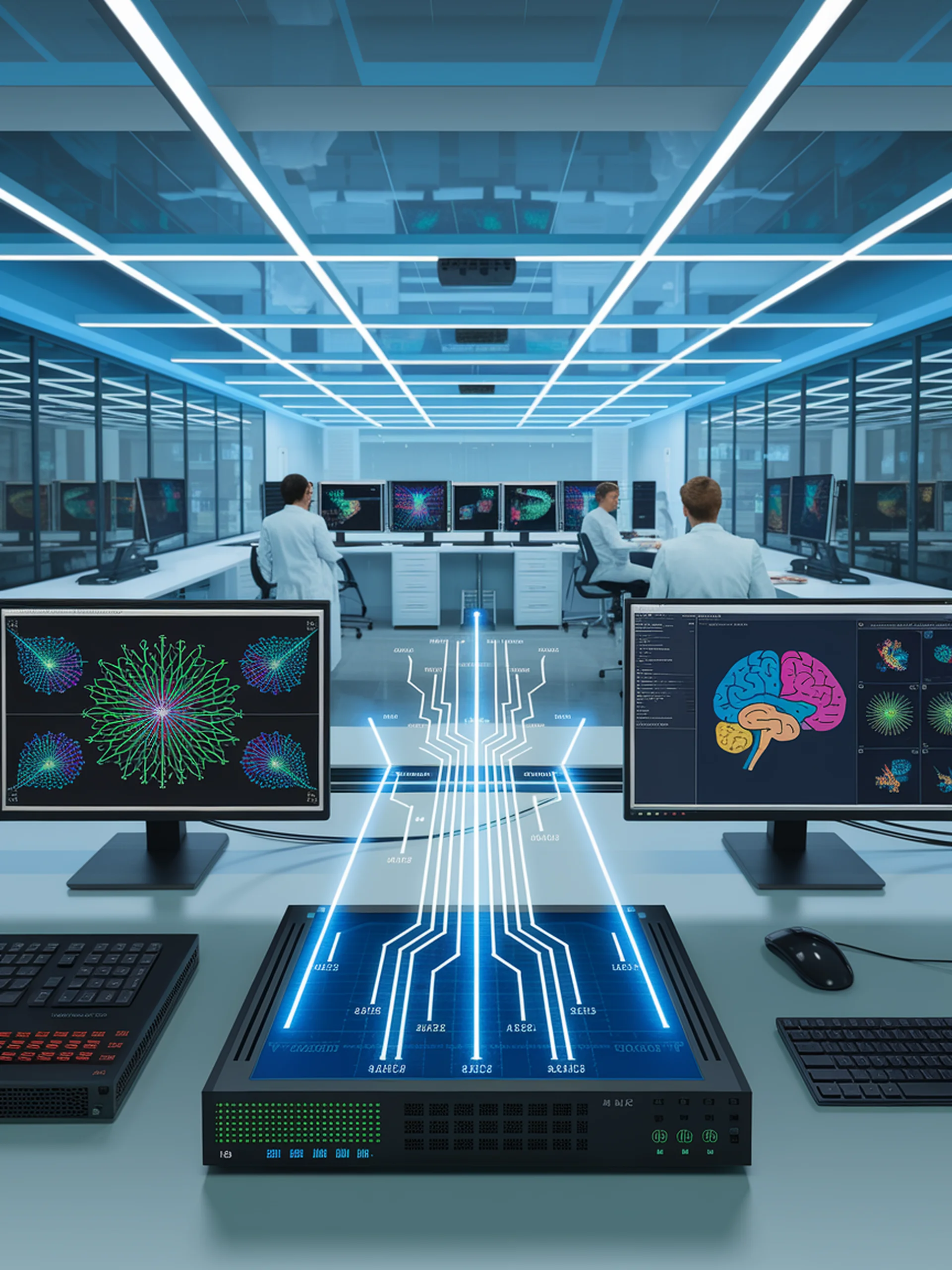
Efficient Reasoning in Language Models
Testing LLM reasoning paths with fewer computational resources
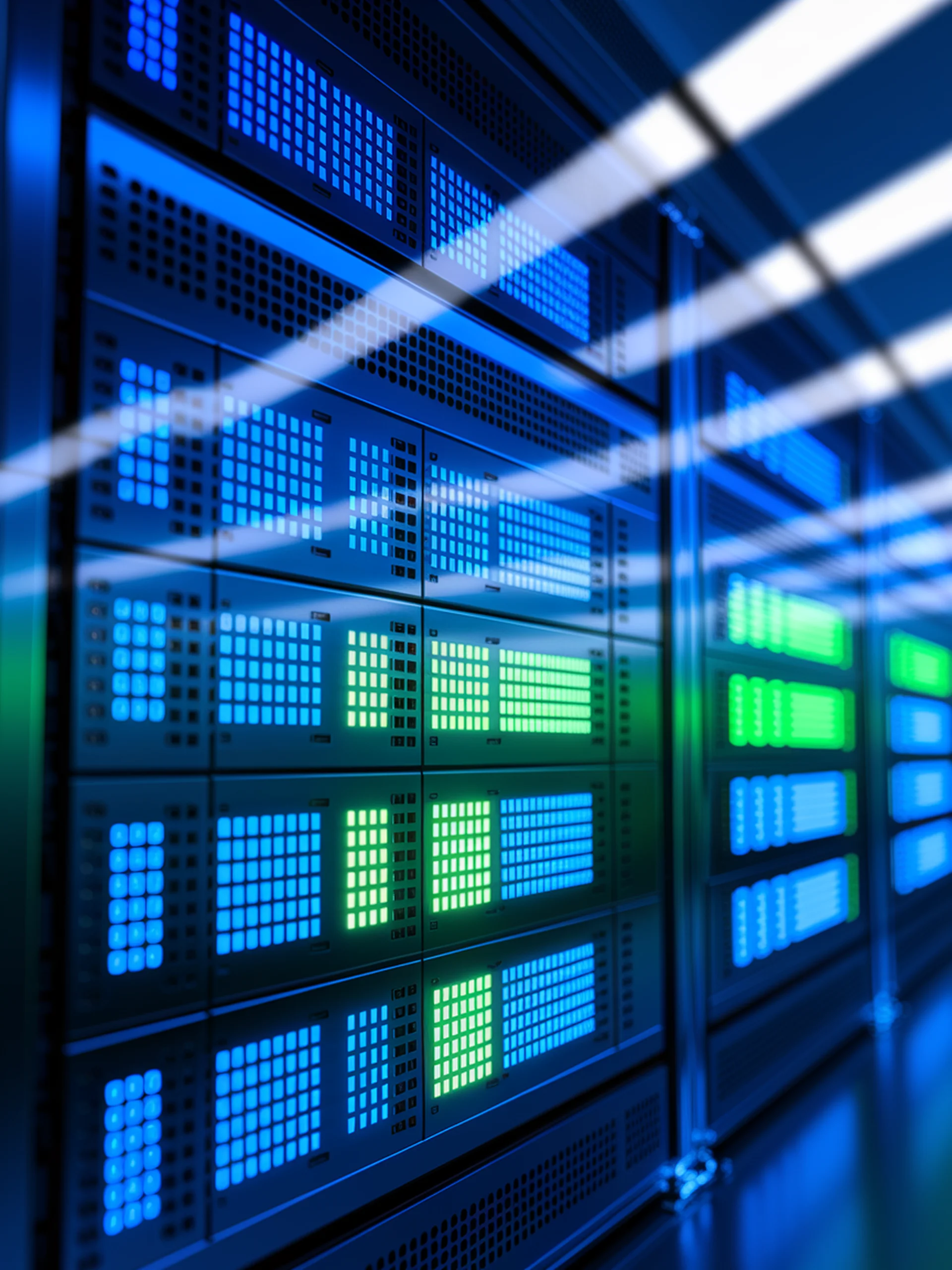
Smarter KV Cache Management for LLMs
Task-adaptive window selection for efficient inference
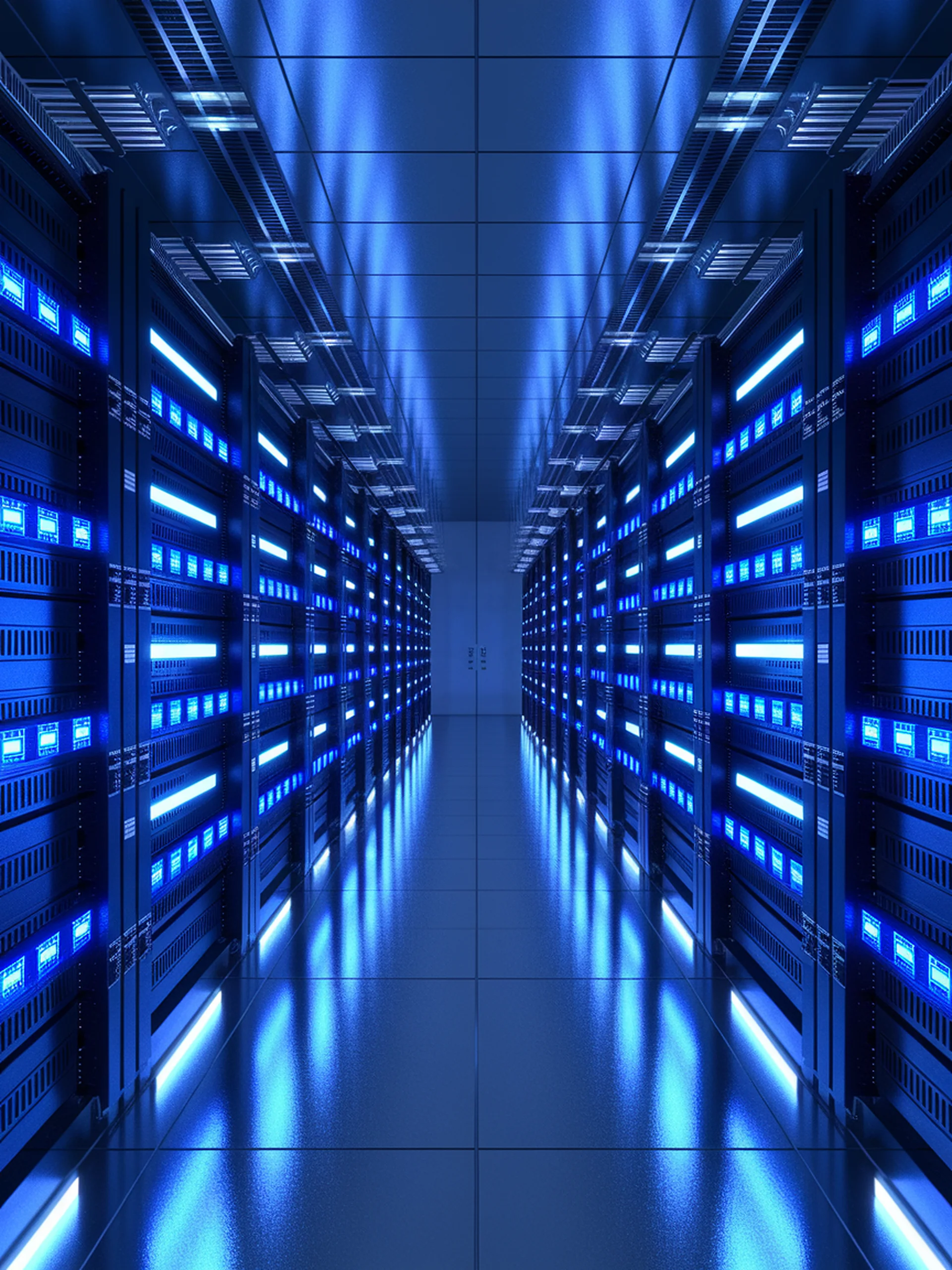
Optimizing LLM Training Efficiency
Introducing Workload-Balanced 4D Parallelism
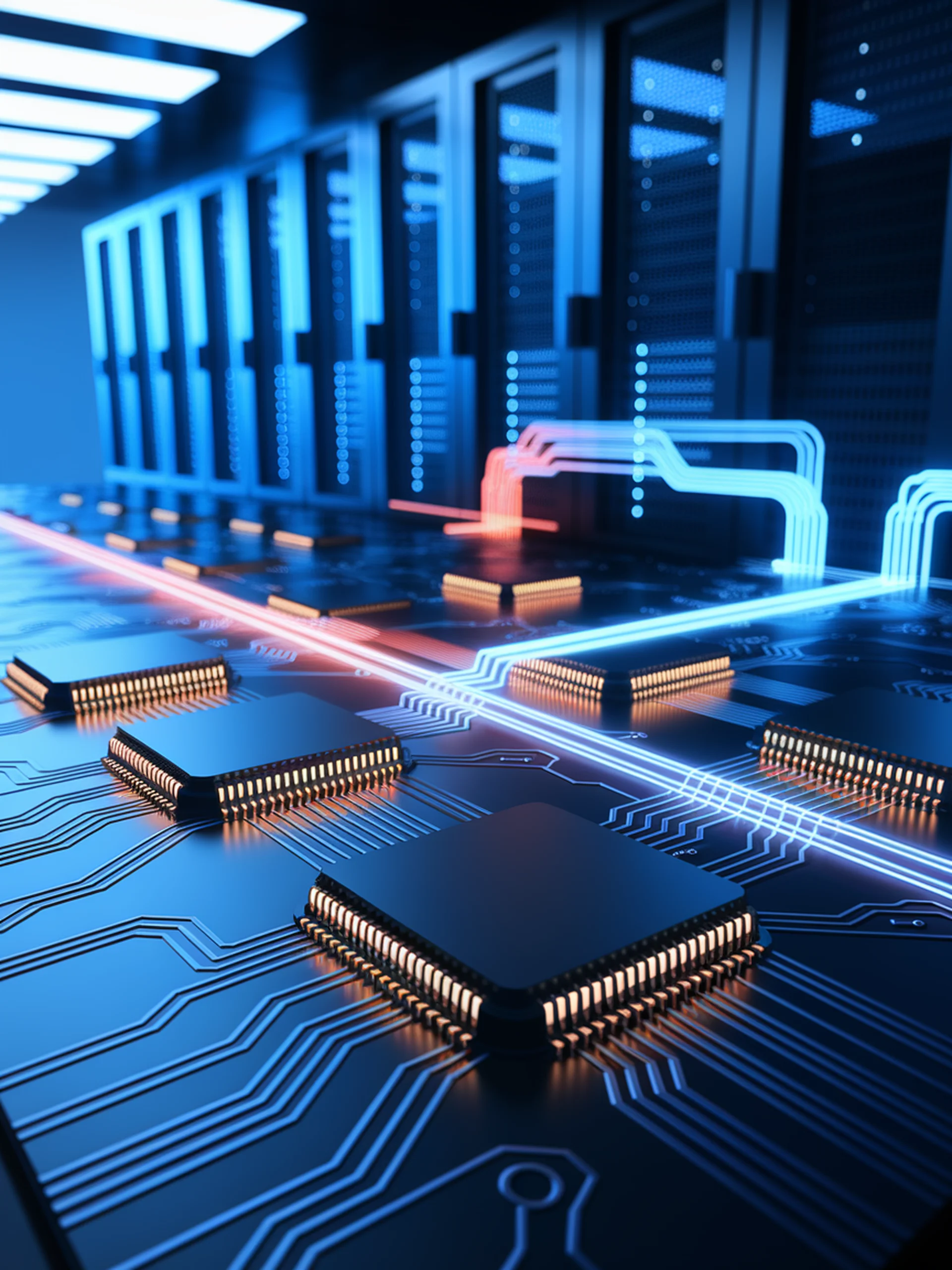
Speeding Up LLMs with RaNA
A breakthrough in transformer efficiency through adaptive rank allocation
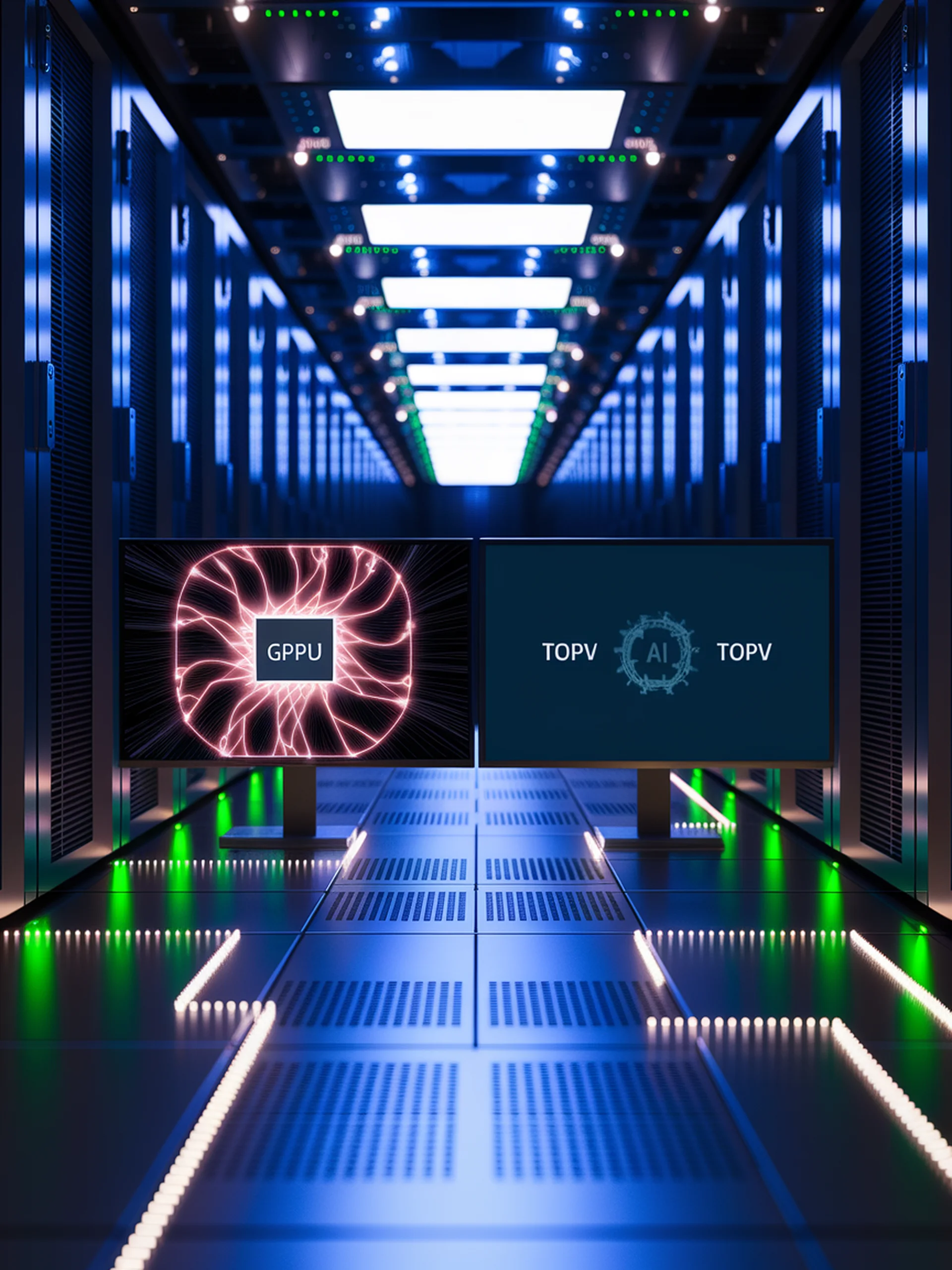
Smarter Vision AI with TopV
Accelerating multimodal models through intelligent token pruning
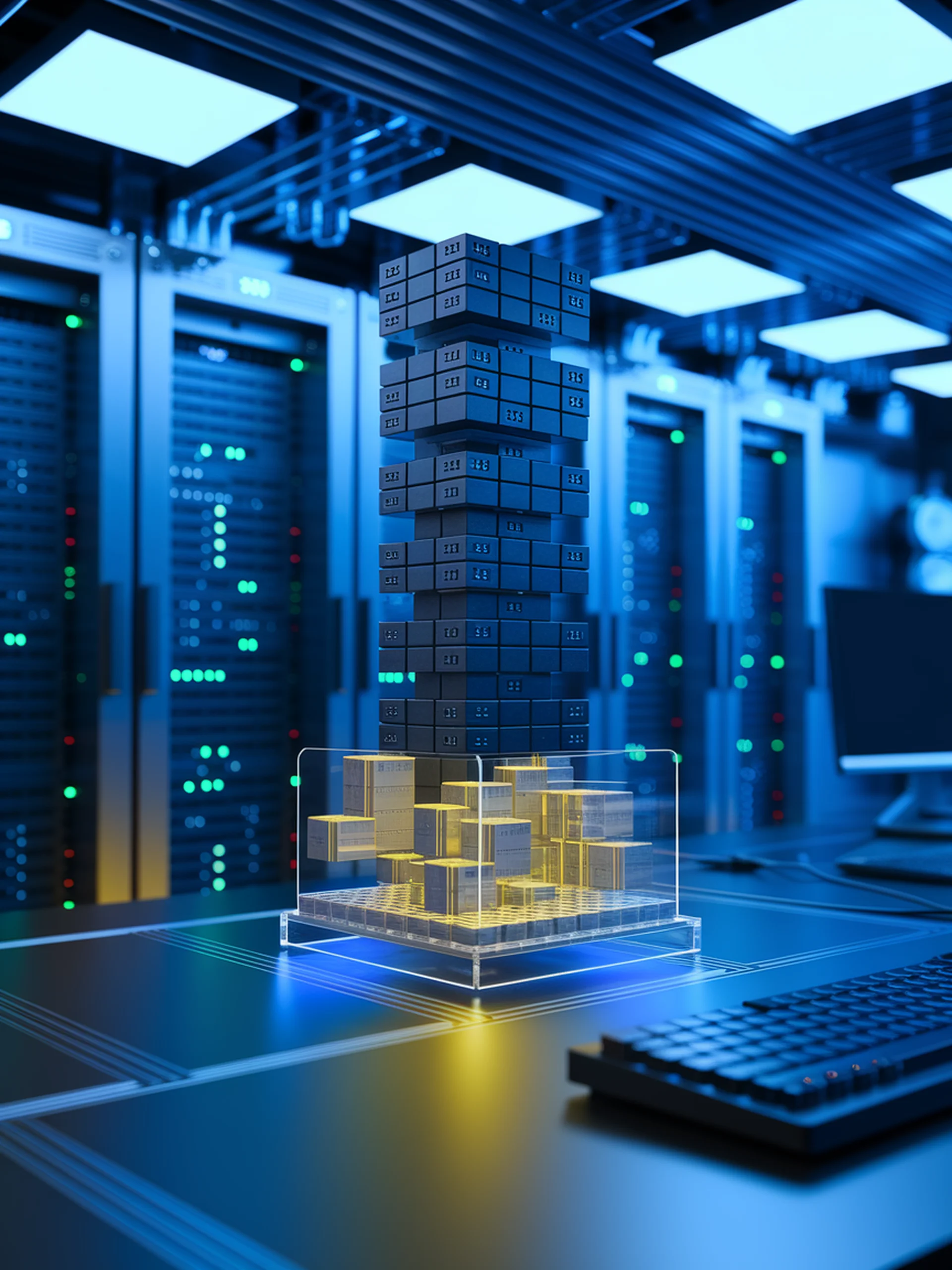
Optimizing LLM Memory: The Jenga Approach
Smart memory management for heterogeneous LLM architectures
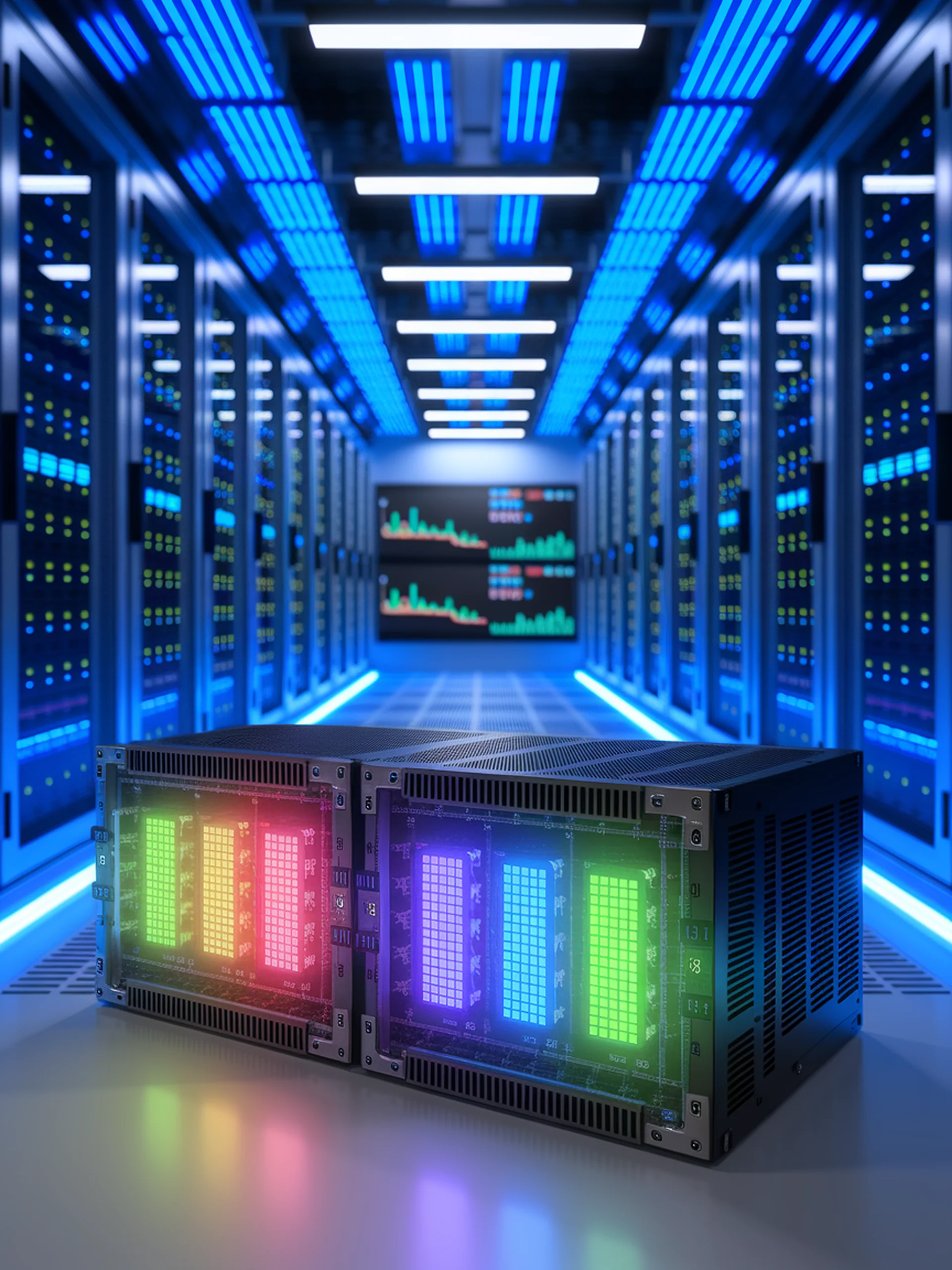
Accelerating LLM Serving with Smart Memory Management
Hybrid KV Cache Quantization for Faster, More Efficient LLM Deployment
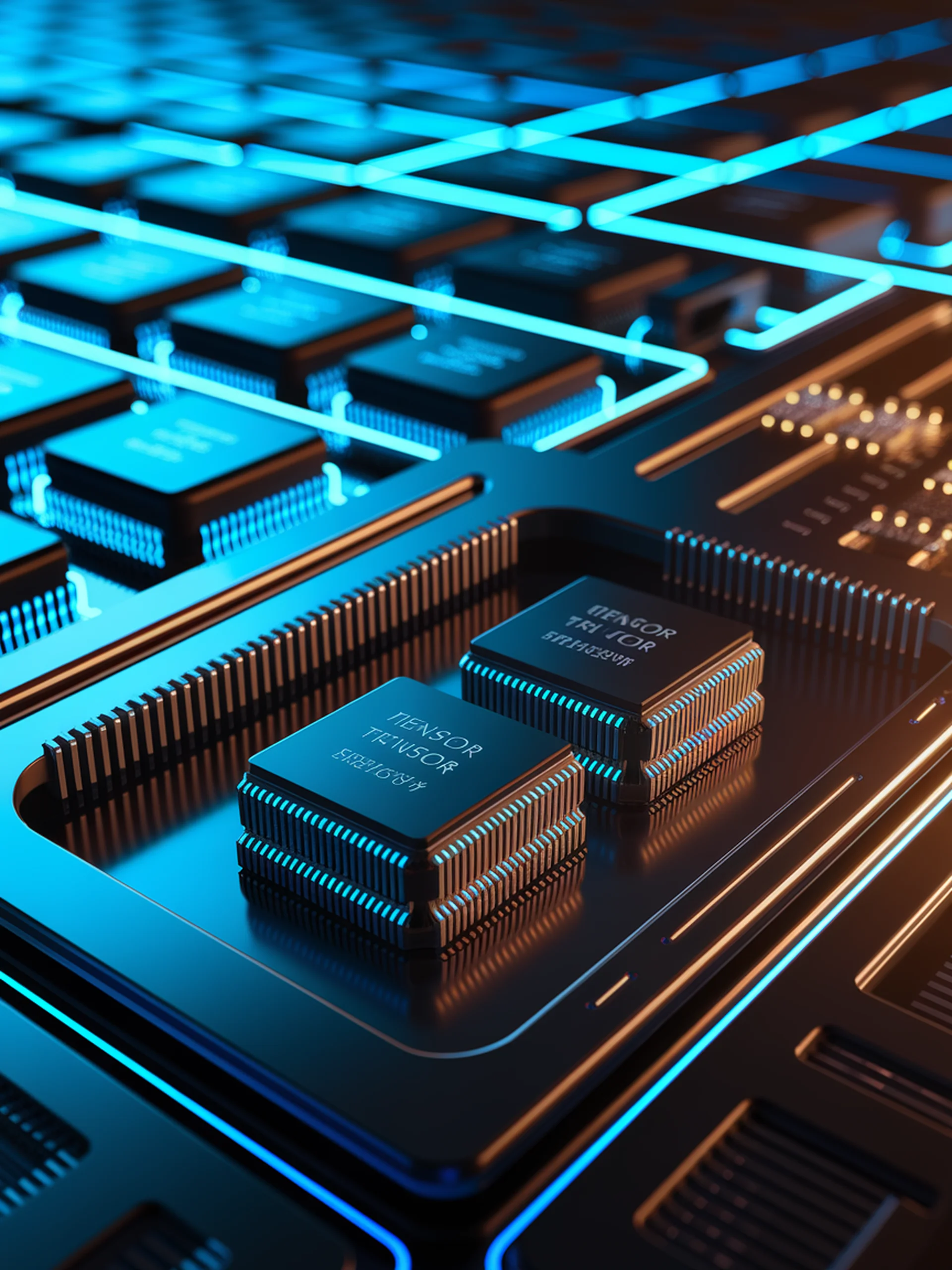
Accelerating LLM Inference with BitDecoding
Using tensor cores for efficient low-bit KV cache processing
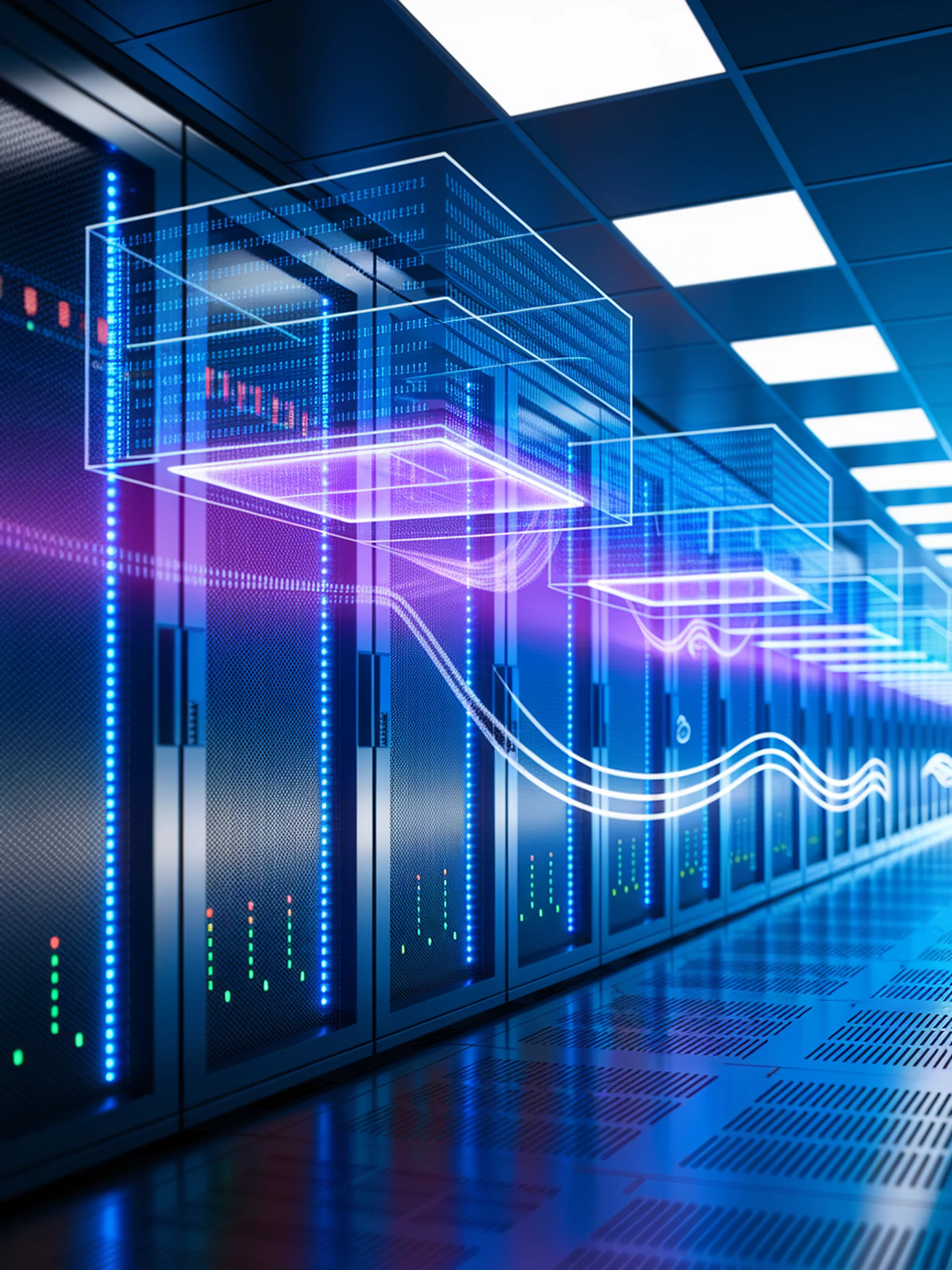
Smarter KV-Cache Compression
Reducing LLM Memory Footprint with Cross-Layer SVD

Speeding Up AI: The FFN Fusion Breakthrough
Reducing Sequential Computation in Large Language Models
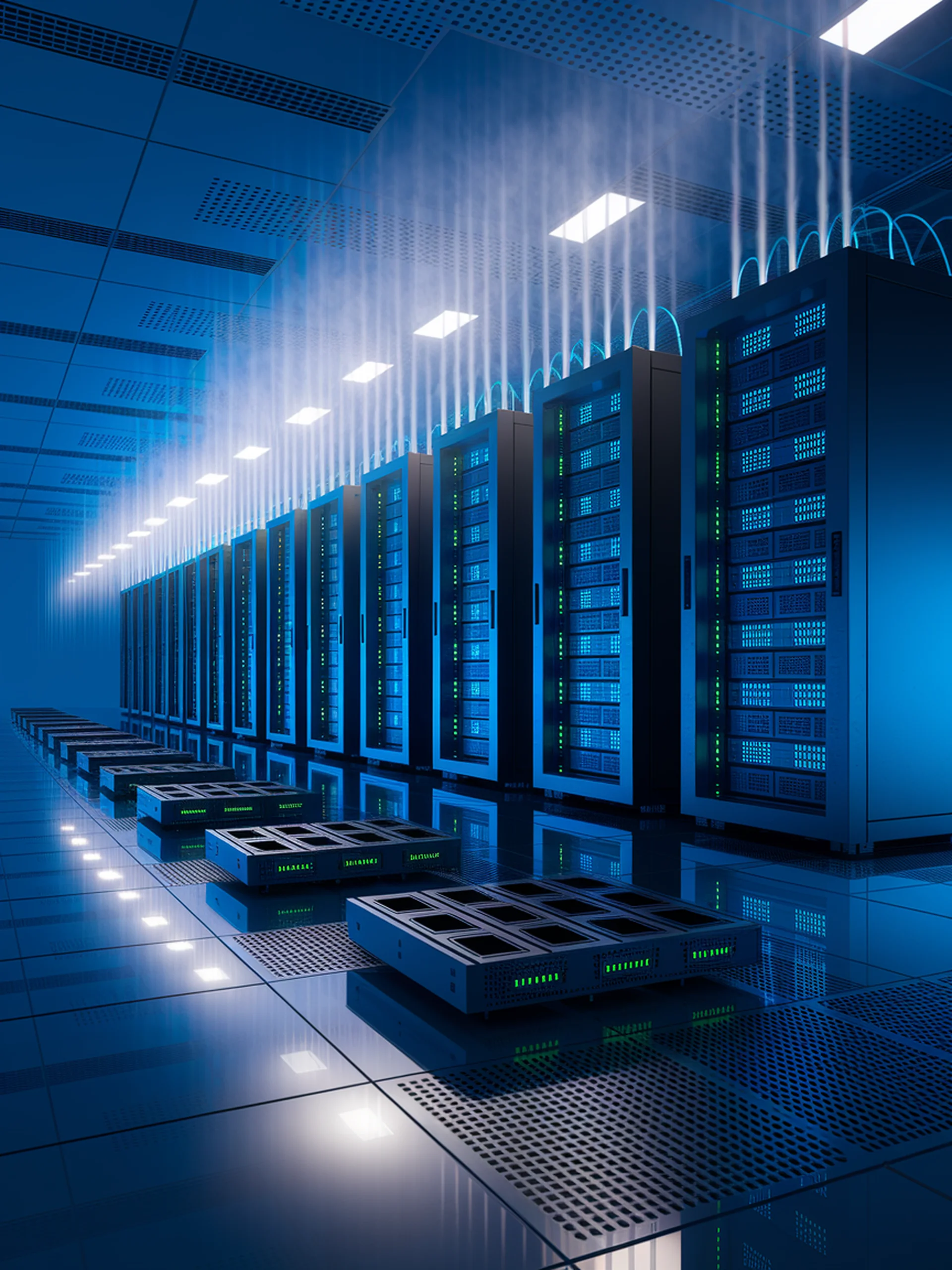
Optimizing LLM Training Efficiency
Memory and Parallelism Co-optimization for Faster LLM Training
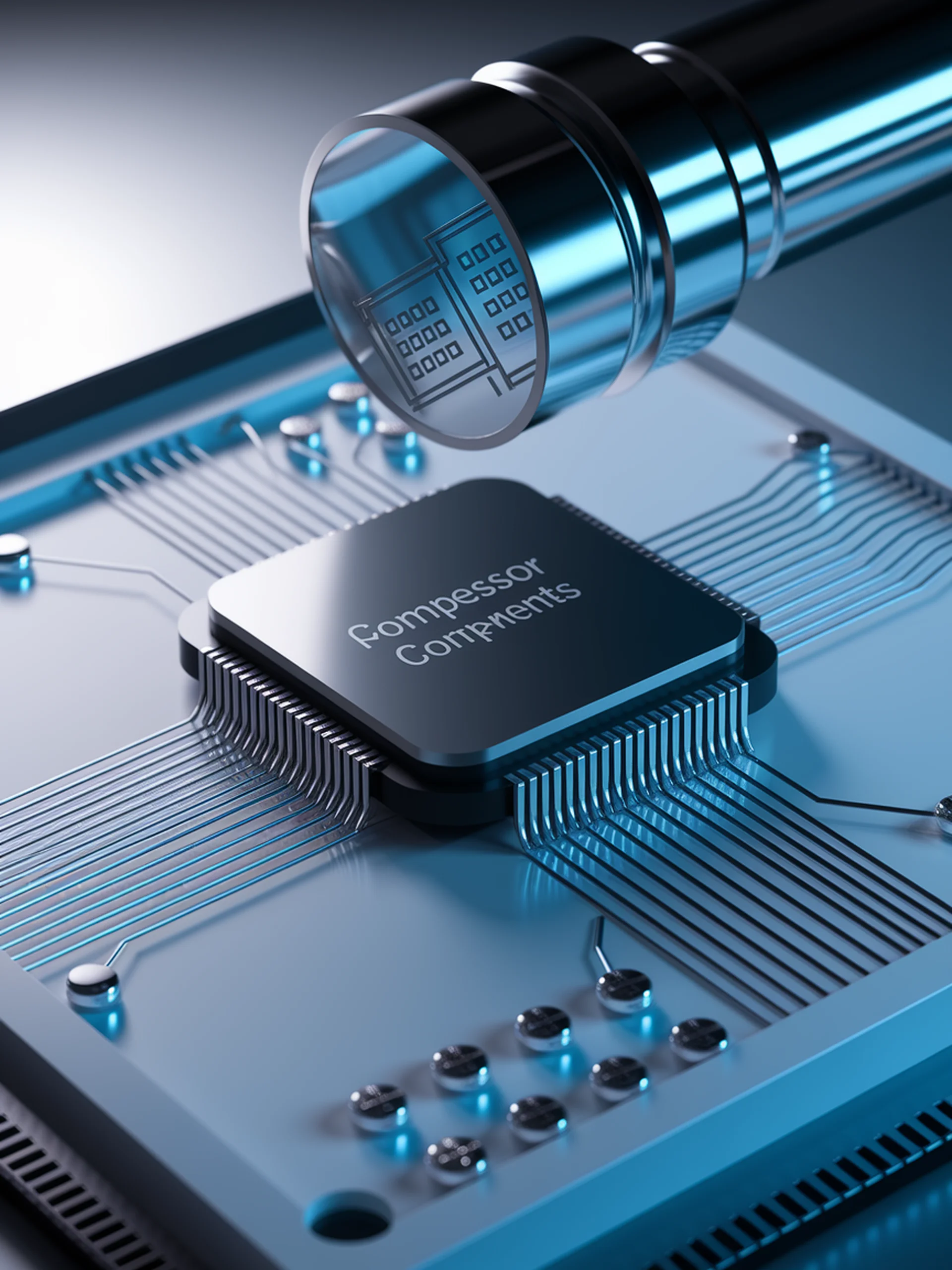
Shrinking LLMs Without Losing Power
Breakthrough in 4-bit quantization through activation decomposition
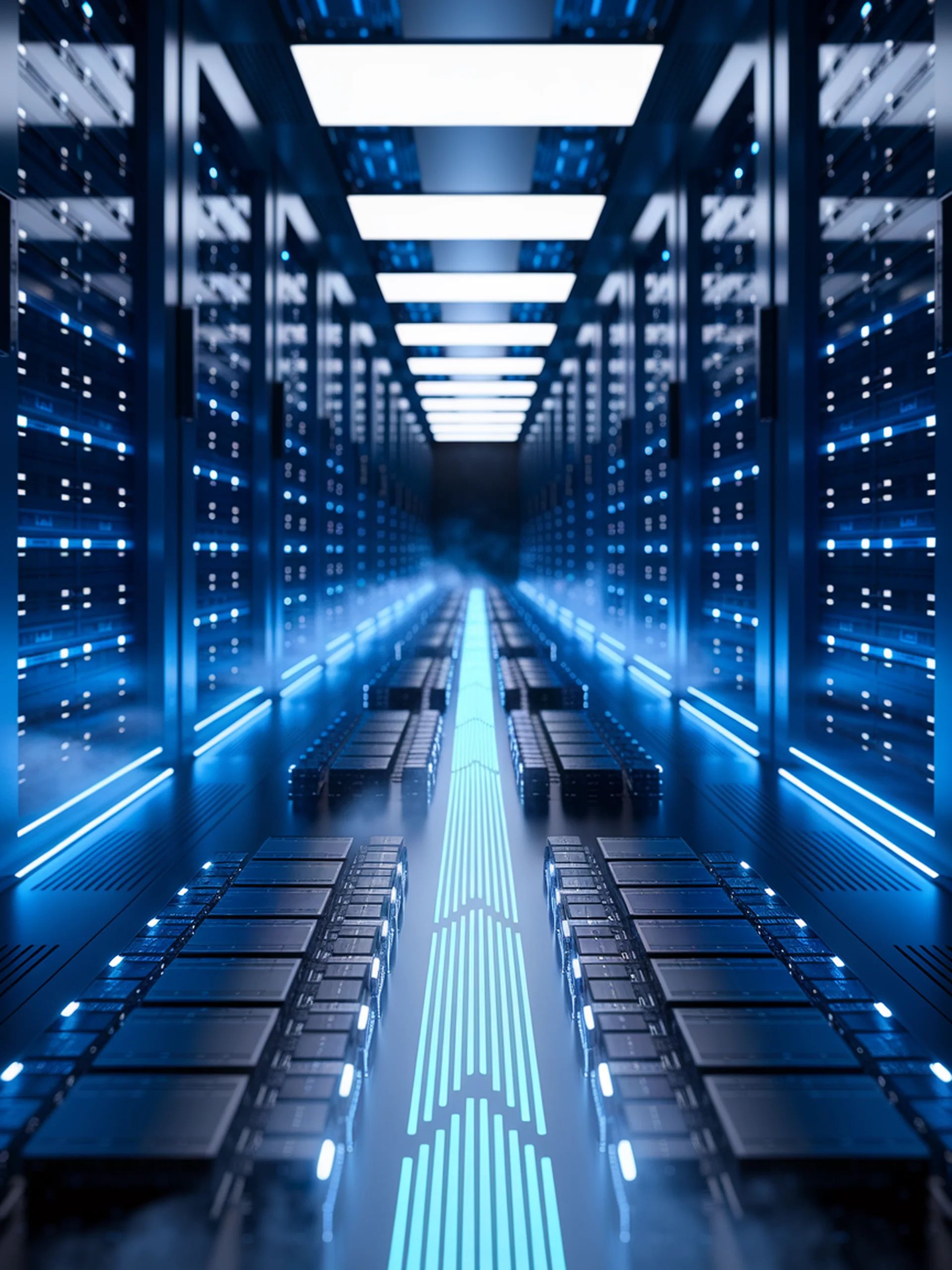
LogQuant: Transforming LLM Memory Efficiency
2-Bit Quantization for KV Cache with Minimal Performance Loss
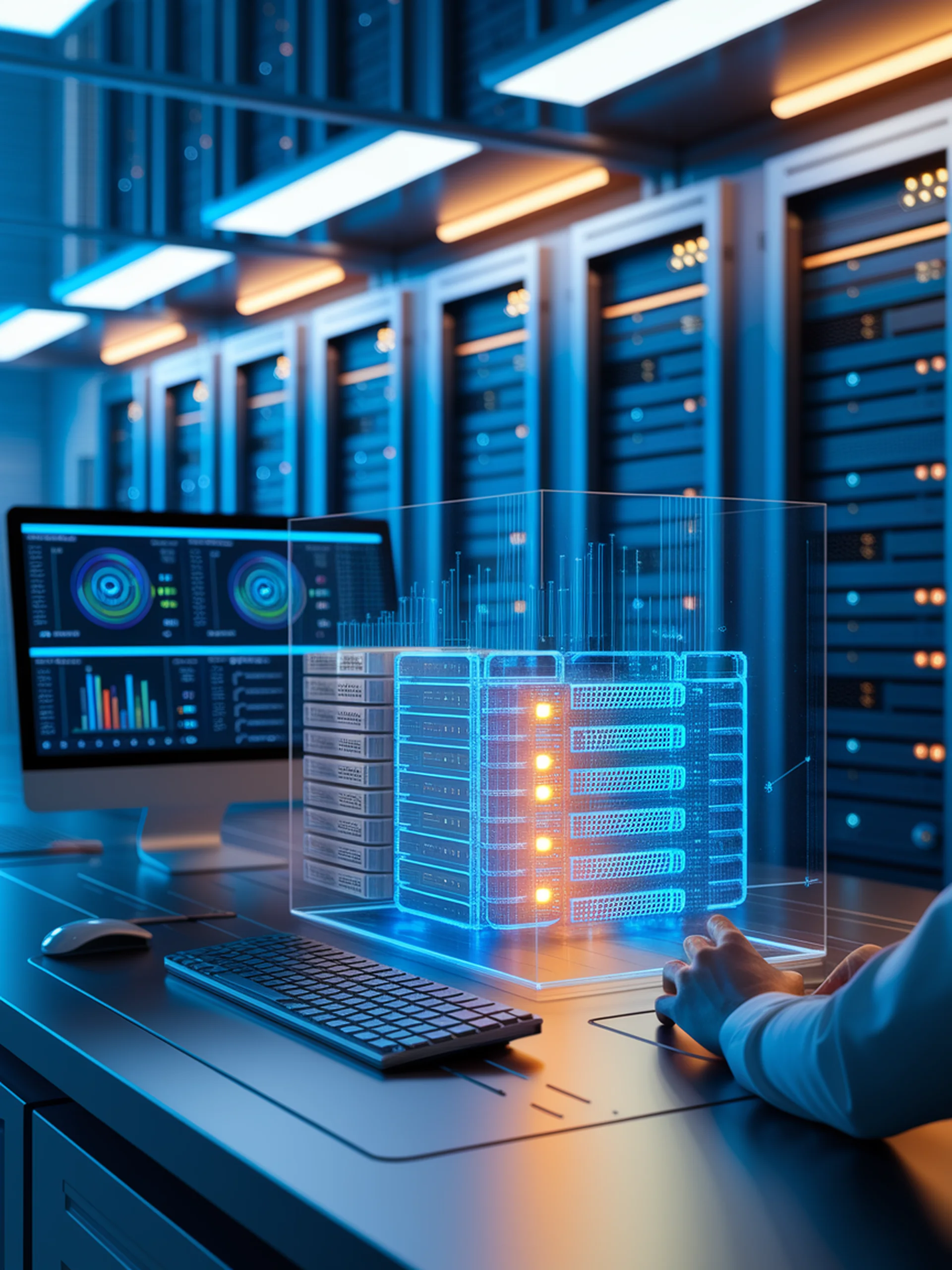
Optimizing AI Training with Virtual Accelerators
Reducing costs and improving efficiency through emulation
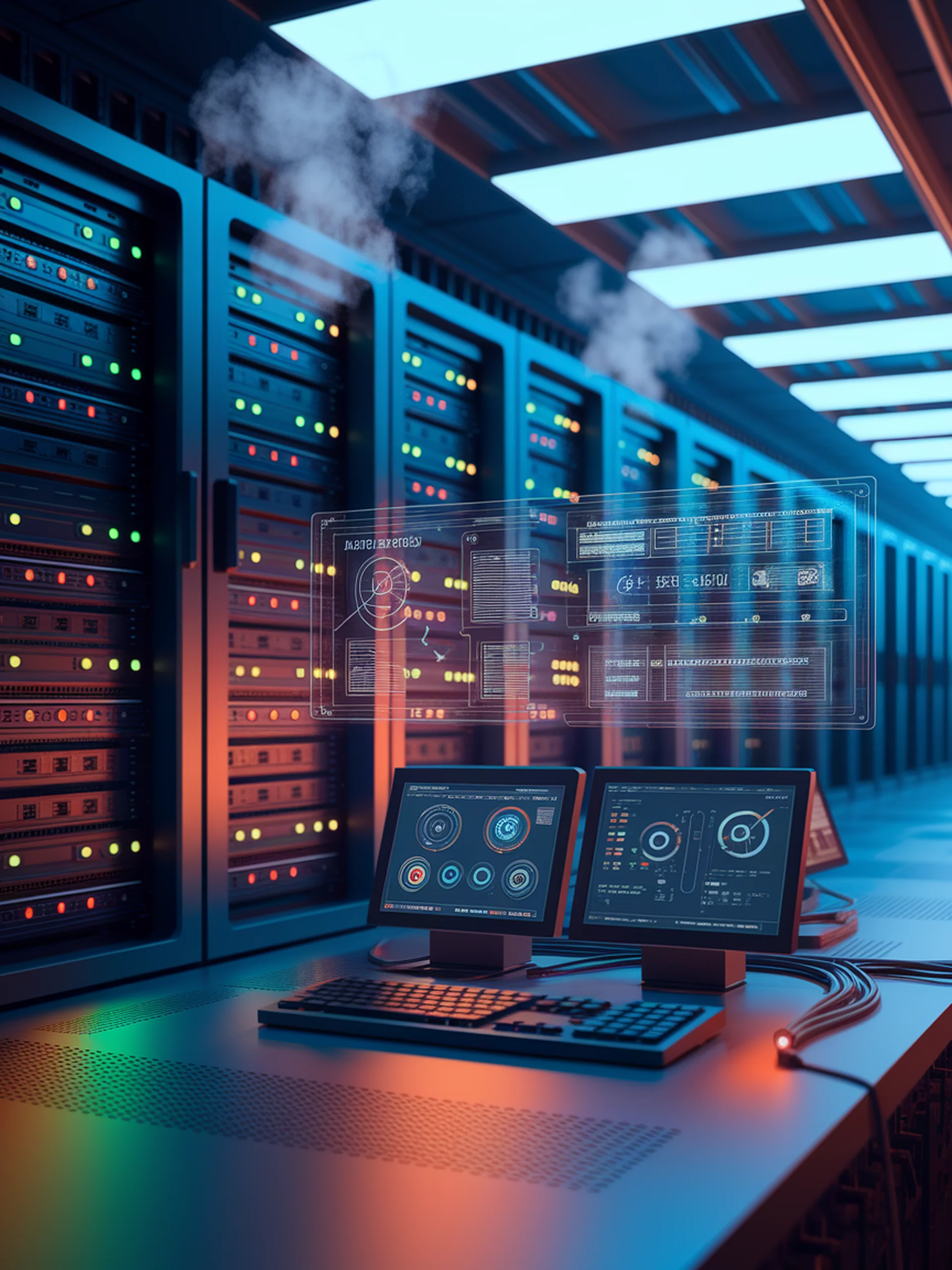
Automating LLM Training Failure Diagnosis
Reducing costly training failures through intelligent log analysis
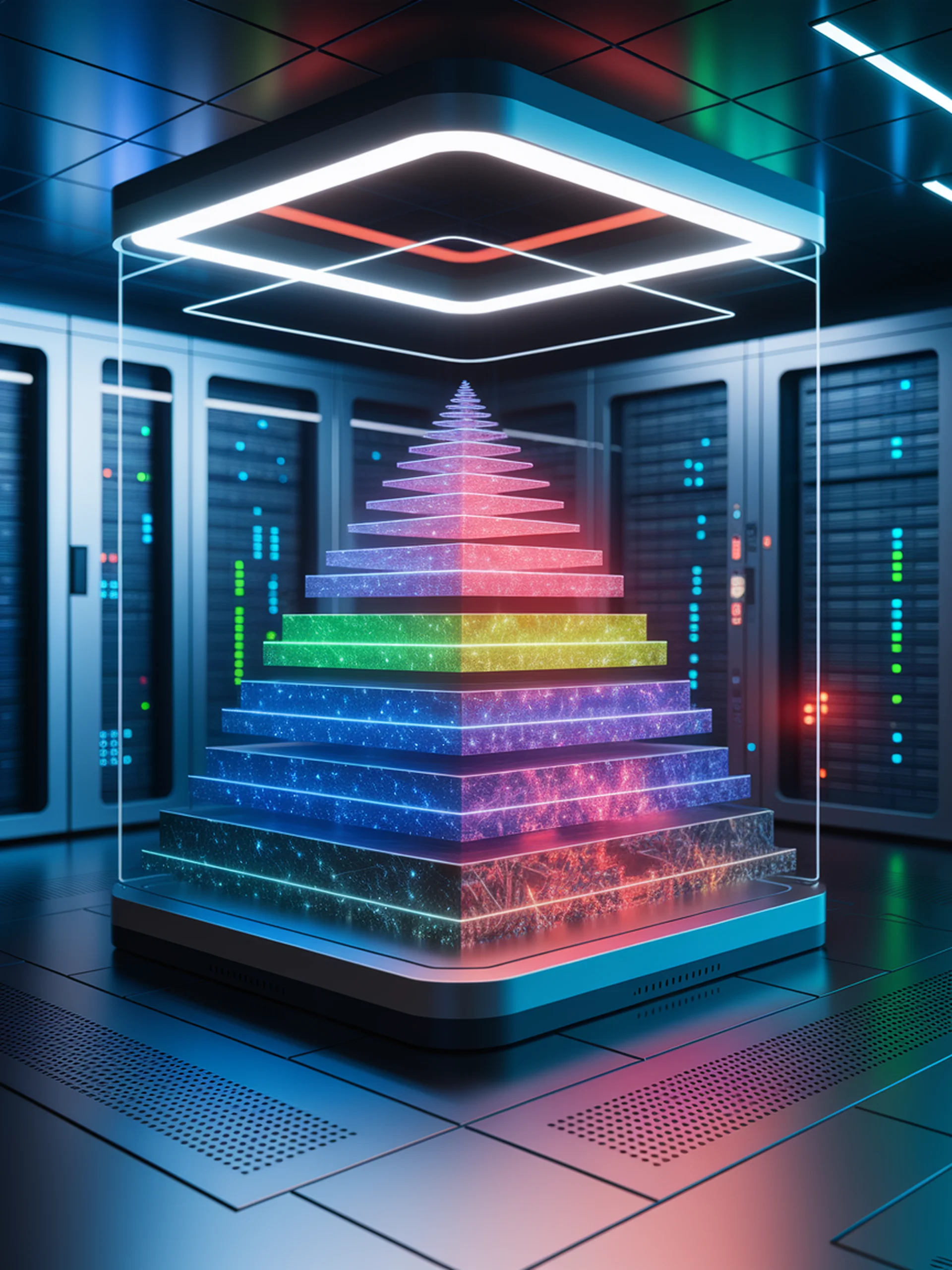
Smart Visual Compression for AI Models
Improving efficiency without sacrificing performance in multimodal systems
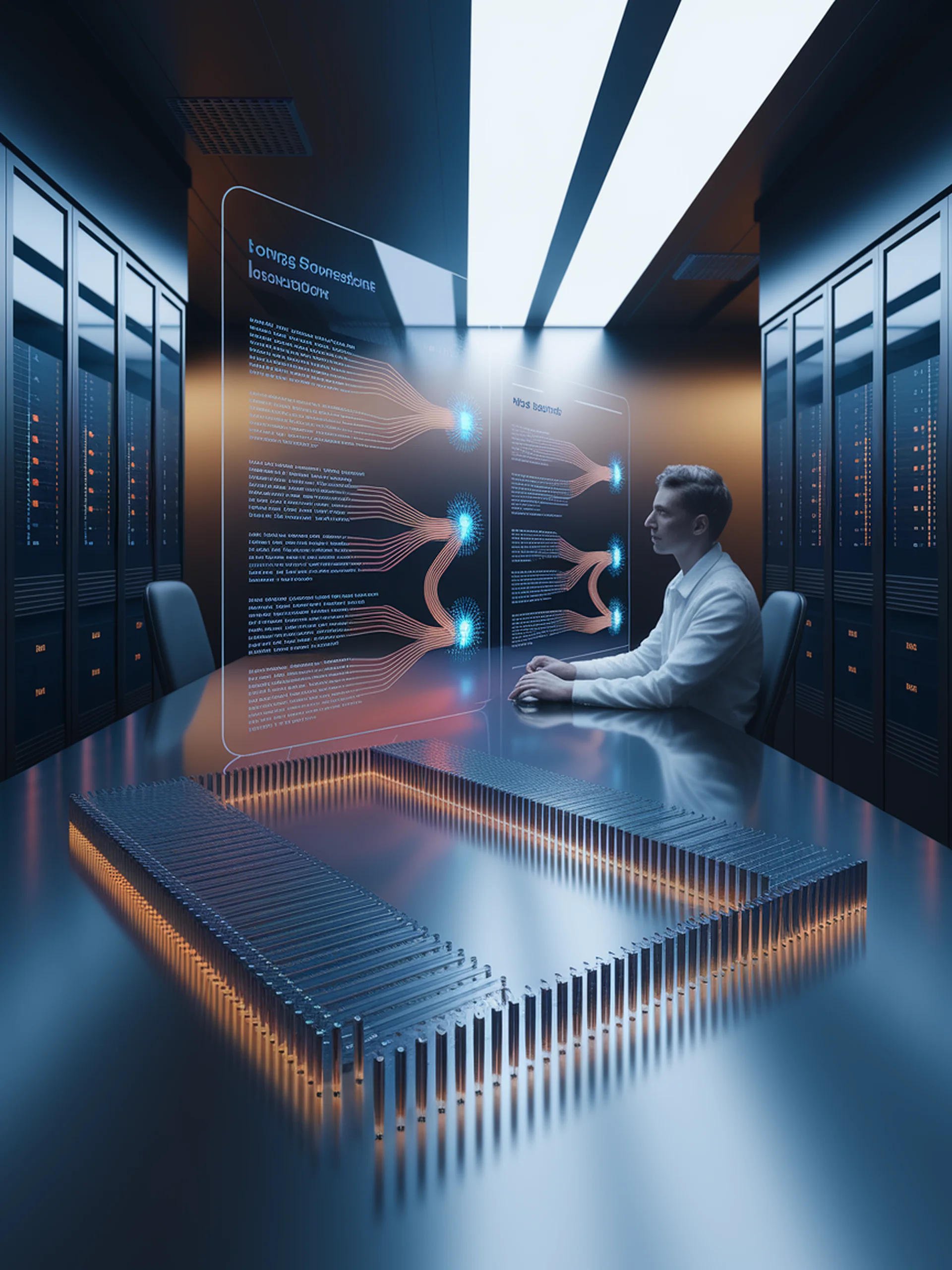
Breaking Long Context Barriers
A New Embedding Model for Enhanced RAG Systems
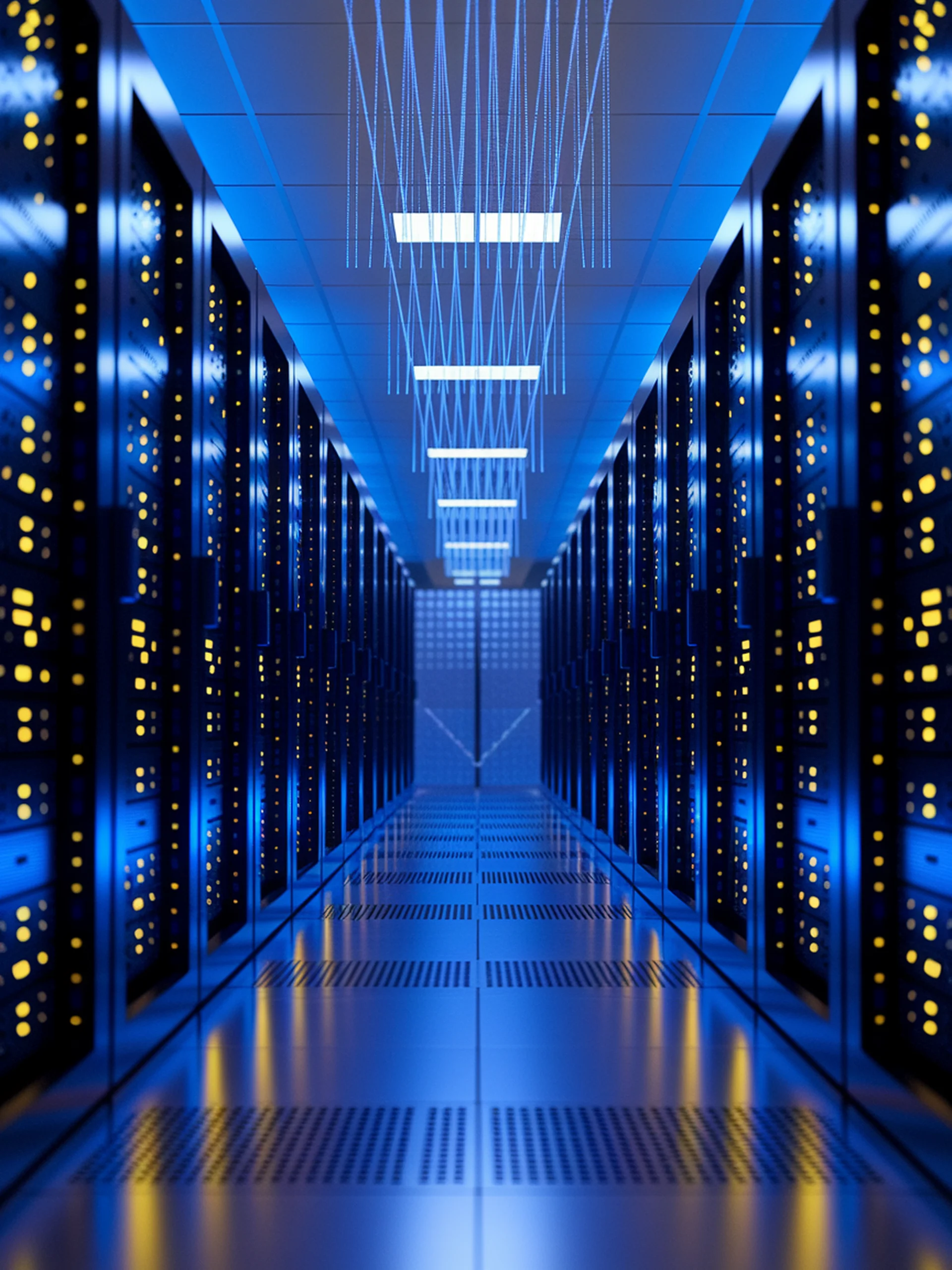
Boosting LLM Performance with Attention Disaggregation
A novel approach to optimize resource utilization in LLM serving systems
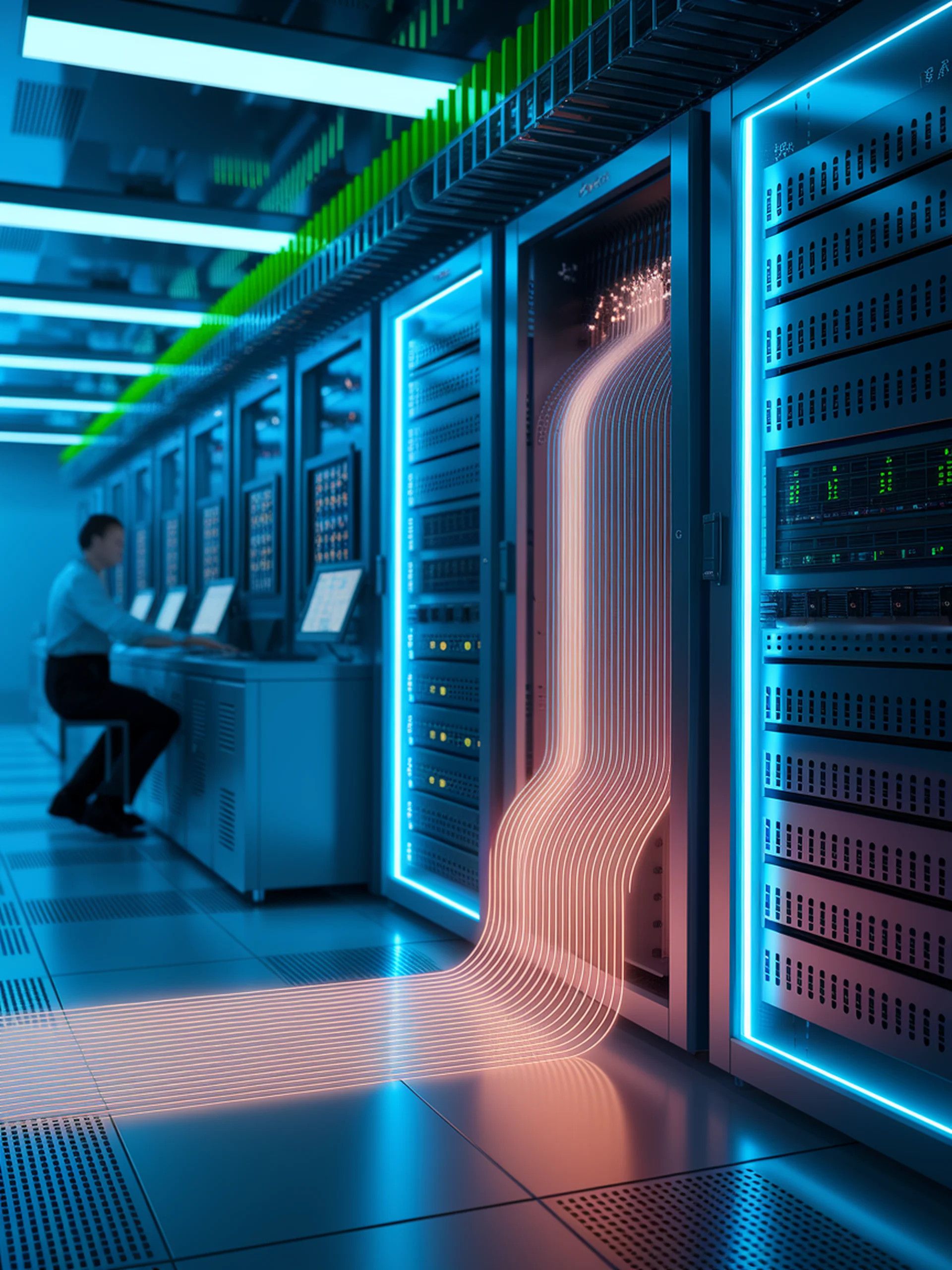
Smart Compression for Next-Gen AI Models
Enhancing MoE Model Efficiency Through Multi-stage Quantization
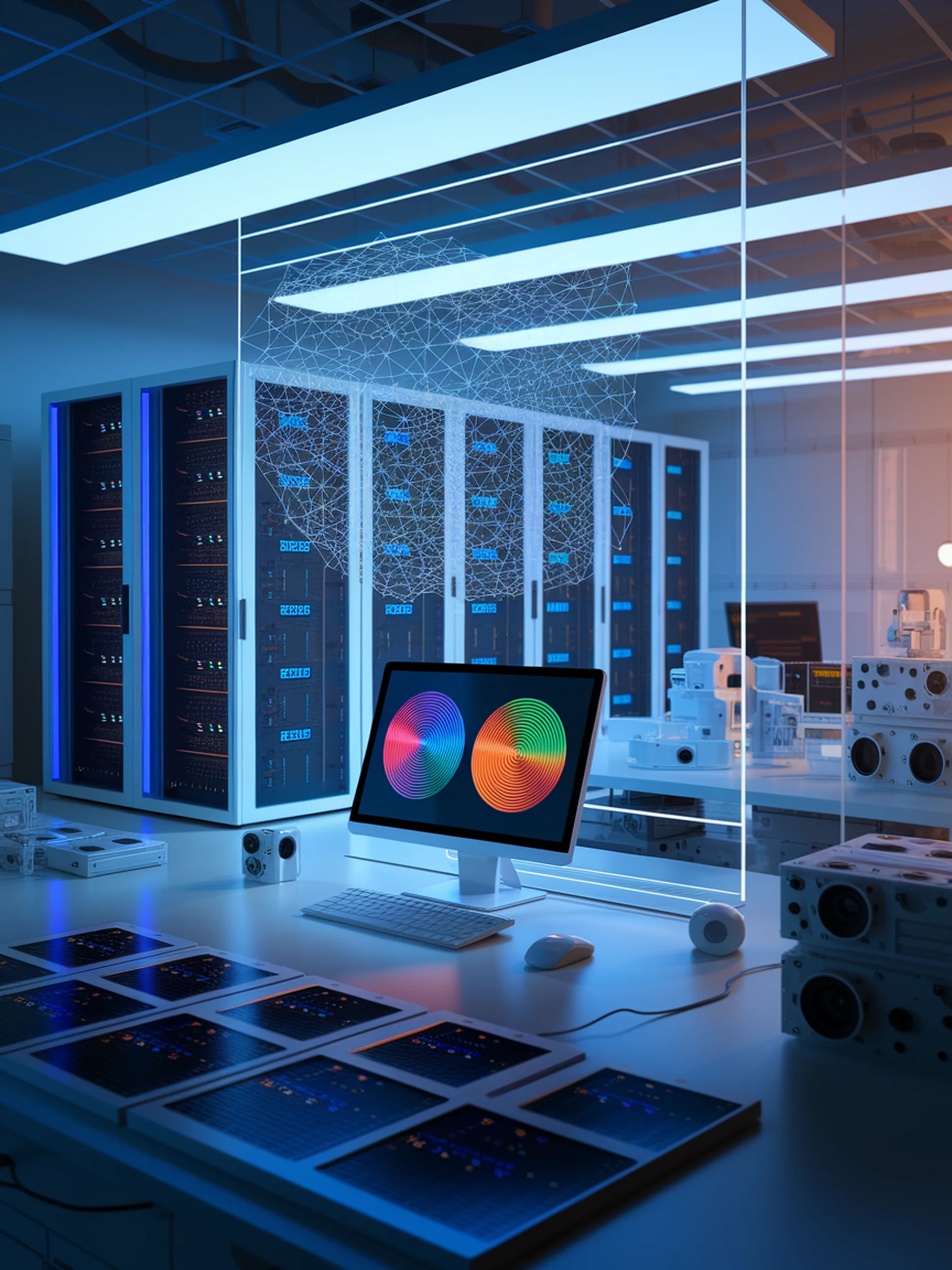
Accelerating Vision-Language Models
Adaptive Token Skipping for Efficient Multimodal Processing
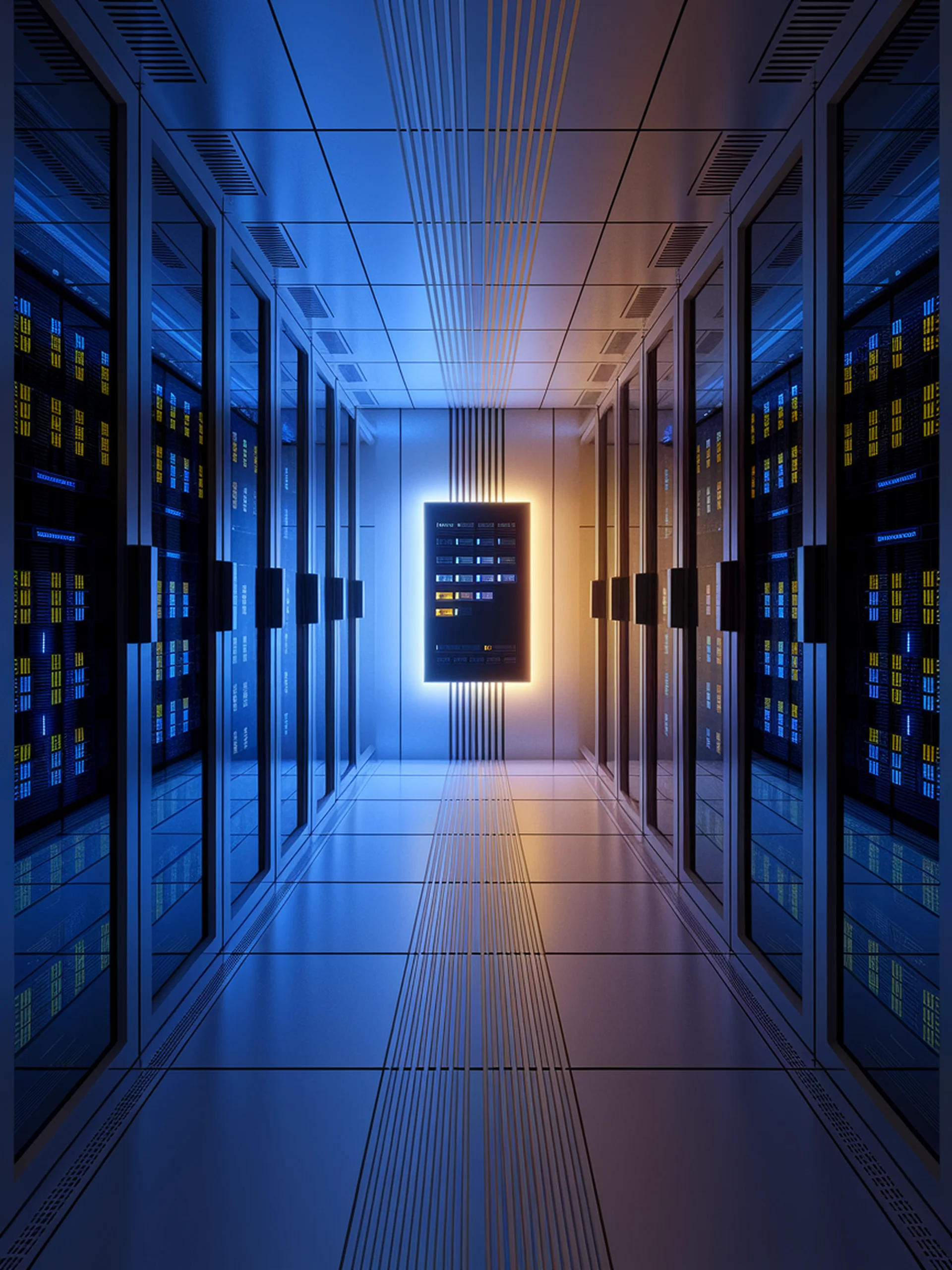
Breaking LLM Inference Silos
A unified framework for efficient LLM serving across workload types
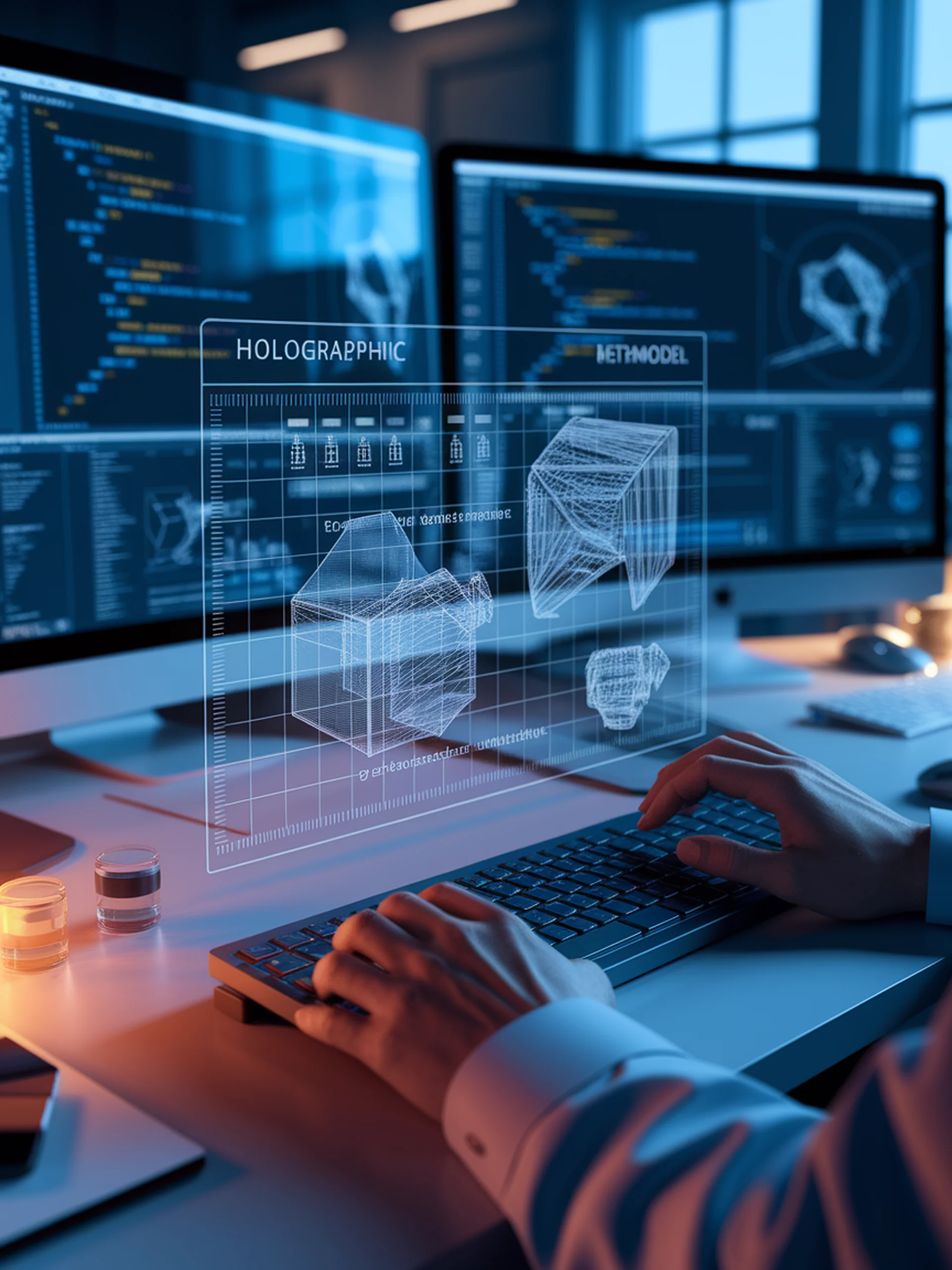
AI-Powered Model Generation
Automating Instance Models with Large Language Models
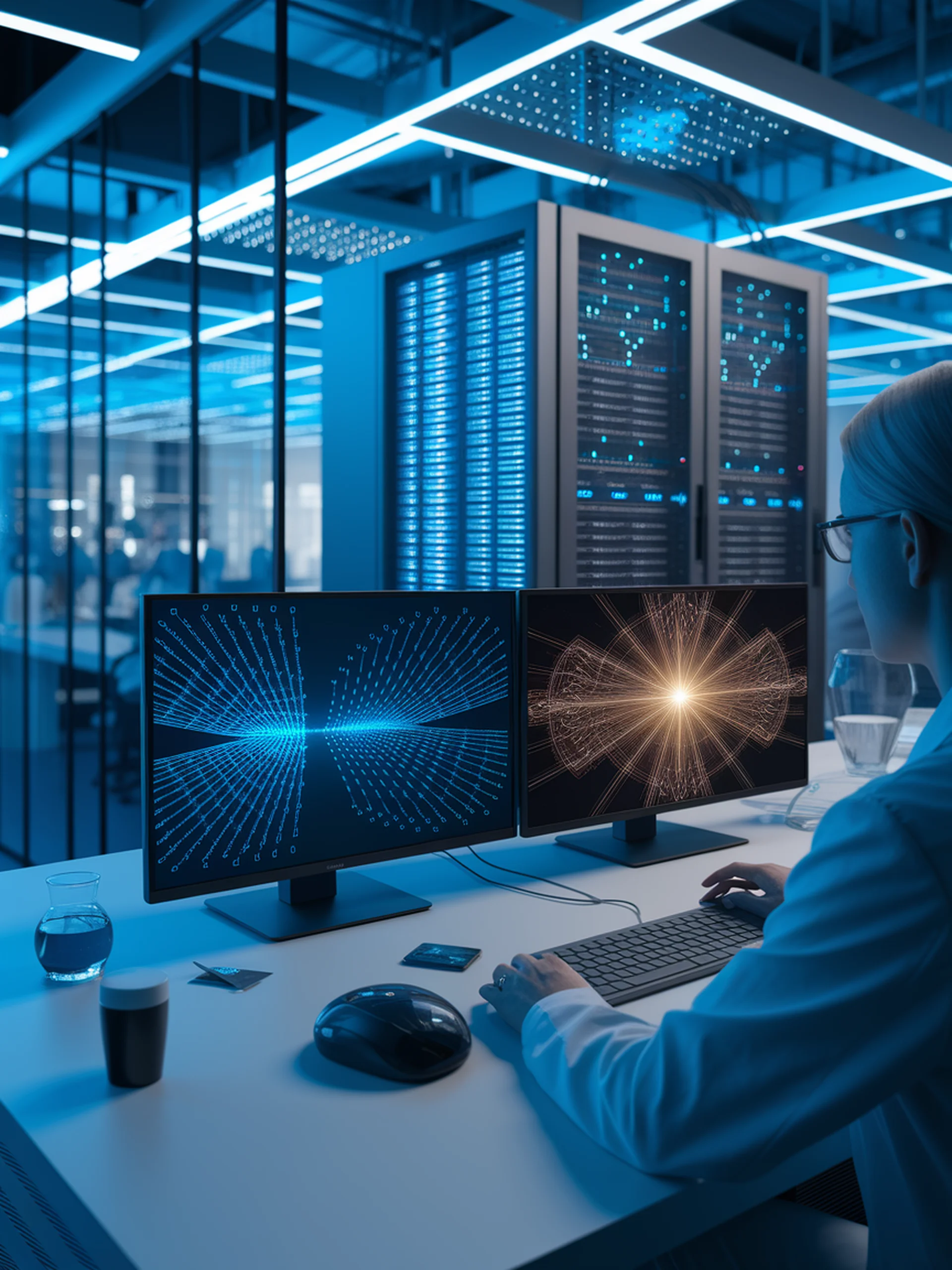
Optimizing Large Reasoning Models
Strategies for efficient AI reasoning without sacrificing quality
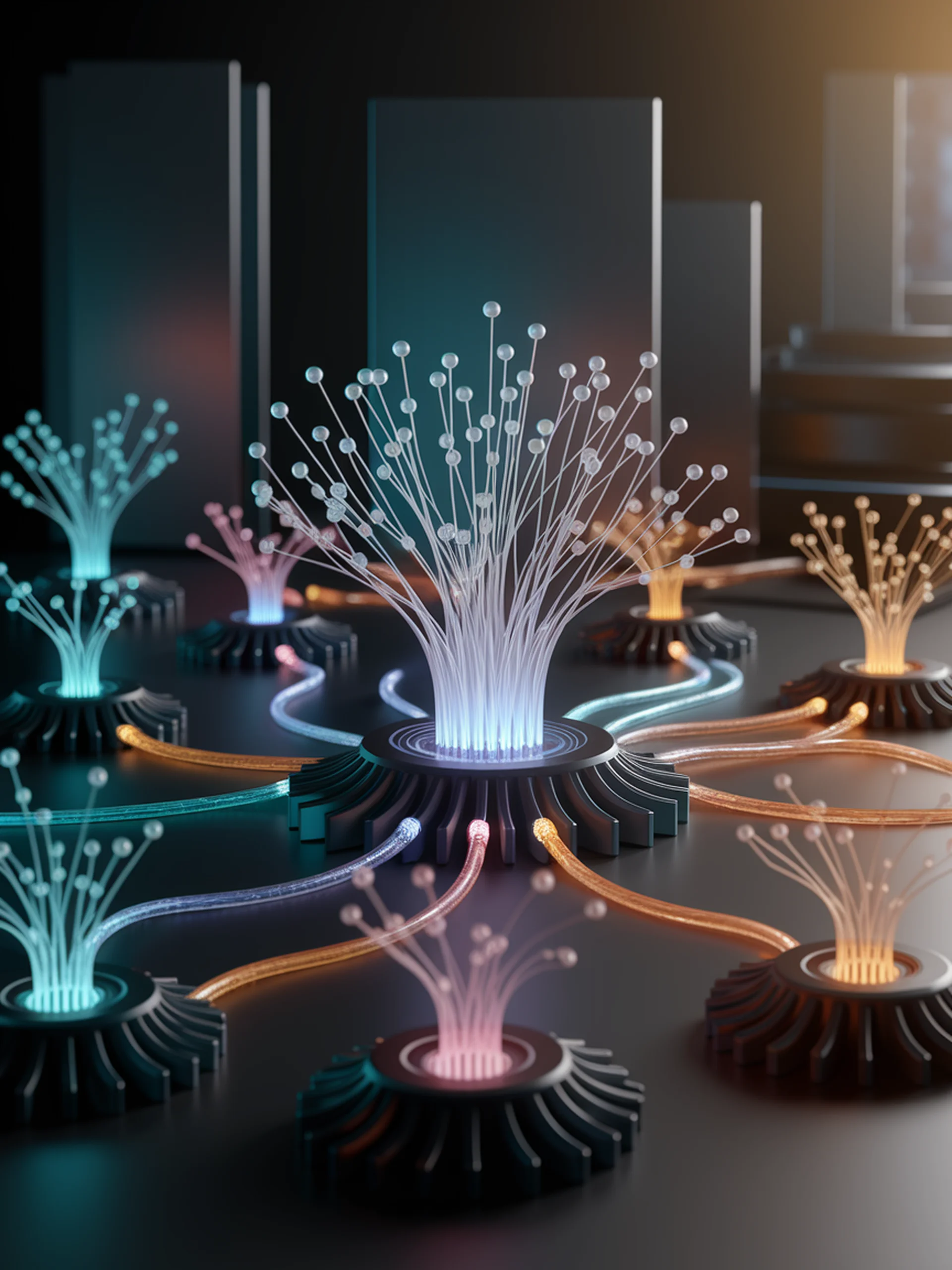
Smarter LLM Architecture: Mixture of Latent Experts
A resource-efficient approach to scaling language models
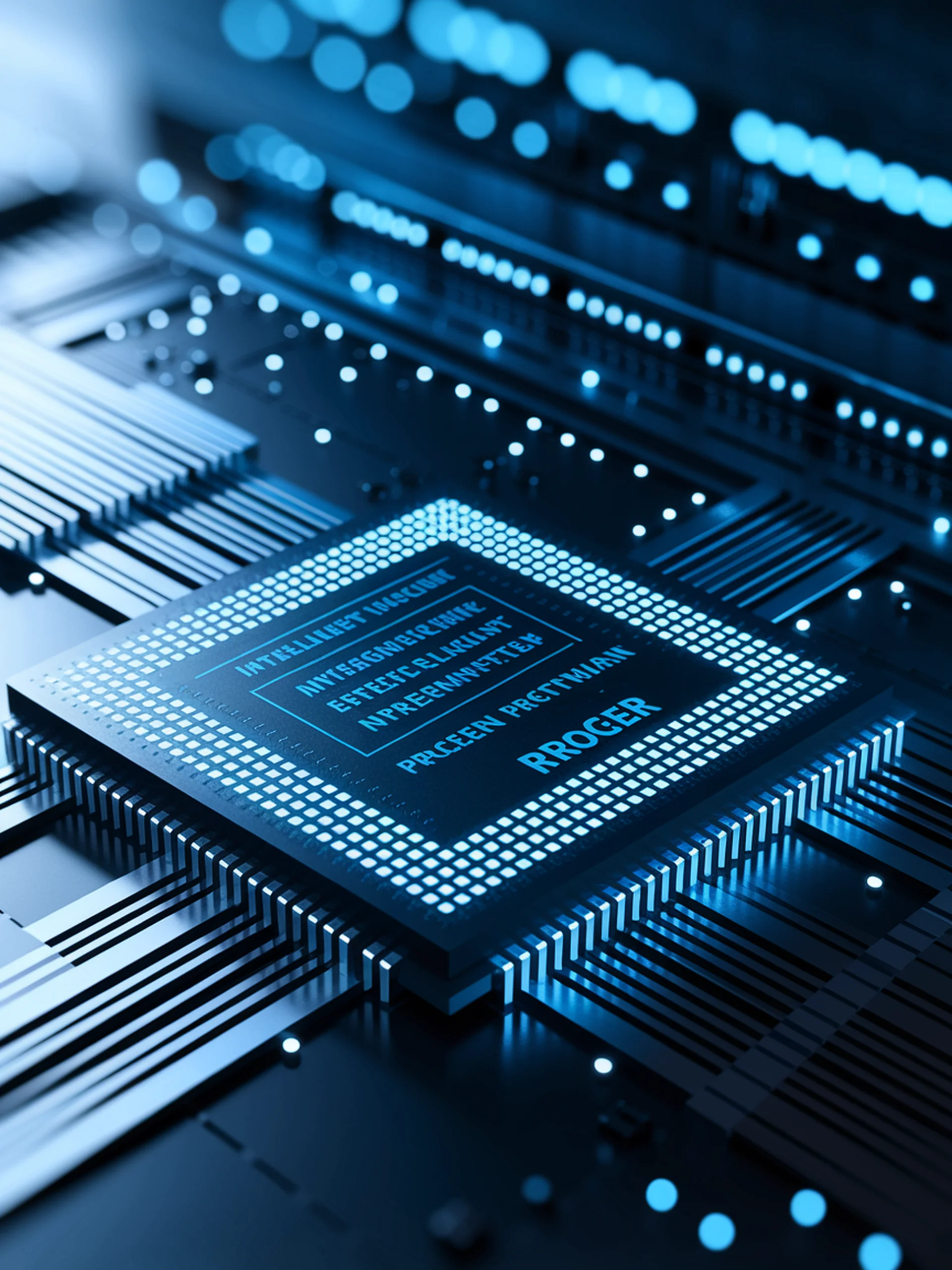
Smarter Token Processing for Faster LLMs
Reducing inference costs without compromising quality
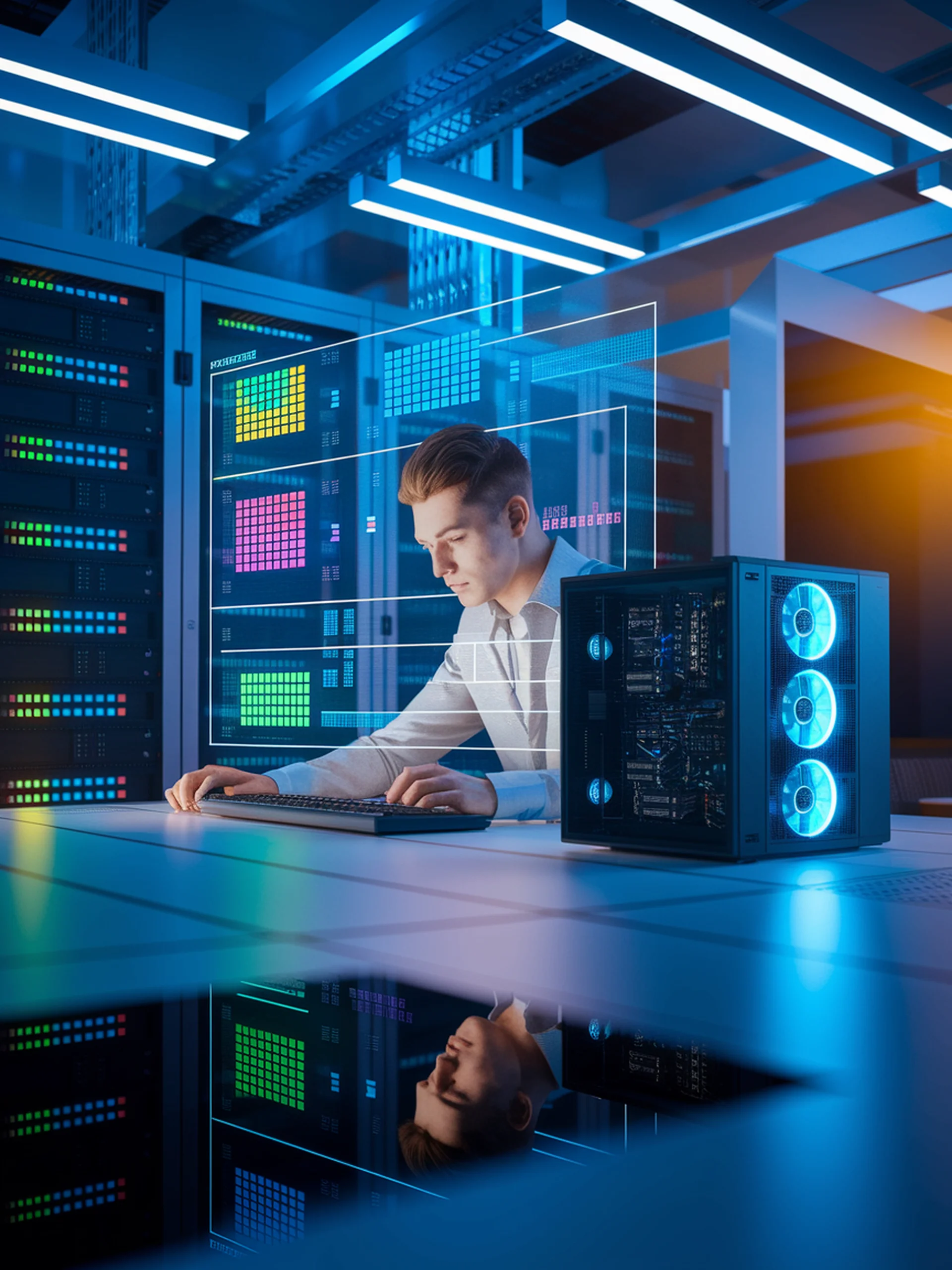
Cocktail: Optimizing LLM Performance for Long Contexts
Chunk-adaptive quantization boosts memory efficiency and inference speed
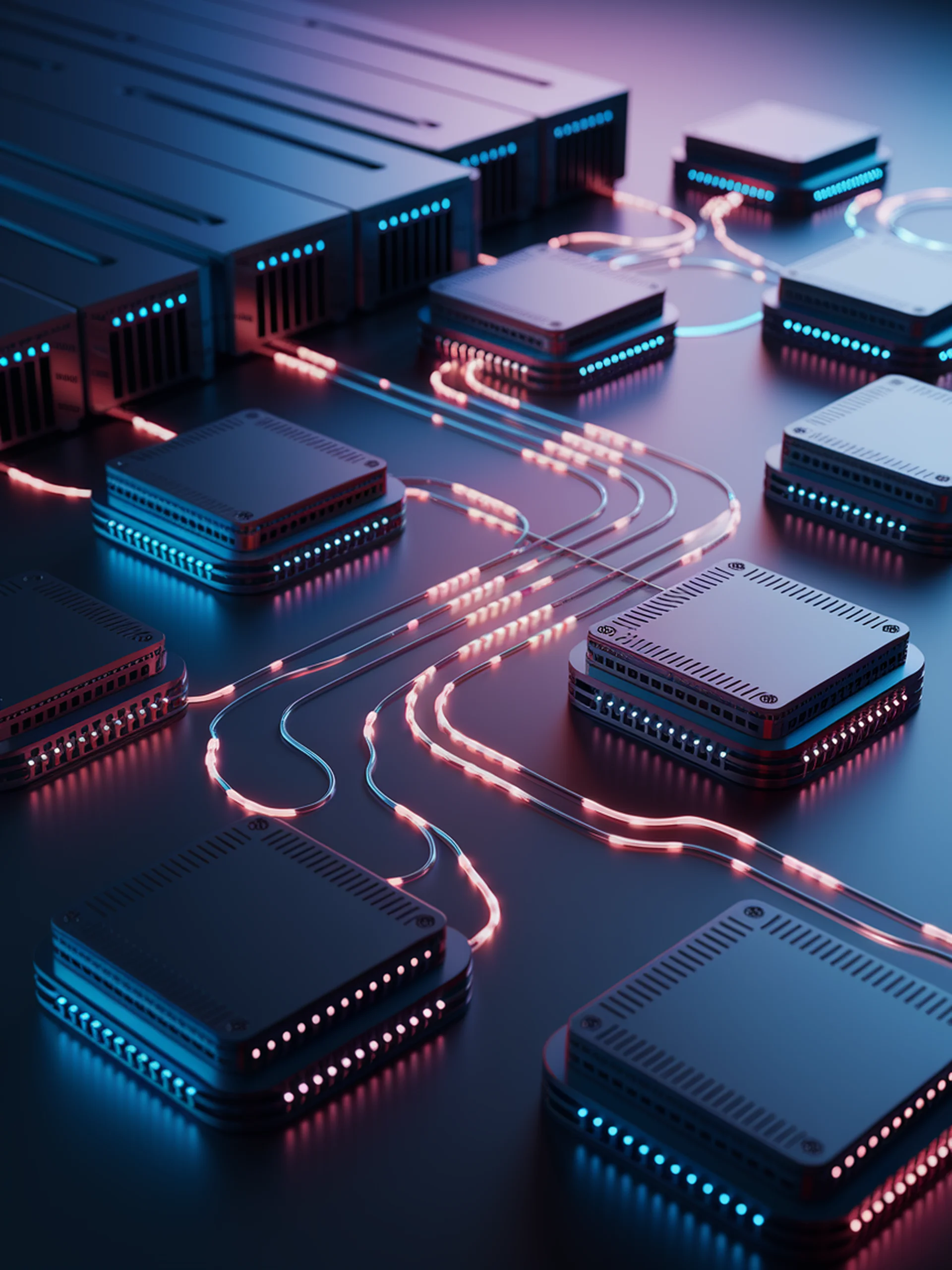
Enhancing LLM Fine-Tuning with Router Mixtures
A more powerful approach than traditional LoRA methods
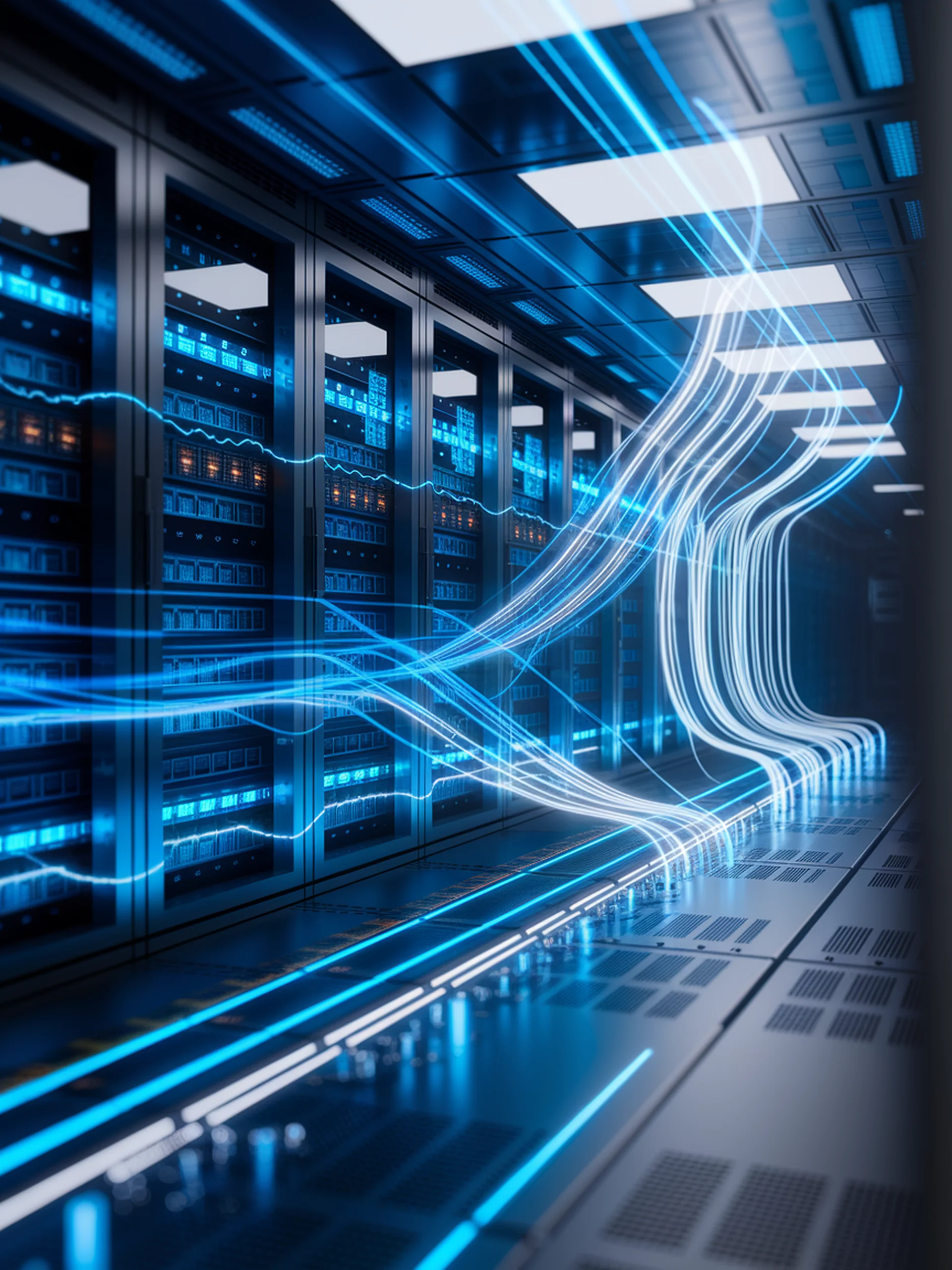
Smart Layer-Skipping for Faster LLMs
Dynamically adjusting computational resources during token generation
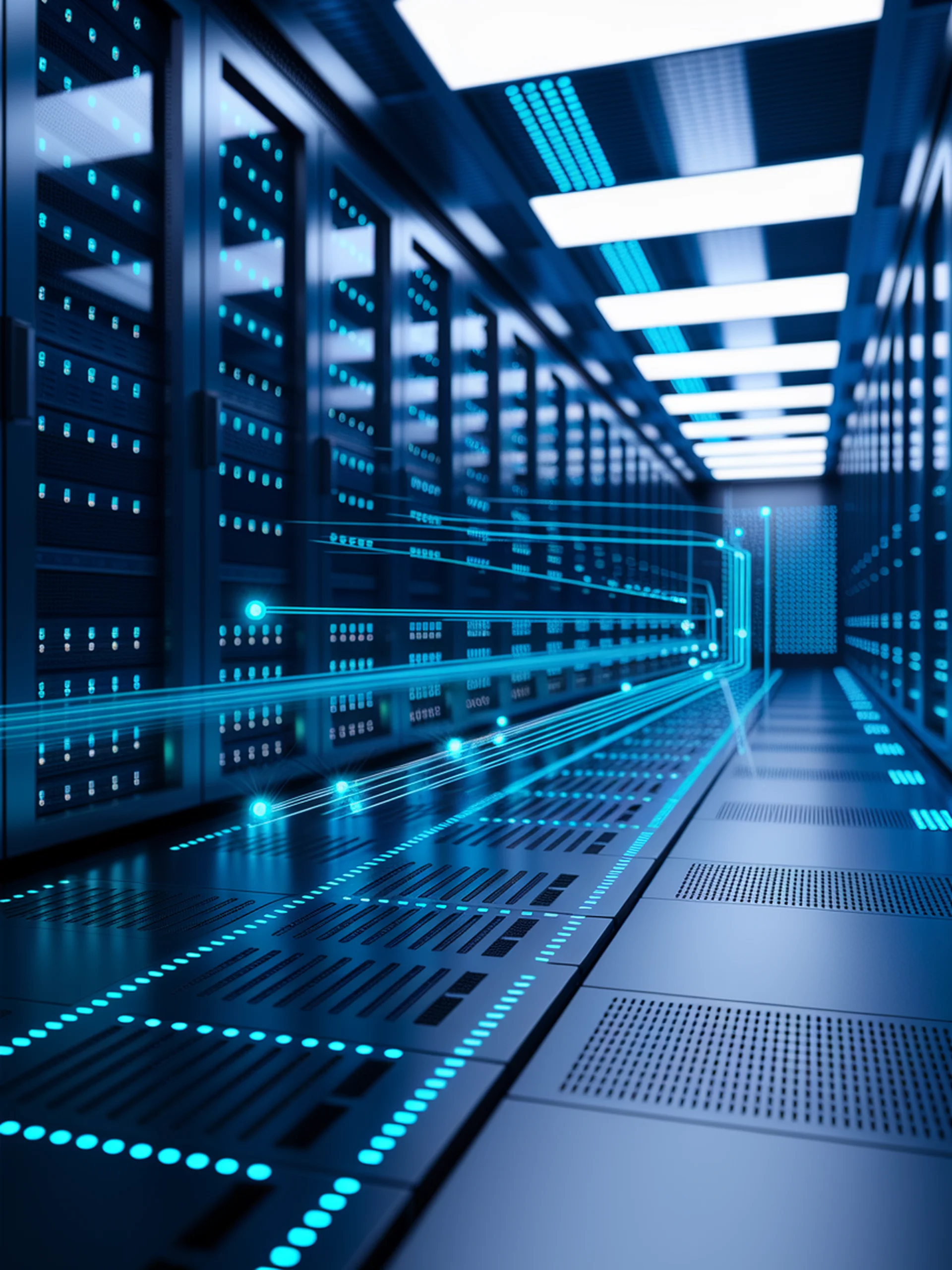
Optimizing LVLMs with AirCache
Reducing memory bottlenecks in vision-language models through intelligent caching

Optimizing KV Cache for LLM Performance
A practical analysis of compression techniques for efficient LLM serving
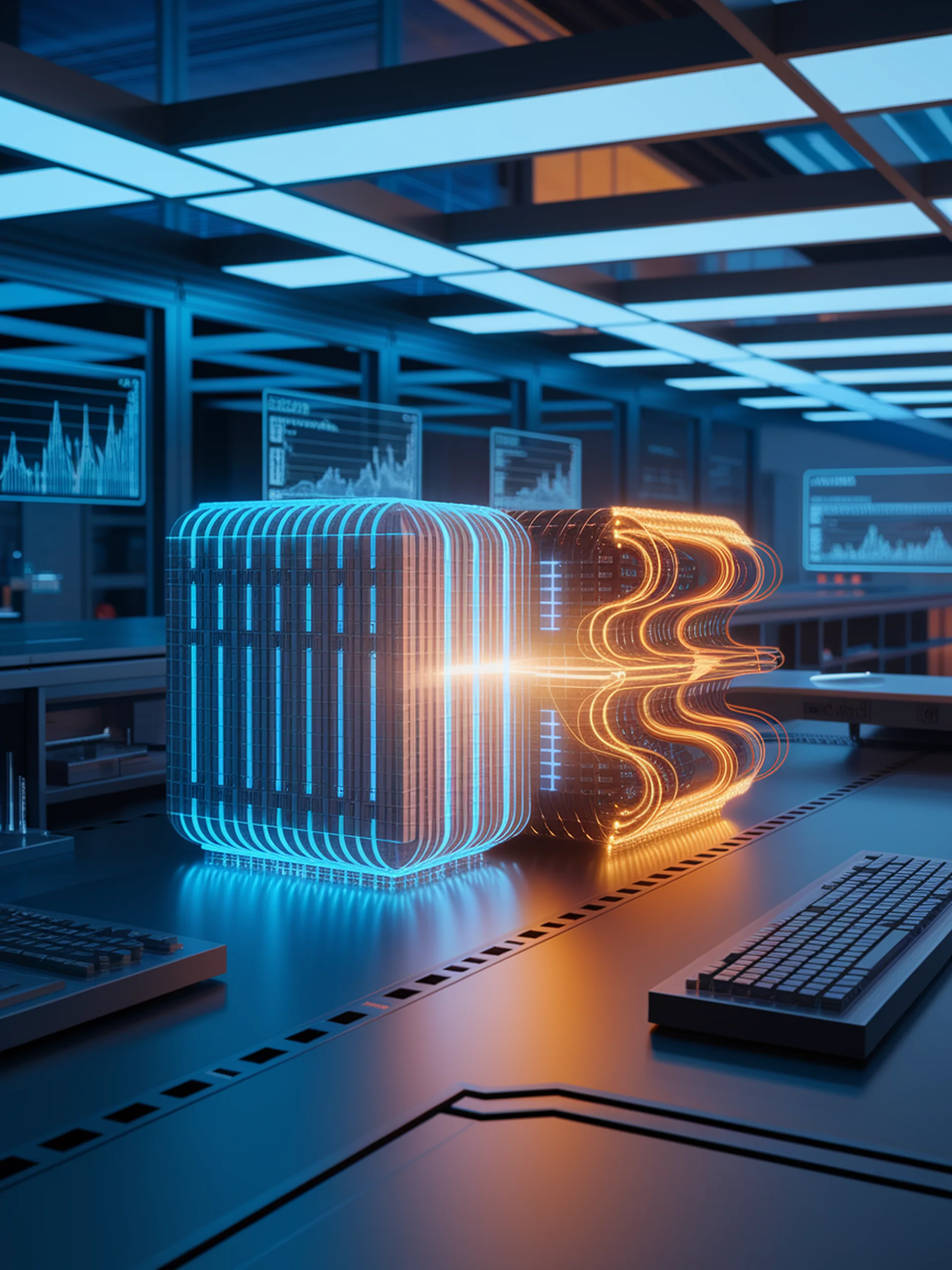
TransMamba: Best of Both Worlds
A hybrid architecture combining Transformer efficiency with Mamba performance
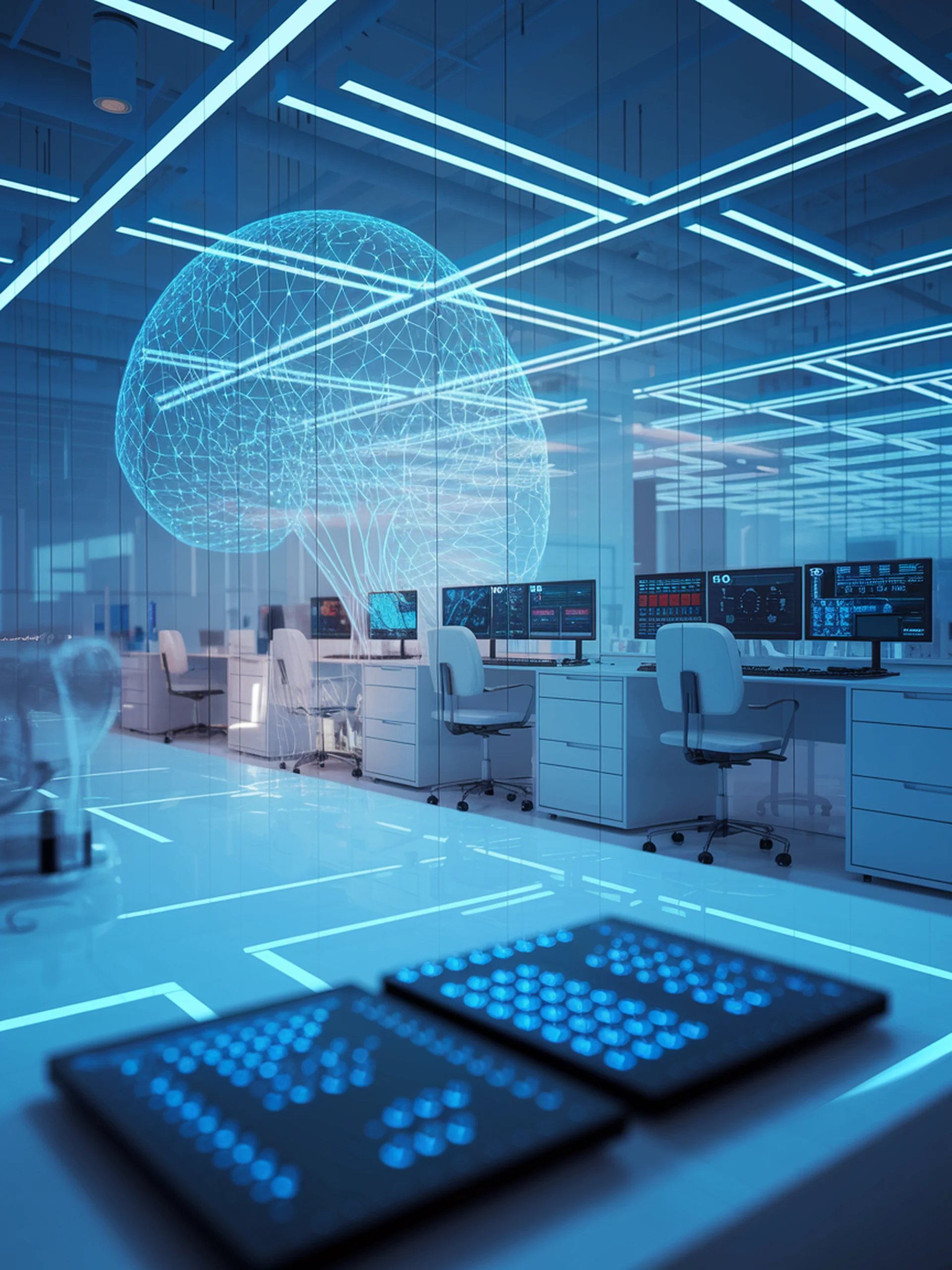
Optimizing Sparse Autoencoders for LLM Interpretability
A Theoretical Framework for Feature Extraction in Large Language Models
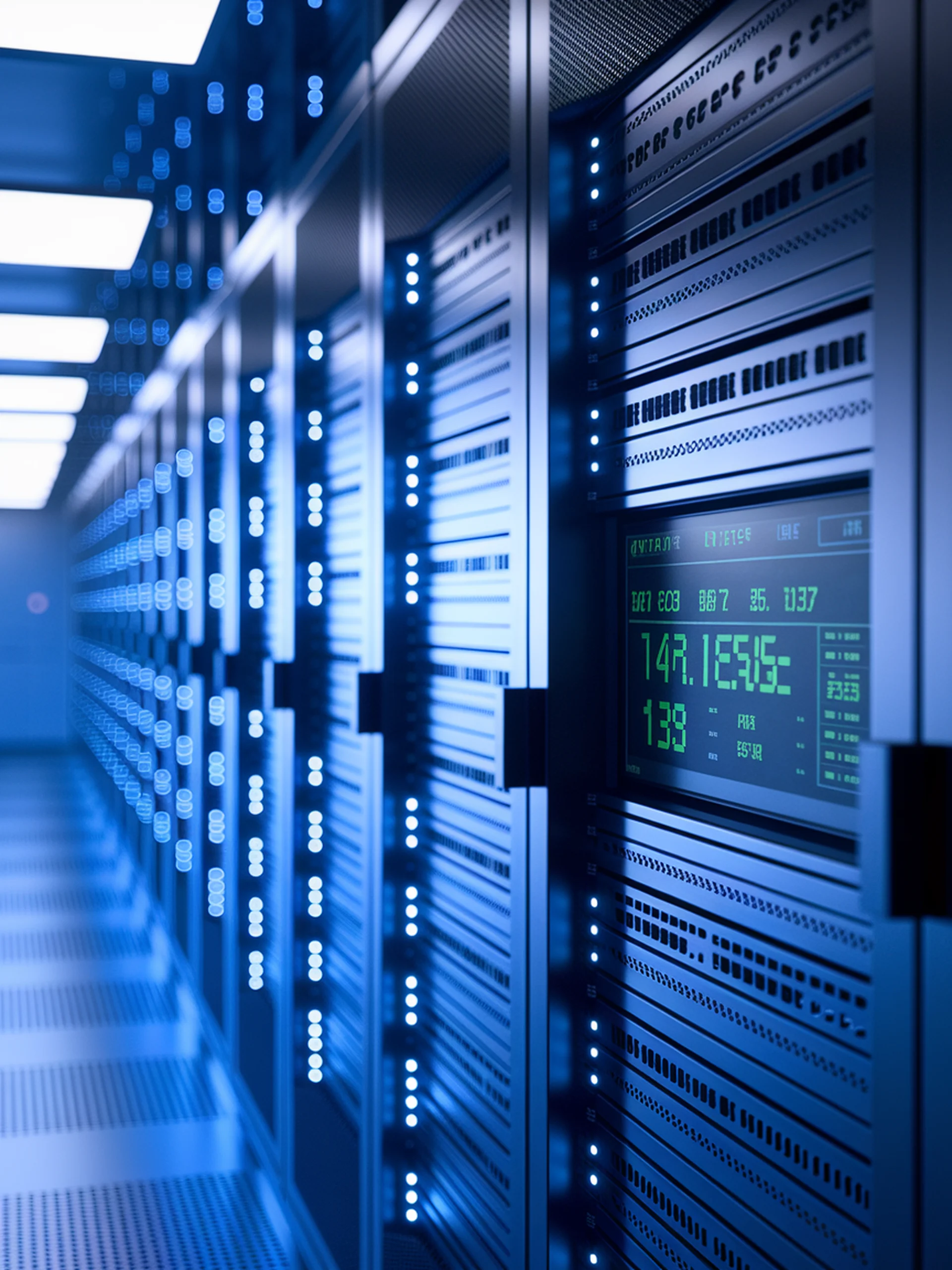
Adaptive Speculation for Faster LLMs
Dynamic calibration to maximize inference speed with minimal waste
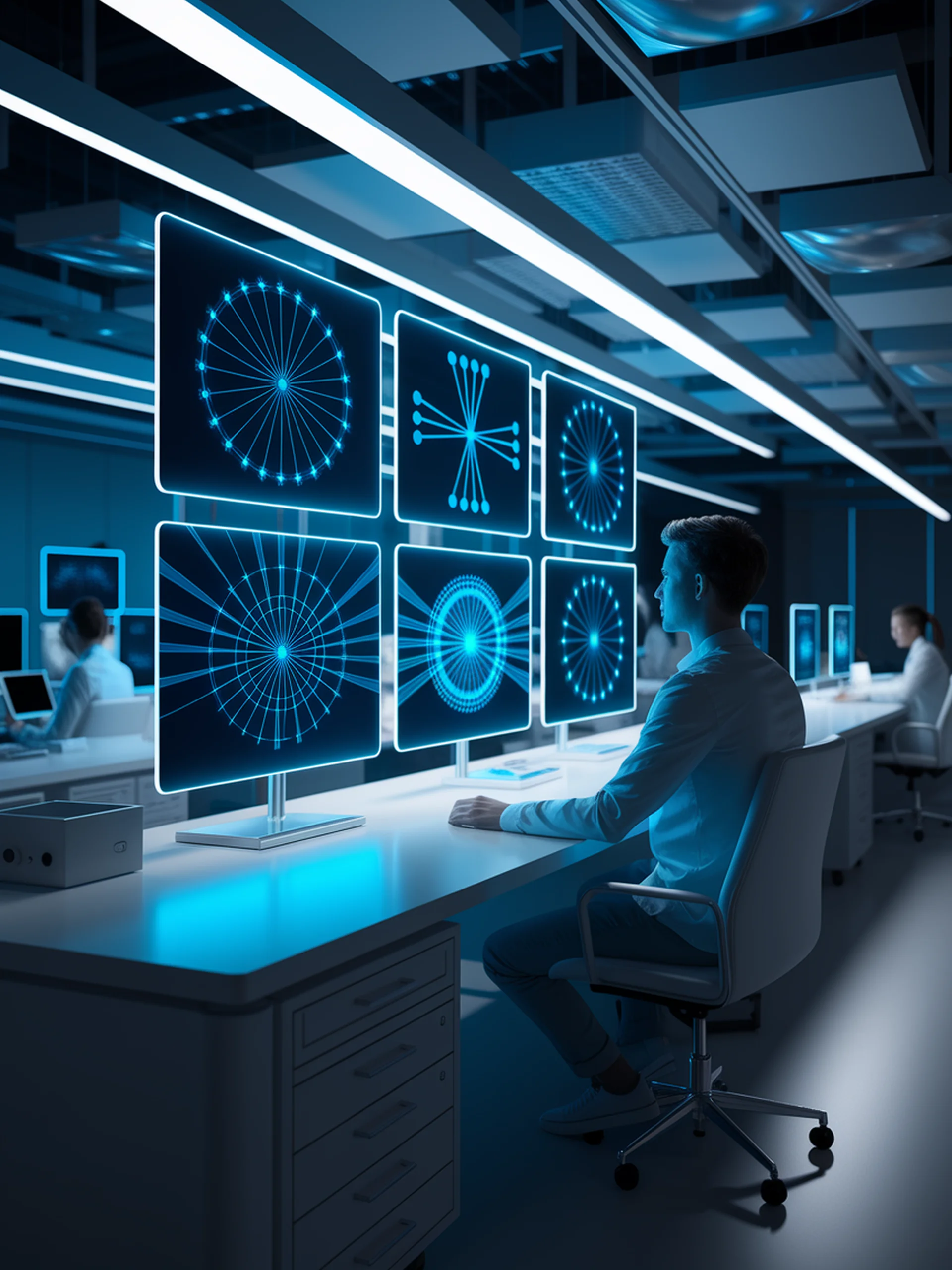
Hawkeye: Streamlining AI Reasoning
Optimizing Chain-of-Thought Processes for Faster, More Efficient LLMs
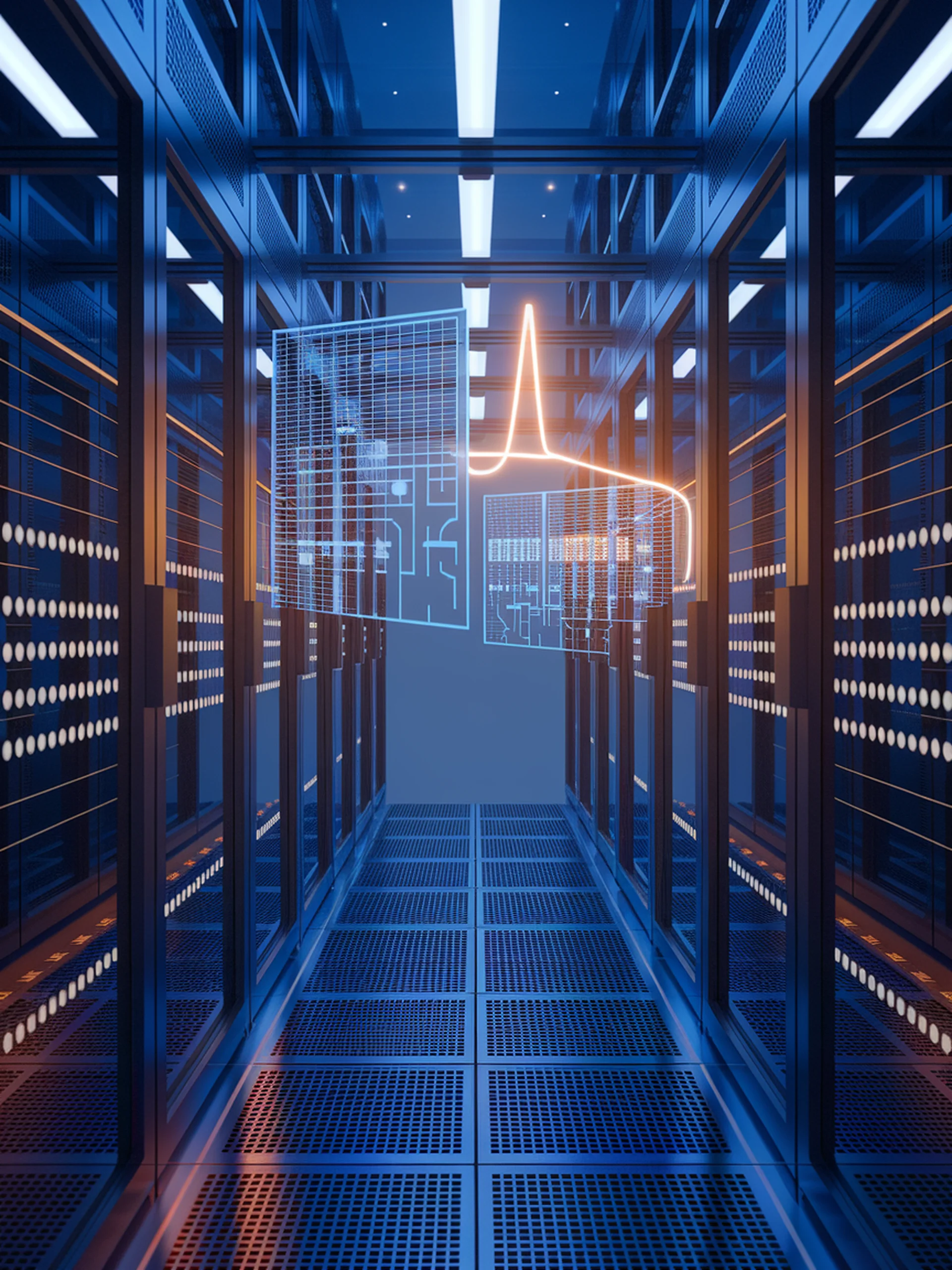
ShortV: Making Multimodal LLMs Faster
Freezing Visual Tokens Where They Don't Matter
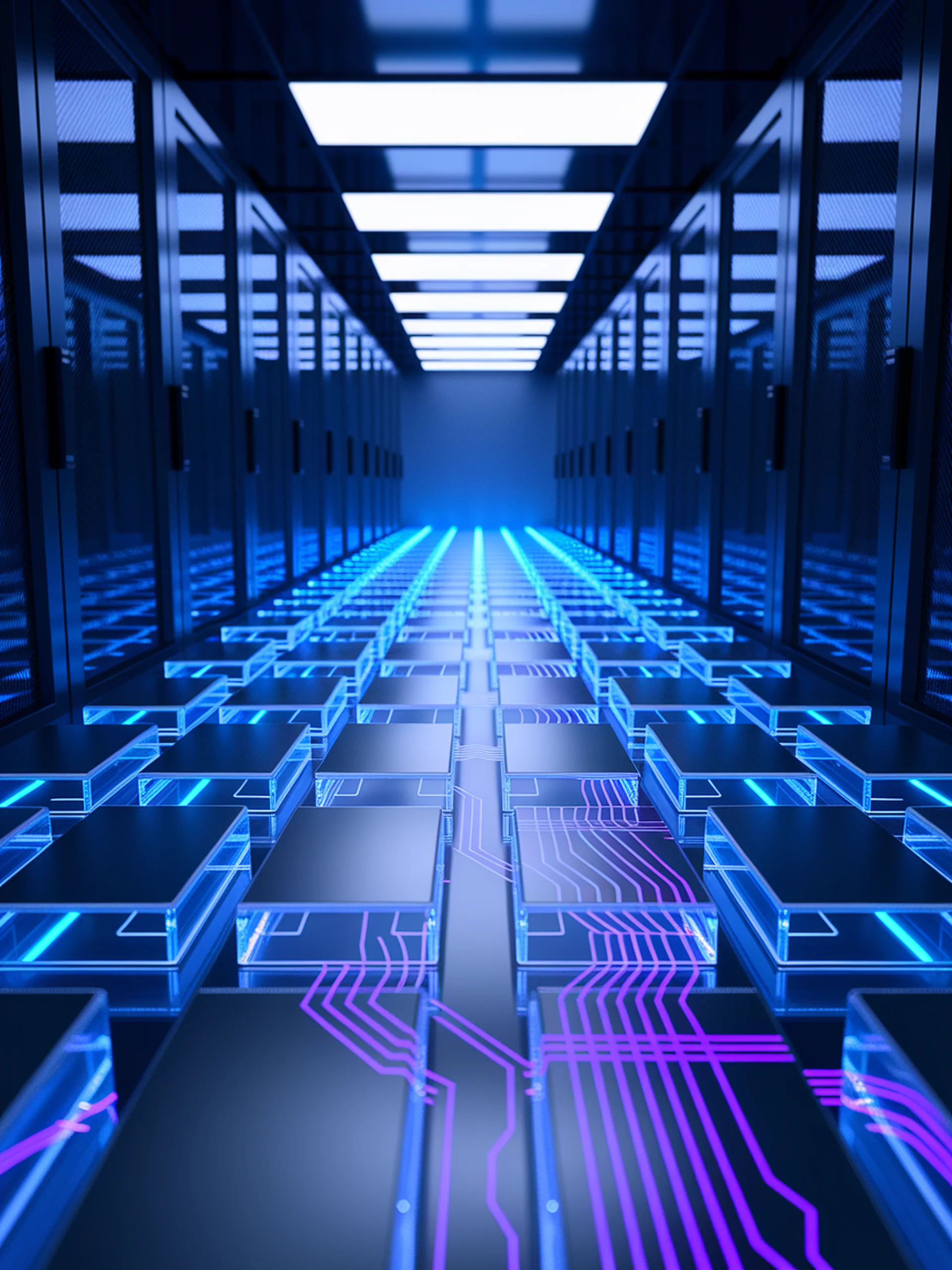
Sentence-Level KV Caching
Boosting LLM Efficiency Through Semantic-Aware Memory Management
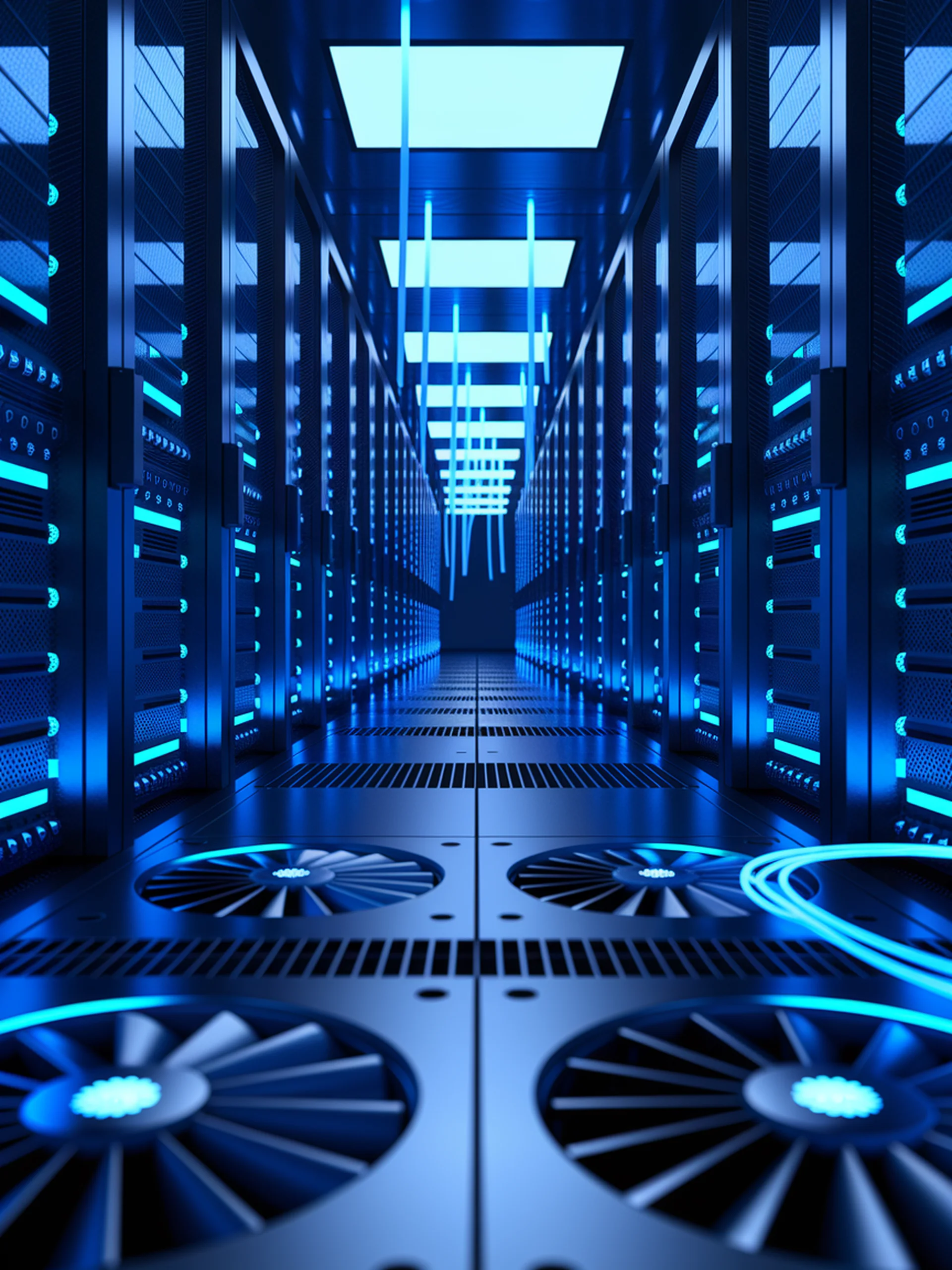
Scaling MoE Models for Efficient Inference
A new architecture for cost-effective AI model deployment
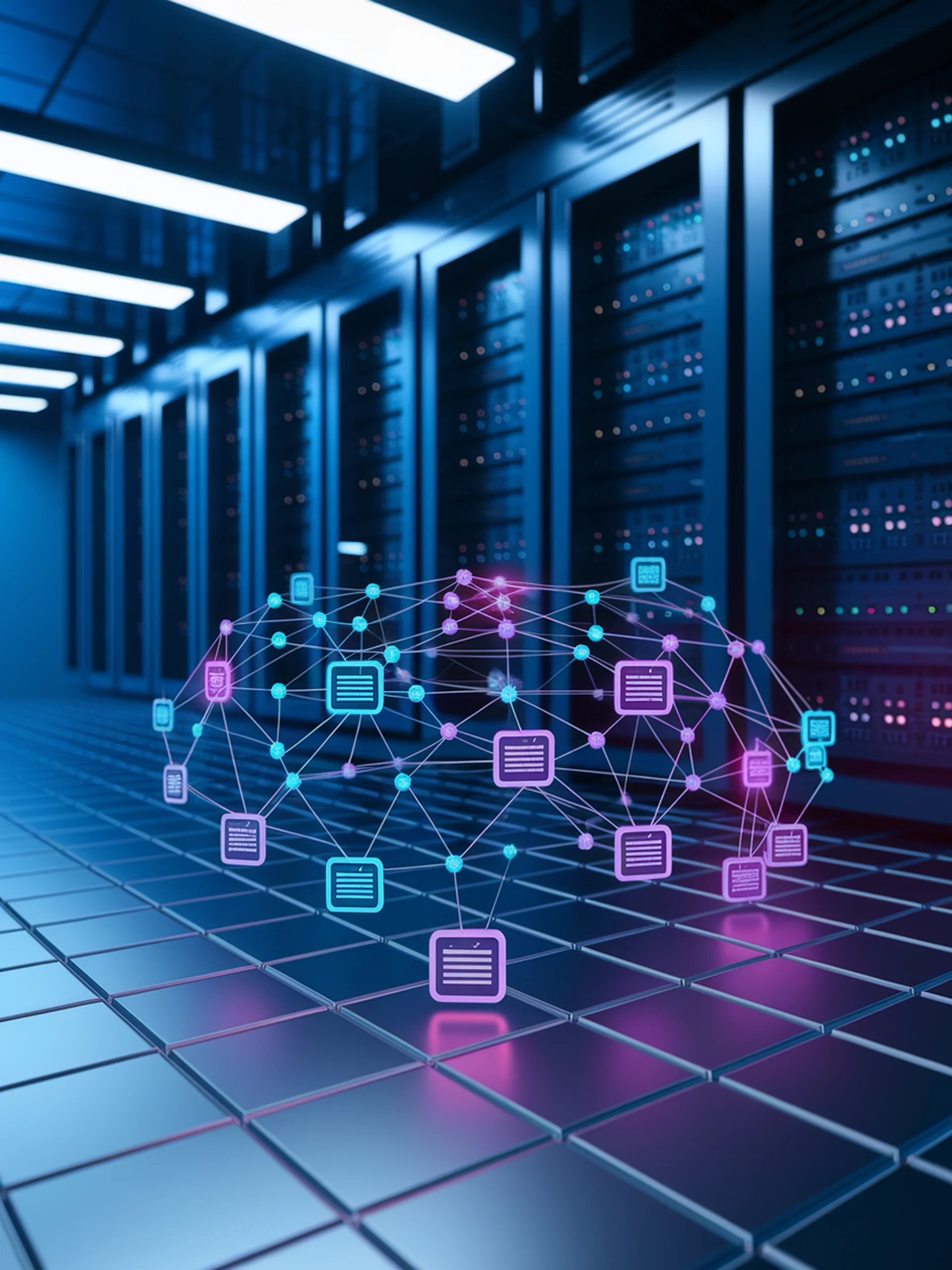
Enhancing Graph Learning with LLMs
A unified framework for robust text-attributed graphs
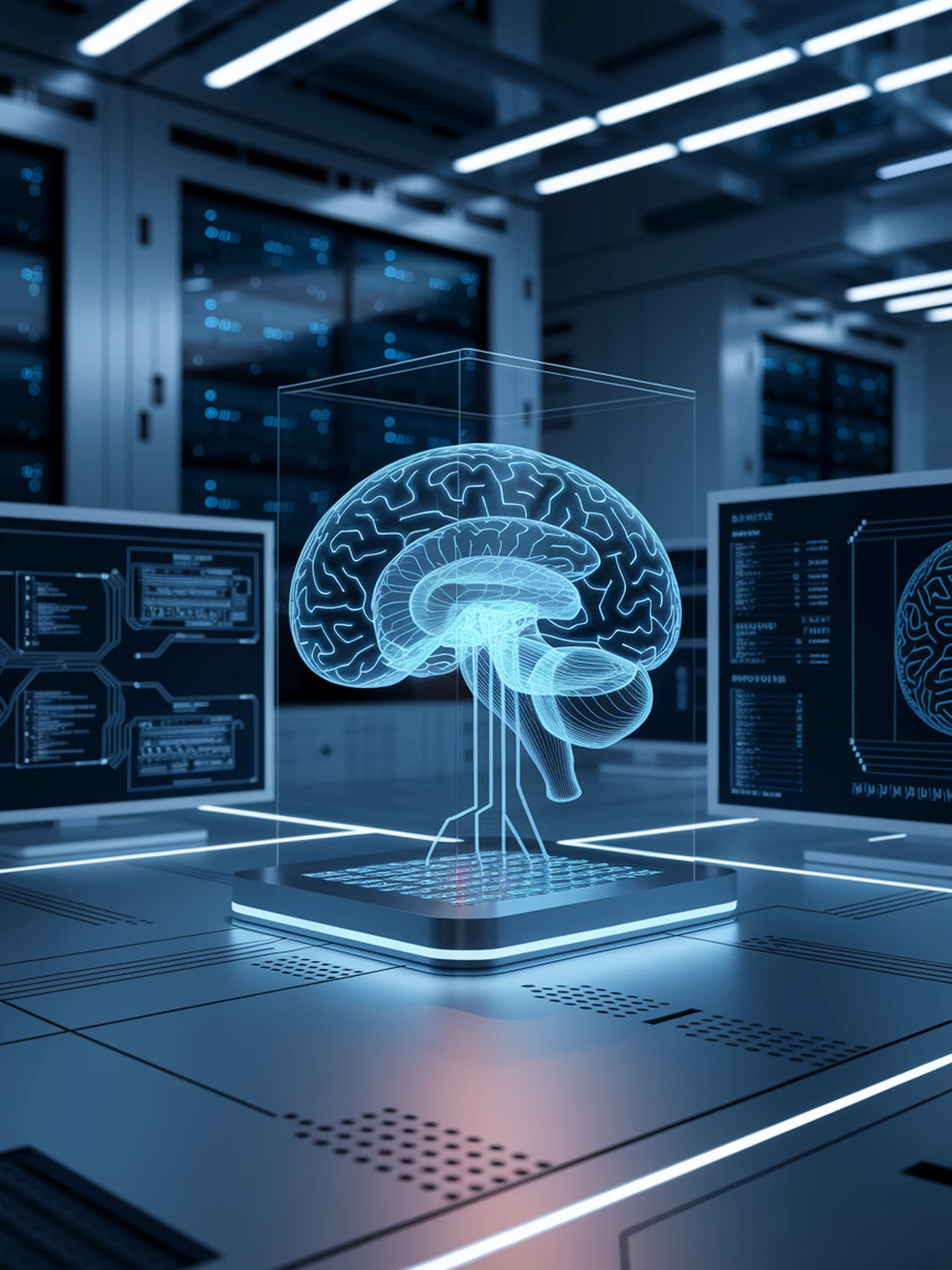
Memory Architecture in LLMs
How LLMs can develop cognitive memory systems to enhance performance
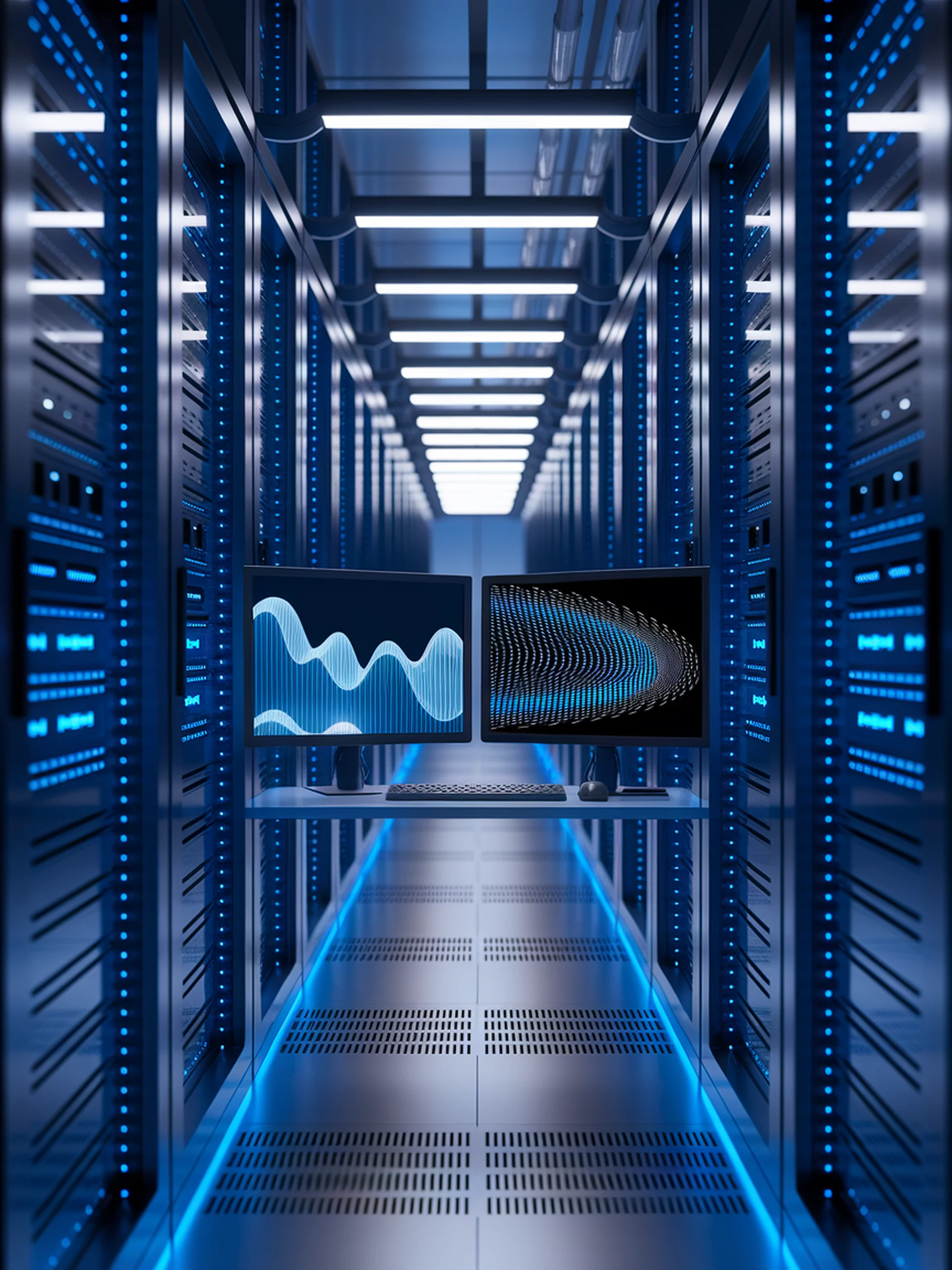
Taming the Spikes in LLM Training
Adaptive Gradient Clipping for More Stable AI Model Development
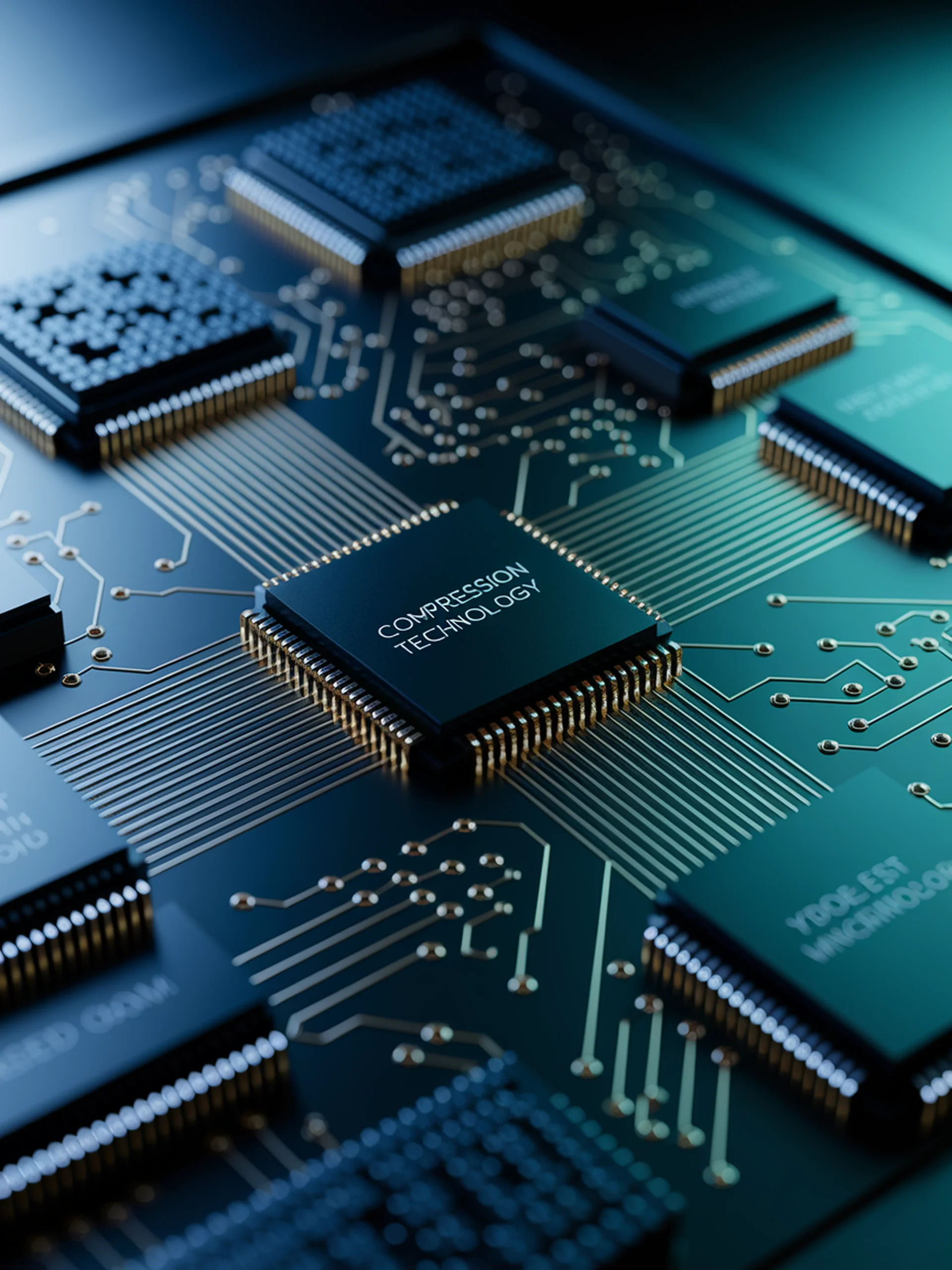
GPTQv2: Smarter Model Compression
Efficient quantization without finetuning using asymmetric calibration
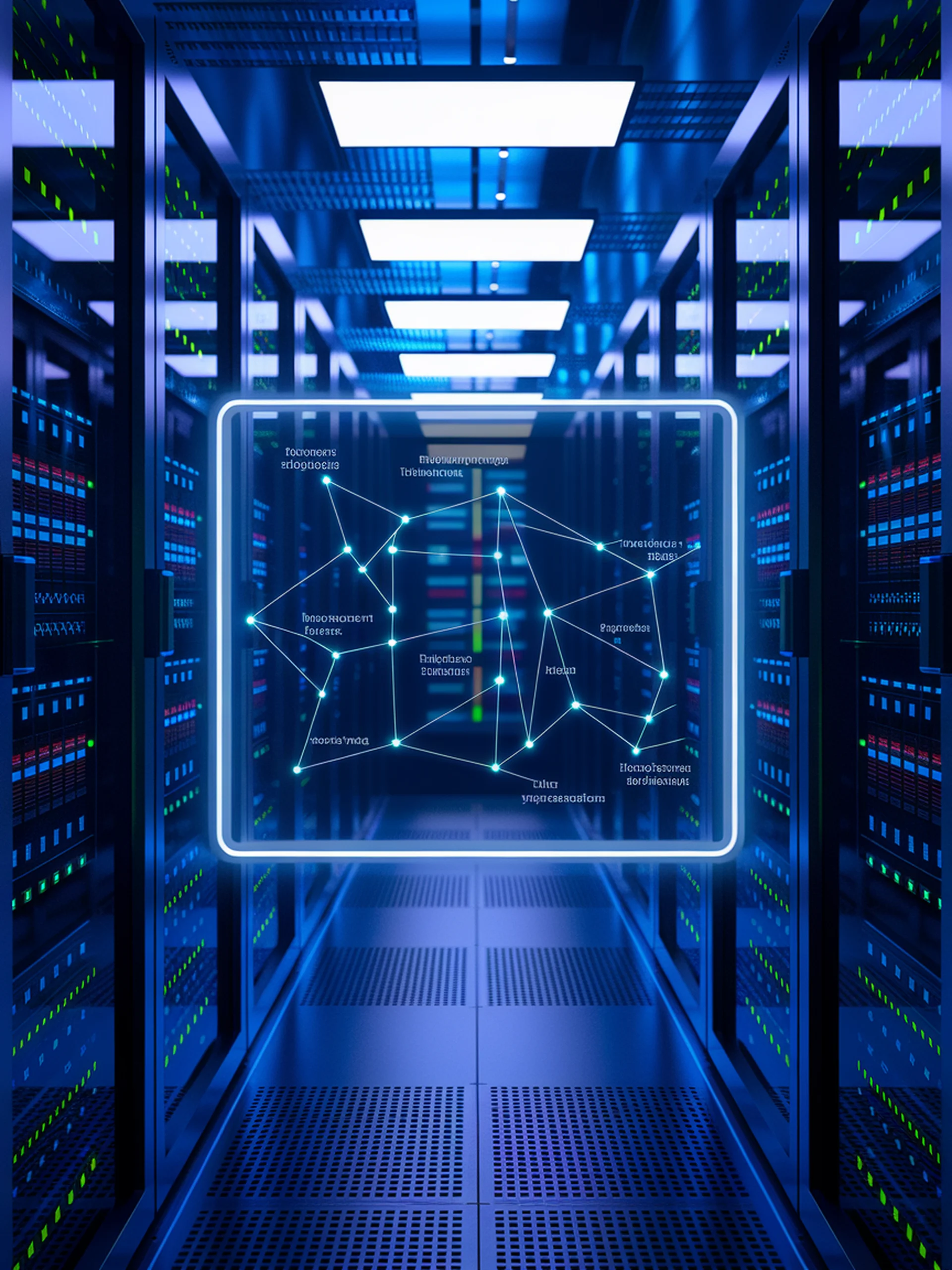
Smart Scheduling for Complex AI Workloads
Optimizing LLM Application Performance Under Uncertainty
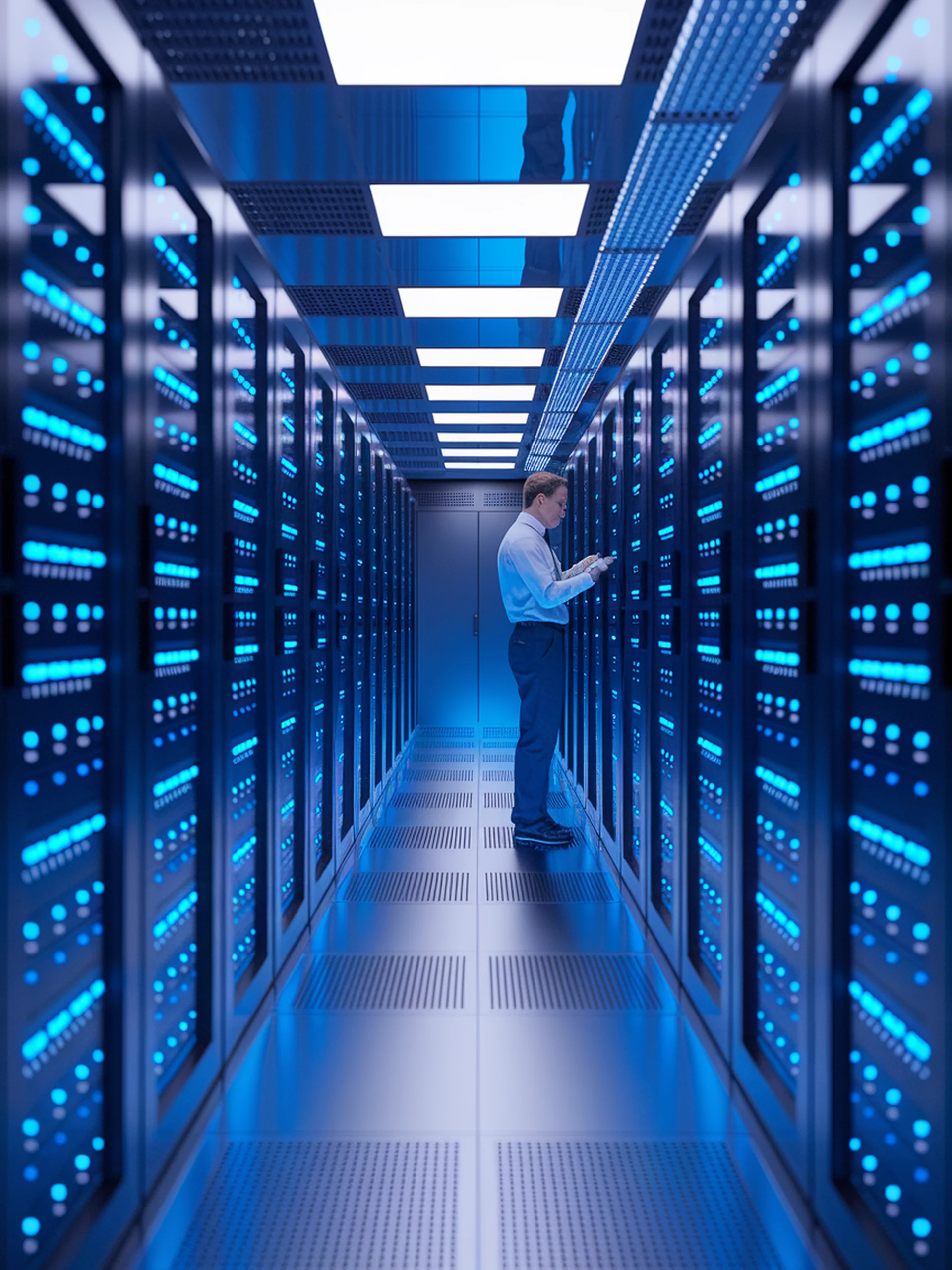
AIBrix: Cutting Costs for LLM Deployment
A cloud-native framework for efficient, scalable LLM inference
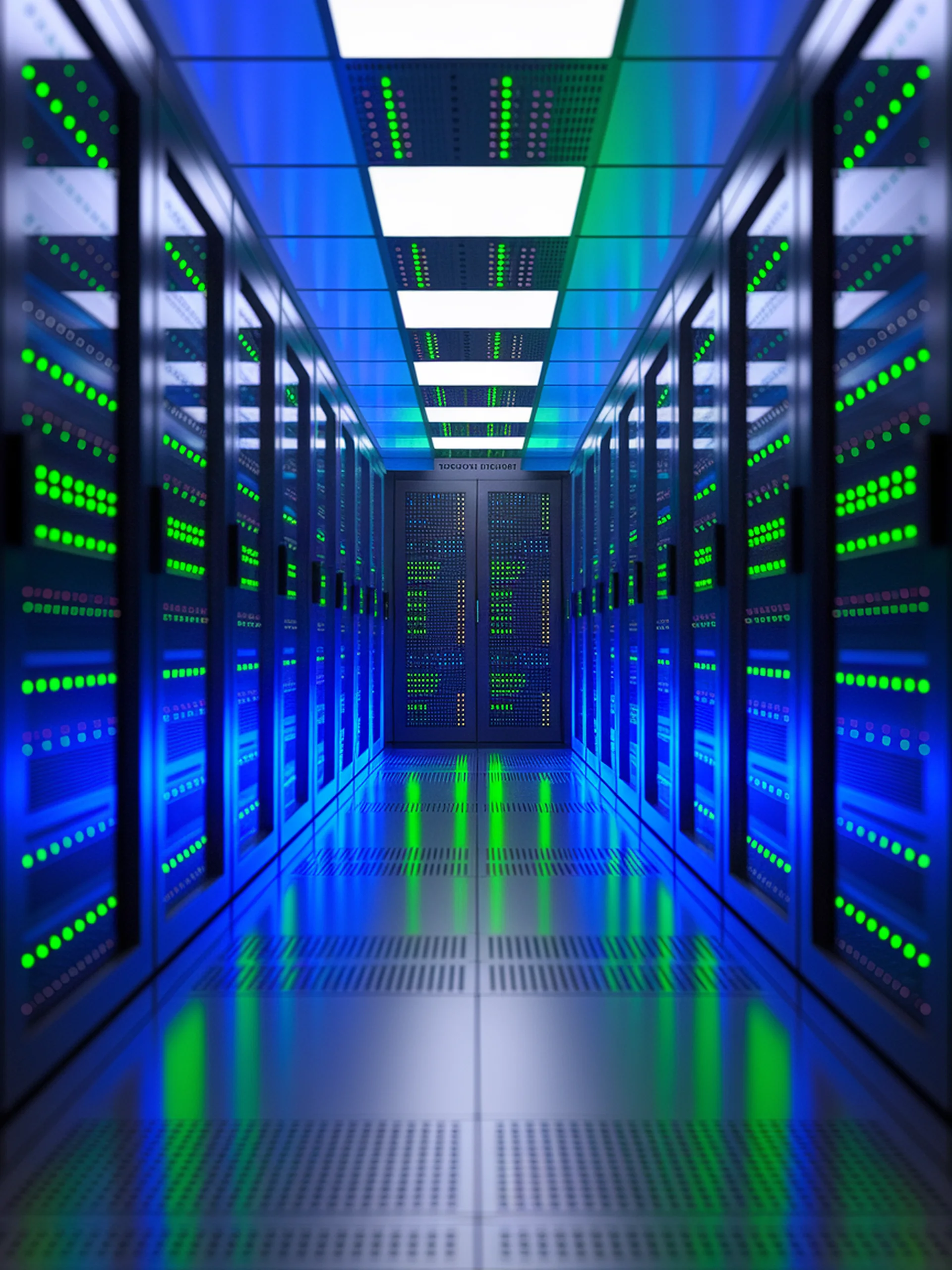
Optimizing LLM Deployment Economics
Efficient co-scheduling of online and offline tasks for better resource utilization
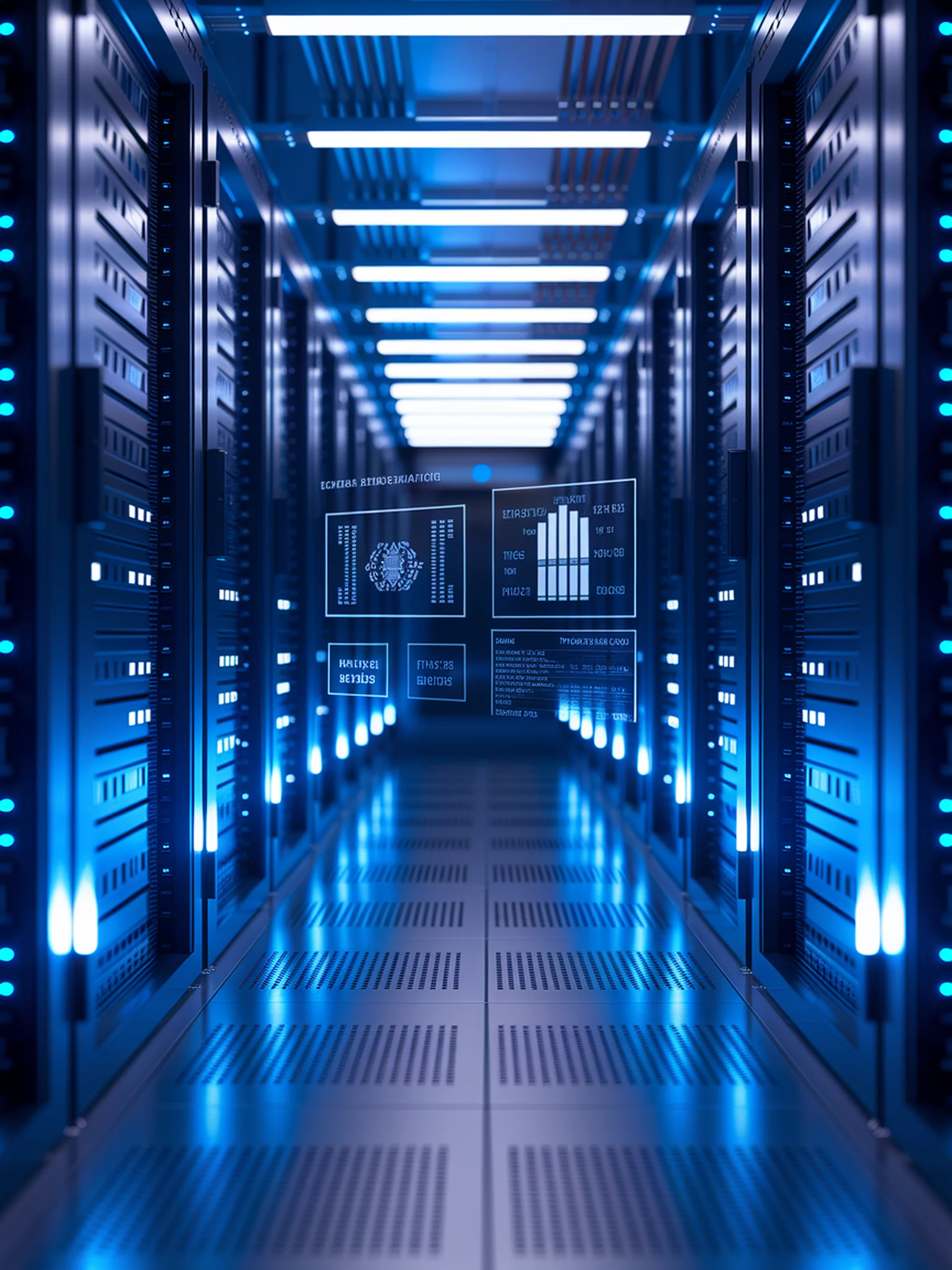
Scaling Up Transformer Training: A Memory-Focused Approach
Optimizing hardware resources for more efficient large model training
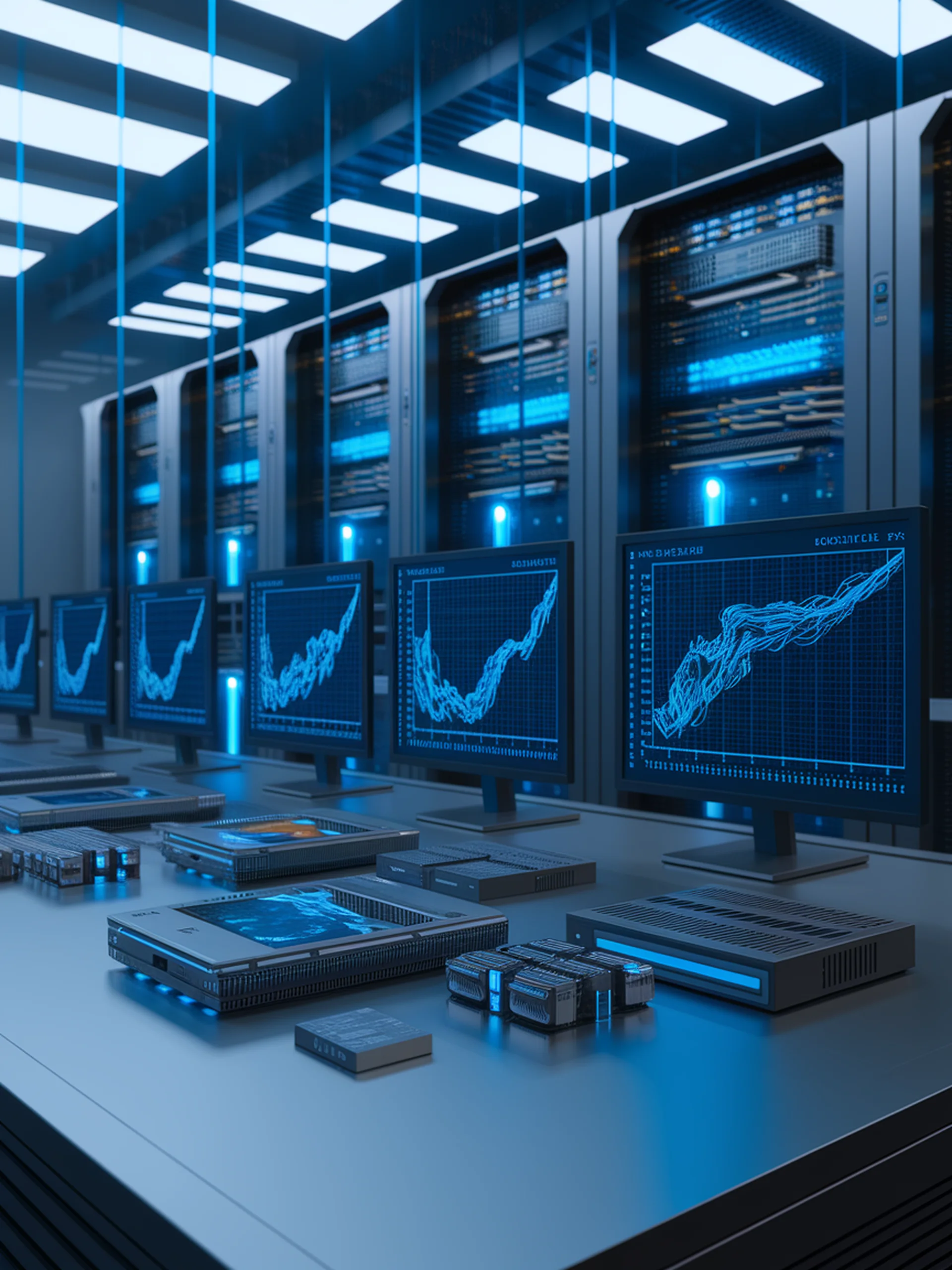
Scaling LLMs for Long-Context Applications
Optimizing Memory & Speed with Advanced Quantization
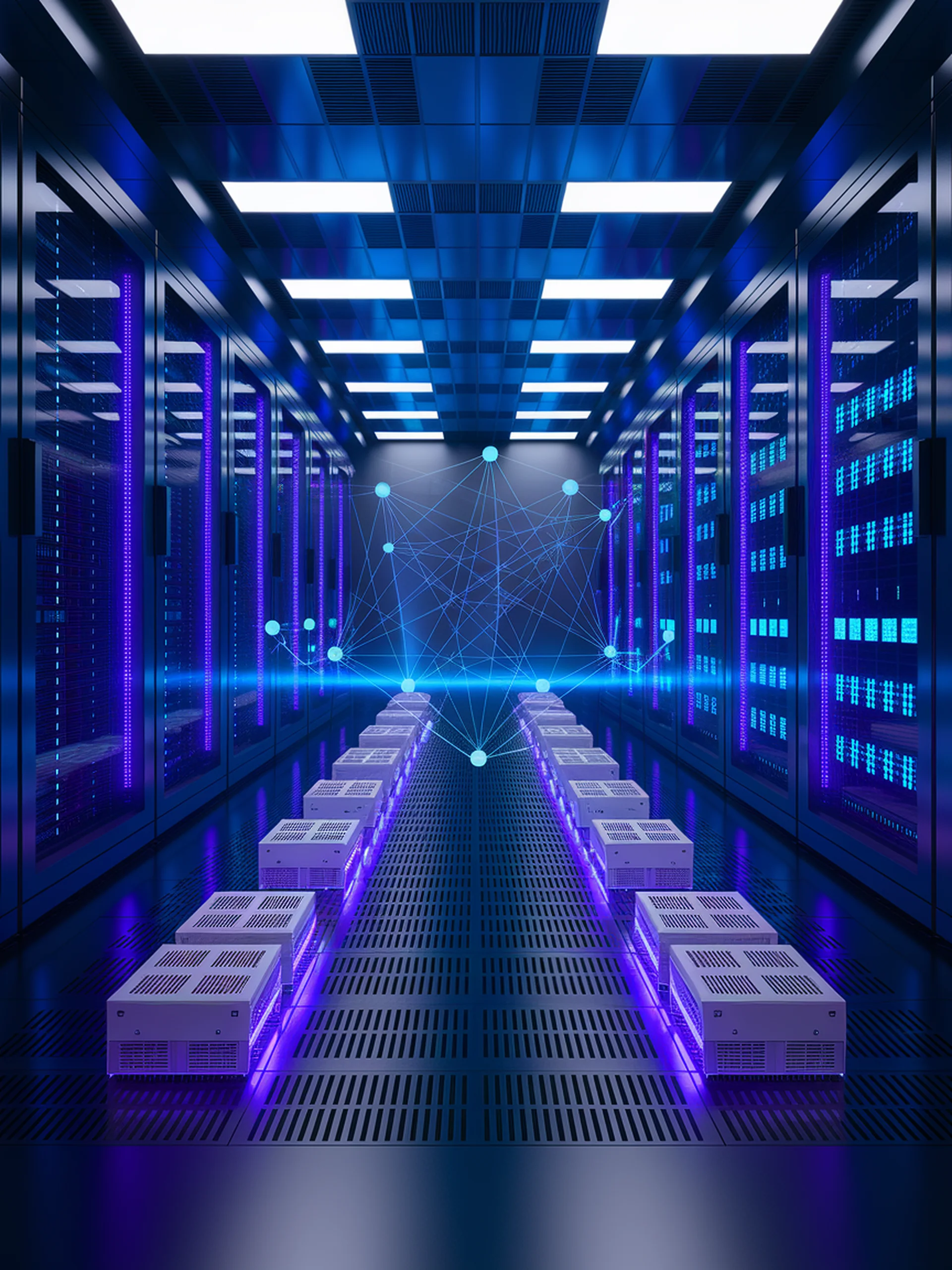
Automating LLM Training at Scale
Dynamic optimization of distributed training for billion-parameter models
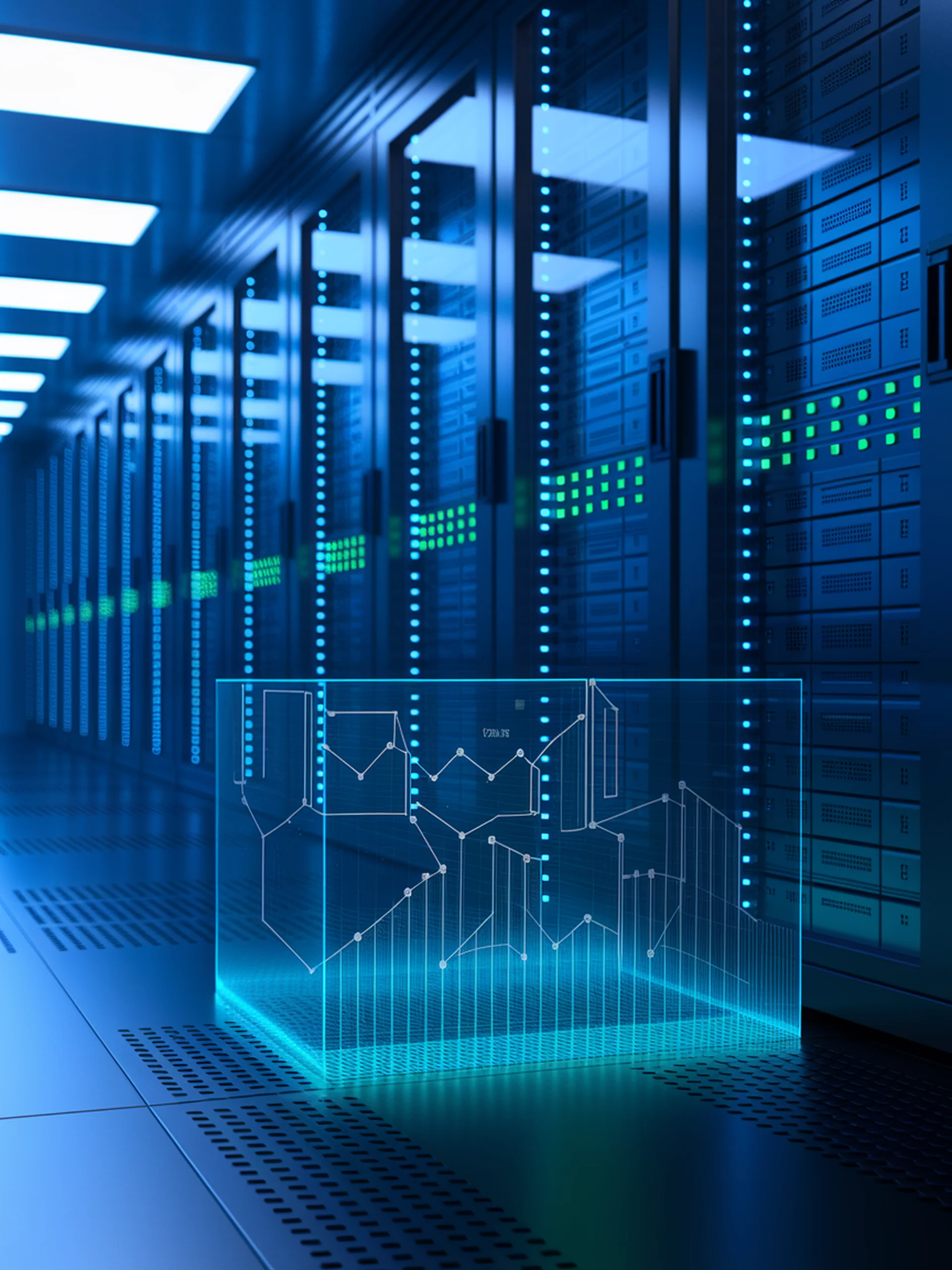
Optimizing LLM Cloud Services
A predictive framework for efficient LMaaS management
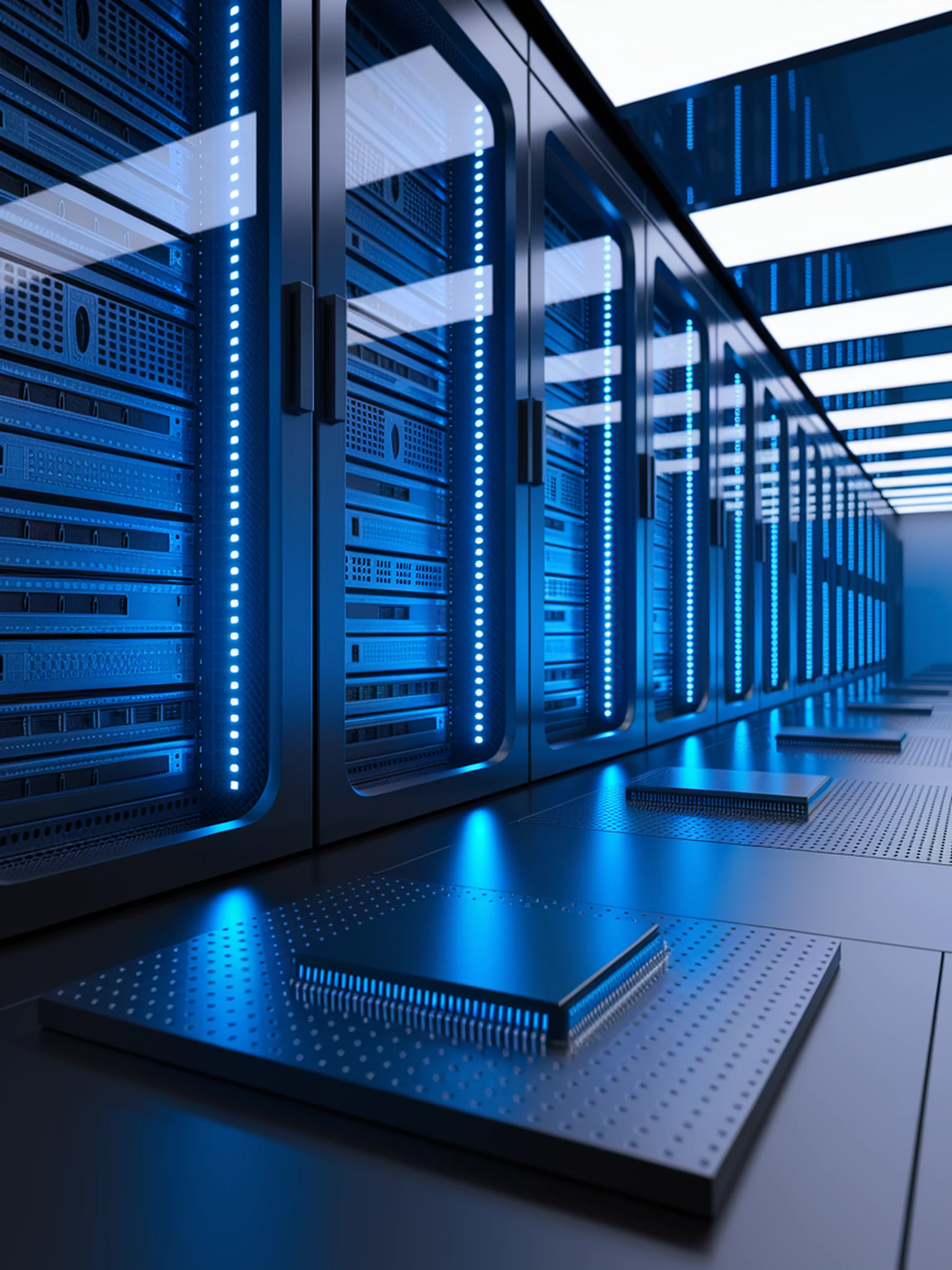
Efficient LLM Quantization
Faster, more flexible model optimization with less data
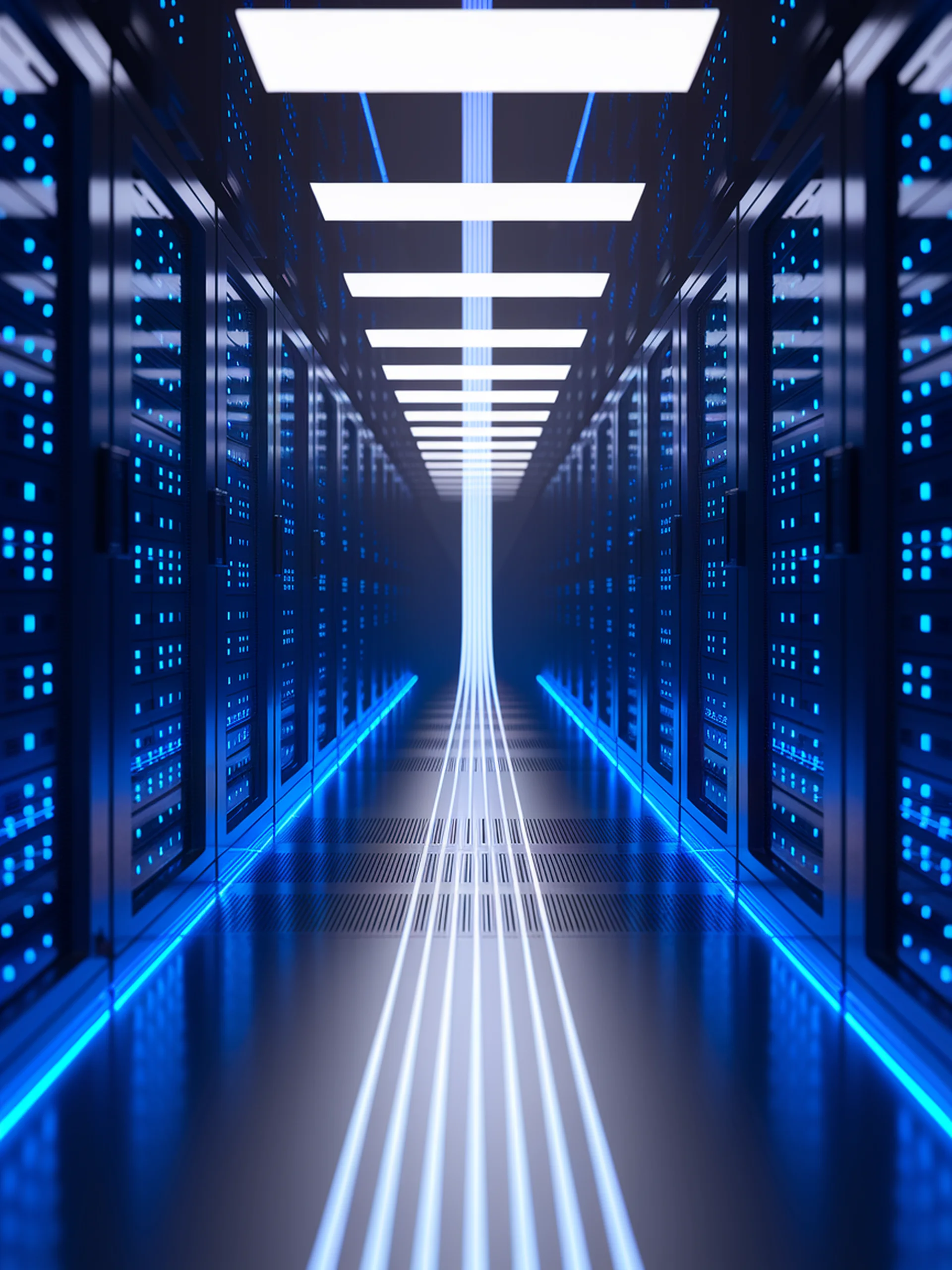
Accelerating LLM Inference with FlowKV
Eliminating bottlenecks in disaggregated inference systems
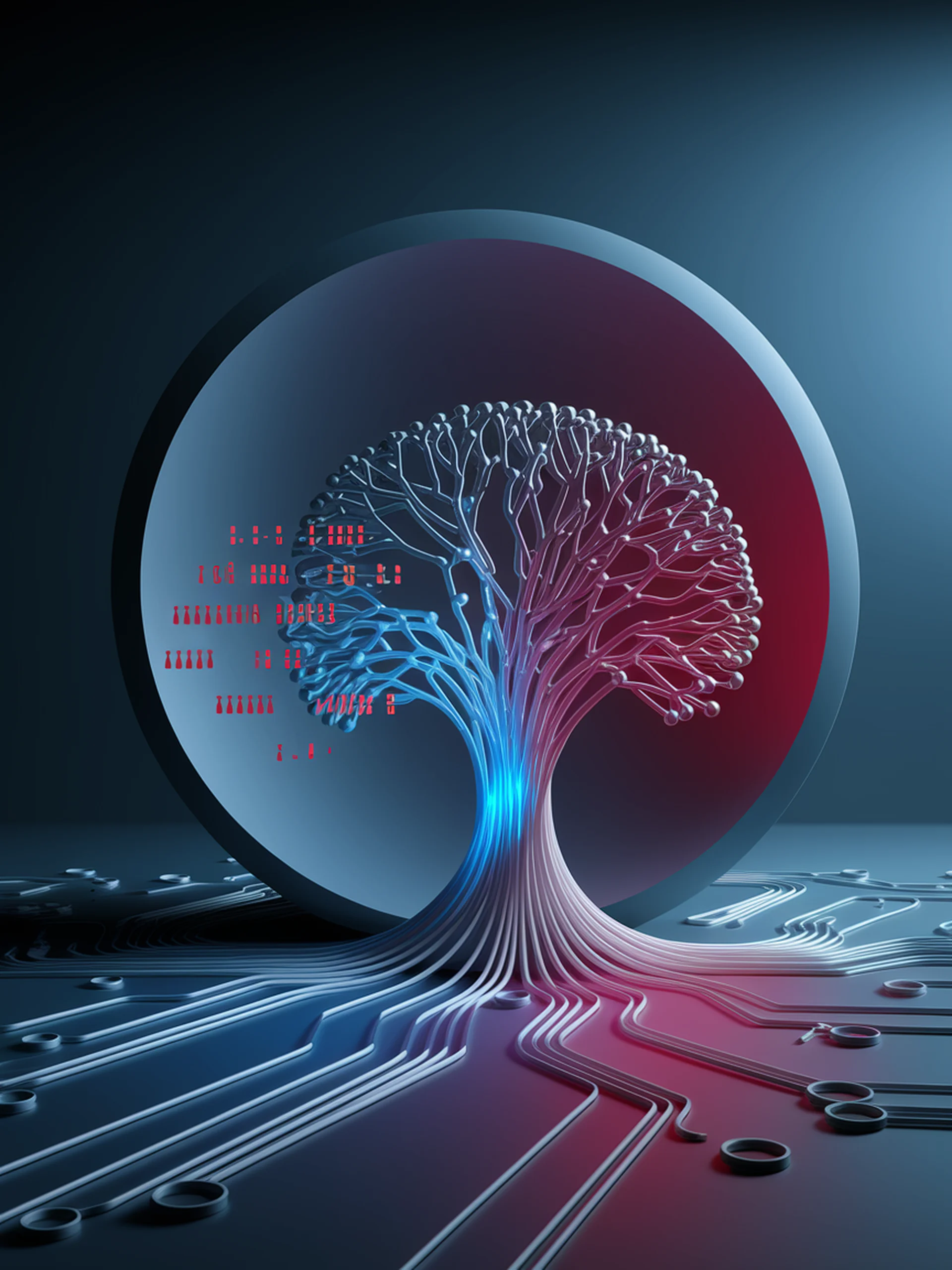
Smarter LLM Pruning with Entropy
Reducing model size while preserving performance
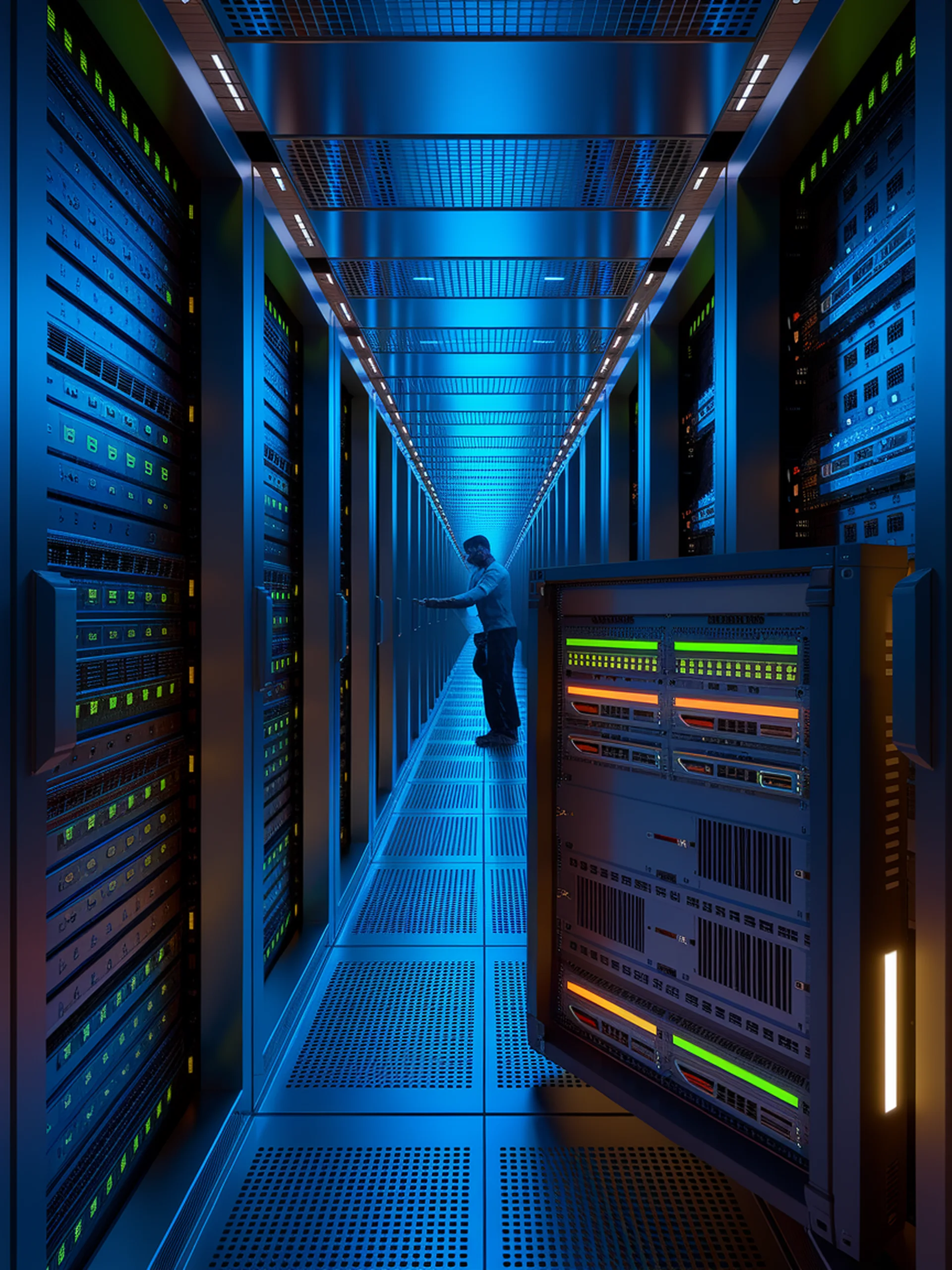
Optimizing MoE Training on Mixed GPU Clusters
A novel approach for efficient LLM training on heterogeneous hardware
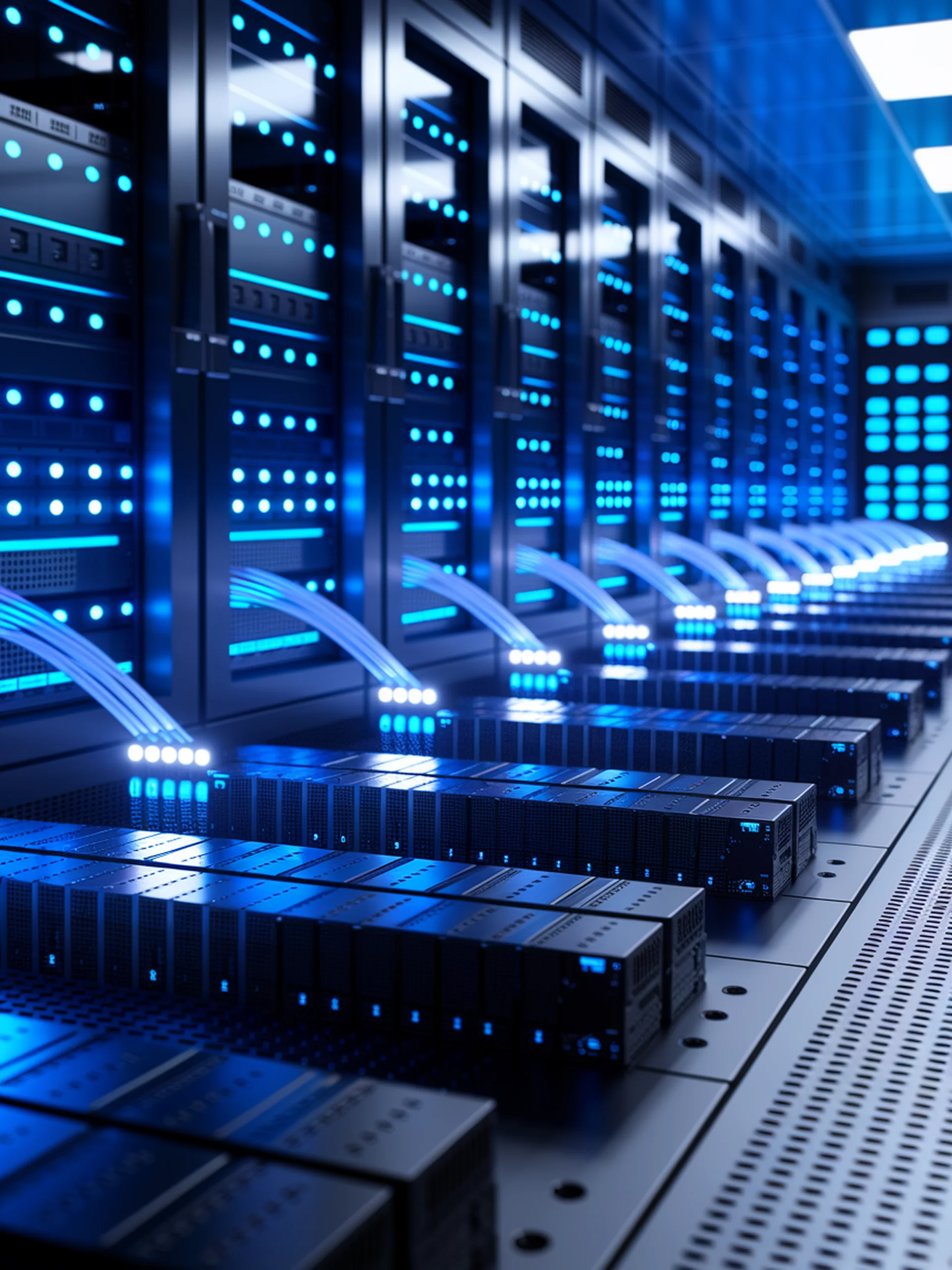
Accelerating LLM Inference with PipeDec
Pipeline-based architecture with dynamic speculative decoding for faster AI responses
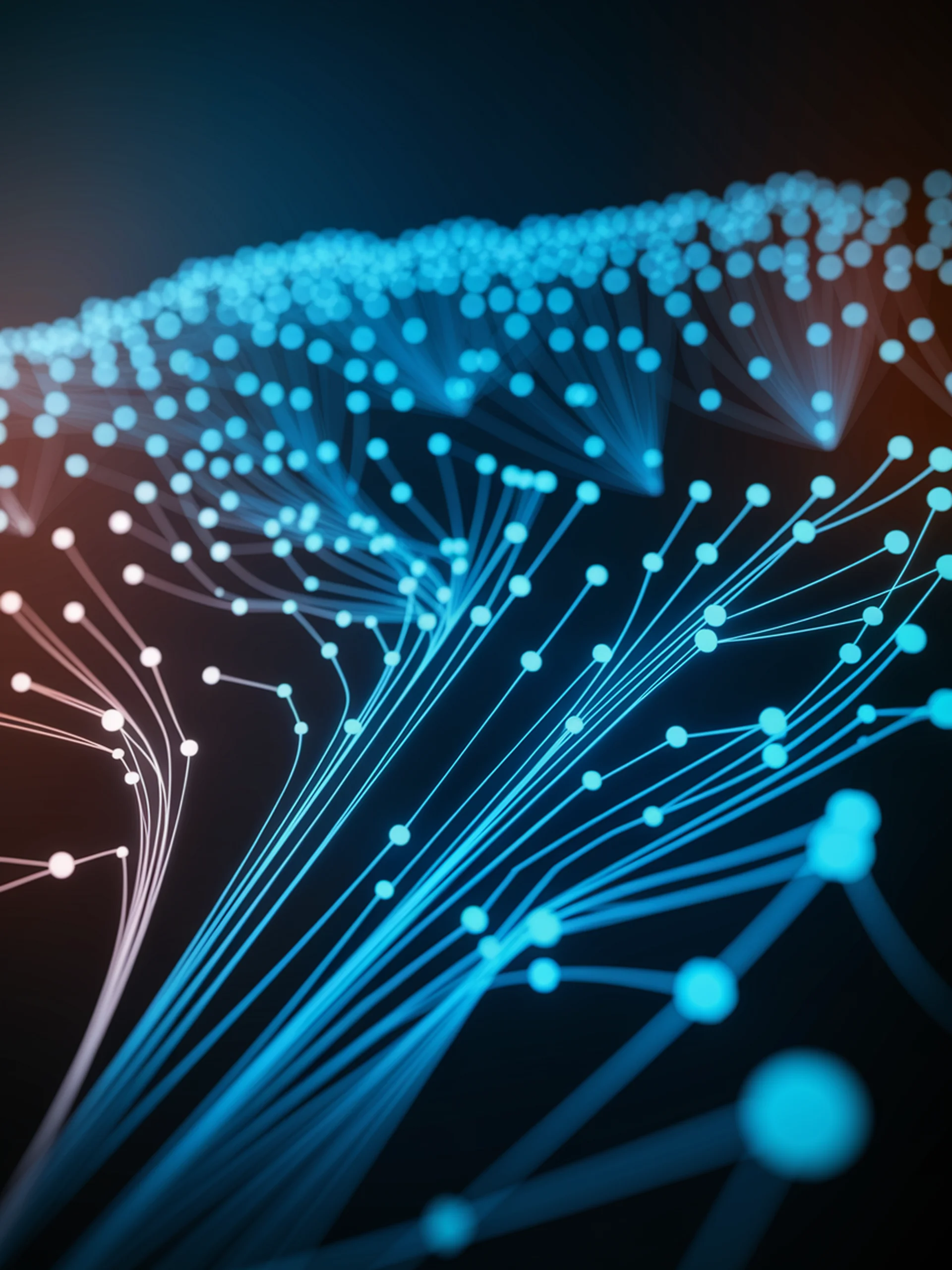
Accelerating LLMs with Smart Token Pruning
Using Saliency Analysis to Reduce Computational Complexity
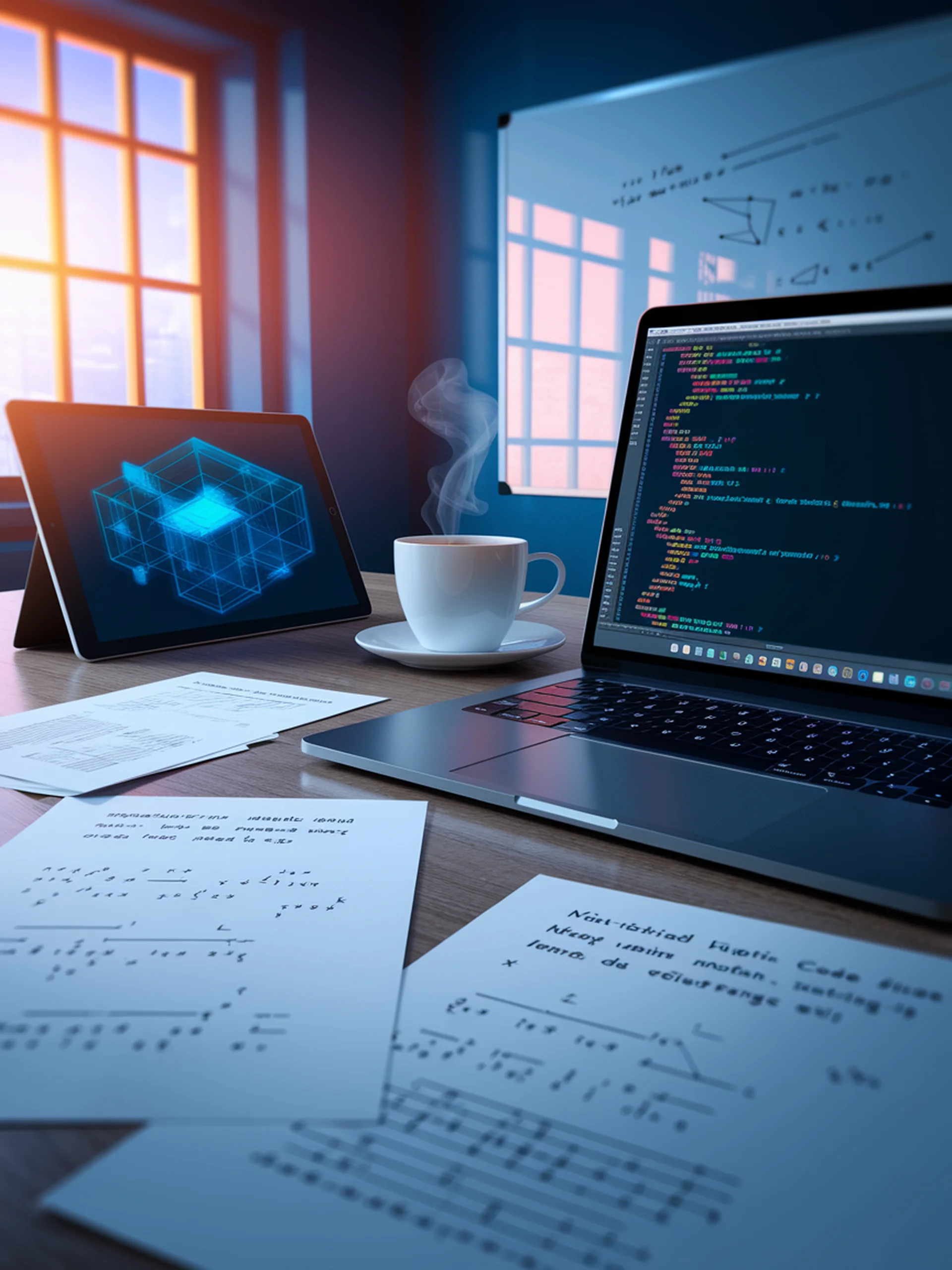
Computing the Hessian Matrix for LLMs
A practical approach to second-order derivatives in large language models
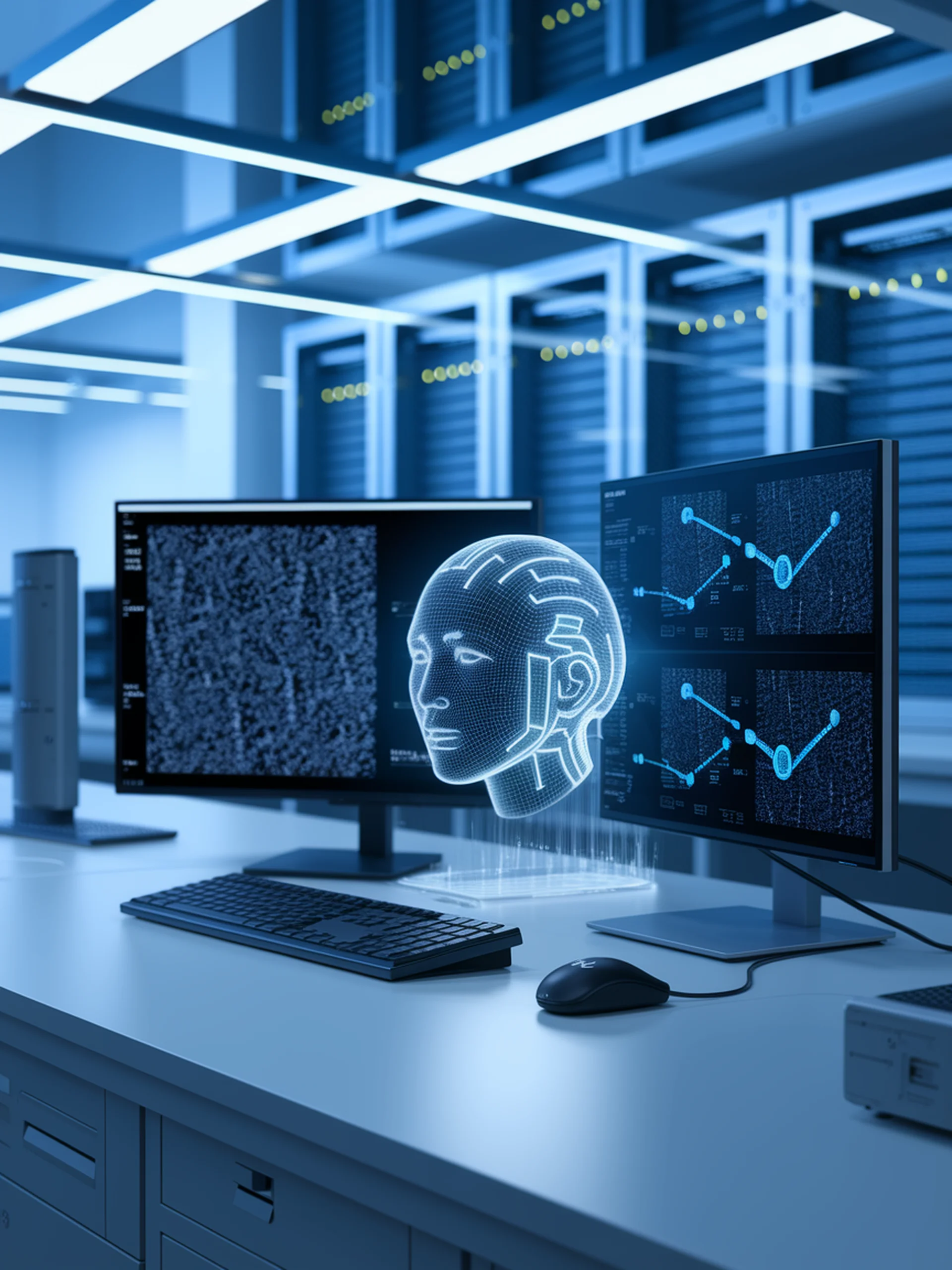
Efficient Visual Intelligence with LEO-MINI
Boosting multimodal LLM efficiency through smart token reduction
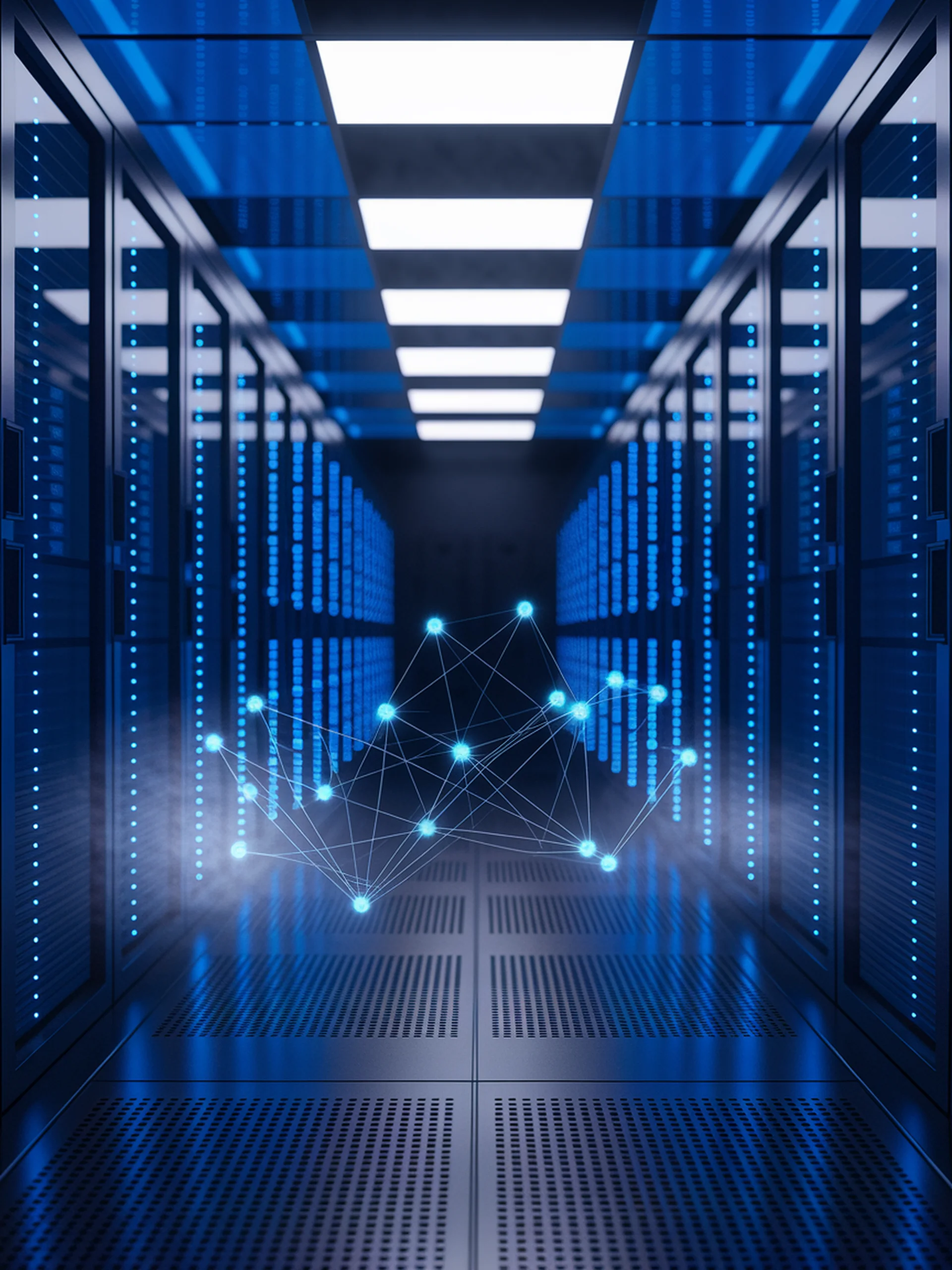
Smart KV Cache Optimization
Using lag-relative information to identify important tokens
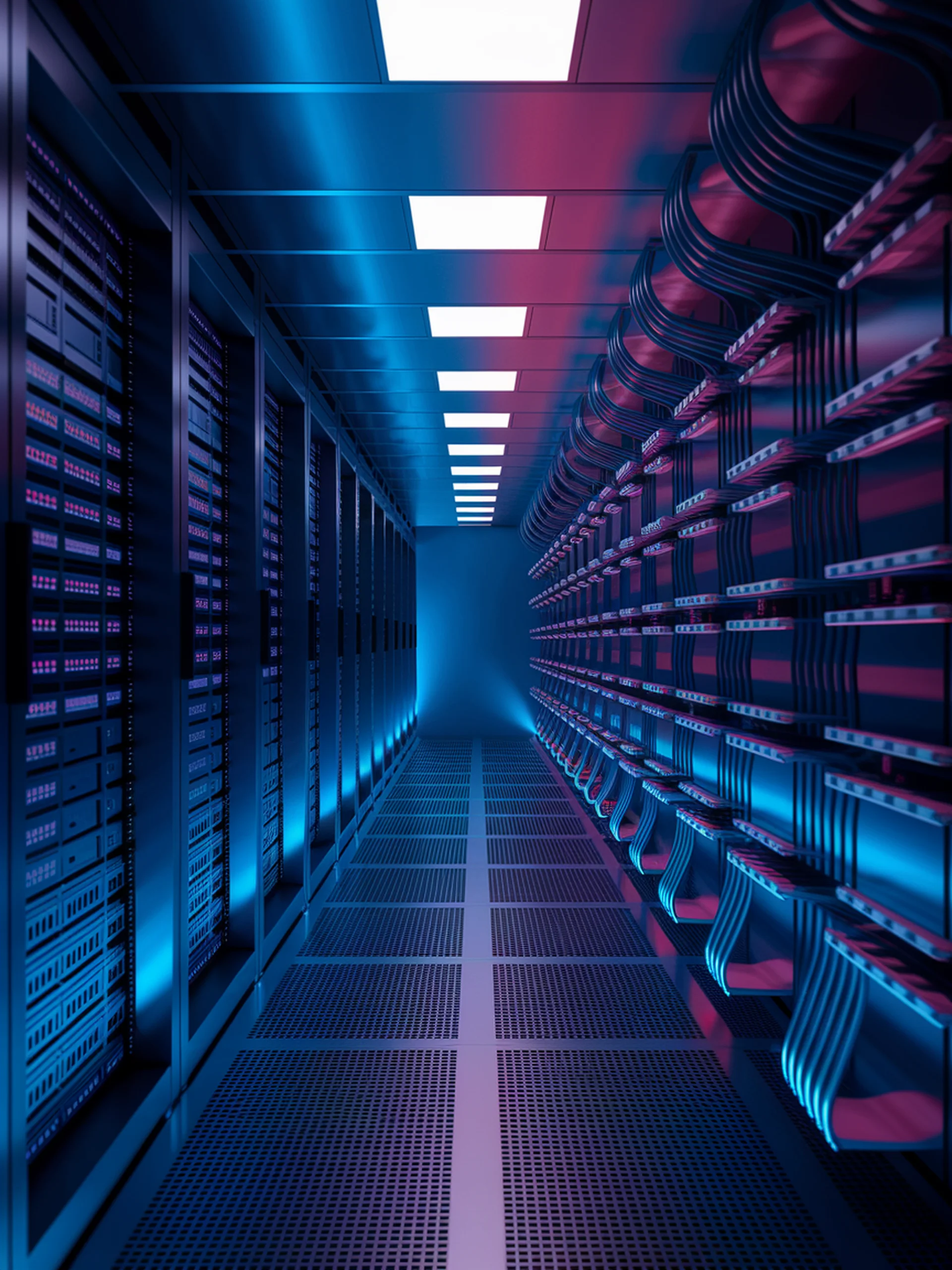
Thanos: Efficient LLM Compression
A block-wise pruning approach that maintains accuracy while reducing model size
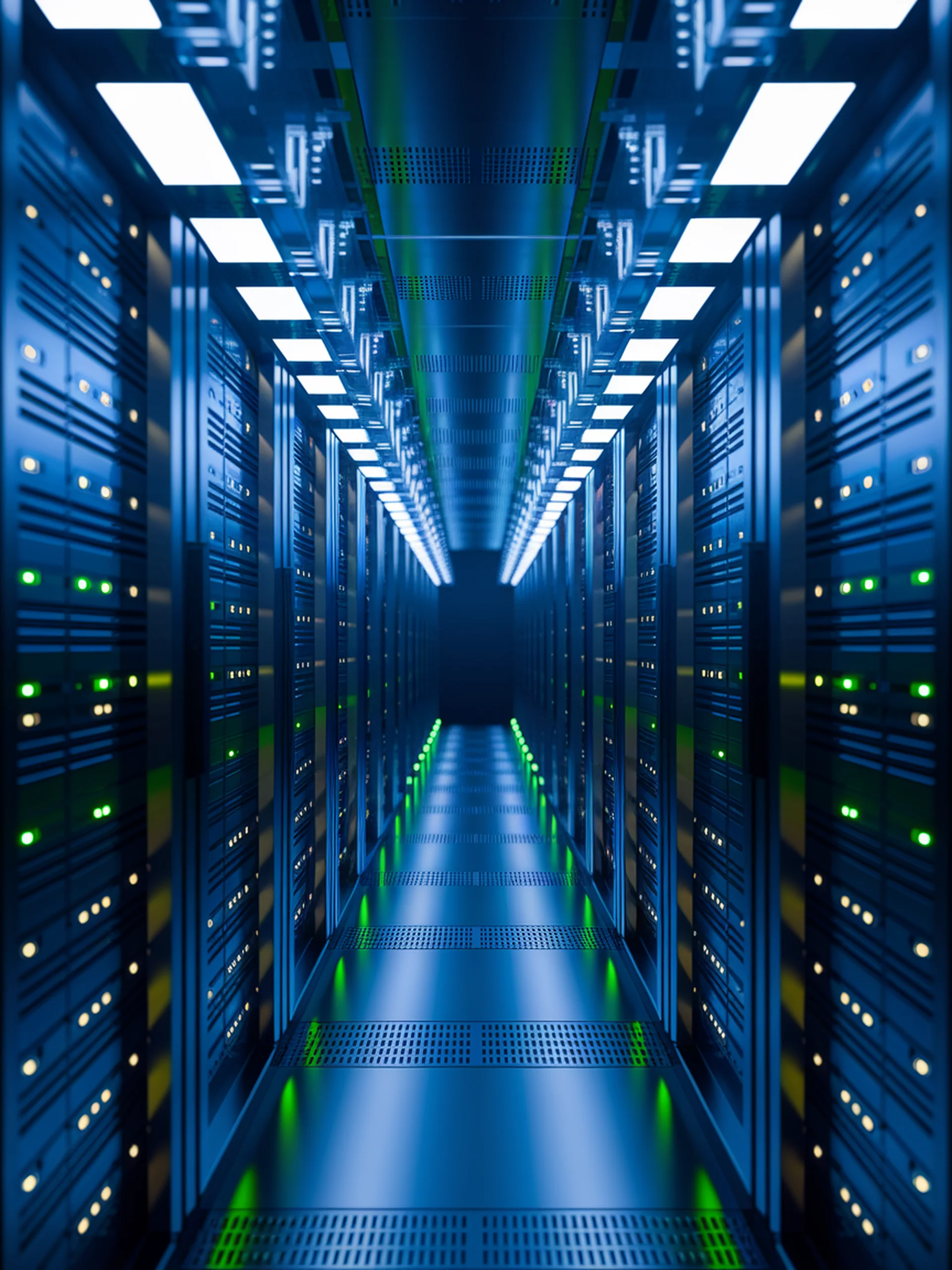
Accelerating LLM Training
Novel gradient compression for transformer-based models
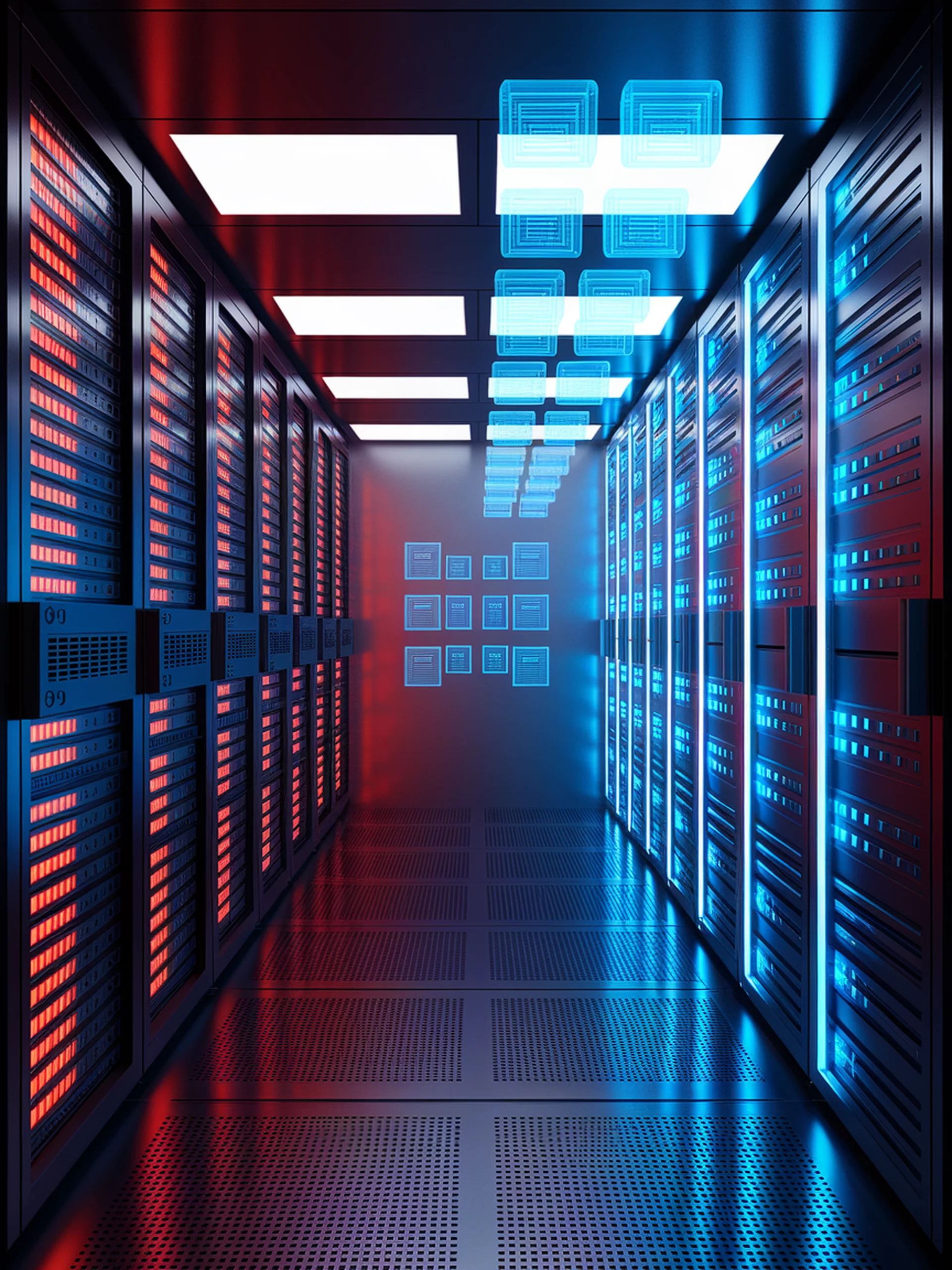
Bridging the Gap: Encoder-Decoder Gemma
Converting decoder-only LLMs for better efficiency-quality balance
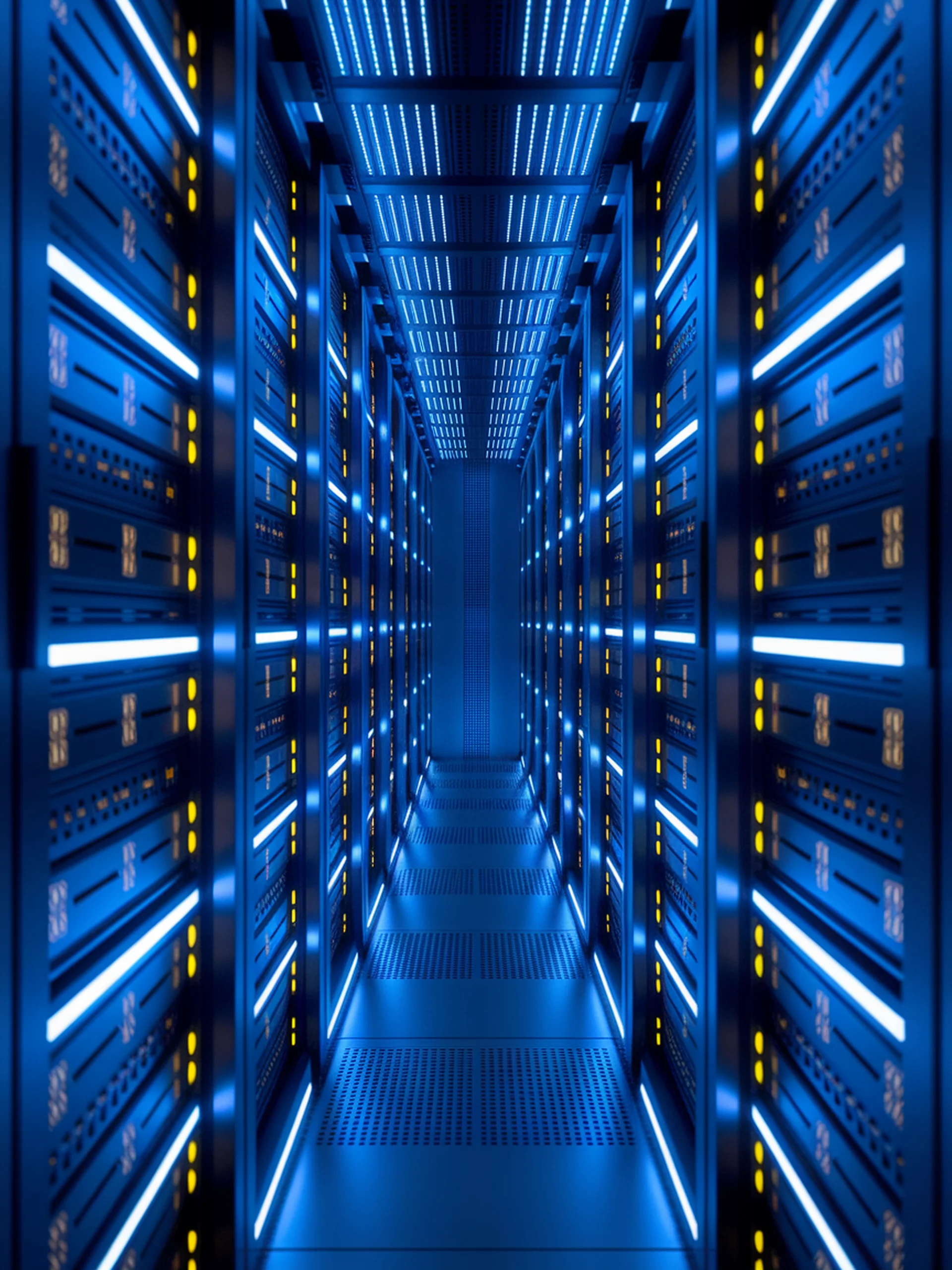
Accelerating LLM Generation Through Parallelization
How Concurrent Attention Enables Faster Large Language Model Performance
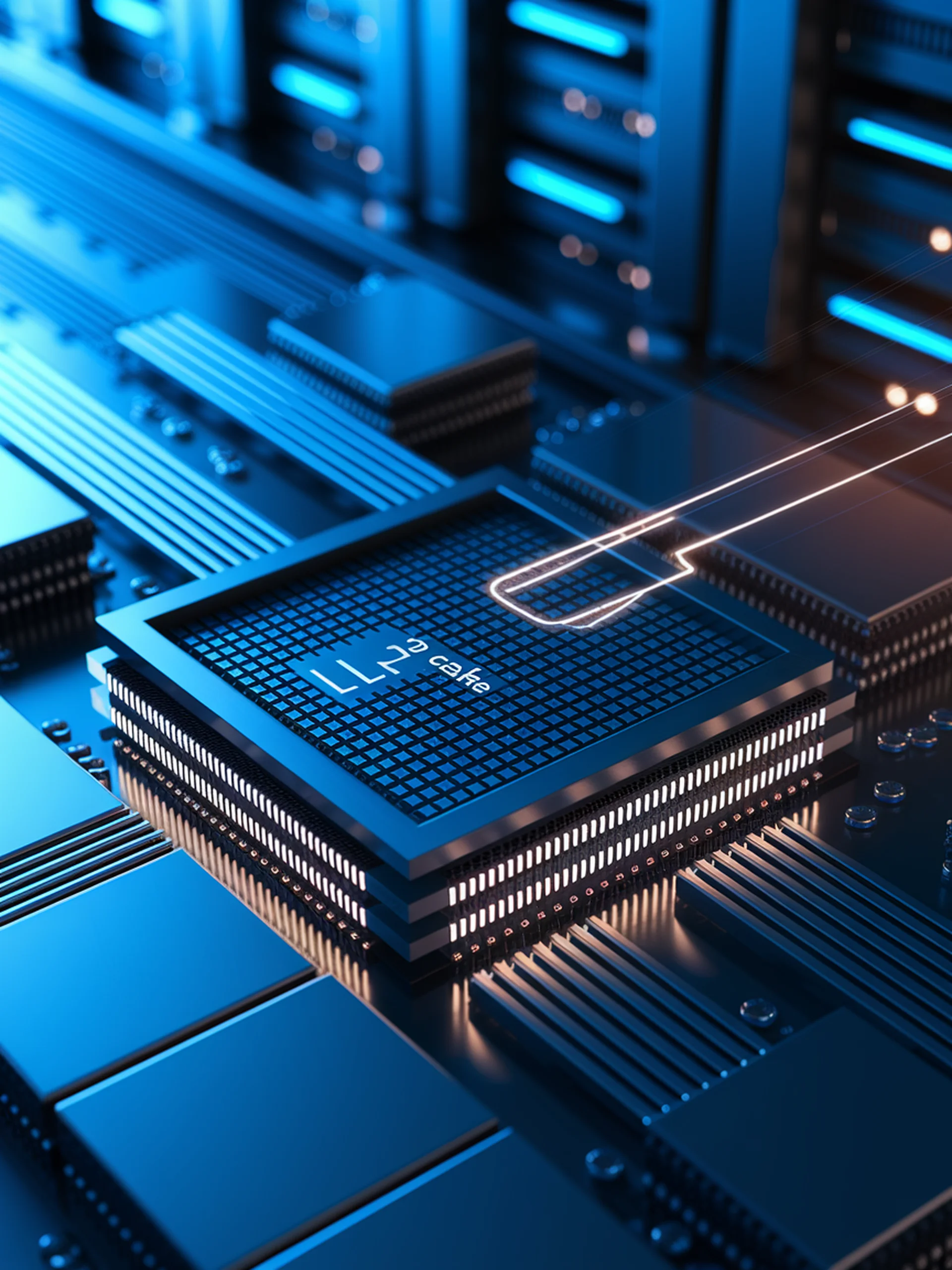
Breaking the LLM Inference Bottleneck
Boosting throughput with asynchronous KV cache prefetching
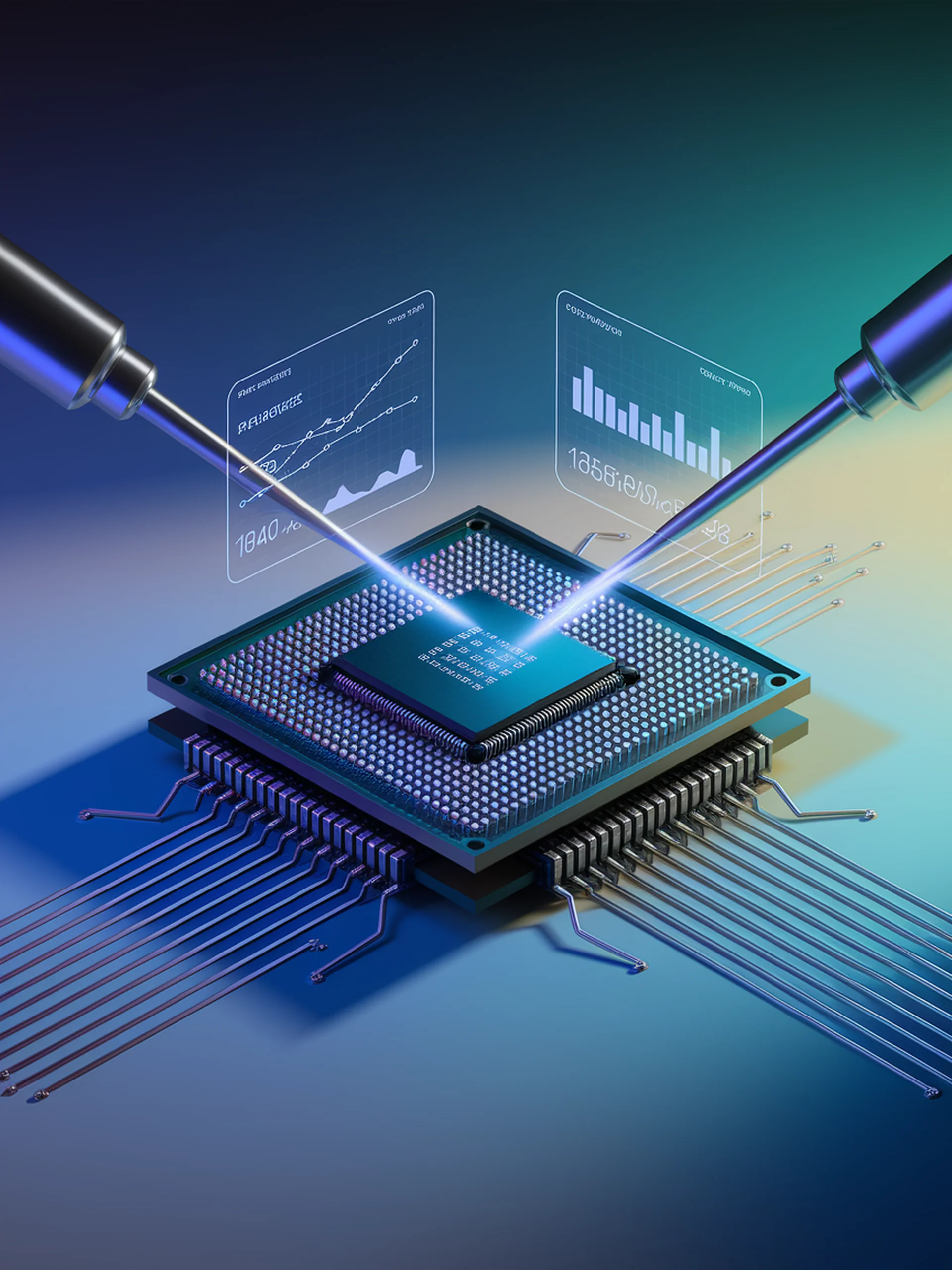
Smarter LLM Pruning for Resource Efficiency
A fine-grained approach that preserves model quality
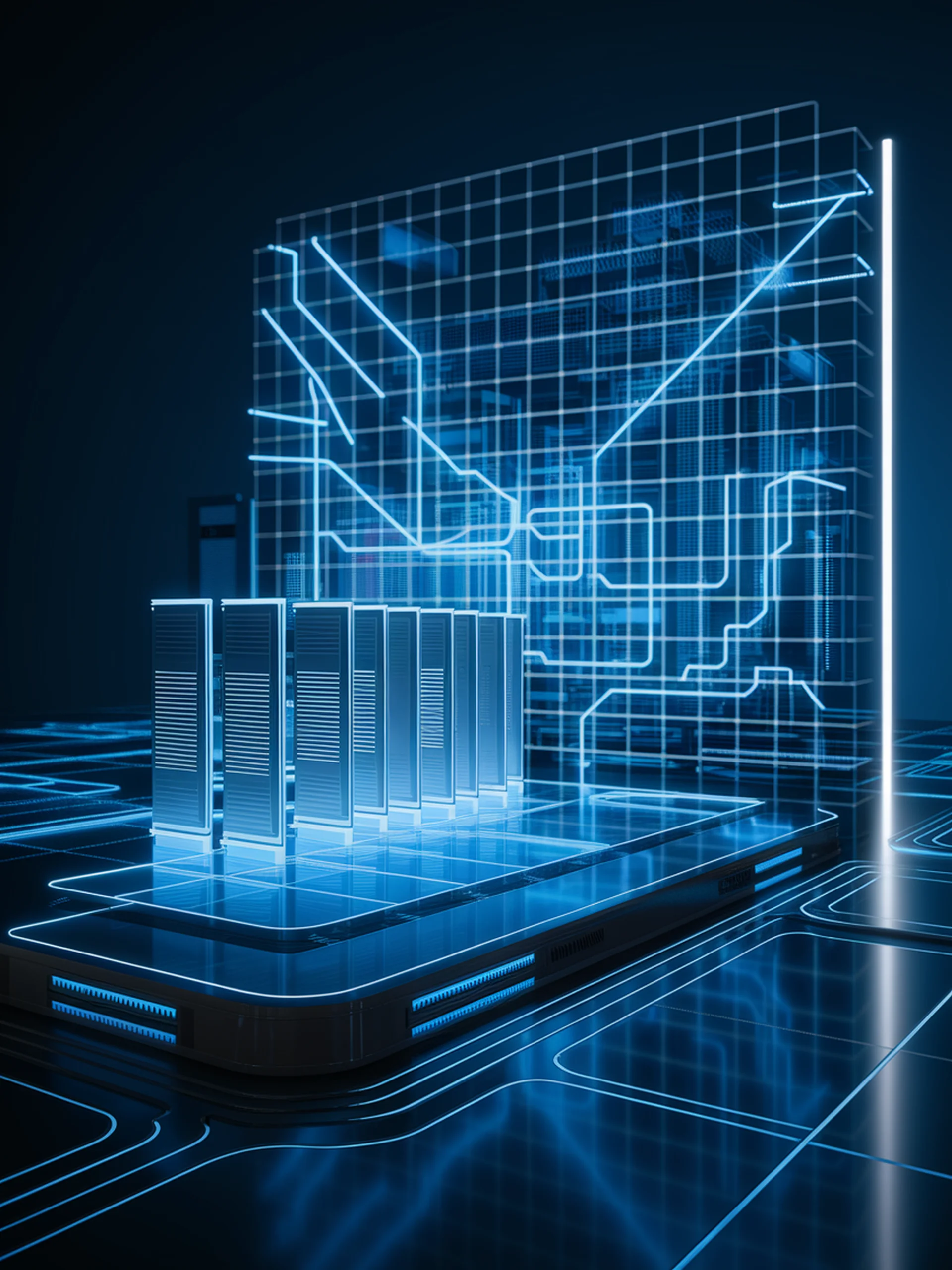
OSCAR: Smarter RAG Compression
Enhancing LLM efficiency without sacrificing performance
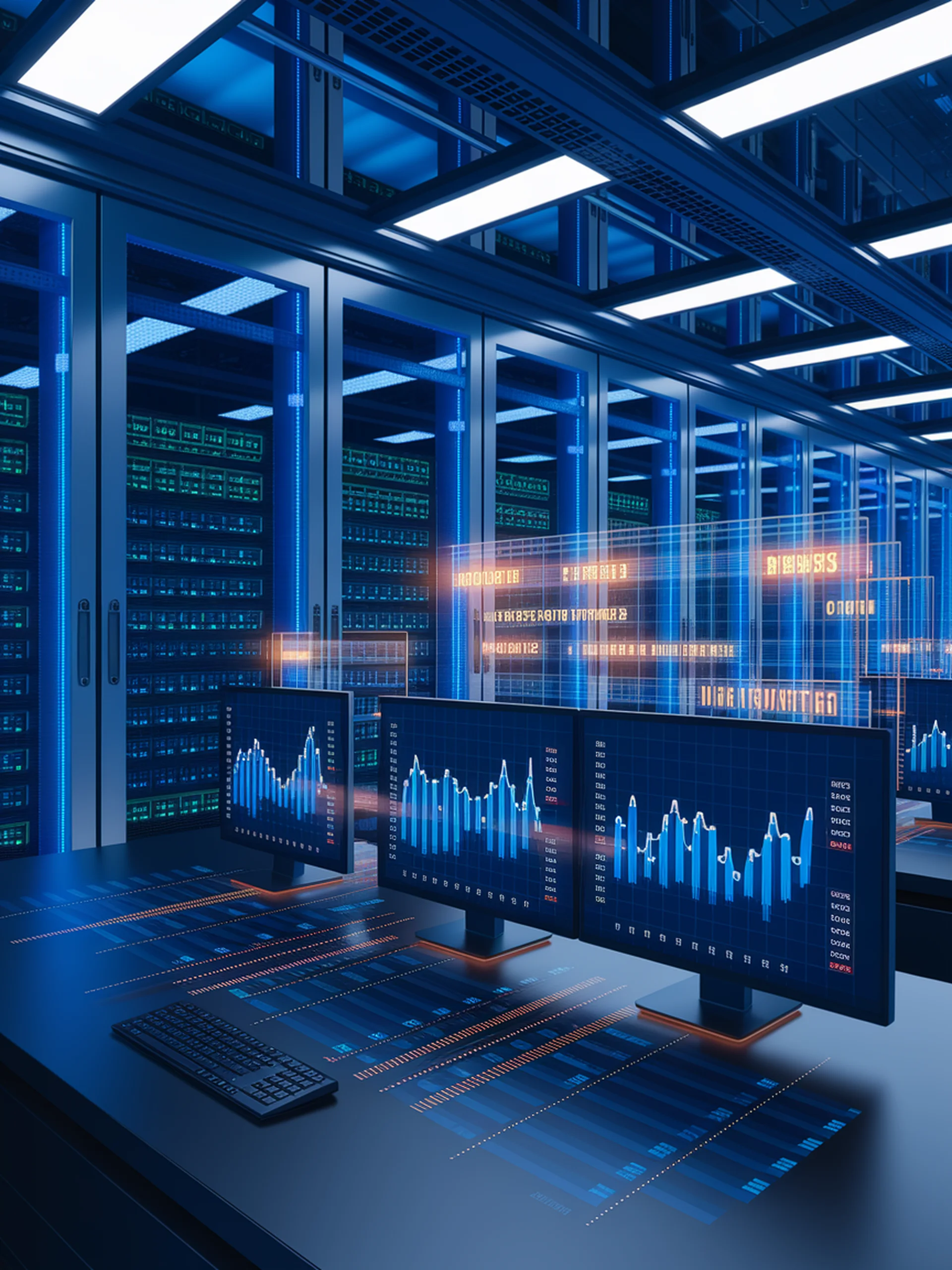
Optimizing LLM Inference Systems
Mathematical queuing models for maximizing LLM throughput
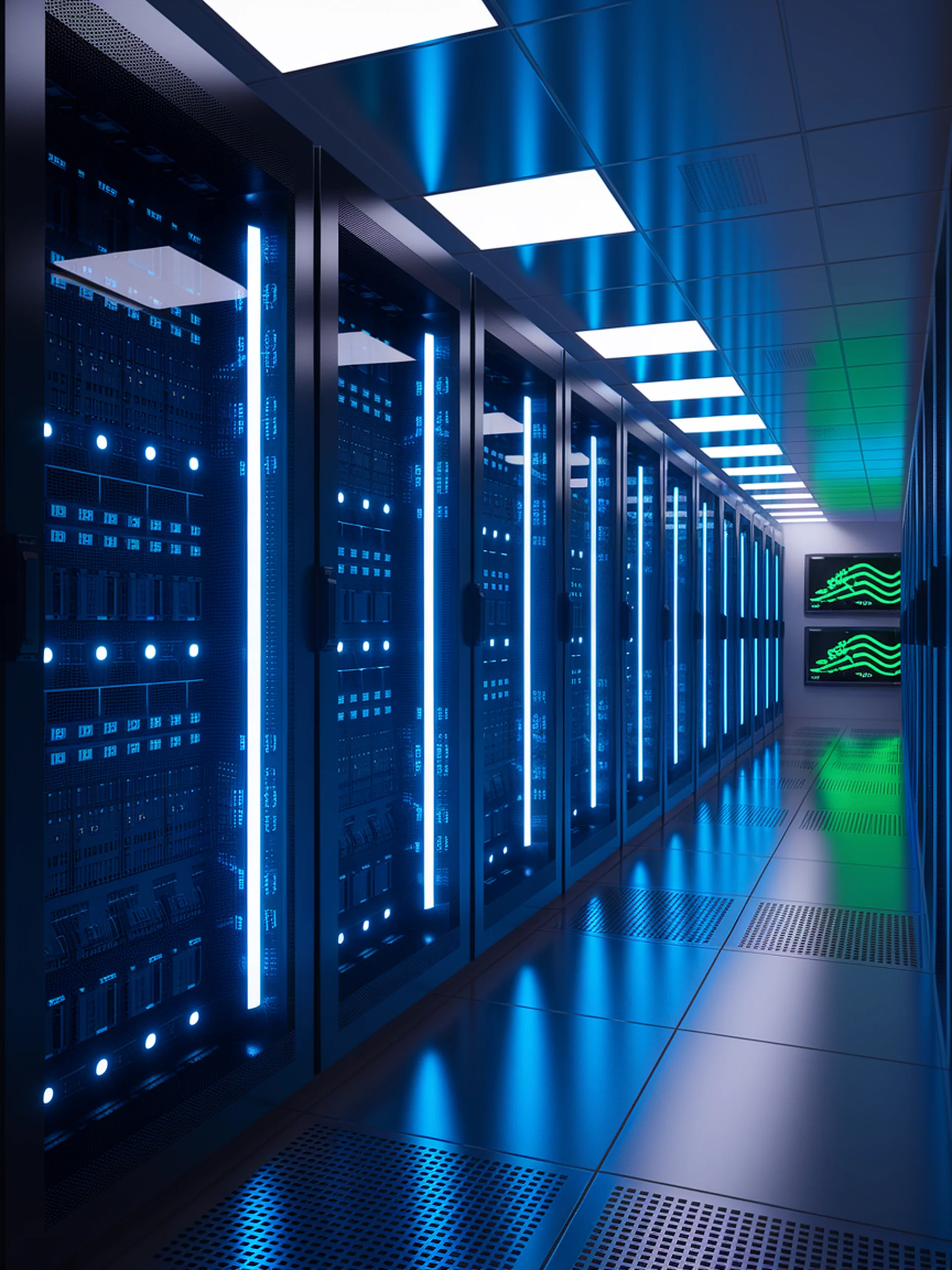
Optimizing LLM Inference at Scale
Adaptive scheduling for faster, more efficient LLM serving
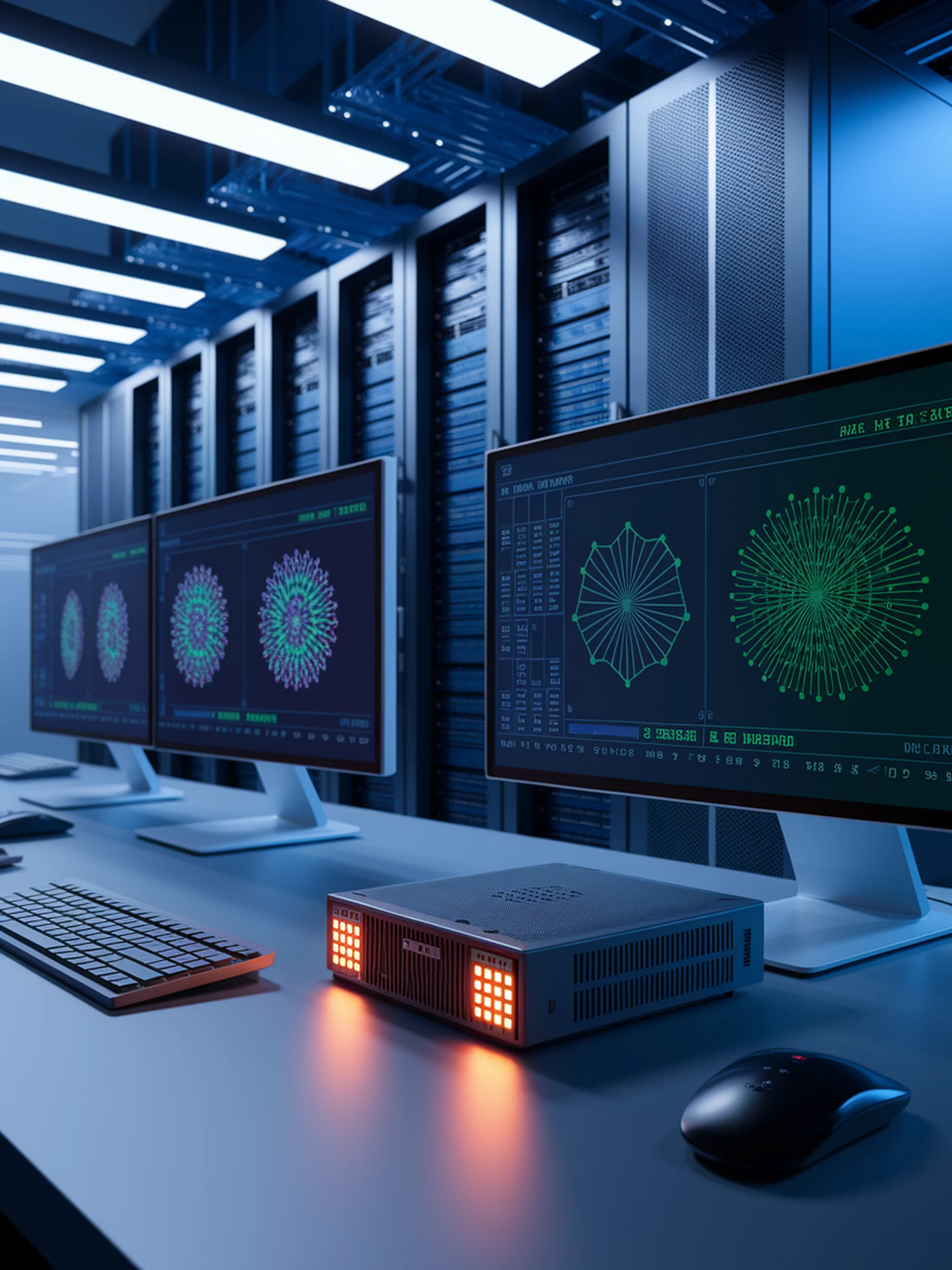
Smarter AI, Smaller Footprint
Strategic Expert Pruning for More Efficient Language Models
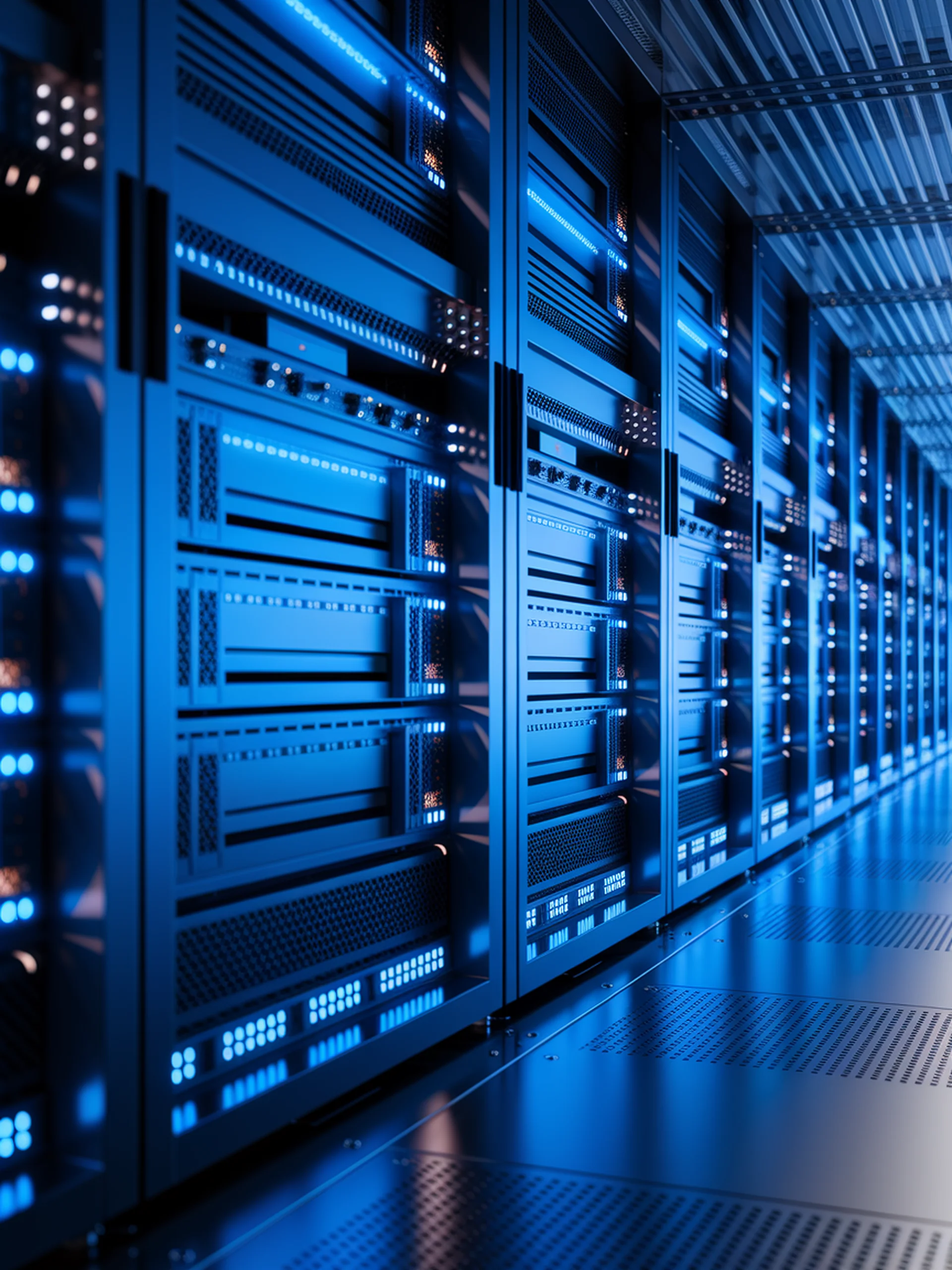
Engineering the Next-Gen LLM Infrastructure
Optimizing 135B-parameter models for Ascend NPUs
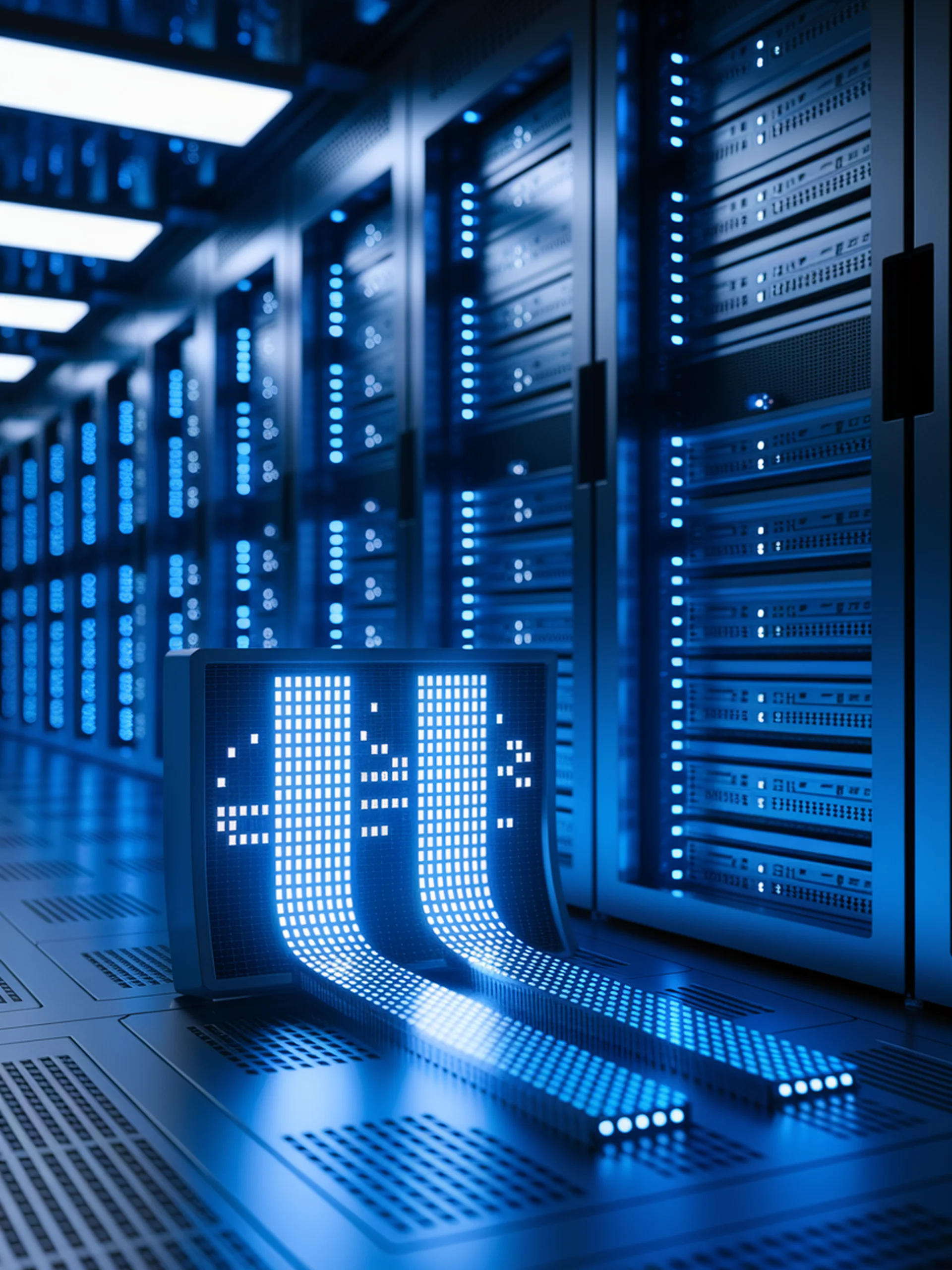
Optimizing LLM Performance with SLOs-Serve
Intelligent token allocation for multi-stage LLM requests
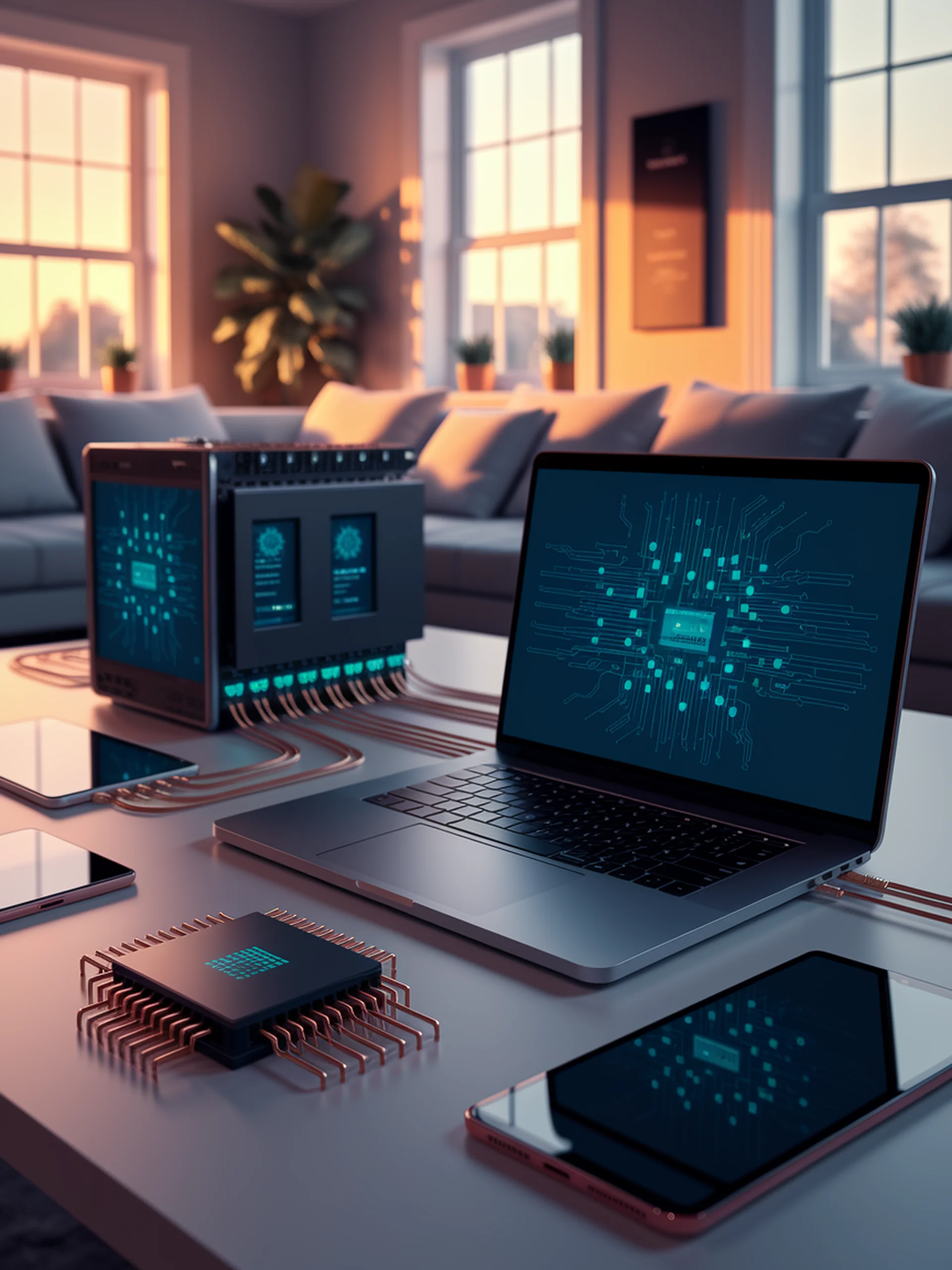
Democratizing LLM Inference
Running 70B-scale models on everyday home devices
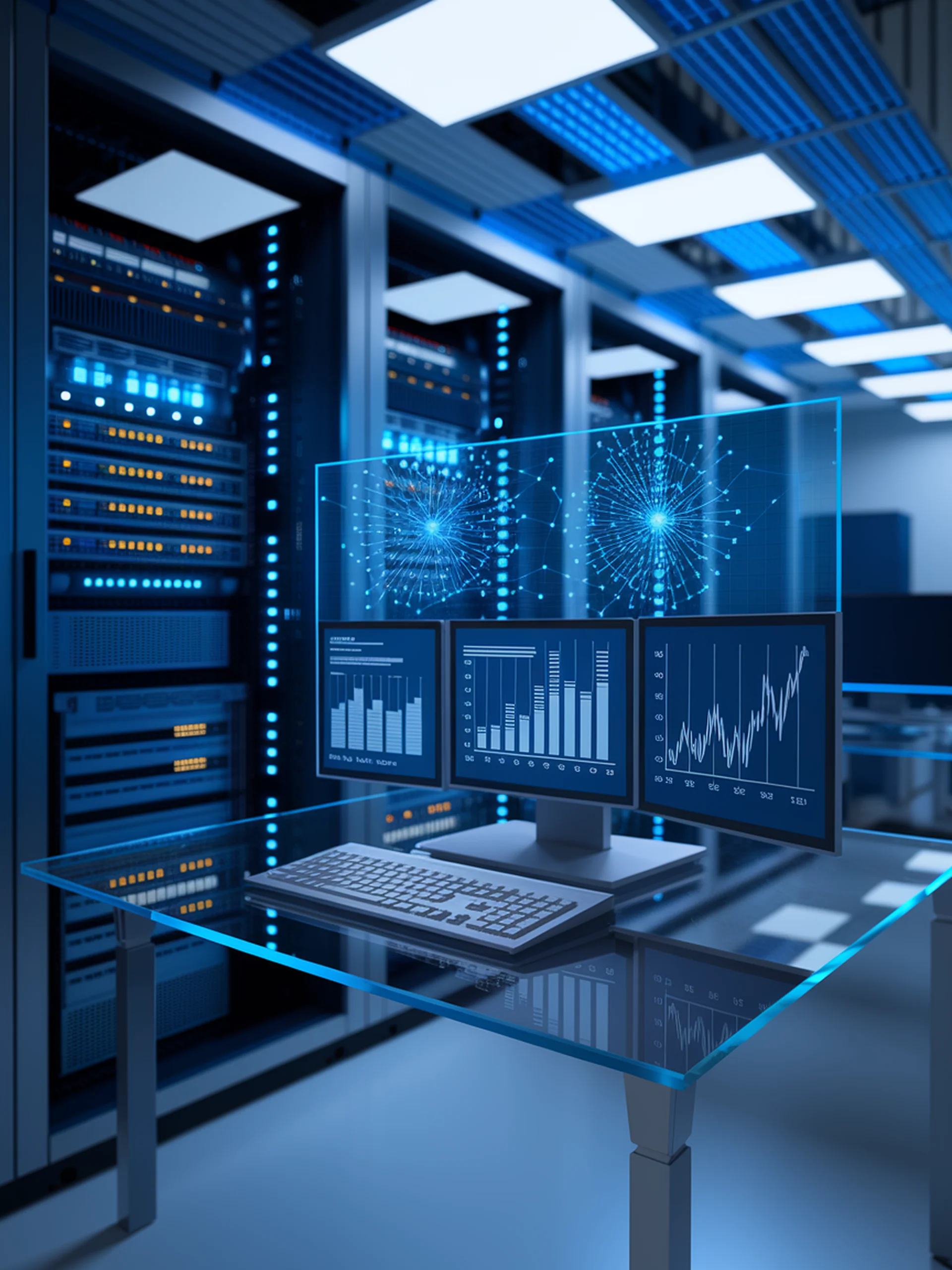
Faster, Smaller, Smarter LLMs
Boosting LLM efficiency with self-distilled sparse drafting
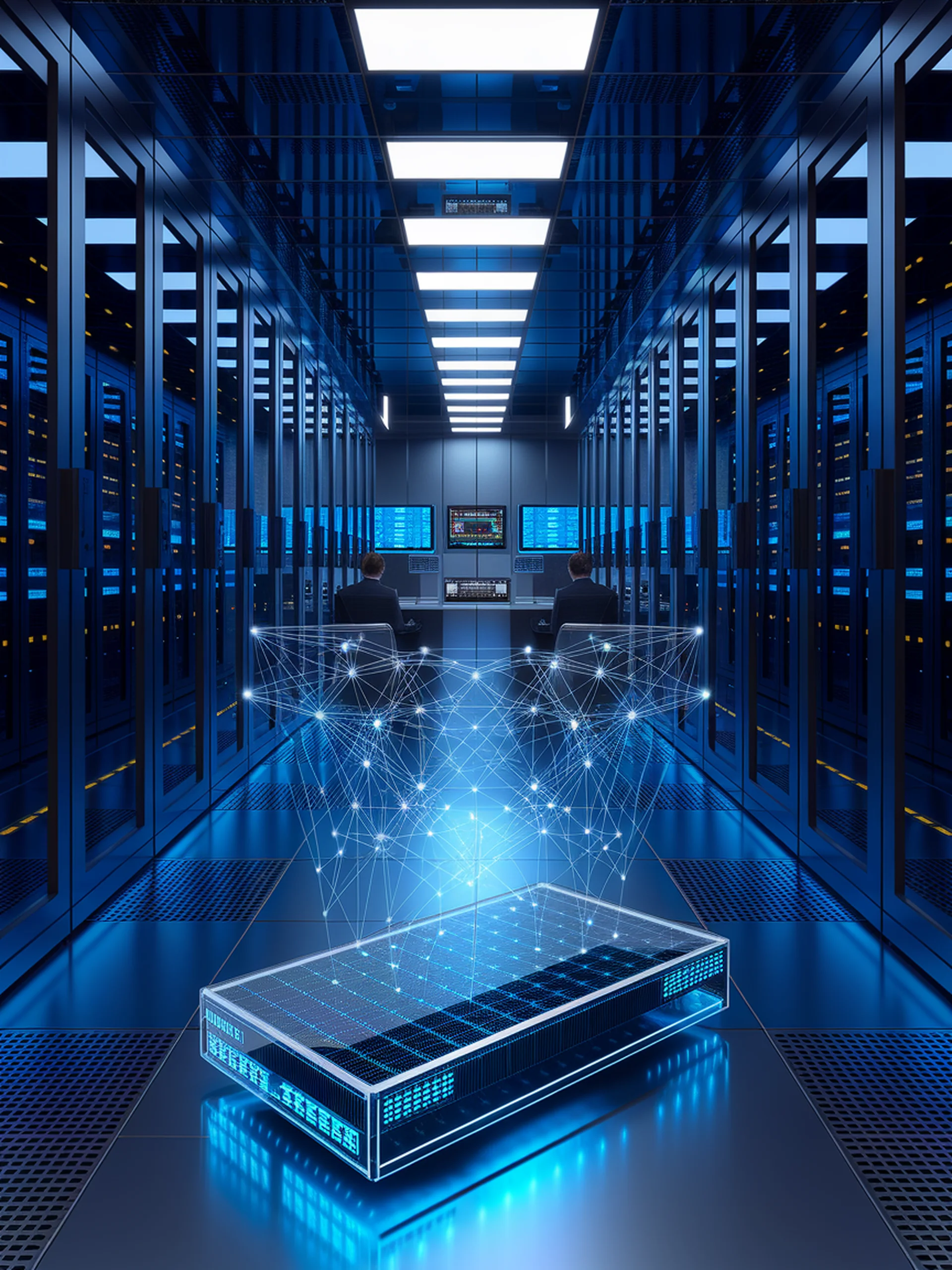
Accelerating LLM Inference with SpecEE
Faster language model processing through speculative early exiting
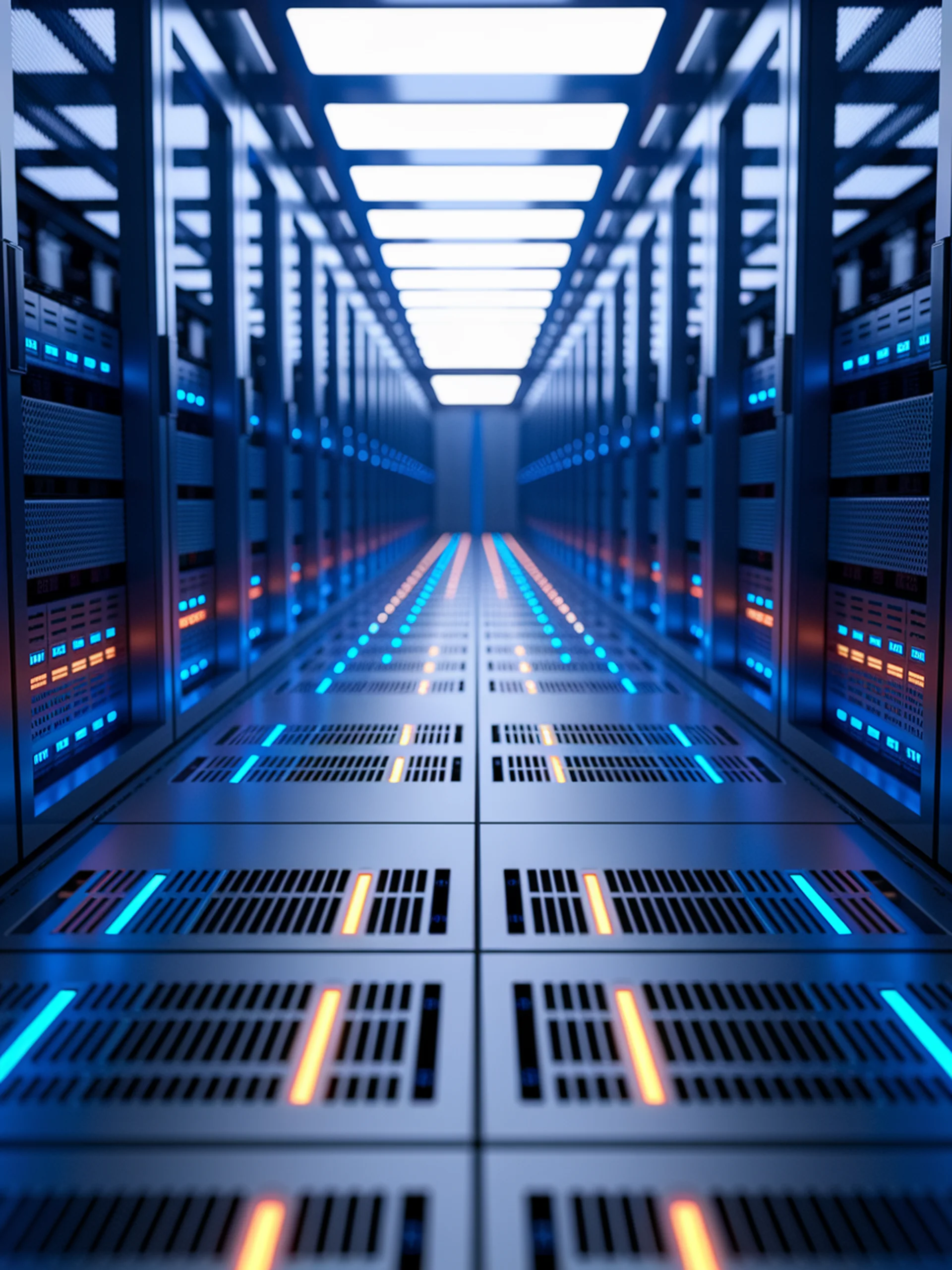
Accelerating RAG with Smart Vector Partitioning
Optimizing CPU-GPU memory usage for faster retrieval in RAG systems
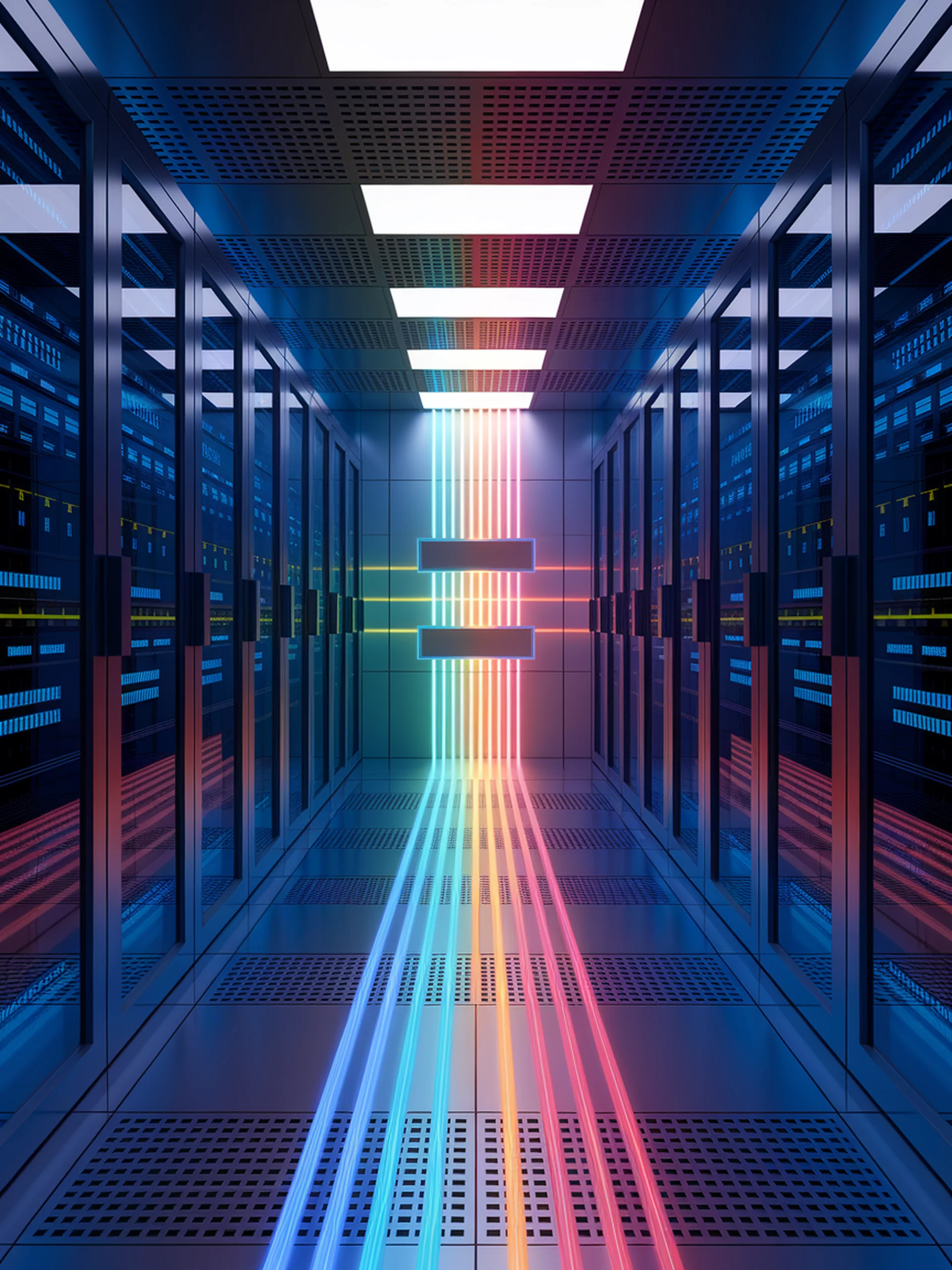
Bridging the Gap for LLM Tool Access
A secure RESTful proxy for Model Context Protocol deployment across platforms
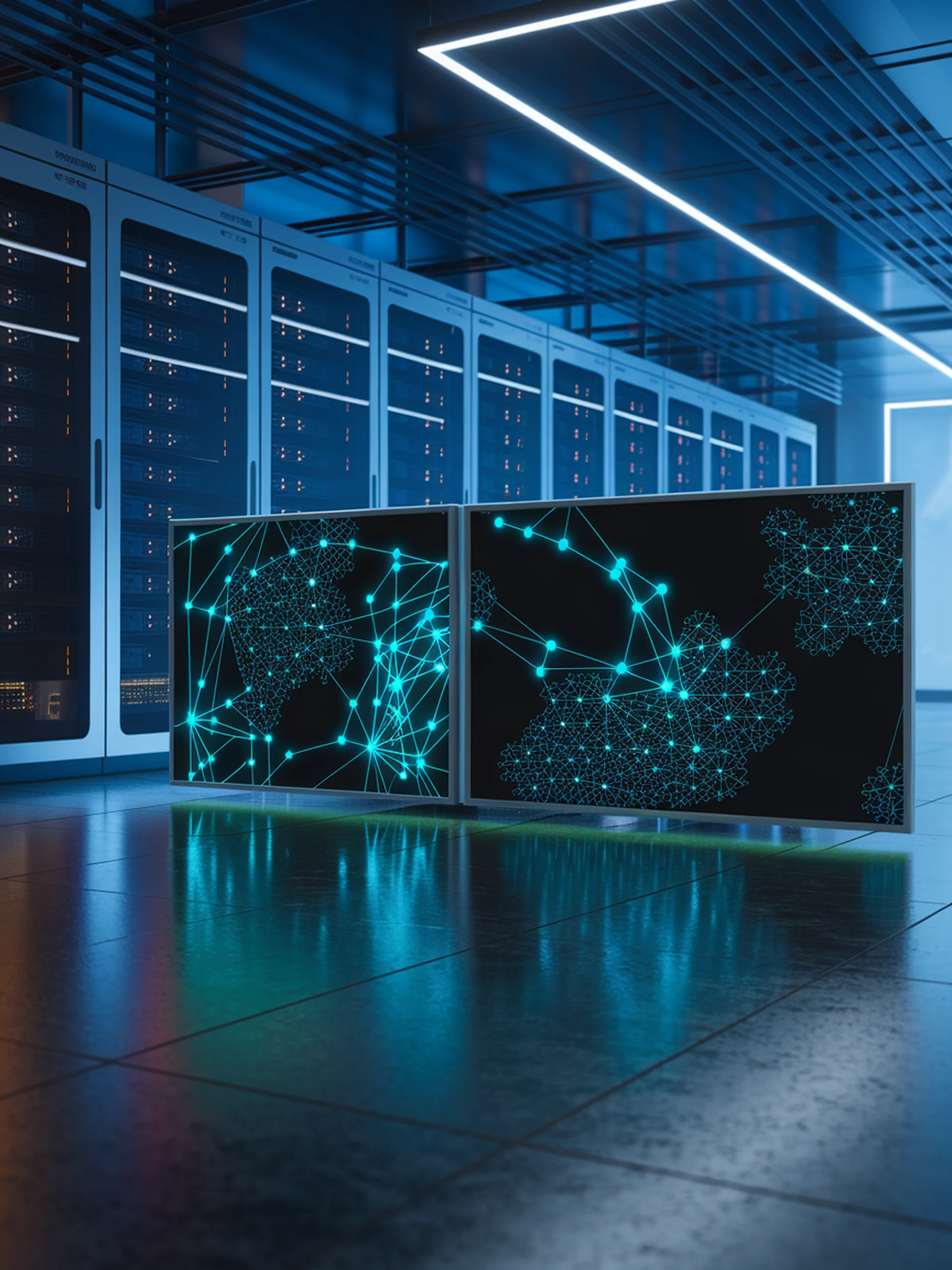
Unbiased Constrained Decoding
A more efficient approach for controlling LLM outputs
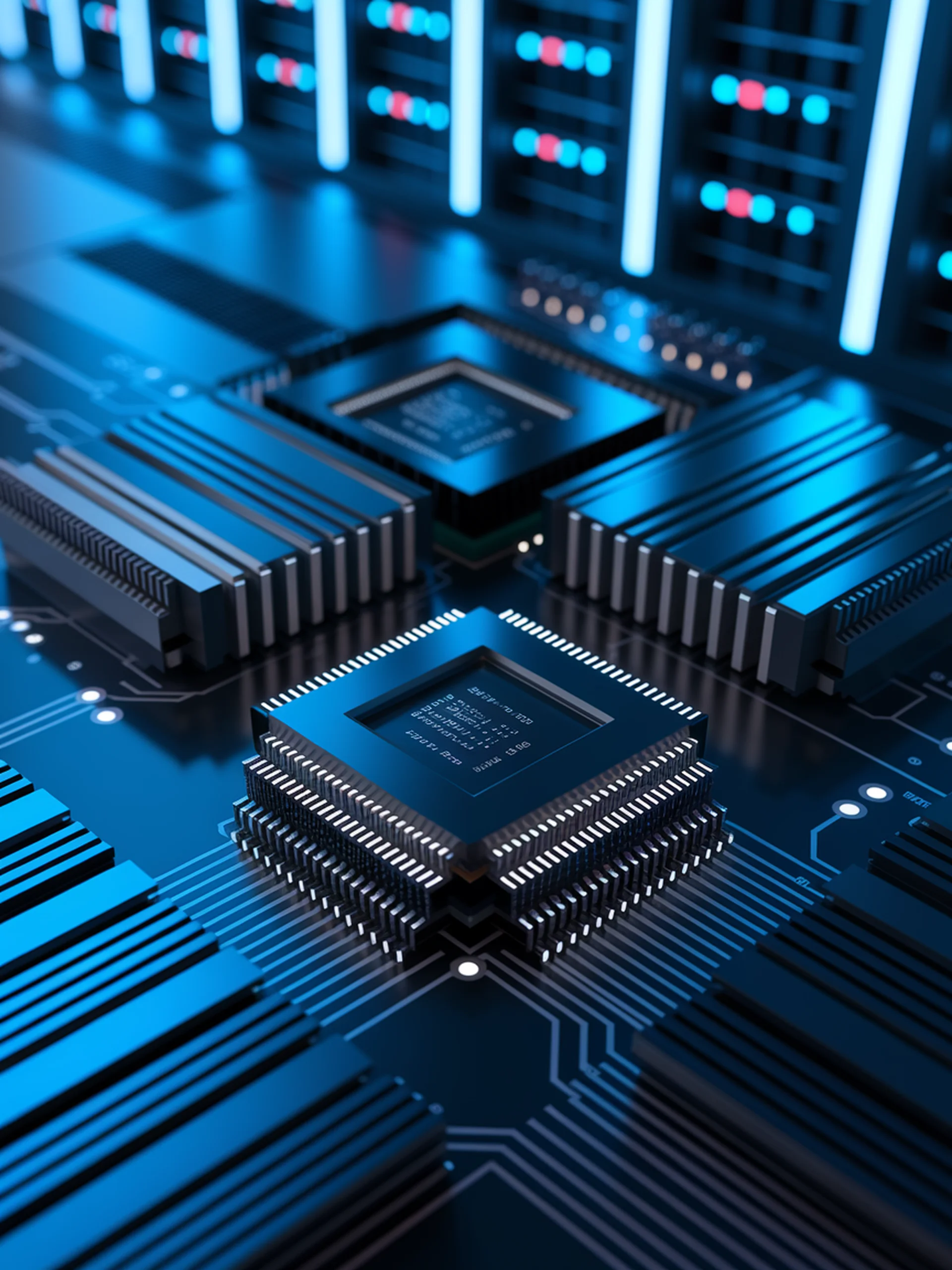
Efficient LLM Inference Through Smart Quantization
A novel approach combining low-rank decomposition with quantization-aware training
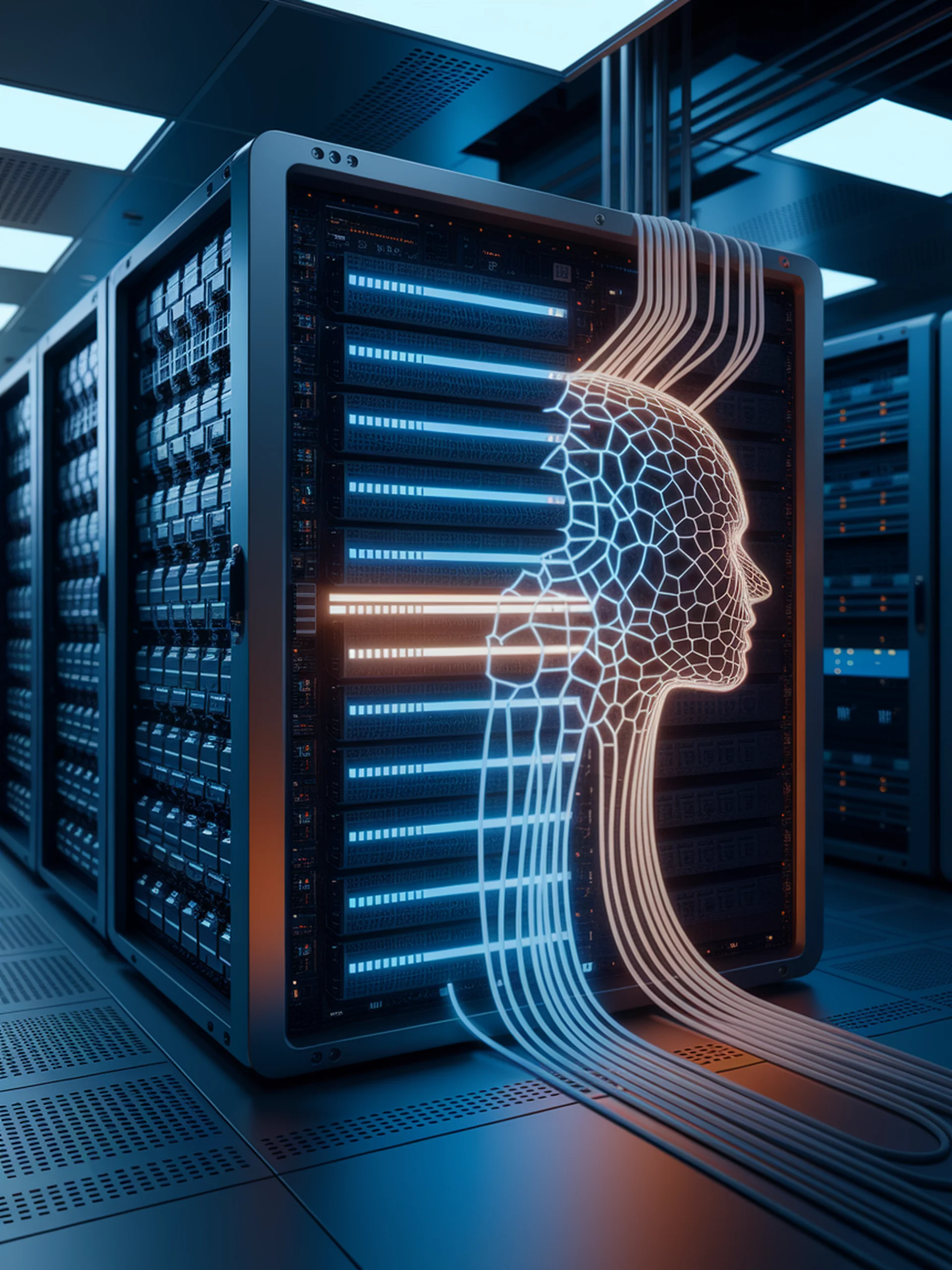
Solving Memory Bottlenecks in Visual AI
Head-Aware Compression for Efficient Visual Generation Models
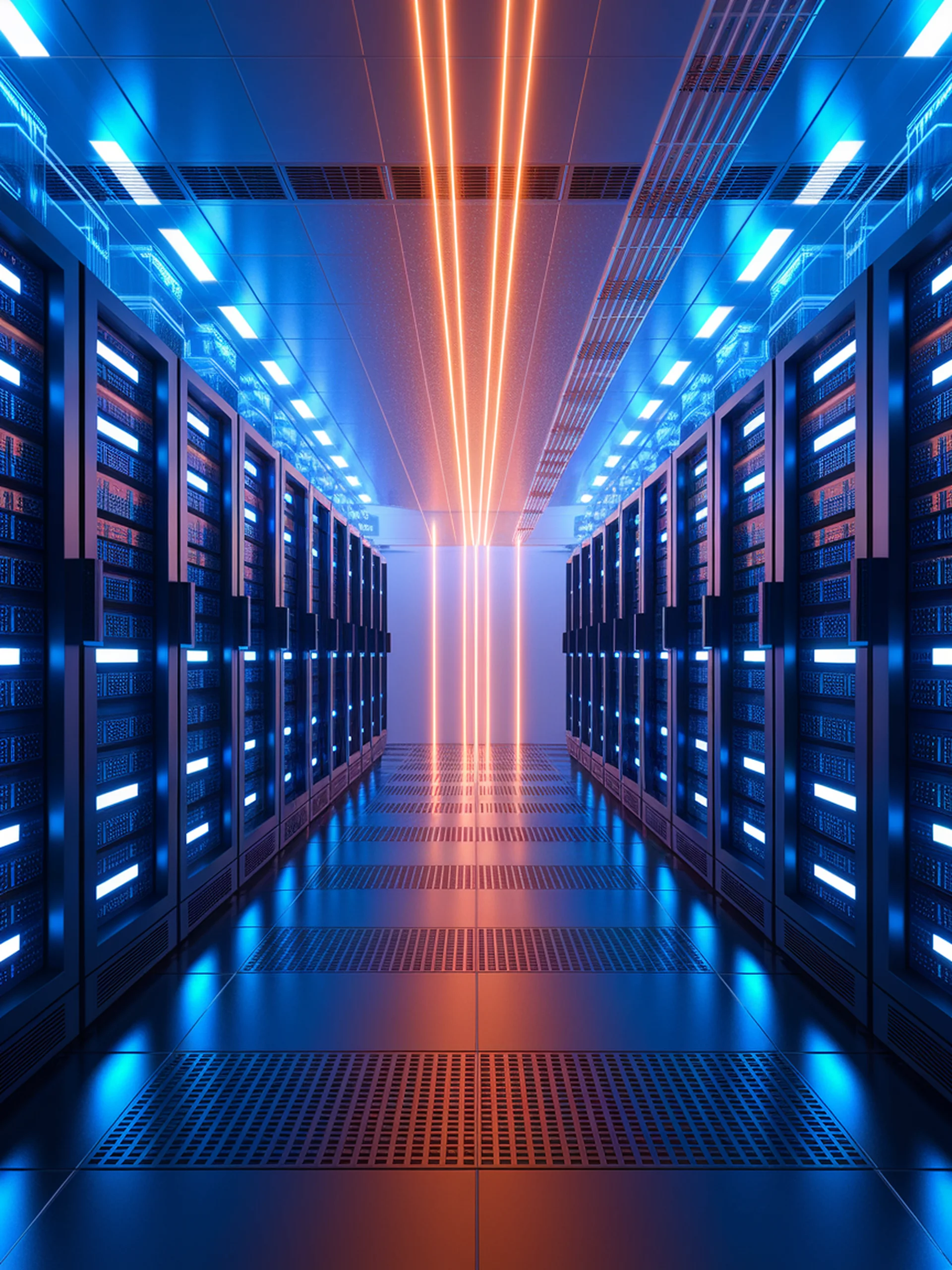
Dynamic LLM Serving Made Efficient
A unified approach to handling variable-length LLM requests
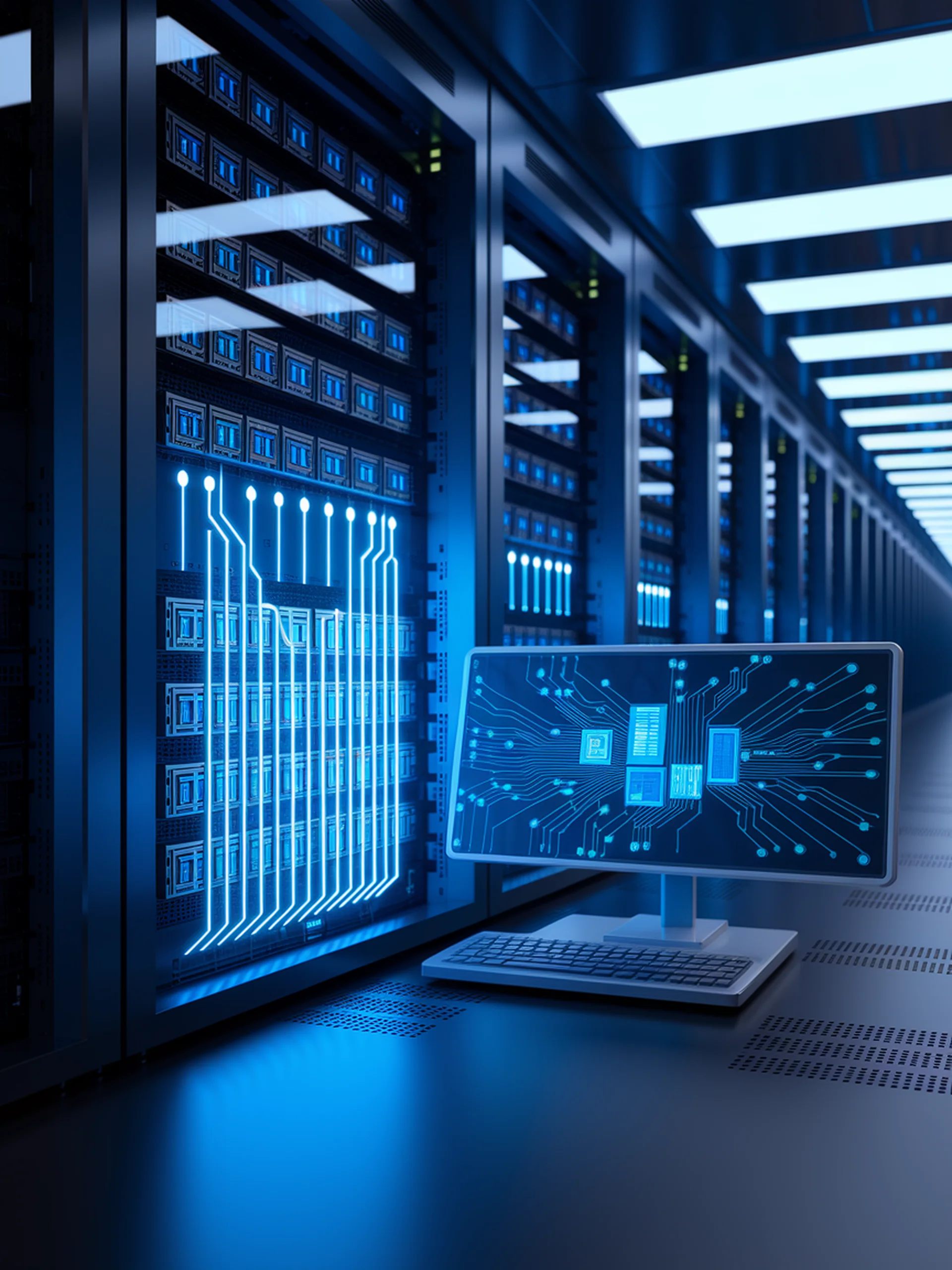
Optimizing MoE LLM Deployment
Maximizing throughput under hardware constraints
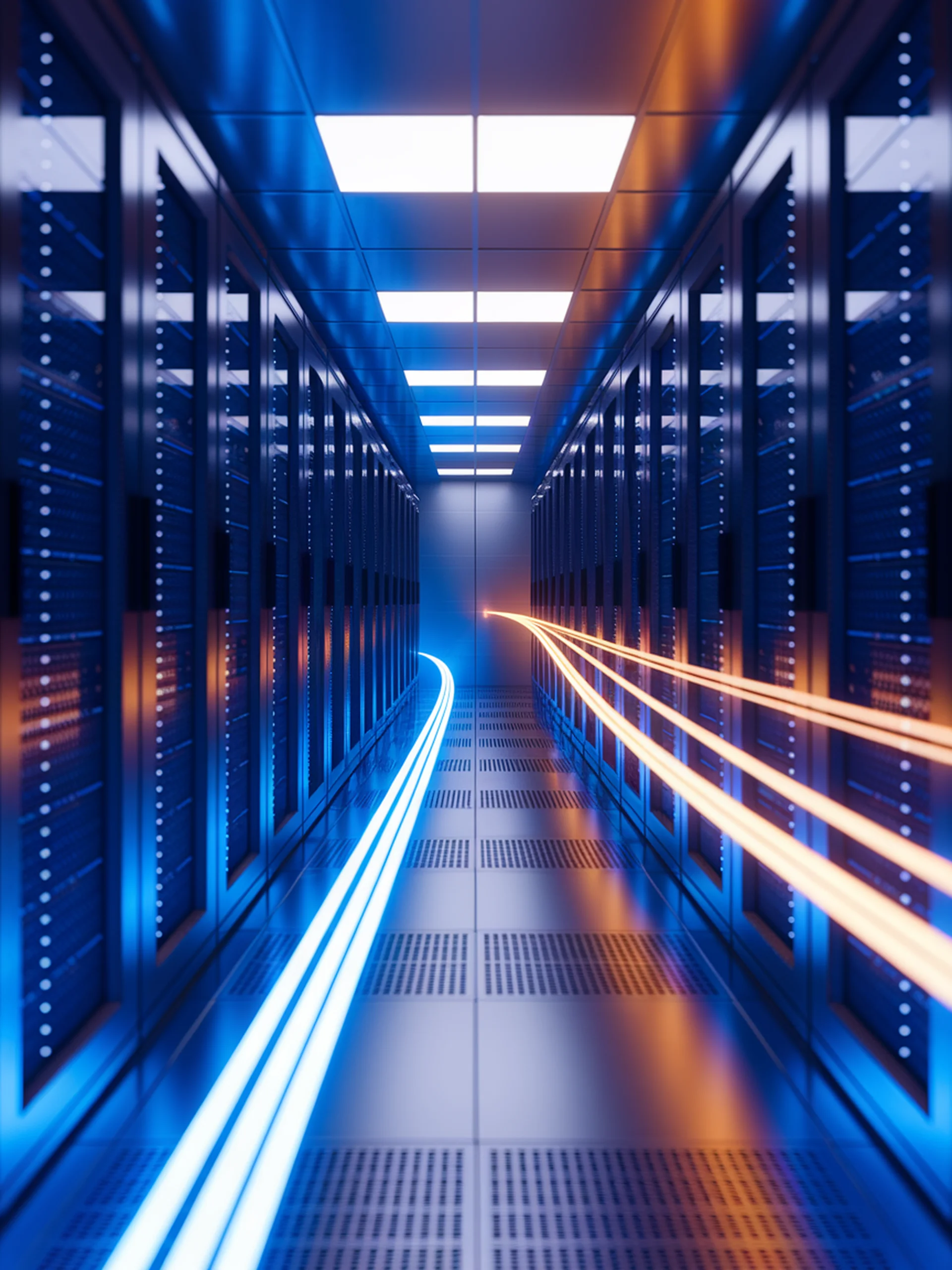
Optimizing LLM Serving for Hybrid Workloads
A novel system for balancing real-time and batch processing requests
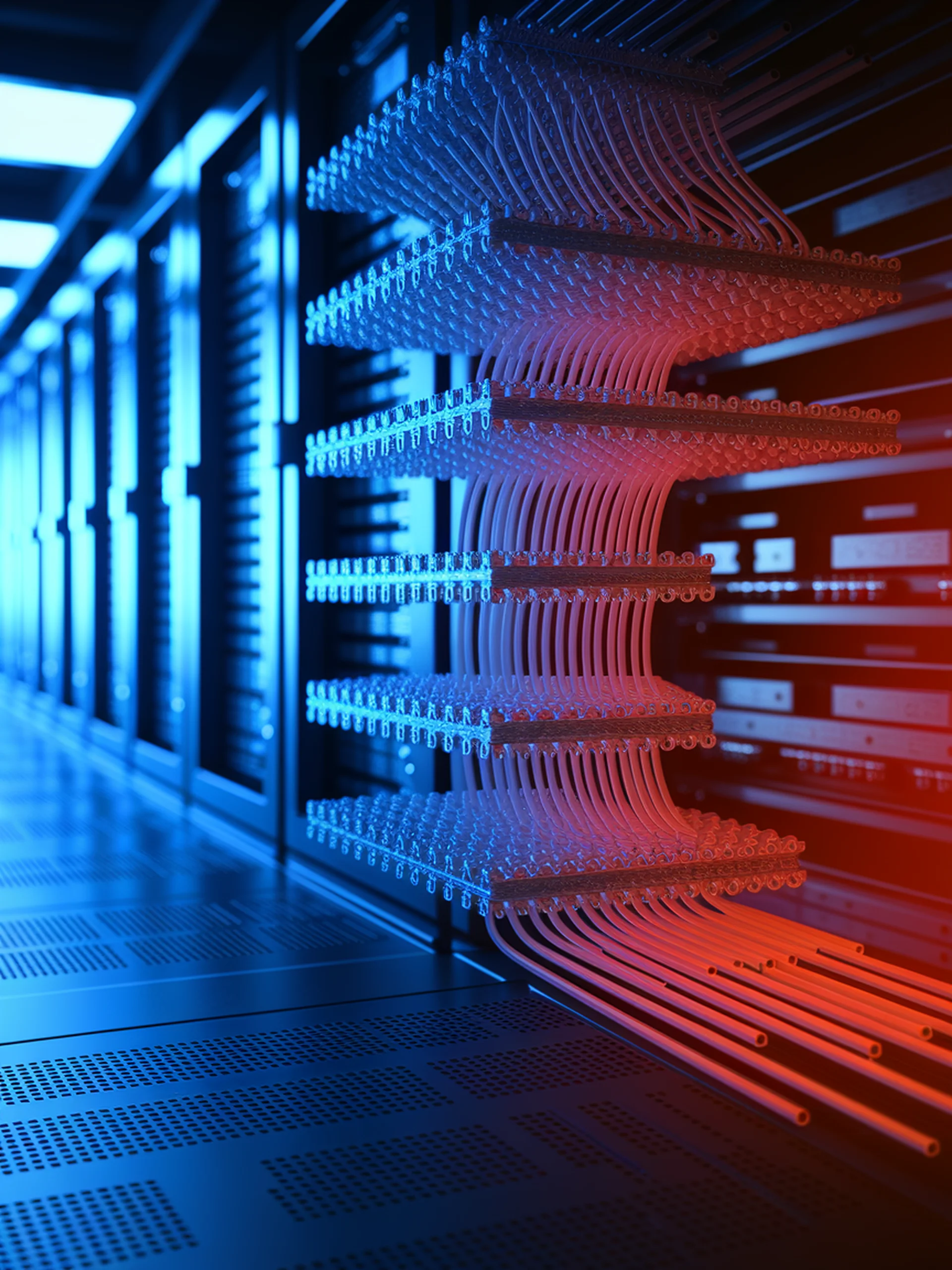
Solving Quantization Error Cascades in LLMs
Addressing the Critical Bottleneck in Model Compression
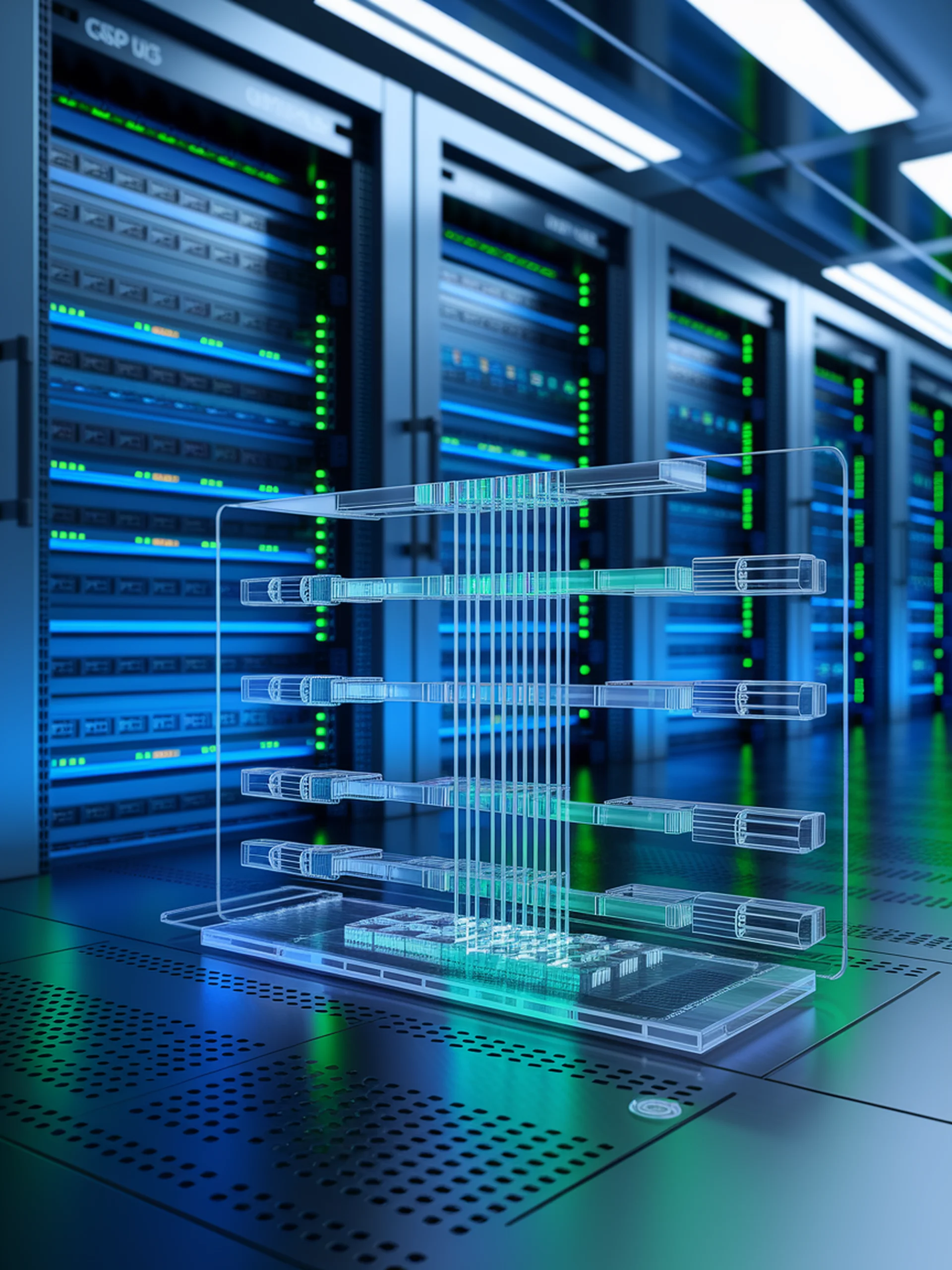
Next-Gen LLM Inference Architecture
Simulating and optimizing multi-stage AI pipelines for heterogeneous hardware
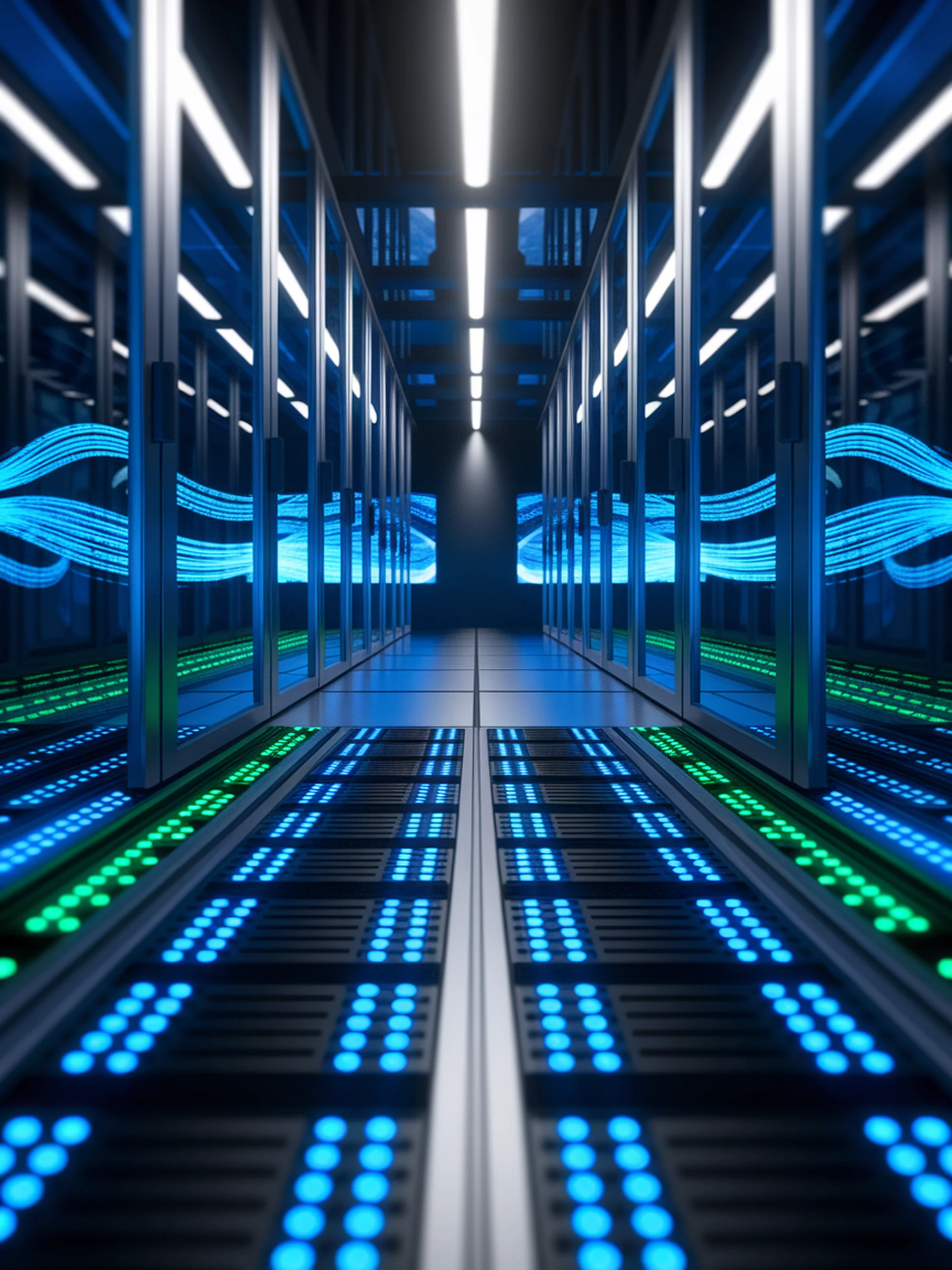
Optimizing LLM Memory: The KeepKV Approach
Achieving efficient inference without sacrificing output quality
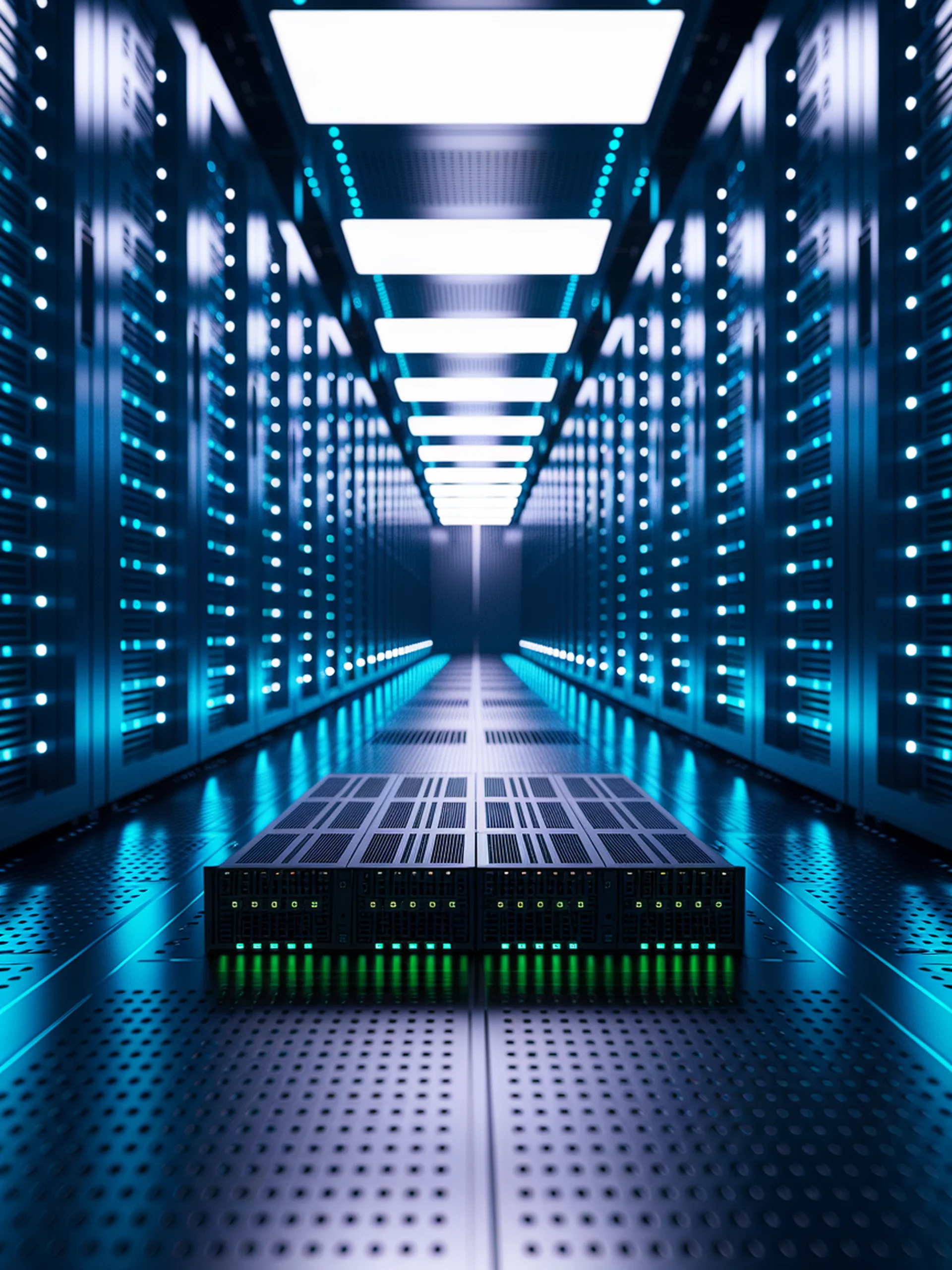
Scaling LLMs on Supercomputers
Lessons from Europe's OpenGPT-X Project
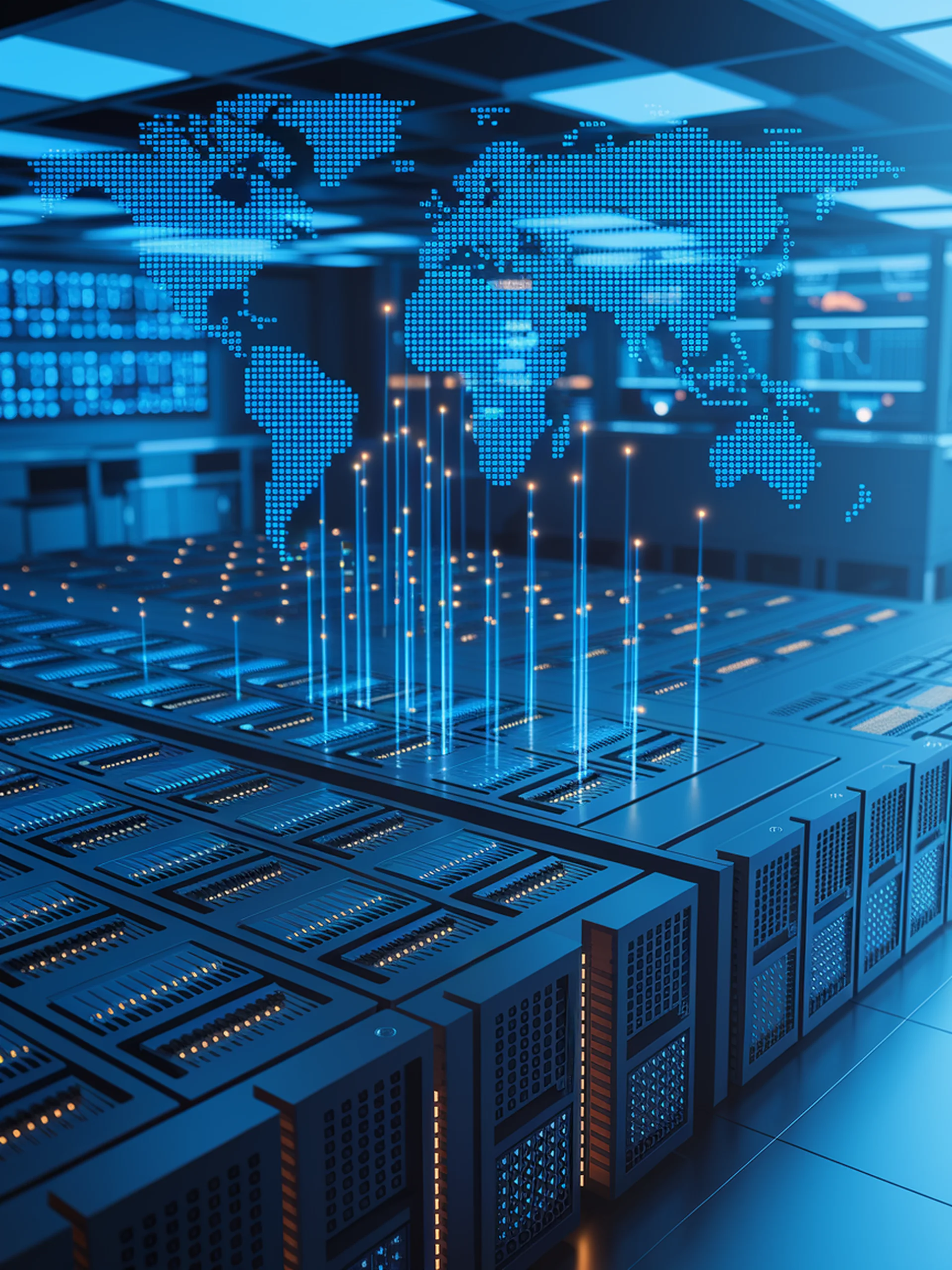
Smart Load Balancing for LLM Inference
Reducing latency in global LLM serving systems through distributed gradient descent
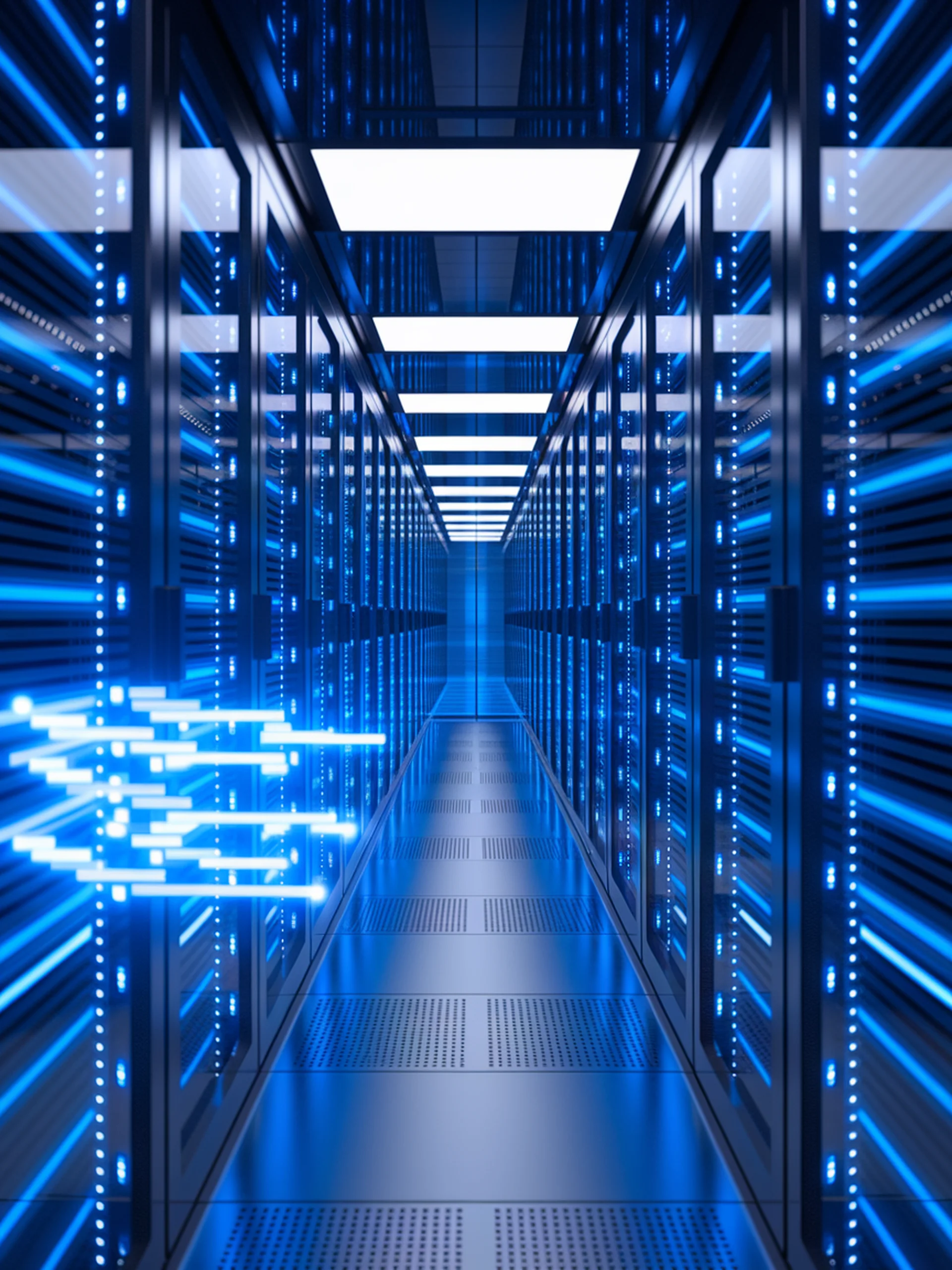
Optimizing LLM Performance with HELIOS
Adaptive Model Selection & Early-Exit Strategies for Efficient AI Deployment
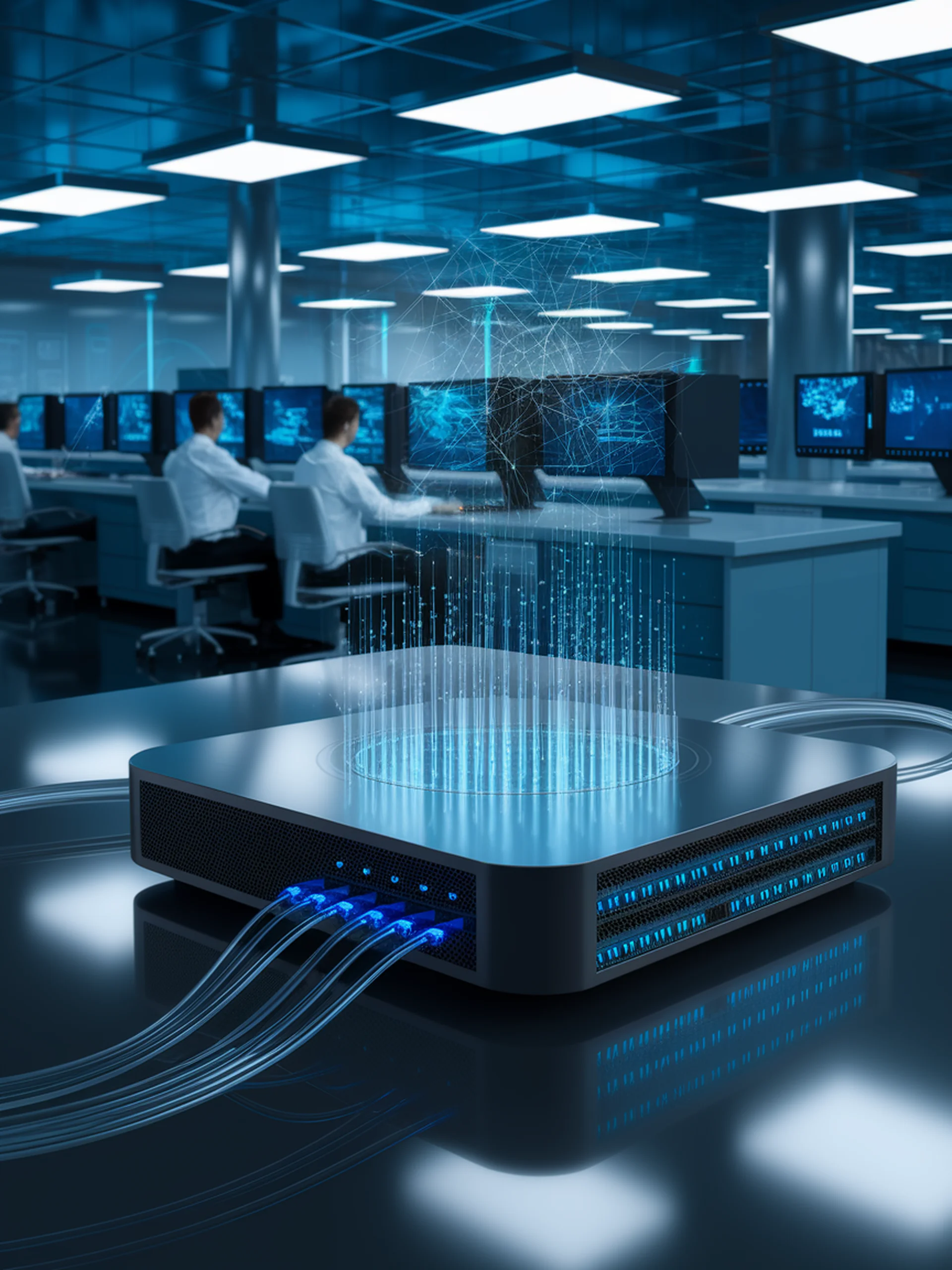
Quantum-Enhanced Attention for LLMs
Reducing computational costs with quantum annealing
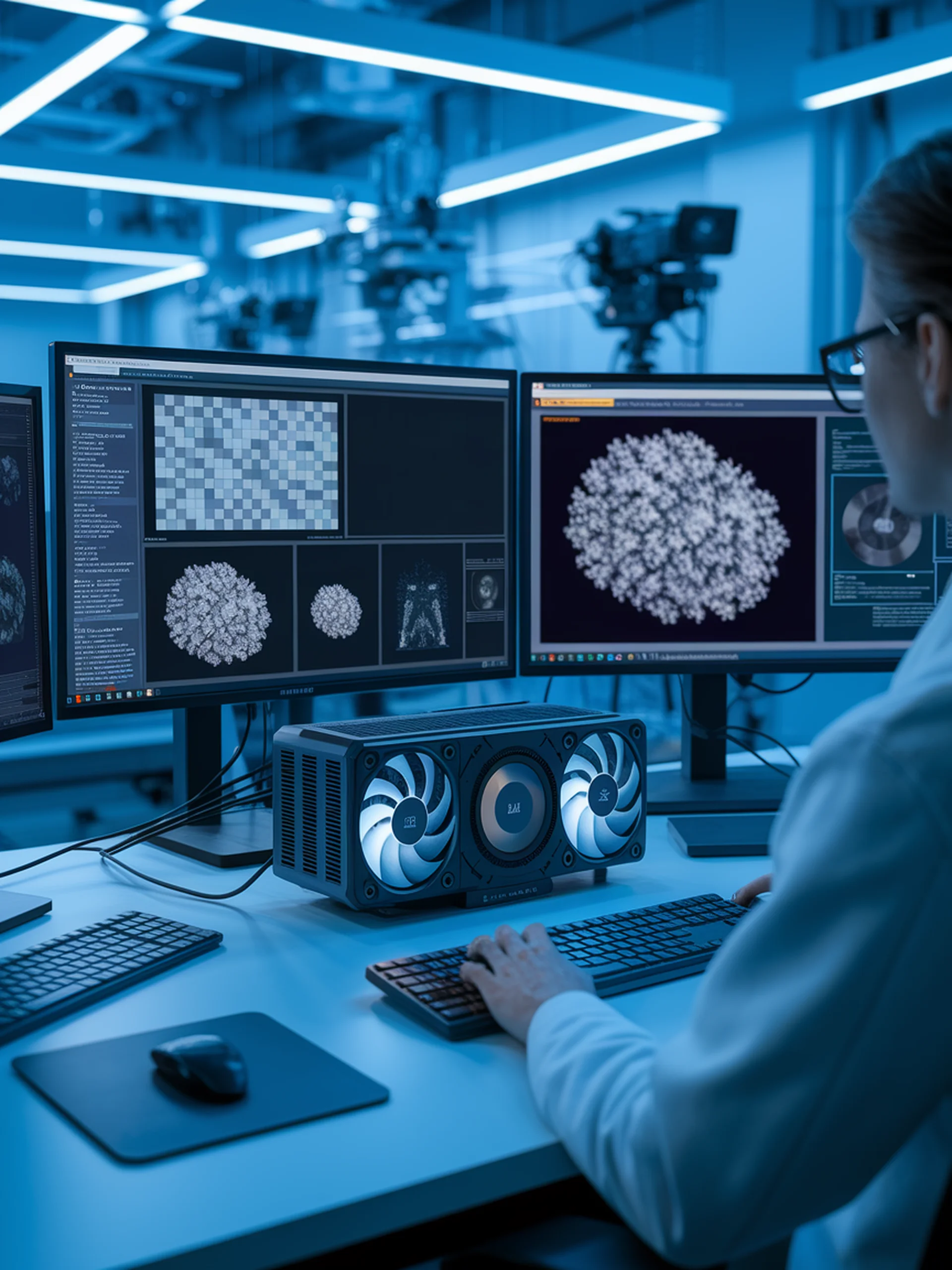
Supercharging Image Quality with Low-Rank Adaptation
Efficient CNN enhancement without expanding model architecture
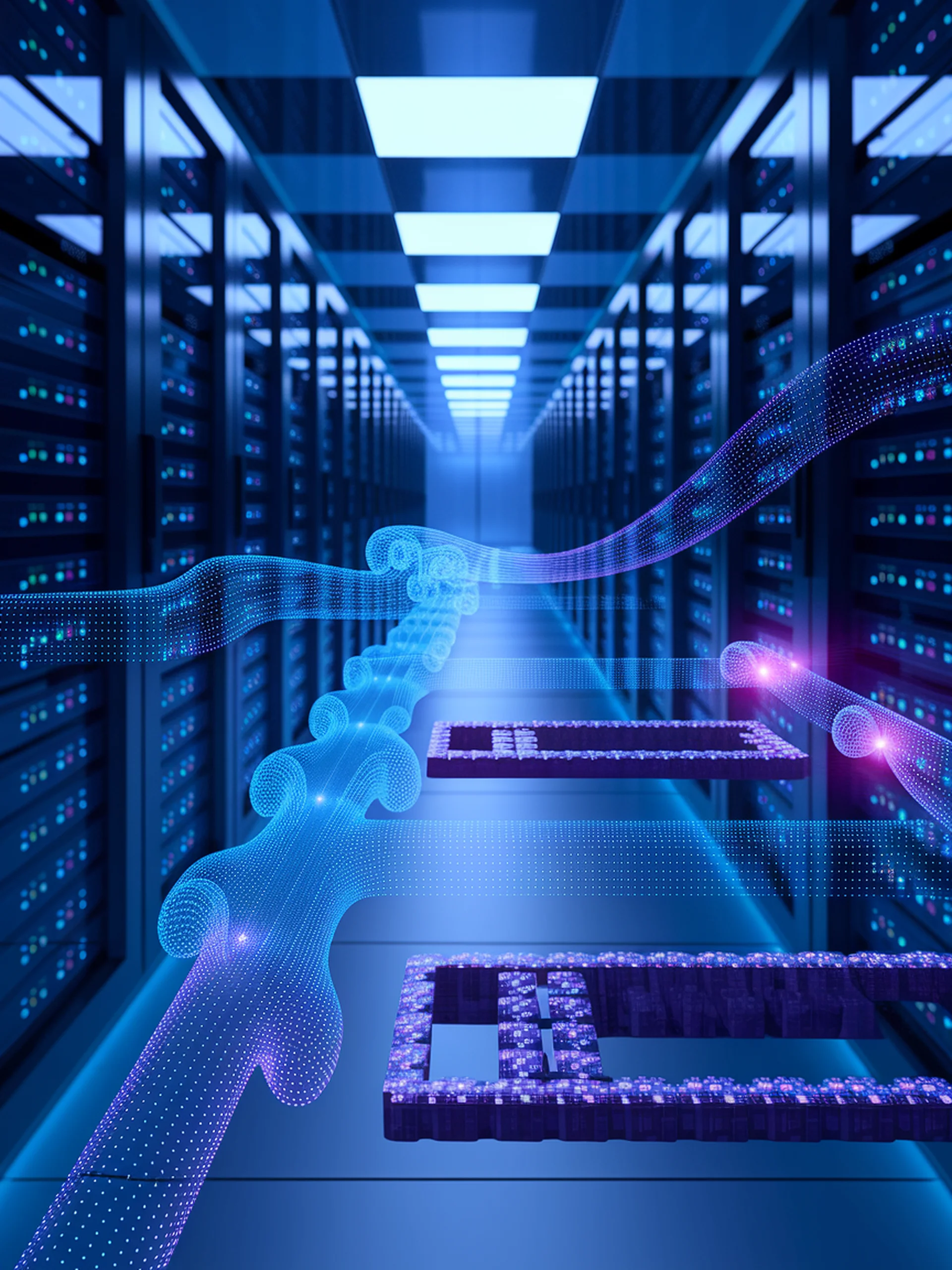
Boosting LLM Performance Under Constraints
A fluid-dynamic approach to optimizing LLM inference with limited memory
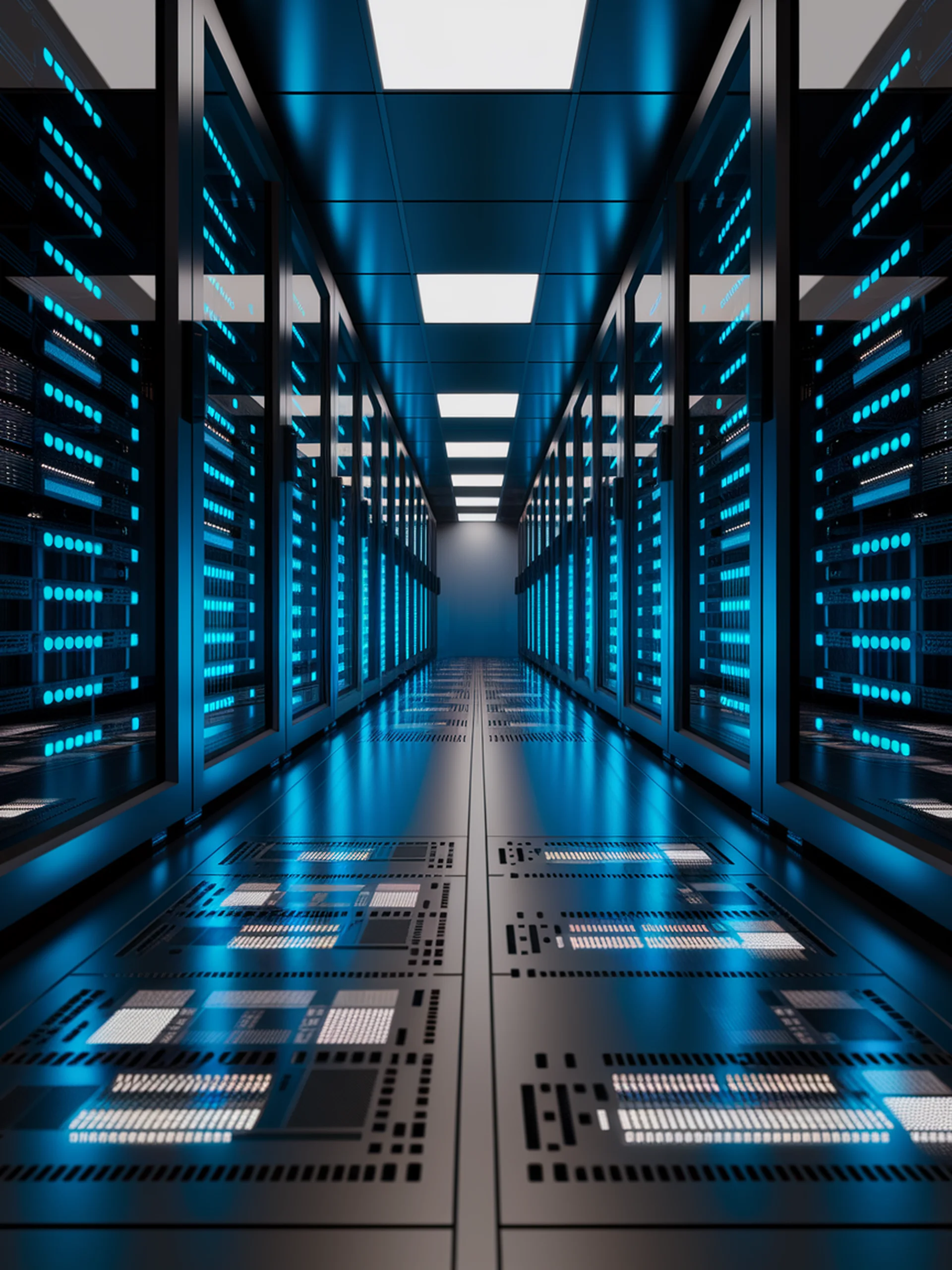
Lossless LLM Compression
30% Size Reduction with Zero Accuracy Loss
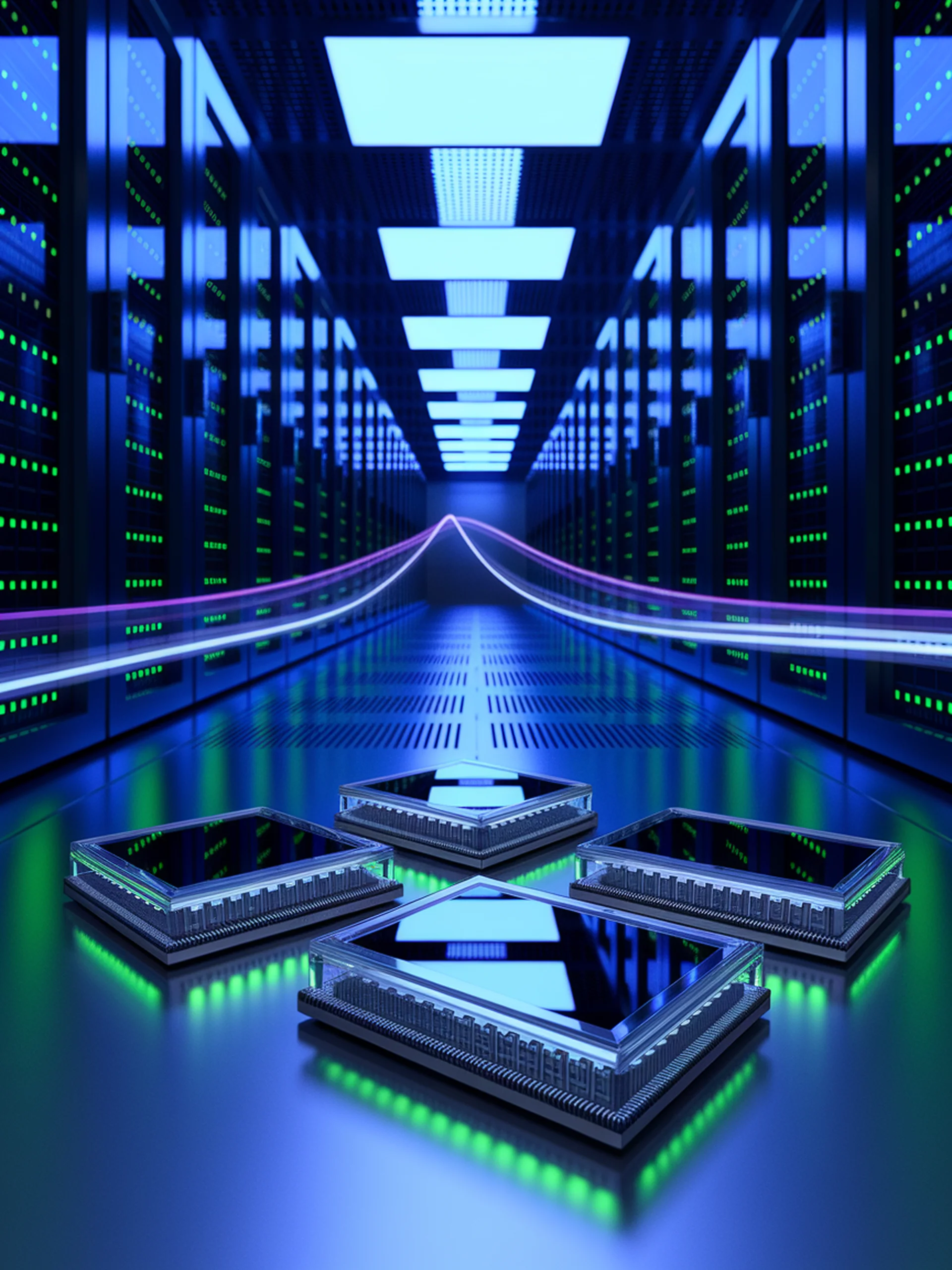
Boosting RAG Performance with Shared Disk KV Cache
Efficient memory management for multi-instance LLM inference
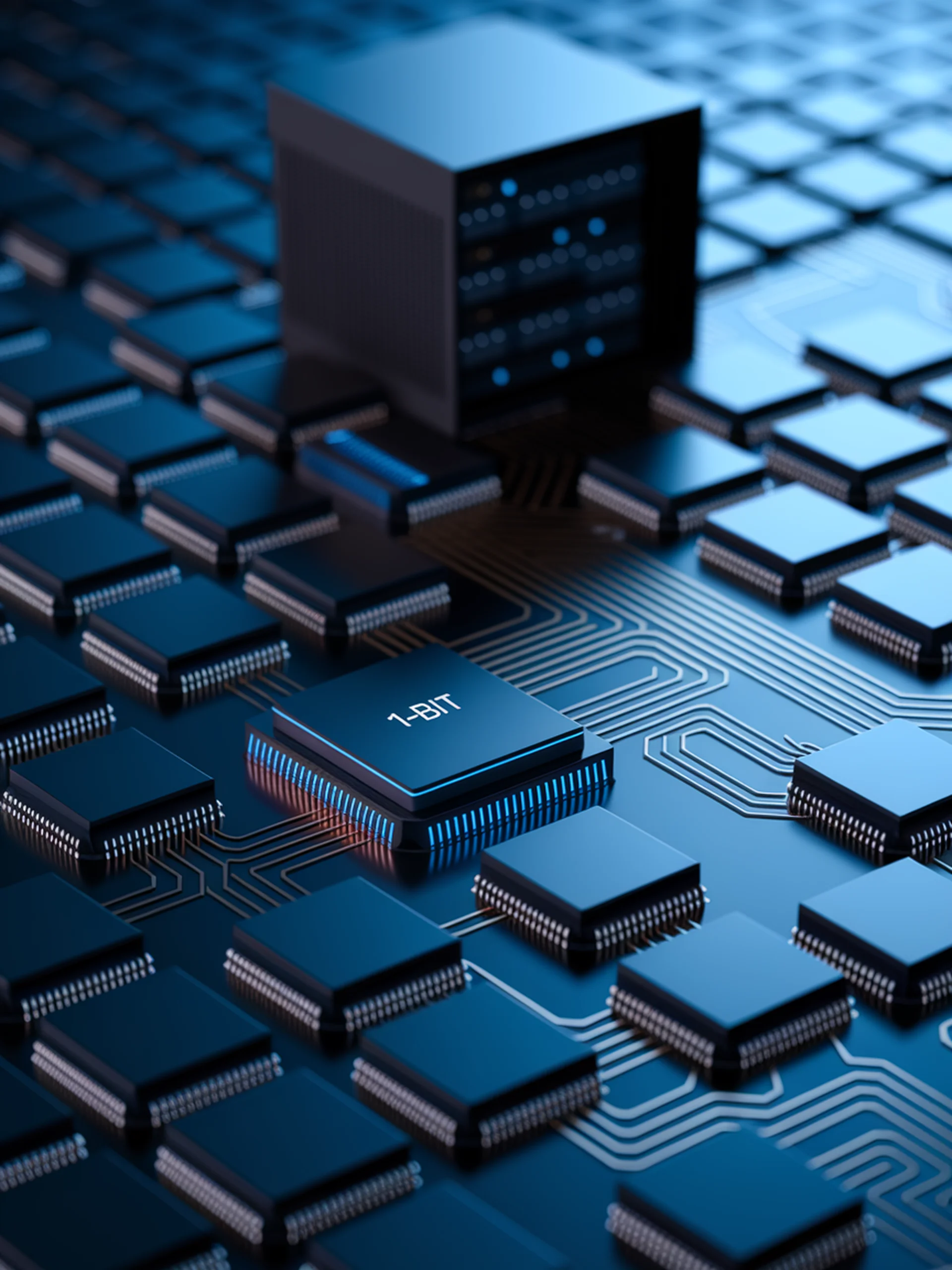